Enhancing employment outcomes for autistic youth: Using machine learning to identify strategies for success
Abstract
BACKGROUND:
The employment rates of autistic young adults continue to be significantly lower than that of their neurotypical peers.
OBJECTIVE:
Researchers in this study sought to identify the barriers and facilitators associated with these individuals’ transition into the workforce to better understand how educators and stakeholders can support students’ post-secondary career plans.
METHODS:
Investigators used a classification tree analysis with a sample of 236 caregivers of autistic individuals, who completed an online survey.
RESULTS:
The analysis identified critical factors in predicting successful employment for respondents 21 years and under and those over 21 years old. These factors included: difficulties in the job search process, challenges with relationships at work, resources used, job maintenance, motivation to work, and the application process.
CONCLUSION:
These findings represent the first use of machine learning to identify pivotal points on the path to employment for autistic individuals. This information will better prepare school-based professionals and other stakeholders to support their students in attaining and maintaining employment, a critical aspect of achieving fulfillment and independence. Future research should consider the perspectives of other stakeholders, autistic individuals and employers, and apply the findings to the development of interventions.
1Introduction
Experts estimate that 50,000 autistic students age out of school services each year and transition to adult life (Centers for Disease Control and Prevention, 2018). While most students with disabilities work towards transition goal(s) related to employment, only 58% of autistic adults reported holding a job in their early twenties (Roux et al., 2017). Research also indicates that autistic individuals report high levels of a desire to work (Hendricks, 2010), yet continue to experience unemployment at significantly higher rates than the general population (Baldwin et al., 2014; Krieger et al., 2012; Nord et al., 2016; Richards, 2012; Roux et al., 2013; Scott et al., 2015; Shattuck et al., 2011). Autistic young adults want to work, and educators can equip them with the skills and experiences necessary to do so effectively in the 21st Century workplace. To assist in this process, researchers, educators and practitioners (e.g., behavior support specialists, employment coaches, other service providers) must better understand what barriers individuals may encounter along the path to employment and what factors help to facilitate obtaining and sustaining work.1
Investigators have found that 35% of autistic young adults have never held a job and approximately half of autistic young adults have worked for pay after high school (Cidav et al., 2012; Roux et al., 2013; Shattuck et al., 2012). Similarly, many autistic individuals have never been part of the workforce nor attend educational programs after high school (Cidav et al., 2012; Roux et al., 2015; Shattuck et al., 2012), and their access to job shadowing, job skills training, job placement support, job coaching, and internship experiences during high school is consistently low (Wagner et al., 2005). These activities not only provide opportunities to gain job skills and experience but also allow the individual to become familiar with the workplace environment and gain confidence in the employee role. Further, employers value past work experience, and adults with disabilities tend to have held fewer volunteer positions, placing them at a significant disadvantage compared to non-disabled applicants. Given these considerations, understanding how pivotal experiences, such as volunteering and internships, positively contribute to the employment outcomes for autistic students is of the utmost importance for educators and family members assisting them in planning for the future (Hurley-Hanson et al., 2020).
Not only are autistic young adults engaging in work-based learning programming and attaining post-secondary employment at lower rates, but they also report lower quality of life outcomes than the general population due to a lack of daytime activities (Taylor & Seltzer, 2011). A survey conducted by Easter Seals (2008) of over 2,500 parents of autistic individuals indicated the quality of their child’s life to be a much deeper concern than for parents of individuals without ASD. Employment is an opportunity for autistic adults to engage in meaningful activities and feel like productive members of society, which leads to a higher quality of life outcomes (Gal et al., 2015; Sheeren et al., 2022).
Previous research suggests that autistic young adults experience significant difficulty in employment and multiple barriers to vocational pathways. Little research has examined the barriers or facilitators that may increase or diminish the likelihood of accessing paid employment. Gathering the perspectives of the parents and caregivers of autistic young adults and the young adults themselves is vital to our understanding of factors enhancing and undermining a student’s ability to obtain and sustain work (Chandroo et al., 2020; Cavendish & Connor, 2018; Sansosti et al., 2017; Snell-Rood et al., 2020). By investigating the critical factors on the path to employment or unemployment, practitioners and educators can design effective and efficient interventions that better prepare autistic students for postsecondary life.
1.1Barriers
According to current literature, autistic individuals are likely to face several barriers to gaining employment. Autistic adults are not likely to use social connections as a support in seeking employment (Baldwin et al., 2014). They report low self-efficacy in their belief in their work abilities (Lorenz et al., 2016), and negative workplace experiences may reinforce these maladaptive beliefs (Heslin et al., 2012). Occupational choice is highly related to one’s beliefs of efficacy, making these experiences discouraging and destabilizing for the individual (Bandura et al., 2001).
Autistic individuals who secure employment also face significant challenges in maintaining employment (Baldwin et al., 2014; Lorenz & Heinitz, 2014; Richards, 2012; Roux et al., 2013). When they encounter conflict or stress at work, autistic adults may quit or miss work without prior notice (Richards, 2012). Perhaps one of the biggest challenges to finding employment for these individuals is navigating the social demands of the workplace (Higgins et al., 2008; Hurlbutt & Chalmers, 2004; Müller et al., 2003). They also are more likely than their neurotypical peers to change jobs frequently and, as a result, endure higher levels of ongoing stress and financial concerns (Baldwin et al., 2014).
After exiting out of their school district, many autistic students use local services to transition to the workplace, such as vocational rehabilitation (VR), supported employment (SE), and customized employment (CE). However, a ten-year analysis using the Rehabilitation Services Administration database uncovered that 62% of autistic clients did not achieve employment (Alverson & Yamamoto. 2017). A significant difference between those who did obtain employment and those who did not gain employment was the number of services used.
1.2Facilitators
Stakeholders must understand the variables related to successful employment outcomes to develop and implement effective interventions. Researchers have found several areas that may be related to positive results. For example, independent living skills such as independent means of transportation, autonomy, and ability to make decisions are highly correlated with favorable employment outcomes for autistic young adults (Mazzotti et al., 2016; Zalewska et al., 2016). Additional research points to inclusion in the general education setting, previous work experience (paid or unpaid), parent involvement with education, high expectations, social skills, and vocational training as critical factors in attaining employment for autistic young adults (Mazzotti et al., 2016). Roux et al. (2013) found that the odds of ever having a paid job were higher for those who were older, from higher-income households, or who had better conversational or functional skills.
Further, when thinking about the job search and application process, employer practices are crucial to successful employment outcomes (Lindsay et al., 2019). Facilitators in the work environment may include improving the employment process by breaking it up into stages, adjusting the environment for the interview, or assessing the specific skills related to the job- rather than a traditional interview (Lindsay et al., 2019).
Though current and past literature shed light on factors that facilitate and pose barriers to obtaining and retaining employment for autistic youth, more research is needed to assist all stakeholders in implementing evidence-based interventions for students transitioning into the workforce. If professionals can better understand the factors facilitating and barricading access to employment, they can more effectively leverage and address these variables and improve vocational outcomes for autistic youth.
1.3Current Study
Researchers designed this study to understand the barriers and facilitators that affect the employment of autistic individuals in order to assist educators and stakeholders in constructing more effective supports and interventions for students transitioning into the workforce. Primary investigators utilized a decision tree methodology to provide a unique perspective on the path to employment. This project focused on autistic youth who were able to obtain employment and the associated factors that led to paid employment. Specifically, this study aims to answer the following research question: What pivotal points exist on the pathway to employment for autistic individuals? These data will allow educators and practitioners to create and implement more impactful employment interventions for transition-aged autistic youth.
2Method
2.1Survey development
Researchers administered an online survey to sample a large population across a sizable geographic region. They designed the survey instrument in many stages, with multiple goals in mind. By conducting a review of the literature and identifying related studies involving various stakeholders (e.g., Newman et al., 2011; Erickson et al., 2014), the team developed a basic framework and a list of questions. Primary authors then constructed the survey instrument using the framework of a three-pronged career process based on Parsons’ (1909) trait-and-factor theory of vocational development. Parsons posited that one’s vocational decisions relied heavily on three areas: awareness of one’s skill sets, knowledge of viable employment opportunities, and the ability to align one’s skill sets to these opportunities. With this theoretical basis in mind, the structure included: (1) early career aspirations and developmental preferences (i.e., skills and interests), (2) training and preparation for work (i.e., requirements of work), and (3) working life (i.e., finding employment). The survey contained questions about the potential obstacles that interrupt this process and the facilitators that may improve outcomes. Researchers then determined common obstacles by identifying difficulties that these young adults had faced during their progression through the three steps. Authors purposefully grouped the questions into categories for later data organization and analysis.
Throughout survey construction, primary authors also consulted multiple groups for suggested revisions. These groups included: administrators from community service provider agencies, school district and university personnel and two local parent support groups. The individuals provided informal feedback about content and readability. Another staff member volunteer, who identified as autistic took the survey in a meeting with the authors by reading aloud and answering each question on the computer and verbally. The volunteer asked questions for clarification and delivered feedback throughout the process. To garner insights from the familial perspective, a parent of an autistic individual evaluated the survey in a similar exercise. The research team discussed each question and all feedback provided from consulting parties and reviewed for readability as well as survey structure. The final instrument consisted of 37 questions, some of which required responses to related sub-questions, and took approximately 20 minutes to complete online. After obtaining Institutional Review Board approval, the research team finalized the survey instrument in the electronic survey platform (Qualtrics), which generated a unique resource locator (URL).
2.2Study sample
A total of 378 respondents submitted the online survey. Of those respondents, 279 were considered caregivers, and 26 were the young adults themselves. Although the survey was directed to both caregivers and young adults, we, unfortunately, did not receive enough responses from young adults to analyze the data and hope to gather further data and conduct further analyses in a future study.
Of the caregiver respondents, 271 answered our target question: Has the young adult ever been employed and earned money? The analysis data set was filtered using the following inclusion criteria. Investigators narrowed down the original sample of 378 submitted surveys to the 271 respondents who indicated whether the young adult had ever held a paying job, which served as the target variable. Next, they included only the 261 surveys about young adults over the age of 15. They further subsampled their final dataset to the 236 surveys about young adults with an ASD diagnosis. All statistics detailed in the results section will correspond to the final dataset of 236 surveys.
2.3Data collection
To access a broad range of caregivers and young adults who may be in and out of the high school setting, investigators requested assistance in recruitment efforts from the county regional center, two local parent/caregiver support groups, and a community mental health service agency that supports transitional-aged autistic youth. The research team selected these groups based on the population they serve, the services they provided, as well as each organizations’ expressed willingness and interest in participating. Each of these communities resided in California at the time of the study. Participating agencies and support groups agreed to send out an email invitation to their clientele. They also shared this information across their social media channels. The research team provided agencies with an invitation text describing the study and a URL survey link to send to their established contacts. Recipients of the survey invitation included caregivers or family members who are knowledgeable about the pre-employment and employment experiences of autistic individuals. Those eligible to participate included the autistic person, their parent(s), or another caregiver(s). As we did not receive enough responses from autistic individuals to effectively analyze the data, we focused this study on the parents and caregivers of such individuals. Respondents did not receive monetary compensation for their participation. The survey link remained active and open for a total of 12 weeks. The investigators could not calculate the percentage of returns because the survey did not include an individual identifier, and it is possible that further distribution occurred through original groups (e.g., word of mouth, text and email forwarding).
By utilizing a traditional analytical process, the researchers had no knowledge of who was responding, only the demographic categories that the respondents provided. Primary authors collected quantitative and qualitative data via the survey, although the only reported quantitative data here. The team obtained quantitative data primarily through forced-choice or ranking questions. Authors used standard survey nomenclature for most questions (e.g., Likert scales) and included a short text box for several questions allowing respondents to provide a more detailed response. (Dillman et al., 2014).
2.4Data analysis
Researchers in this study utilized machine learning to help predict a path for autistic young adults to obtain a paying job successfully. Although there are more contemporary algorithms, such as deep neural network architectures, these models are “black boxes” and do not provide insight into how the prediction is made. First introduced by Breiman, Friedman, Stone, and Olshen (1984), classification and regression tree (CART) models offer a clear and easily understandable explanation for why a specific prediction was made for a provided input. For the purposes of this work, decision trees have several appealing properties over more basic statistical techniques. They are robust to outliers, are able to capture non-linear relationships among independent and dependent variables, and simultaneously provide a quantitative measure of variable importance in the model along with a human-readable set of criteria (i.e., rules) that specify the model. This allows users to easily apply the technique and consumers of research to easily understand the outcomes by both domain and non-domain experts.
The goal of a decision tree is to correctly separate a sample population into homogeneous subgroups, such that following the tree from its root to its leaves provides the decision criteria to classify a specific data point. This study utilized a classification tree to assign an individual into one of two categories: having been paid for employment or never having been paid for employment. The classification tree predicts the likelihood of reaching the target value, along with a clear path that leads to the decision. The tree is recursively constructed by posing a sequence of logical if-then conditions from independent variables with the answers’ determining the next condition, if any. These conditions are known as splits in the tree. At each node, the model creates a set of possible splits for each predictor variable and chooses the variable which will generate the greatest purity (i.e., homogeneity) in the resulting child nodes (i.e., nodes below the split). The variables used to make the splits indicate a significant contribution to the prediction of the target variable. To better understand those contributors, researchers considered variable importance measures. Regardless of whether they appear in the final tree construction, the program ranked all variables with a variable importance measure. Breiman et al. (1984) provides a description of the CART algorithm and methodology. Statistically, the CART algorithm produces a model consisting of optimal discriminants for predicting the classification target from the provided training data. A key advantage is that the resulting model is human-readable in the sense that traversing the tree corresponds to applying a set of if-then rules learned by the model
A classification tree begins with a primary (i.e., root) node consisting of the entire sample (i.e., first subgroup). Each independent variable is explored as a way to split the parent node into child nodes (i.e., subgroups), using a goodness of split based on a purity measure, for example, information entropy, that corresponds to classification accuracy. The variable with the greatest predictive power will be used to split the nodes. This process repeats until a pre-specified limit is reached or until splitting no longer improves the model. Child nodes with no splits are known as terminal nodes. Terminal nodes correspond to the final classification of the group– in this case, whether or not the individual had ever held compensated employment.
Two common impurity measures are information gain based on the decrease in entropy and the Gini impurity index. The algorithm considers two types of splits when constructing a classification tree: primary and surrogate. Variables that yield the highest purity measure after splitting a node are the primary splits that appear in the final tree construction (see Fig. 1). The variable importance measure, however, will include the variables with the top-five highest purity measures for each primary split. Surrogate splits are a method for handling missing data and are used to continue down a path when the primary split is missing. Meaning, if the respondent didn’t answer the survey question used to make the primary split in the tree, the model would use their response to the question about the surrogate split to decide which path to follow. Surrogate splits never appear in the final tree construction. However, the variable importance measure will include the five variables that can mimic the primary splits at a given node. Regardless, if the variable appeared in the tree that may be surrogate split, the variable importance measure generally has a positive correlation with predictive power on the target variable.
Fig. 1
Classification decision tree.
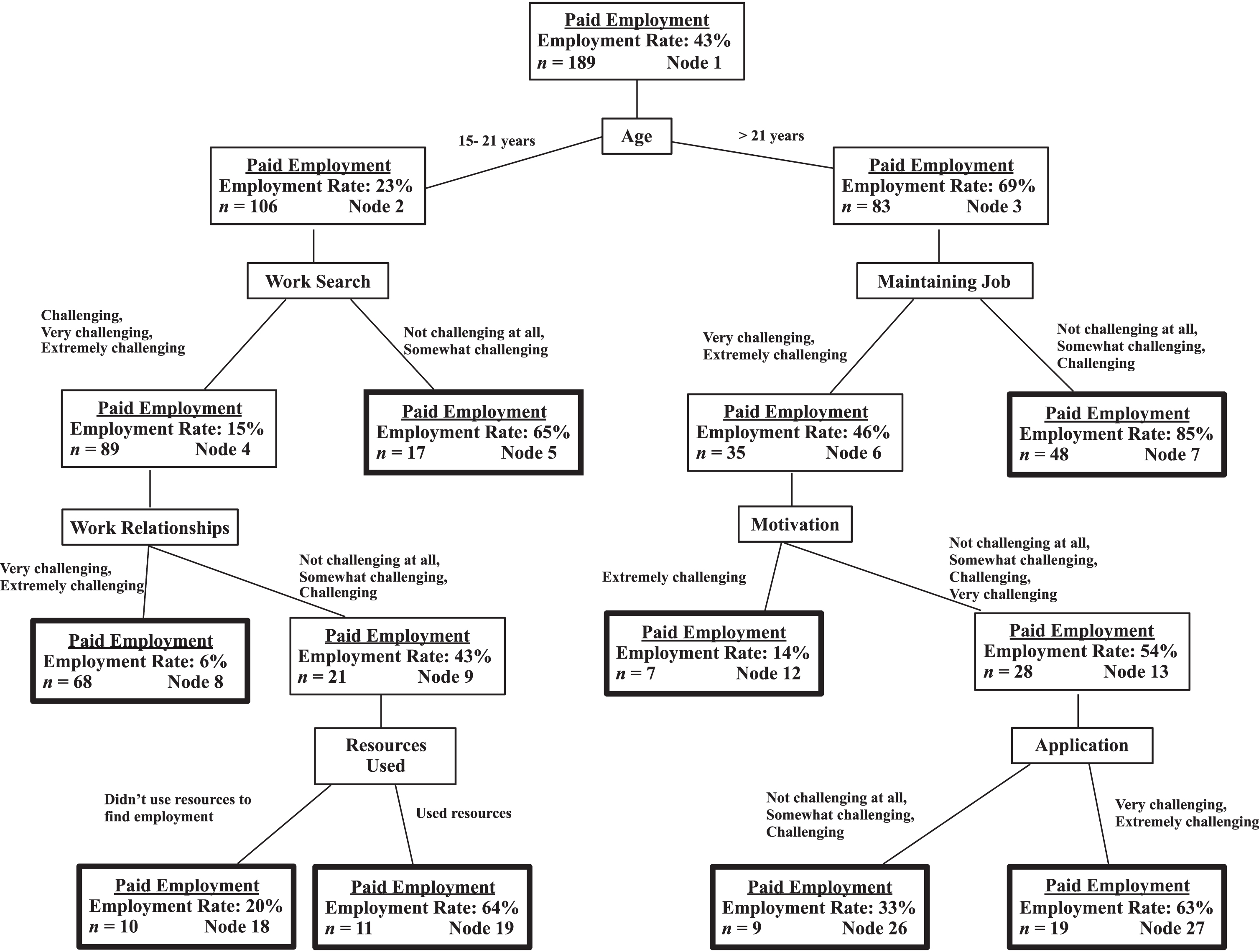
Researchers use classification trees in a variety of studies, including the investigation of employment factors for individuals with disabilities (Catalano et al., 2006; Chan et al., 2011; Chang et al., 2006). Sima et al. (2015) also used this methodology to evaluate employment risk factors for youth with disabilities, and by Hyde et al., (2018) to predict employer recruitment of autistic individuals. We expand on this previous work here by using this machine learning approach to identify the facilitators and barriers surrounding the transition of autistic individuals into the workplace, quantify their importance, and provide an interpretable set of rules to predict placement in a compensated position.
3Results
3.1Study sample demographics
Respondents reported data across a distribution of ages. For those included in our final sample, data reflected information about young adults between the ages of 15 and 17 years old (20%), 18 to 21 years old (38%), 22 to 25 years old (25%), 26 to 29 years old (11%), and over 30 years old (6%). A majority of respondents (57%) indicated only an ASD diagnosis, while the remaining respondents reported at least one comorbid diagnosis. The survey participants included primarily people who identified as mothers, and the sex of the autistic individual the respondent described in the survey was predominantly male (78%). To gather information about the individual’s level of “functioning,” authors integrated a survey item based on the same descriptions of “support required” as outlined in the Diagnostic and Statistical Manual of Mental Disorders, 5th edition (DSM-5; American Psychiatric Association, 2013). The majority of the survey outcomes indicated the young adult needed the minimum level of “support” described. See Table 1 for specific data regarding demographic characteristics.
Table 1
Demographic characteristics of survey respondents and individual of focus (n = 236)
Variable | Frequency | Percentage |
(n) | (%) | |
Comorbid Diagnoses | ||
Autism Spectrum Disorder Only | 134 | 57 |
Intellectual Disability | 30 | 13 |
Communication/Speech/Language | 33 | 14 |
Attention Deficit | 40 | 17 |
Specific Learning Disorder | 18 | 8 |
Mental Health Disorder | 42 | 18 |
Other disorder | 18 | 8 |
Role | ||
Mothers | 177 | 75 |
Another Relative | 33 | 14 |
“Other” Type of Caregiver | 26 | 3 |
Sex of Autistic Individual | ||
Male | 184 | 78 |
Female | 52 | 22 |
Level of Support Required | ||
Individual needs support | 107 | 46 |
Individual needs substantial support | 82 | 35 |
Individual needs very substantial support | 47 | 20 |
3.2Data analysis
Authors created 28 discrete numeric predictor variables using 44 of the survey questions and sub-questions. This set included the majority of the survey questions unless the item did not have a large enough n to assume that the responses were generalizable, or there was no natural ordering in the response options to create an ordered numeric variable, which needed for the model. After performing Pearson’s chi-square test between the predictor and target variables, 19 variables (see Table 2 for variables and associated survey questions) were independent, using α= 0.05 as the significance level (Bender & Lange, 2001).
Table 2
Variable name and survey items
Variable | Associated Survey Item |
Age | Age of the young adult. |
Support | In general, the young adult requires: support, substantial support, very substantial support. (choose one) |
Volunteer | Did the young adult participate in volunteer work? Length of time that the young adult has volunteered. |
High school | Is the young adult currently in high school? If not, did the young adult graduate from high school with: high school diploma, certificate of completion? (choose one) |
Outside classes | Did the young adult participate in lessons or classes outside of high school? |
Job interest | Has the young adult expressed interest in pursuing a specific job or career? |
Hobbies | Did the young adult participate in hobbies? |
Work understanding | How realistic is the young adult’s general understanding of the world of work? |
Resources used | In the past 6 months, which of the following has the young adult used in seeking employment? (select from 11 options) |
Resume | How challenging is developing a resume for the young adult? |
Work experience | How challenging is gaining relevant work experience for the young adult? |
Skills match understanding | How challenging is understanding the match between skills and job for the young adult? |
Work search | How challenging is searching for work for the young adult? |
Applications | How challenging is completing application materials for the young adult? |
Interviewing | How challenging is interviewing for the young adult? |
Maintaining job | How challenging is maintaining a job for the young adult? |
Work relationships | How challenging is managing interpersonal nature of professional relationships for the young adult? |
Motivation to work | How challenging is motivation to work for the young adult? |
Work environment | How challenging is finding a work environment that is supportive of youth with special needs for the young adult? |
Using the Gini index impurity measure (Breiman et al., 1984), primary authors constructed a classification decision tree (Fig. 1), using the 19 independent variables to predict a young adult’s paid employment status. To avoid overfitting, the team trained the model using 80% of the data, hereinafter referred to as the training sample. They then tested on the remaining 20%, while preserving the overall class distribution for the target variable. The proportion of target responses was consistent in the training, testing, and overall sample. Investigators created the training and testing sets randomly, and no duplicate data points exist across them. The training sample, used to create the model, was completely independent of the data used to test the model’s accuracy (i.e., the testing sample) to minimize the chance of overfitting. Researchers determined the depth of the tree by minimizing the misclassification rate, and they employed the surrogate method to handle any missing predictor variables.
The authors reported the overall frequency, employment rate, and variable importance for each of the 16 predictor variables with a variable importance measure of at least one, or rounded to one, in Table 3. The table also includes similar statistics for each variable level.
Table 3
Variable importance (N = 236)
Variable | n | Employed n (%) | Importance |
Age | 20.21 | ||
15–17 years | 48 | 4 (8%) | |
18–21 years | 89 | 23 (26%) | |
22–25 years | 60 | 37 (62%) | |
26–29 years | 26 | 19 (73%) | |
30+ years | 13 | 12 (92%) | |
Work search | 10.81 | ||
Not challenging at all | 10 | 9 (90%) | |
Somewhat challenging | 26 | 20 (77%) | |
Challenging | 35 | 16 (46%) | |
Very challenging | 47 | 18 (38%) | |
Extremely challenging | 100 | 22 (22%) | |
High school | 9.03 | ||
Not in high school | 4 | 1 (25%) | |
In high school | 81 | 10 (12%) | |
Certificate of completion | 60 | 25 (42%) | |
Diploma | 90 | 59 (65%) | |
Maintaining job | 5.96 | ||
Not challenging at all | 31 | 25 (81%) | |
Somewhat challenging | 26 | 18 (70%) | |
Challenging | 44 | 20 (45%) | |
Very challenging | 41 | 8 (20%) | |
Extremely challenging | 71 | 15 (21%) | |
Work relationships | 5.62 | ||
Not challenging at all | 7 | 6 (86%) | |
Somewhat challenging | 27 | 19 (70%) | |
Challenging | 35 | 21 (60%) | |
Very challenging | 49 | 16 (33%) | |
Extremely challenging | 98 | 23 (23%) | |
Motivation to work | 3.66 | ||
Not challenging at all | 52 | 31 (60%) | |
Somewhat challenging | 44 | 15 (34%) | |
Challenging | 40 | 21 (53%) | |
Very challenging | 32 | 16 (50%) | |
Extremely challenging | 48 | 3 (6%) | |
Applications | 2.87 | ||
Not challenging at all | 20 | 13 (65%) | |
Somewhat challenging | 37 | 21 (57%) | |
Challenging | 44 | 22 (50%) | |
Very challenging | 38 | 12 (32%) | |
Extremely challenging | 77 | 17 (22%) | |
Resources used | 2.55 | ||
Did not utilize | 118 | 29 (27%) | |
Utilized | 115 | 63 (55%) | |
Job Interest | 2.36 | ||
Did not express interest | 84 | 23 (19%) | |
Expressed interest | 152 | 72 (47%) | |
Work understanding | 2.23 | ||
Not at all realistic | 62 | 15 (24%) | |
Somewhat realistic | 122 | 42 (34%) | |
Moderately realistic | 38 | 26 (68%) | |
Extremely realistic | 14 | 12 (86%) | |
Volunteer | 2.11 | ||
Not challenging at all | 141 | 44 (31%) | |
Somewhat challenging | 19 | 9 (47%) | |
Challenging | 27 | 13 (49%) | |
Very challenging | 31 | 16 (52%) | |
Extremely challenging | 18 | 13 (72%) | |
Skills match understanding | 2.00 | ||
Not challenging at all | 17 | 12 (71%) | |
Somewhat challenging | 34 | 19 (56%) | |
Challenging | 43 | 19 (44%) | |
Very challenging | 54 | 21 (39%) | |
Extremely challenging | 70 | 14 (20%) | |
Work Experience | 1.42 | ||
Not challenging at all | 9 | 8 (89%) | |
Somewhat challenging | 28 | 18 (64%) | |
Challenging | 40 | 23 (58%) | |
Very challenging | 54 | 20 (37%) | |
Extremely challenging | 84 | 15 (18%) | |
Hobbies | 1.35 | ||
Did not participate | 116 | 36 (31%) | |
Participated | 120 | 59 (49%) | |
Support | 1.35 | ||
Support | 108 | 61 (56%) | |
Substantial support | 82 | 24 (29%) | |
Very substantial support | 46 | 10 (22%) | |
Resume | 0.85 | ||
Not challenging at all | 27 | 21 (78%) | |
Somewhat challenging | 30 | 13 (43%) | |
Challenging | 43 | 22 (51%) | |
Very challenging | 33 | 13 (39%) | |
Extremely challenging | 86 | 17 (20%) |
The research team classified terminal tree nodes into three categories. Those with employment rates at least 10% lower than the overall sample of 40% were considered below average, those at least 10% above the overall sample were considered above average, and other terminal nodes were considered comparable to the overall average. Researchers then performed data management and analysis using the software environment R (R Core Team, 2018), and constructed the classification tree t using the R package rpart (Therneau, 2018).
3.3Descriptive results
Of the 236 sample individuals, 95 (40%) had at least one instance of paid employment. The three groups with the highest employment rates were those individuals over the age of 30 (92%), and those who did not find searching for work (90%) or gaining work experience (89%) challenging at all. Upon examining the individuals over the age of 30, 92% were male, and most required support (46%), or substantial support (38%), with only 15% requiring very substantial support. All members of this group earned a high school diploma (77%) or certificate of completion (23%). Surprisingly, only 23% found searching for work not challenging at all, 15% found it somewhat challenging, and 54% found it very challenging or extremely challenging. Only 69% found the motivation to work not challenging at all or somewhat challenging; however, 54% found it very or extremely challenging to manage the interpersonal nature of professional relationships. Maintaining a job was not challenging (15%), somewhat challenging (23%), or challenging (31%) for most over the age of 30, while only 23% found it very or extremely challenging. Completing application materials was not challenging or somewhat challenging for 46%; 15 % found it challenging, and 23% found it very or extremely challenging.
The group with the lowest employment rate (6%) involved those who found it extremely challenging to be motivated to work. Within this group, 33% were aged 15–17, none of whom had been employed for pay, volunteered, or used available resources to search for employment in the past six months. Those aged 15–17 in this group almost always (94%) responded very or extremely challenging to questions about the barriers to finding a job, and all are currently in high school. The majority (44%) of respondents who found it extremely challenging to be motivated to work were aged 18–21 and also had no instances of paid employment. They responded extremely challenging 91% of the time to questions on the barriers to finding a job. Most individuals in this group between 18–21 years old were still in high school (76%), while the rest had earned a certificate of completion (19%) or a diploma (5%). The remaining respondents (23%) who found it extremely challenging to be motivated to work were aged 22–29 years old and had a paid employment rate of 27%. All in this group, aged 22–29, found it extremely challenging to be motivated to work and find a work environment that supports youth with special needs. One individual (9%) was not enrolled in or completed high school, one was currently enrolled (9%), 45% earned a certificate of completion, and 27% received a high school diploma. Most found it extremely challenging to maintain a job (55%), while the remaining respondents reported it challenging (18%) and very challenging (18%). Completing the application materials was not at all challenging for 27%, challenging for 9%, very challenging for 9%, and extremely challenging for 55% of individuals in this subgroup. No individuals over 30 years old found it extremely challenging to be motivated to work.
3.4Decision tree model
The decision tree had a total of 15 nodes, seven terminal nodes, seven splits, and four levels. The model predicted employment status with 93% accuracy and 85% specificity on the testing data set (20% of the overall sample). All of the following statistics pertaining to the nodes of the decision tree were based on the training sample of 289 surveys (80% of the overall sample). The data indicated that 45% of the individuals used to train the model were in the two terminal nodes with below-average employment rates (6%, 14%, 20%), while 56% of the individuals were in the four terminal nodes with above-average employment rates (63%, 64%, 65%, 85%). The one terminal node with an employment rate (33%) comparable to the overall average accounted for 5% of the individuals.
One of the benefits of using a classification tree is it removes human bias and splits the data into groups using mathematical formulas. This type of model splits the data into smaller and smaller groups by identifying patterns that can help make predictions. In this case, the variable that provided the most predictive power for having paid employment was age; we call this the primary split of the tree. Not only does the model identify age as an important variable, but it also determined, mathematically, that splitting the data at age 21 would provide the most accuracy in the prediction. The primary split of our classification tree was on the age of the individuals. Participants aged 21 or less (Node 2) had one-third of the employment rate of those over the age of 21 (Node 3) with rates of 23% vs. 69%. Those who found it challenging, very challenging, and extremely challenging to search for work were used as a split for those younger than 21 in Level 2 (Nodes 4, 5) with employment rates of 15% and 65%, respectively. Those who found it very or extremely challenging to maintain a job were considered for splitting criteria in Level 2 for those over 21 (Nodes 6, 7) with respective employment of 46% and 85%. One could infer that those in the younger group had less opportunity and practice to search for work, which may pose a challenging obstacle to future employment. It is also possible that those over 21 years old have been able to experience the work search process for some years. These individuals have had jobs but may struggle to maintain their job once obtained.
For those 21 and under, Level 3 splits using the challenges of maintaining work relationships (Nodes 8, 9), with respective employment of 6% for those who find it very or extremely challenging and 43% for those who find it not at all challenging, somewhat challenging, or challenging. Navigating relationships in the professional setting can be particularly overwhelming for autistic individuals. Some individuals may require additional skills training and supports once they obtain employment. The challenge of being motivated to work provides the split in Level 3 for those over 21 (Nodes 12, 13). There is a 14% employment rate for those who find it extremely challenging and a 54% employment rate for those who found it less than extremely challenging to be motivated to work. Individuals who are not motivated to work are unlikely to seek employment or present as a viable candidate to a potential employer.
Level 4 considers whether a young adult has used resources to seek employment in the past six months (Nodes 18, 19) as the splitting criteria for those over 21. This split generates an employment rate of 20% for those who did not use resources to seek employment and 64% for those who did. Although this may seem obvious, accessing the available resources may be a primary goal when developing a transition-to-work plan. For young adults over 21 years old, Level 4 splits on the challenges of completing application materials (Nodes 26, 27). Surprisingly, those who found it very or extremely challenging to complete application materials had an employment rate of 63%. Those who found it not challenging, somewhat challenging, or “just” challenging had an employment rate of 33%. Below, the authors describe the decision tree terminal nodes grouped by employment rate classification.
Below-average employment rates
Node 8. This node contained 68 individuals (36% of the training sample) age 21 and younger who found it challenging, very challenging, or extremely challenging to search for work. They also found it very or extremely challenging to maintain work relationships. This group had an employment rate of 6%, which was the lowest in the model.
Node 12. This node contained seven individuals (4% of the training sample) over the age of 21 who found it very or extremely challenging to maintain a job as well as extremely challenging to be motivated to work. This group had an employment rate of 14%.
Node 18. This node contained ten individuals (5% of the training sample) under 21 years old who found it challenging, very challenging, or extremely challenging to search for work. They responded that it was not challenging, somewhat challenging, or challenging to maintain work relationships. They did not use any resources to seek employment. This group had an employment rate of 20%.
Comparable employment rates
Node 26. This node contained nine individuals (5% of the training sample) over 21 years old who found it very or extremely challenging to maintain employment. The motivation to work was less than extremely challenging. Moreover, it was not challenging, somewhat challenging, or challenging to complete the application process for this group and they had an employment rate of 33%.
Above-average employment rates
Node 27. This node contained 19 individuals (10% of the training sample) over the age of 21, who found it very or extremely challenging to maintain employment. They did not find it extremely challenging to be motivated to work and found it very or extremely challenging to complete the application process. This group had an employment rate of 63%.
Node 19. This node contained 11 individuals (6% of the training sample) under 21 years old who found it challenging, very challenging, or extremely challenging to search for work. They found it not challenging, somewhat challenging, or challenging to maintain work relationships, and did use resources to seek employment in the past six months. This group had an employment rate of 64%.
Node 5. This node contained 17 individuals (9% of the training sample) age 21 and younger who found it not challenging or somewhat challenging to search for work. This group had an employment rate of 65%.
Node 7. This node contained 48 individuals over the age of 21 (44% of the training sample) who found it not challenging, somewhat challenging, or challenging to maintain employment. This group had an employment rate of 85%, and the highest employment rate in the model.
4Discussion
The results of this study support many of the conclusions in related studies regarding barriers and facilitators to employment. However, this particular data set and analysis helped identify pivotal points of intervention on the path to paid work. These results also allow educators and stakeholders to consider transitioning to post-secondary life in two unique and distinct ways: early preparation of youth under 21 years old for employment and the employment experiences of those who over 21 years old. The decision tree analysis indicated that age was an important marker in thinking about and planning for employment. Given the split results, the authors discuss findings in these two areas.
Youth under 21 years old tended to have lower employment rates than the older group. These rates make sense, as the individuals likely have had fewer opportunities for employment and perhaps supporting stakeholders (e.g., parents, teachers, counselors, etc.) have placed less emphasis on employment during their adolescent years. The search for work is a clear indicator of employment. If the individual found it difficult to search for work, they were less likely to be employed than if the individuals found it easier to search for work. This finding reaffirms the importance of explicit instruction on the job search process and the skills required of prospective positions as a potential area of intervention (Rowe et al., 2018).
Moreover, the perception of difficulty associated with developing and maintaining relationships in the workplace is a strong indicator of future employment. For many autistic individuals, interacting with other individuals, particularly those in an unfamiliar environment, can feel intimidating and overwhelming. Concerns about workplace interactions could significantly impact employment outcomes and, as a result, stakeholders should assist in the development of interpersonal skills within job preparedness programming. As indicated in the literature, through volunteer work and other on-site opportunities, youth can gain real-world experience and practice skill application in authentic workplace environments. On-site experiences also allow for the development of job-related soft skills, building confidence, and gaining an understanding of the world of work (Bellman et al., 2014). Learning how to develop workplace relationships during an internship or volunteer position might make it easier to navigate the social dynamics of a workplace later. Promoting pro-social relationships amongst peers in the classroom is also an opportunity to practice interacting with “colleagues” in a structured environment, where students feel supported and can receive timely feedback on skill application. Educators can also supplement these skills by providing lower stakes classroom activities that allow for continued practice and reinforcement of social skills required of students in work-based learning experiences. Helping youth under 21 gain experience through volunteer work, strengthening work-related social skills through instructional activities in the classroom setting, and developing their job search skills is crucial to enhancing employment outcomes (Walsh et al., 2018).
For respondents over 21 years old, maintaining a job was an essential marker in the employment rates of these individuals. This outcome indicates that although practitioners invest much time in the job search and attainment of skills, it is essential to focus on building the appropriate skills and support for maintaining employment. Identifying critical vocational skills and relevant academic skills and providing ample practice and instruction on those skills could improve a student’s ability to maintain work (Bartholomew et al., 2015). An Individualized Education Plan (IEP) team can also provide information and resources on community-based services to older students and their support networks to continue building skills once they have aged-out of the public school system.
Motivation for work was a crucial indicator of employment success. Specifically, those who reported it extremely challenging to be motivated to work had low rates of employment (14%). There are many potential reasons that someone would feel unmotivated or present as unmotivated. Investigators hypothesize, perhaps that individual had multiple experiences of failure during his or her school years. Alternatively, it is possible that the struggle to gain employment led to participants giving up the pursuit entirely. Individuals may be avoiding the work experience due to fear of failure, or related mental health conditions, such as anxiety or depression.
Classroom educators should consider integrating various strategies to cultivate and sustain motivation as a necessary part of pre-vocational skill-building. Actively participating in their IEP meeting is one way transition-aged autistic youth can develop skills related to motivation, such as self-efficacy. Educators can assist in this process by holding a pre-IEP meeting in which the student has time to review their IEP and discuss with their case carrier how they plan to contribute to the meeting (Martin et al., 2004; Cavendish et al., 2017). Collaboration with and referrals to school- or community-based psychological service providers can also assist autistic youth for whom lack of motivation is a result of more significant mental health issues.
The job application experience was also an essential factor in predicting employment outcomes. As reported above, those who viewed the application process as very or extremely challenging were more likely to be employed. Perhaps those who were unsuccessful in obtaining a job or had not spent much time on the application process would not view it as important or challenging. Alternatively, some of those individuals may not have completed an application before and did not expect it to be a challenging task. With these factors in mind, special education teachers and vocational specialists can include learning objectives and goals aligned to application processes (e.g., interviewing strategies, completing application paperwork, crafting a resume, etc.) to bolster student preparedness.
Notably, variable importance measures, whether or not they appear on the decision tree, are good indicators of employment rates. Here, the authors outline some of the variables with the highest power in predicting paid employment to provide a sense of student needs and tools for educators and practitioners to consider when assisting youth in planning for employment. The following variables were indicators for the entire training sample but may be more helpful to some individuals than others regarding obtaining viable employment: the work search process, job maintenance, professional relationships, applications, and utilization of resources. All of these variables appear in the literature; however, using variable importance measures can help school communities and stakeholders know where to start. Given these pivotal points along the path to paid employment, educators can prioritize and develop educational opportunities aligning to the training and experiential needs of those entering today’s workforce.
A strength of this study is the research teams’ approach to data analysis. To our knowledge, this study is the first use of this analysis method to further understand employment barriers and facilitators from the perspective of caregivers of autistic individuals. Those conducting disability research are increasingly using classification trees to better understand the factors that influence eligibility, services, and outcomes (Chan et al., 2006; Kolakowsky-Hayner, 2010; Hurley et al., 2008). The classification tree methodology highlights key variables that pertain to helping to prepare individuals for post-secondary life, and, in turn, improved employment outcomes. This approach indicates that these variables do not affect employment in a linear, additive fashion. Instead, relevant variables behave in a manner that is suggestive of a complex, highly interactive framework. Understanding this framework is necessary to propose effective interventions for individuals who are preparing for employment.
4.1Implications for future research and practice
There are several implications for future research and practice when considering the results of this study. By identifying subpopulations of youth with disabilities who are more at-risk for poor employment outcomes based on an analysis of modifiable characteristics researchers, educators and other practitioners can create more targeted interventions. For example, researchers and educators can develop tools to assist in the work search process. These tools could help individuals gain the skills needed to reduce feelings of being overwhelmed or intimidated by the process of searching for work. Specifically, this information yielded from this study allows for educators and transition specialists to thoroughly assess the needs and support the skill development of groups at increased risk of future employment difficulties, by considering traits associated with vocational challenges.
This study is the first phase in understanding the potential path to paid employment for autistic individuals who are preparing to enter the workforce or currently in the job search process. Gathering information from caregivers of autistic youth allows supporting parties to gain a deeper understanding of the needs of students with disabilities and, in turn, more effectively advocate for equitable vocational opportunities. Obtaining additional data (i.e., follow-up survey items, interviews, focus groups, etc.) from varied respondents to contextualize the results of the present study might allow researchers and practitioners to better understand the role and impact of certain variables such as diminished motivation amongst autistic youth. Additionally, we must understand the perspectives of the other key stakeholders (e.g., autistic individuals, employers, educators). Future research would benefit from collecting more perspectives from a larger national or international sample to expand our understanding of what constitutes effective post-secondary preparation. Once these perspectives are understood, stakeholders can then use these data to enhance preparation and intervention programs in schools, post-secondary institutions, and the workplace.
4.2Limitations
There are several limitations researchers and educators should consider when reviewing this study. The authors chose decision tree analysis because it clarifies how the model develops the predictions that allow researchers and readers to understand the career path more clearly. Nevertheless, this analysis is more susceptible than other types of analyses to changes in data. For decision tree analysis, the more individuals one has, the more accurate and stable the analysis becomes. Although this study’s sample size was adequate to run these analyses, in the future, obtaining more responses would provide a more stable model.
Further, due to the sample size, the research team had to limit the number of variables used to avoid overfitting the model (i.e., to make the results more generalizable). Finally, the target variable used did not account for the individual’s success once employed; instead, it concerned initially acquiring employment. Thus, future research should address issues of employment experiences and maintenance once employed. Other considerations could include designing additional survey questions to allow respondents to elaborate on traits that tend to be more nebulous and varied across individuals (e.g., motivation). Obtaining additional qualitative information would help to contextualize the results of the decision tree analysis as themes related to common response patterns amongst various groups could be identified.
5Conclusion
Although many studies have identified the same barriers and facilitators as potential issues related to employment confirmed in this study, the unemployment rates of autistic individuals continue to remain unacceptably low. There has not been a study that looked at these factors through decision tree analysis. This process allows users to identify variables that are most impactful on an individual’s path to employment. While many stakeholders are working together to improve employment outcomes for autistic individuals daily, our systems of support are stressed, and the challenges are overwhelming. All parties need a clearer picture of priorities to ensure efficiency and effectiveness in our approach to improving employment. This study is an initial start in building a better understanding of where to make the most pivotal and impactful changes to our intervention process.
Acknowledgments
None to report.
Conflicts of interest
None to report.
Ethics statement
This study was approved by the Institutional Review Board at Chapman University (Approval Number: 1617H091).
Funding
This study was funded by The Thompson Policy Institute on Disability (no funding number assigned).
Informed consent
All participants were presented with a written document containing required information and consent was obtained from each participant prior to completion of the survey.
Data availability
The data that support the findings of this study are available from the corresponding author, AJG, upon reasonable request.
References
1 | Ali, M. , Schur, L. , Blanck, P. ((2011) ) What types of jobs do people with disabilities want? Journal of Occupational Rehabilitation 21: (2), 199–210. https://doi.org/10.1007/s10926-010-9266-0 |
2 | Alverson, C. Y. , Yamamoto, S. H. ((2017) ) Employment outcomes of vocational rehabilitation clients with autism spectrum disorders. Career Development and Transition for Exceptional Individuals 40: , 144–155. https://doi.org/10.1177/2165143416629366 |
3 | American Psychiatric Association. ((2013) ). Diagnostic and statistical manual of mental disorders. 5th ed. Author. https://doi.org/10.1176/appi.books.9780890425596 |
4 | Anthony, W. A. , Blanch, A. ((1987) ) Supported employment for persons who are psychiatrically disabled: An historical and conceptual perspective. Psychosocial Rehabilitation Journal 11: (2), 5–23. https://doi.org/10.1037/h0099582 |
5 | Baldwin, S. , Costley, D. , Warren, A. ((2014) ) Employment activities and the experiences of adults with high-functioning autism and Asperger’s disorder. Journal of Autism and Developmental Disorders 44: , 2440–2449. https://doi.org/10.1007/s10803-014-2112-z |
6 | Bandura, A. , Barbaranelli, C. , Caprara, G. V. , Pastorelli, C. ((2001) ) Self-efficacy beliefs as shapers of children’s aspirations and career trajectories. Child Development 72: , 187–206. https://doi.org/10.1111/1467-8624.00273 |
7 | Bartholomew, A. , Papay, C. , McConnell, A. , Cease-Cook, J. ((2015) ) Embedding secondary transition in the common core state standards. Teaching Exceptional Children, 329–335. https://doi.org/10.1177/0040059915580034 |
8 | Bellman, S. , Burgstahler, S. , Ladner, R. ((2014) ) Work-based learning experiences help students with disabilities transition to careers: A case study of University of Washington Projects. Work, 48: 399–405. https://doi.org/10.3233/WOR-131780 |
9 | Bender, R. , Lange, S. ((2001) ) Adjusting for multiple testing— When and how? Journal of Clinical Epidemiology 54: , 343–349. https://doi.org/10.1016/S0895-4356(00)00314-0 |
10 | Breiman, L. , Friedman, J. , Stone, C. J. , Olshen, R. A. ((1984) ) Classification and regression trees. Wadsworth & Brooks. https://doi.org/10.1201/9781315139470 |
11 | Catalano, D. , Pereira, A. P. , Wu, M.-Y. , Ho, H. , Chan, F. ((2006) ) Service patterns related to successful employment outcomes of persons with traumatic brain injury in vocational rehabilitation. Neuro Rehabilitation 21: , 279–293. https://doi.org/10.3233/NRE-2006-21403 |
12 | Cavendish, W. , Connor, D. , Rediker, E. ((2017) ) Engaging students and parents in transition-focused individualized education programs. Intervention in School and Clinic, 228–235. https://doi.org/10.1177/1053451216659469 |
13 | Cavendish, W. , Connor, D. ((2018) ) Towards authentic IEPs and transition plans: Student, parent, and teacher perspectives. Learning Disability Quarterly 41: (1), 32–43. https://doi.org/10.1177/0731948716684680 |
14 | Centers for Disease Control and Prevention (CDC). ((2018) ) Autism spectrum, disorder: Data and statistics https://www.cdc.gov/ncbddd/autism/data.html |
15 | Chan, F. , Cheing, G. , Chan, J. Y. C. , Rosenthal, D. A. , Chronister, J. ((2006) ) Predicting employment outcomes of rehabilitation clients with orthopedic disabilities: A CHAID analysis. Disability and Rehabilitation 28: , 257–270. https://doi.org/10.1080/09638280500158307 |
16 | Chan, J. Y. , Keegan, J. P. , Ditchman, N. , Gonzalez, R. , Zheng, L. X. , Chan, F. ((2011) ) Stigmatizing attributions and vocational rehabilitation outcomes of people with disabilities. Rehabilitation Research, Policy & Education 25: , 135–148. https://doi.org/10.1891/216866511805001019 |
17 | Chandroo, R. , Strnadová, I. , Cumming, T. M. ((2020) ) Is it really student-focused planning? Perspectives of students with autism. Research in Developmental Disabilities 107: . https://doi.org/10.1016/j.ridd.2020.103783 |
18 | Chiang, H. M. , Cheung, Y. K. , Li, H. , Tsai, L. Y. ((2013) ) Factors associated with participation in employment for high school leavers with autism. Journal of Autism and Developmental Disabilities 43: , 1832–1842. https://doi.org/10.1007/s10803-012-1734-2 |
19 | Cidav, Z. , Marcus, S. C. , Mandell, D. S. ((2012) ) Implications of childhood autism for parental employment and earnings. Pediatrics 129: , 617–623. https://doi.org/10.1542/peds.2011-2700 |
20 | Dillman, D.A. , Smyth, J.D. , Christian, L.M. ((2014) ) Internet, phone, mail and mixed-mode surveys 4th ed. Wiley. |
21 | Erickson, W. A. , von Shrader, S. , Bruyere, S. M. , VanLooy, S. A. ((2014) ) The employment environment: Employer perspectives, policies, and practices regarding the employment of persons with disabilities. Rehabilitation Counseling Bulletin 57: (4), 195–208. https://doi.org/10.1177/0034355213509841 |
22 | Gal, E. , Selanikyo, E. , Bar-Haim Erez, A. , Katz, N. ((2015) ) Integration in the vocational world: How does it affect quality of life and subjective well-being of young adults with ASD. International Journal of Environmental Research and Public Health 12: , 10820–10832. https://doi.org/10.3390/ijerph120910820 |
23 | Gerhardt, P. F. , Lainer, I. ((2011) ) Addressing the needs of adolescents and adults with autism: A crisis on the horizon. Journal of Contemporary Psychotherapy 41: , 37–45. https://doi.org/10.1007/s10879-010-9160-2 |
24 | Hendricks, D. ((2010) ) Employment and adults with autism spectrum disorder: Challenges and strategies for success. Journal of Vocational Rehabilitation 32: , 125–134. https://doi.org/10.3233/JVR-2010-0502 |
25 | Heslin, P. A. , Bell, M. P. , Fletcher, P. O. ((2012) ) The devil without and within: A conceptual model of social cognitive processes whereby discrimination leads stigmatized minorities to become discouraged workers. Journal of Organizational Behavior 33: , 840–862. https://doi.org/10.1002/job.1795 |
26 | Hillier, A. , Galizzi, M. ((2014) ) Employment outcomes for young adults with autism spectrum disorders. A Review of Disability Studies: An International Journal 10: , 69–82. https://doi.org/10.1352/1944-7558-119.1.64 |
27 | Higgins, K. K. , Koch, L. C. , Boughfman, E. M. , Vierstra, C. ((2008) ) School-to-work transition and Asperger syndrome, Work 31: (3), 291–298. |
28 | Hurlbutt, K. , Chalmers, L. ((2004) ) Employment and adults with Asperger syndrome. Focus on Autism and Other Developmental Disabilities 19: (4), 215–222. https://doi.org/10.1177/10883576040190040301 |
29 | Hyde, K. , Griffiths, A. J. , Giannantonio, C. , Hurley-Hanson, A. , Linstead, E. ((2018) ) Predicting employer recruitment of individuals with autism spectrum disorders with decision trees. Publication in Proceeding of 2018 17th IEEE International Conference on Machine Learning and Applications (ICMLA), Orlando, FL, pp. 1366–1370. https://doi.org/10.1109/ICMLA.2018.00222 |
30 | Kolakowsky-Hayner, S. A. ((2010) ) Acceptance rates in state-federal vocational rehabilitation of clients with brain injury: Is racial disparity an issue? Brain Injury 24: , 1428–1447. https://doi.org/10.3109/02699052.2010.523039 |
31 | Kraus, L. , Lauer, E. , Coleman, R. , Houtenville, A. ((2018) ) 2017 Disability statistics annual report. University of New Hampshire. |
32 | Krieger, B. , Kinebanian, A. , Prodinger, B. , Heigl, F. ((2012) ) Becoming a member of the workforce: Perceptions of adults with Asperger syndrome. Work 43: (2), 141–157. https://doi.org/10.3233/WOR-2012-1392 |
33 | Lavelle, T. A. , Weinstein, M. C. , Newhouse, J. P. , Munir, K. , Kuhlthau, K. A. , Prosser, L. A. ((2014) ) Economic burden of childhood autism spectrum disorder. American Academy of Pediatrics 133: , 520–520. https://doi.org/10.1542/peds.2013-0763 |
34 | Lee, G. K. , Carter, E. W. ((2012) ) Preparing transition-age students with high-functioning autism spectrum disorders for meaningful work. Psychology in the Schools 49: (10), 988–1000. https://doi.org/10.1002/pits.21651 |
35 | Leigh, J. P. , Du, J. ((2015) ) Brief report: Forecasting the economic burden on autism in 2015 and 2025 in the United States. Journal of Autism and Developmental Disorders 45: , 413–419. https://doi.org/10.1007/s10803-015-2521-7 |
36 | Lindsay, S. , Cagliostro, E. , Leck, J. , Shen, W. , Stinson, J. ((2019) ) Employers’ perspectives of including young people with disabilities in the workforce, disability disclosure and providing accommodations. Journal of Vocational Rehabilitation 50: (2), 141–156. https://doi.org/10.3233/JVR-180996 |
37 | Lorenz, T. , Frischling, C. , Cuadros, R. , Heinitz, K. ((2016) ) Autism and overcoming job barriers: Comparing job-related barriers and possible solutions in and outside of autism-specific employment. PloS One 11: . https://doi.org/10.1371/journal.pone.0147040 |
38 | Lorenz, T. , Heinitz, K. ((2014) ) Aspergers– different, not Less: Occupational strengths and job interests of individuals with Asperger’s syndrome. Plos One 9: (6). https://doi.org/10.1371/journal.pone.0100358 |
39 | Martin, J. , Marshall, L. H. , Sale, P. ((2004) ) A 3-year study of middle, junior high, and high school IEP meetings. Exceptional Children 70: , 285–297. https://doi.org/10.1177/001440290407000302 |
40 | Mazzotti, V. K. , Rowe, D. A. , Sinclair, J. , Poppen, M. , Woods, W. E. , Shearer, M. L. ((2016) ) Predictors of post-school success: A systematic review of NLTS2 secondary analyses. Career Development and Transition for Exceptional Individuals 39: , 196–215. https://doi.org/10.1177/2165143415588047 |
41 | McMahon, B. T. , Hurley, J. E. , Chan, F. , Rumrill, P. D. , Jr , Roessler, R. ((2008) ) Drivers of hiring discrimination for individuals with disabilities. Journal of Occupational Rehabilitation 18: , 133–139. https://doi.org/10.1007/s10926-008-9136-1 |
42 | Müller, E. , Schuler, A. , Burton, B. A. , Yates, G. B. ((2003) ) Meeting the vocational support needs of individuals with Asperger syndrome and other autism spectrum disabilities, Journal of Vocational Rehabilitation 18: (3), 163–175. |
43 | Newman, L. , Wagner, M. , Knokey, A.-M. , Marder, C. , Nagle, K. , Shaver, D. , Wei, X. , with Cameto, R. , Contreras, E. , Ferguson, K. , Greene, S. , Schwarting, M. ((2011) ) The Post-High School Outcomes of Young Adults With Disabilities up to 8 Years After High School. A Report From the National Longitudinal Transition Study-2 (NLTS2) (NCSER 2011-3005). SRI International. www.nlts2.org/reports/ |
44 | Nord, D. K. , Stancliffe, R. J. , Nye-Legerman, K. , Hewitt, A. S. ((2016) ) Employment in the community for people with and without autism: A comparative analysis. Research in Autism Spectrum Disorders 24: , 11–16. https://doi.org/10.1016/j.rasd.2015.12.013 |
45 | Parsons, F. ((1909) ) Choosing a vocation. Houghton Mifflin. |
46 | Richards, J. ((2012) ) Examining the exclusion of employees with Asperger syndrome from the workplace. Personnel Review 41: (5), 630–646. https://doi.org/10.1108/00483481211249148 |
47 | R Core Team. ((2018) ) R: A language and environment for statistical computing. R Foundation for Statistical Computing. |
48 | Roux, A. M. , Shattuck, P. T. , Cooper, B. P. , Anderson, K. A. , Wagner, M. , Narendorf, S. C. ((2013) ) Postsecondary employment experiences among young adults with an Autism Spectrum Disorder: Employment in young adults with autism. Journal of the American Academy of Child & Adolescent Psychiatry 52: , 931–939. https://doi.org/10.1016/j.jaac.2013.05.019 |
49 | Roux, A. M. , Shattuck, P. T. , Rast, J. E. , Rava, J. A. , Anderson, K. A. ((2015) ) National autism indicators report: Transition into young adulthood (Life Course Outcomes Research Program). AJ Drexel Autism Institute, Drexel University. |
50 | Rose, J. , Saunders, K. , Hensel, E. , Kroese, B. S. ((2005) ) Factors affecting the likelihood that people with intellectual disabilities will gain employment. Journal of Intellectual Disabilities 9: (1), 9–23. https://doi.org/10.1177/1744629505049725 |
51 | Rowe, D. A. , McNaught, J. , Yoho, L. M. , Davis, M. , Mazzotti, V. L. ((2018) ) Helping students make informeddecisions about transition via web-based resources. Career Development and Transition for Exceptional Individuals 41: (4), 252–259. https://doi.org/10.1177/2165143417736266 |
52 | Sansosti, F. J. , Merchant, D. , Koch, L. C. , Rumrill, P. , Herrera A. ((2017) ) Providing supportive transition services to individuals with autism spectrum disorder: Considerations for vocational rehabilitation professionals. Journal of Vocational Rehabilitation 47: , 207–222. https://doi.org/10.3233/JVR-170896 |
53 | Scheeren, A. , Marieke Buil, J. , Howlin, P. , Bartels, M. , Begeer, S. ((2022) ) Objective and subjective psychosocial outcomes in adults with autism spectrum disorder: A 6-year longitudinal study. Autism 26: (1), 243–255. https://doi.org/10.1177/13623613211027673 |
54 | Scott, M. , Falkmer, M. , Girdler, S. , Falkmer, T. ((2015) ) Viewpoints on factors for successful employment for adults with autism spectrum disorder. PloS One 10: , e0139281. https://doi.org/10.1371/journal.pone.0139281 |
55 | Shattuck, P. T. , Narendorf, S. C. , Cooper, B. , Sterzing, P. R. , Wagner, M. , Taylor, J. L. ((2012) ) Postsecondary education and employment among youth with an autism spectrum disorder. Pediatrics 129: , 1042–1049. https://doi.org/10.1542/peds.2011-2864 |
56 | Sima, A. P. , Wehman, P. H. , Chan, F. , West, M. D. , Leucking, R. G. ((2015) ) An evaluation of risk factors related to employment outcomes for youth with disabilities. Career Development and Transition for Exceptional Individuals 38: , 89–100. https://doi.org/10.1177/2165143414534887 |
57 | Snell-Rood, C. , Ruble, L. , Kleinert, H. , McGrew, J. H. , Adams, M. , Rodgers, A. , Odom, J. , Wong, W. H. , Yu, Y. ((2020) ) Stakeholder perspectives on transition planning, implementation, and outcomes for students with autism spectrum disorder. Autism 24: (5), 1164–1176. https://doi.org/10.1177/1362361319894827 |
58 | Taylor, J. L. , Seltzer, M. M. ((2011) ) Employment and post-secondary educational activities for young adults with autism spectrum disorders during the transition to adulthood. Journal of Autism and Developmental Disorders 41: , 566–574. https://doi.org/10.1007/s10803-010-1070-3 |
59 | Therneau, T. , Atkinson, B. ((2018) ) rpart: Recursive Partitioning and Regression Trees. R package version 4.1-15. https://CRAN.R-project.org/package=rpart |
60 | Wagner, M. , Newman, L. , Cameto, R. , Garza, N. , Levine, P. ((2005) ) After high school: A first look at the postschool experiences of youth with disabilities. A report from the National Longitudinal Transition Study-2 (NLTS2). Retrieved from https://files.eric.ed.gov/fulltext/ED494935.pdf |
61 | Walsh, E. , Holloway, J. , Lydon, H. ((2018) ) An evaluation of a social skills intervention for adults with autism spectrum disorder and intellectual disabilities preparing for employment in Ireland: A pilot study. Journal of Autism and Developmental Disorders 48: (1), 1727–1741. https://doi.org/10.1007/s10803-017-3441-5 |
62 | Wehman, O. , Brooke, V. , Brooke, A. M. , Ham, W. , Schall, C. , McDonough, J. , Lau, S. , Seward, L. A. ((2016) ) Employment for adults with autism spectrum disorder: A retrospective review of a customized employment approach. Research in Developmental Disabilities 53: , 61–72. https://doi.org/10.1016/j.ridd.2016.01.015 |
Notes
1 Rather than using the descriptor person(s) with autism, this paper uses the term autistic, as emerging international research indicates that this population, specifically autistic adults, prefer identity-first language (National Autistic Society, 2021).