Implementing biomarkers to predict motor recovery after stroke
Abstract
BACKGROUND:
There is growing interest in using biomarkers to predict motor recovery and outcomes after stroke. The PREP2 algorithm combines clinical assessment with biomarkers in an algorithm, to predict upper limb functional outcomes for individual patients. To date, PREP2 is the first algorithm to be tested in clinical practice, and other biomarker-based algorithms are likely to follow.
PURPOSE:
This review considers how algorithms to predict motor recovery and outcomes after stroke might be implemented in clinical practice.
FINDINGS:
There are two tasks: first the prediction information needs to be obtained, and then it needs to be used. The barriers and facilitators of implementation are likely to differ for these tasks. We identify specific elements of the Consolidated Framework for Implementation Research that are relevant to each of these two tasks, using the PREP2 algorithm as an example. These include the characteristics of the predictors and algorithm, the clinical setting and its staff, and the healthcare environment.
CONCLUSIONS:
Active, theoretically underpinned implementation strategies are needed to ensure that biomarkers are successfully used in clinical practice for predicting motor outcomes after stroke, and should be considered in parallel with biomarker development.
1Introduction
Stroke remains a leading cause of adult disability, and the global burden of stroke continues to grow (Feigin et al., 2015). Patient outcomes are related to initial stroke severity and stroke lesion volume, as well as patient age and co-morbidities; however, these factors are related to global outcomes, such as death and disability (Heiss & Kidwell, 2014; van Almenkerk, Smalbrugge, Depla, Eefsting, & Hertogh, 2013; Veerbeek, Kwakkel, van Wegen, Ket, & Heymans, 2011). There is growing interest in the use of biomarkers early after stroke to predict subsequent recovery and outcomes for individual patients (Boyd et al., 2017; Kim & Winstein, 2016; Stinear, 2017). The majority of work thus far has focused on predicting recovery from motor impairment and motor function outcomes. There are two broad categories of motor system biomarkers that have received the most research attention to date: transcranial magnetic stimulation (TMS), and magnetic resonance imaging (MRI). This review will briefly describe the accumulating evidence for the use of these motor system biomarkers during the initial days and weeks after stroke, and then discuss the potential challenges and benefits of implementing these biomarkers in clinical practice.
1.2Motor system biomarkers
Biomarkers of the functional and structural integrity of the corticomotor system can predict recovery from motor impairment and motor function outcomes, in individual patients. TMS is a safe, painless, and non-invasive technique that can be used to elicit motor evoked potentials (MEPs) in contralateral muscles. Patients in whom TMS can elicit MEPs (MEP+) in the affected upper limb within the first 7 days after stroke will experience proportional recovery from upper limb impairment (Byblow, Stinear, Barber, Petoe, & Ackerley, 2015; Stinear, Byblow, et al., 2017b) and better upper limb functional outcomes than MEP- patients (Bembenek, Kurczych, Karli Nski, & Czlonkowska, 2012; Stinear, 2017). Importantly, patients with initially severe motor impairment can be MEP+ (Stinear, Barber, Petoe, Anwar, & Byblow, 2012; Stinear, Byblow, Ackerley, Barber, & Smith, 2017). Determining MEP status might therefore be particularly important for these patients, to distinguish between those with potential for good versus poor motor recovery and outcomes.
The predictive value of lower limb MEP status has received less attention, and studies to date have produced conflicting results. An early study reported that MEP status predicts recovery from distal lower limb impairment but not independent walking (Hendricks, Pasman, van Limbeek, & Zwarts, 2003). Subsequent studies have reported that MEP status predicts the return of independent walking (Chang, Do, & Chun, 2015; Piron, Piccione, Tonin, & Dam, 2005), but is not superior to clinical predictors (Smith, Barber, & Stinear, 2017), and does not predict proportional recovery from lower limb impairment (Smith, Byblow, Barber, & Stinear, 2017). These conflicting results may reflect that postural control is likely to be a greater contributor to achieving independent walking than the return of voluntary movement in the leg itself (Kollen, Van De Port, Lindeman, Twisk, & Kwakkel, 2005; Smith, Barber, & Stinear, 2017). Further work is needed to evaluate the usefulness of MEP status as a biomarker for lower limb motor recovery and outcomes after stroke.
Magnetic resonance imaging (MRI) can also be used to evaluate the structure and function of the corticomotor system. MRI has the advantage of being able to obtain measures from the entire sensorimotor network, in contrast to TMS which is largely confined to the primary motor cortex and corticospinal tract (CST). Despite this advantage, thus far the most robust imaging biomarkers for predicting motor recovery and outcomes after stroke are measures of CST integrity. The microstructural characteristics of white matter tracts, such as the CST, can be evaluated with diffusion-weighted imaging (Puig et al., 2017). This allows calculation of metrics such as fractional anisotropy within specific volumes of interest in the brain and along white matter tracts such as the CST. Several studies have shown that upper limb recovery and outcomes are predicted by fractional anisotropy asymmetry between the hemispheres at key points along the CST, such as the posterior limbs of the internal capsules (Byblow et al., 2015; Puig et al., 2011) and the pons (Puig et al., 2013). The stroke lesion load calculated along the length of the CSTs can also predict subsequent upper limb motor recovery and outcomes (Doughty et al., 2016; Feng et al., 2015).
Functional MRI can be used to assess the patterns and extent of cortical activity during passive and active upper limb movement after stroke. While there are clear relationships between fMRI measures and upper limb motor performance at the time of scanning (Buma, Lindeman, Ramsey, & Kwakkel, 2010; Favre et al., 2014; Grefkes & Ward, 2014), few studies have identified fMRI measures that can predict motor performance at future time points. Two studies have identified fMRI measures that predict subsequent upper limb motor outcomes (Hannanu et al., 2017; Rehme et al., 2015), though these measures were not used to make predictions for individual patients. While structural MRI measures of the CST and stroke lesion load show promise for making individualised predictions, there is currently no consensus regarding the use of functional MRI biomarker for predicting motor recovery or outcomes after stroke.
1.3Clinical usefulness of motor system biomarkers
Motor impairment is a common symptom of stroke, and regaining motor function is important for the patient’s independence in daily activities (Langhorne, Coupar, & Pollock, 2009). Being able to predict functional motor outcomes could help clinicians, patients, and families to set appropriate rehabilitation goals and make suitable plans for the level of support the patient is likely to need after discharge from hospital. However, making accurate predictions for individual patients based on clinical experience or assessment alone can be difficult (Nijland, van Wegen, Harmeling-van der Wel, Kwakkel, 2013). This lack of accuracy is concerning, given that the subjective prediction of discharge destination has a major influence over what rehabilitation is provided and patient outcomes (Luker, Bernhardt, Grimmer, & Edwards, 2014). Combining clinical assessment with the biomarkers outlined above may enable more accurate predictions (Kim & Winstein, 2016).
To date, only one approach has been explored for combining clinical assessment with biomarkers to make upper limb predictions for individual patients at the beginning of rehabilitation after stroke. The PREP algorithm sequentially combines assessment of paretic upper limb impairment with TMS and MRI within days of stroke to predict upper limb function at 3 months post-stroke. The PREP algorithm was developed in a group of 40 first-ever ischaemic stroke patients (Stinear et al., 2012), and has since been refined and validated in an independent cohort of 192 patients, including those with haemorrhagic and previous stroke (Stinear, Byblow, Ackerley, Barber, et al., 2017). The PREP2 algorithm has recently been reported (Fig. 1), which replaces the MRI biomarkers used in PREP with stroke severity measured with the NIHSS score (Stinear, Byblow, et al., 2017a). The PREP2 algorithm is therefore likely to be more readily implemented in clinical practice, and is described below.
Fig. 1
The PREP2 algorithm predicts upper limb functional outcome at 3 months post-stroke. The four possible upper limb outcomes are colour-coded. The coloured dots depict the proportion of patients expected to achieve each colour-coded outcome, depending on their pathway through the algorithm, based on the results of a CART analysis. Patients who achieve a SAFE score of five or more within 72 hours of stroke symptom onset, and are less than 80 years old, are most likely to have an Excellent upper limb outcome. Patients who achieve a SAFE score of five or more within 72 hours of stroke symptom onset and are 80 years old or more, are most likely to have an Excellent upper limb outcome provided their SAFE score is at least 8; otherwise they are likely to have a Good upper limb outcome. Patients whose SAFE score is less than five at 72 hours after stroke symptom onset need TMS to determine MEP status in the paretic upper limb, a key biomarker of corticospinal tract integrity. If a MEP can be elicited (MEP+) approximately 5 days post-stroke then the patient is likely to have at least a Good upper limb outcome. If a MEP cannot be elicited, the NIHSS score obtained 3 days post-stroke can be used to predict either a Limited outcome if the score is less than seven, or a Poor outcome if the score is seven or more.
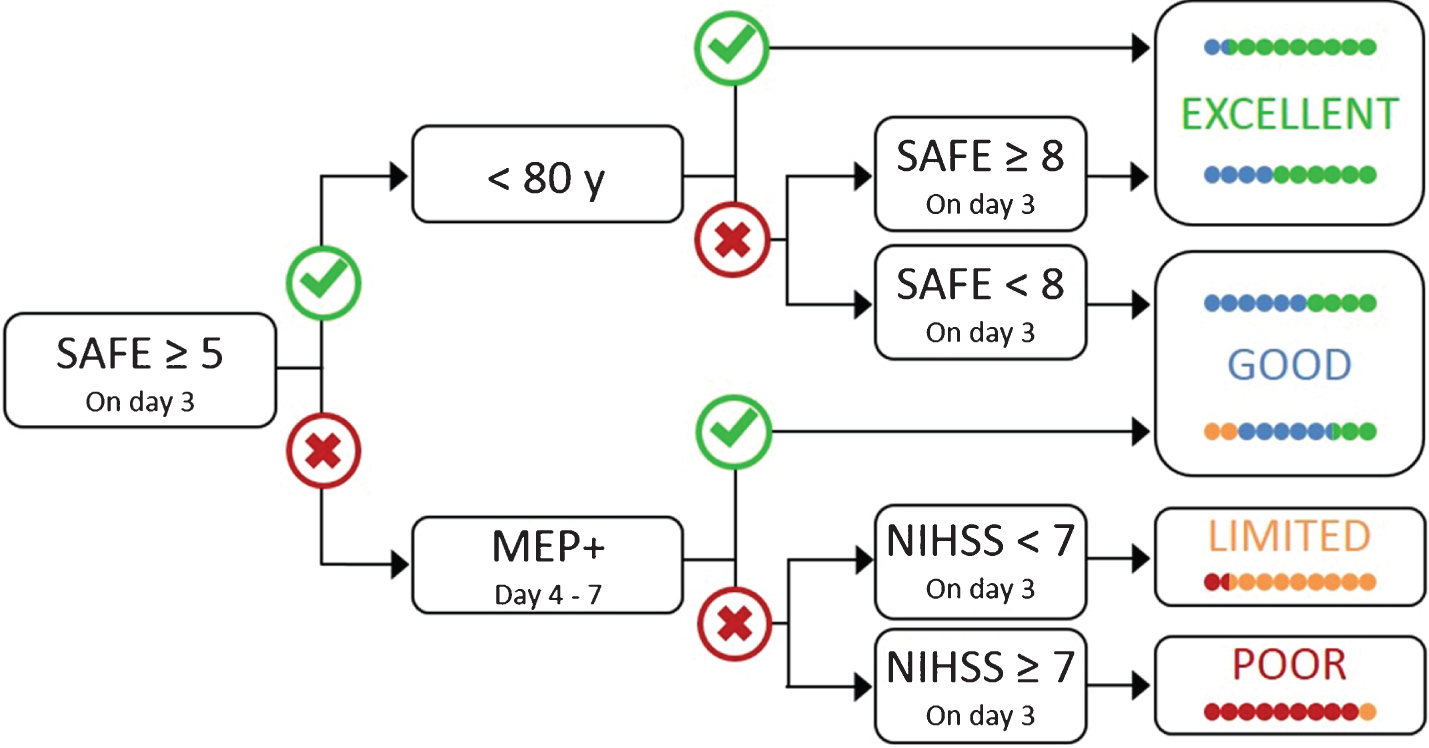
The algorithm begins with an evaluation of paretic shoulder abduction and finger extension (SAFE), using the Medical Research Council grades. The scores for these movements, out of five, are summed to produce the SAFE score, out of ten. Patients who achieve a SAFE score of at least five within 72 hours of stroke symptom onset are most likely to have an excellent upper limb outcome within three months provided they are aged less than 80 years (Table 1). If they are aged 80 years or more, they need to achieve a SAFE score of at least eight in order to have an excellent upper limb outcome; otherwise they are most likely to have a good upper limb outcome within three months. Patients with more severe initial upper limb impairment (SAFE score <5) at 72 hours post-stroke, are assessed with TMS to determine the MEP status of their paretic wrist extensors. Patients who are MEP+ are also likely to have a good upper limb motor outcome. Patients who are MEP- are most likely to have a limited upper limb outcome if their NIHSS score at 72 hours post-stroke is less than seven; otherwise they are most likely to have a poor upper limb outcome.
Table 1
Algorithm predictions
Predicted outcome | Description | Rehabilitation focus |
Excellent | Potential to make a complete, or near-complete, recovery of hand and arm function within three months | Promote normal use of the affected hand and arm with task-specific practice, while minimising adaptation and compensation. |
Good | Potential to be using the affected hand and arm for most activities of daily living within three months, though with some weakness, slowness, or clumsiness | Promote normal function of the affected hand and arm by improving strength, coordination, and fine motor control with repetitive and task-specific practice. Minimise compensation with the other hand and arm, and the trunk. |
Limited | Potential to regain movement in the affected hand and arm within three months, but daily activities are likely to require significant modification | Promote movement and reduce impairment by improving strength and active range of motion. Promote adaptation in daily activities, incorporating the affected upper limb wherever safely possible. |
Poor | Unlikely to regain useful movement of the hand and arm within three months | Prevent secondary complications such as pain, spasticity and shoulder instability. Reduce disability by learning to complete daily activities with the stronger hand and arm. |
Researchers who have the skills and resources to obtain MRI data from their participants can evaluate the stroke lesion load on the sensorimotor tracts of the ipsilesional hemisphere. T1-weighted imaging can be combined with a template of the primary sensorimotor tracts to calculate the lesion load on these tracts. Patients with a lesion load less than 15% are most likely to have a limited upper limb outcome, while those with a lesion load of 15% or more are most likely to have a poor upper limb outcome (Stinear, Byblow, et al., 2017a). The overall accuracy of the algorithm is essentially the same when using either this MRI biomarker of stroke lesion load or NIHSS score for MEP- patients (Stinear, Byblow, et al., 2017a). The sequential nature of the algorithm means that more sophisticated and expensive biomarkers are only obtained as required, with TMS needed for around one third of patients, and the NIHSS score or MRI needed for around one sixth (Stinear, Byblow, Ackerley, Barber, et al., 2017; Stinear, Byblow, et al., 2017a).
The effects on clinical practice of using biomarkers to make predictions for individual patients have been explored in one study thus far (Stinear, Byblow, Ackerley, Barber, et al., 2017). This study found that using PREP algorithm predictions to guide upper limb rehabilitation increased therapist confidence, altered the content but not dose of upper limb therapy, and was associated with a reduction in length of stay by 6 days, with no detectable negative effects on patient outcomes (Stinear, Byblow, Ackerley, Barber, et al., 2017). These results indicate that using CST biomarkers to predict upper limb outcomes for individual patients might improve rehabilitation efficiency. However, implementation of the PREP algorithm, or biomarkers more generally, has not yet been attempted outside of a research context. Subjective predictions of discharge destination have a major influence on what rehabilitation is provided and patient outcomes (Luker et al., 2014), and patients and carers report wanting more information (Luker, Lynch, Bernhardsson, Bennett, & Bernhardt, 2015; Luker et al., 2017). It is therefore likely that implementation of motor system biomarkers will improve patient experience and outcome, but this needs to be formally evaluated.
While there has been an exponential growth in research into biomarkers within medicine, little attention has been given to behaviour change of healthcare professionals in the development and implementation of biomarkers. There are several potential barriers and facilitators to implementation of biomarkers in stroke rehabilitation practice, and these are discussed below using the PREP2 algorithm as an example.
2Implementation of motor system biomarkers
There are two broad elements to consider in the implementation of biomarkers to predict motor outcomes for individual patients. A process for obtaining the prediction needs to be implemented, along with a process for communicating and using the prediction in the care of the patient. Here we use the Consolidated Framework for Implementation Research (Damschroder et al., 2009) as a guide for systematically considering potential barriers and facilitators for each of these processes.
2.1Obtaining the prediction
2.1.1Characteristics of the predictors
In general, prediction algorithms using biomarkers are more likely to be implemented if they are from a credible source, validated by high quality evidence, and clearly more accurate than clinical judgement. Complexity, adaptability, and cost are also important features. Biomarkers that require specialised technical expertise to obtain and analyse, such as sophisticated fMRI measures, may be too complex for widespread implementation in clinical practice. The complexity of the algorithm in which biomarkers are embedded may also create a barrier to implementation. Algorithms requiring several pieces of information from different sources, combined in multiple steps, are likely to be more difficult to implement than algorithms combining only a few pieces of information in a small number of steps. The PREP2 algorithm requires only the SAFE score for two-thirds of patients, and this simple score can be readily obtained as part of routine clinical practice.
Algorithms that require biomarkers to be obtained using strict protocols and on a rigid timeline have low adaptability, which is another potential barrier to implementation. Ideally, prediction algorithms will clearly identify components that require high fidelity, and those that can be adapted within specified parameters. For example, the PREP2 algorithm provides time windows for obtaining the SAFE score and TMS measures, and the PREP algorithm provides a time window for obtaining MRI measures. Future research may determine if these time windows can be further expanded. Maximising flexibility will foster implementation while allowing for variations in patient availability due to factors such as their medical stability and time spent on other tests and treatments, as well as variations in staff availability due to factors such as case load, weekends and leave.
The cost of obtaining biomarkers is another important factor influencing their implementation. Biomarkers that can be obtained at low cost, using existing resources, are more likely to be implemented than those that require greater investment to set up and sustain in practice. The PREP2 algorithm begins with a low cost measure (SAFE score) and moves to more costly TMS only as required. The costs of purchasing the necessary equipment for TMS and ongoing training for staff tasked with obtaining MEP status are a potential barrier to implementation. Similarly, the cost of an MRI scan that might not be part of routine care, as well as the cost of ongoing training for staff tasked with obtaining MRI biomarkers, are barriers that are likely to confine these biomarkers to the realm of research rather than clinical care. Implementation costs might be offset by savings associated with increased rehabilitation efficiency and shorter length of stay. Any site considering implementing the PREP2 algorithm would therefore need to carry out an economic analysis to determine whether implementation of the algorithm would be cost-effective, in addition to considering its other possible benefits such as helping to personalise rehabilitation for patients.
2.1.2Clinical setting
Several features of the clinical setting’s inner environment will affect the implementation of motor system biomarkers. These include the available resources and implementation climate. Time and money are known to be important factors in varied implementation theories and frameworks (Damschroder et al., 2009; Greenhalgh, Robert, Bate, Macfarlane, & Kyriakidou, 2005; Murray et al., 2010) and empirical studies (Huijg et al., 2015; Varsi, Ekstedt, Gammon, & Ruland, 2015).
Having sufficient numbers of trained staff members who are responsible for obtaining algorithm information and making predictions is important for implementation. These staff also need ready access to the equipment and space needed to obtain biomarker information. The first step of the PREP2 algorithm is the SAFE score, which can be obtained in the patient’s bedspace with no special equipment, as part of routine clinical assessment. If needed, the TMS step in the algorithm requires specialised equipment that can be used in the patient’s bedspace.
The implementation climate also has a powerful influence on implementation (Varsi et al., 2015). This includes leadership engagement, which is a key factor in successful implementation (Connell, McMahon, Harris, Watkins, & Eng, 2014; Damschroder & Lowery, 2013). Therefore to facilitate the use of prediction algorithms including biomarkers, it will be important to have the clinical service leaders on board, acting as opinion leaders to ensure the use of biomarkers is compatible with their service and seen as a relative priority. This includes ensuring staff have the necessary time, resources, information and feedback for successful implementation. For the PREP2 algorithm, this means helping staff to understand that using the algorithm is compatible with their values, and that those tasked with obtaining predictions are supported with the necessary time and resources to do so.
2.1.3Characteristics of the health care professionals
Characteristics of the staff involved in obtaining algorithm information are also important. The beliefs of healthcare staff about interventions are often more influential than other factors such as the strength of evidence for the intervention (Connell, McMahon, Tyson, Watkins, & Eng, 2016; Huijg et al., 2014). Biomarkers are more likely to be implemented in settings where staff recognise the value of accurate prediction information, and the limitations of predictions made on clinical assessment alone. The desire to improve prediction accuracy and rehabilitation efficiency is compatible with implementing biomarkers in clinical practice. Staff will also need to have the right skills to undertake the assessments required and feel confident in their abilities. Therefore some training is likely to be required. Implementation depends on identifying staff members who will be responsible for obtaining algorithm and biomarker information. It is not clear exactly whose role obtaining the measures should be, and it is likely to require a multi-disciplinary approach, with different members obtaining different measures, adding to the complexity. The SAFE score can be readily obtained by physical and occupational therapists, and could become part of current routine practice. Consistency in muscle testing technique and recording of the SAFE score on appropriate days by the whole team will need to be actively implemented in order to use PREP2 in clinical practice. In contrast, the TMS biomarker requires a different skillset, and will need to be obtained by specifically trained members of the team. Obtaining MRI biomarkers is likely to remain the responsibility of researchers who have the specialised skills required.
2.2Communicating and using the prediction
Once a prediction has been obtained for an individual patient, this information needs to be communicated and used in order to be of value. Knowledge alone is unlikely to change behaviour. Several factors are likely to affect the implementation of using prediction information.
2.2.1Characteristics of the prediction
Predictions that are relatively simple and easily understood by all parties will be more readily communicated and used in clinical practice. Some studies simply dichotomise the predicted outcome (Nijland, van Wegen, Harmeling-van der Wel, Kwakkel, 2010; Persson, Alt Murphy, Danielsson, Lundgren-Nilsson, & Sunnerhagen, 2015), which is easily understood but not very precise. Other studies have used multivariable linear regression analyses and produced mathematical equations combining several variables to predict the patient’s score on a clinical assessment scale. The patient’s numerical score might be predicted with reasonable precision, but not give a clear picture of the level of function the patient can expect to achieve in their daily activities. The PREP2 algorithm makes one of four predictions of upper limb functional outcome, which are easily understood in terms of what the patient will probably be able to do in their daily life (Table 1) (Stinear, Byblow, et al., 2017a). These features of the predictions facilitate their implementation.
The successful implementation of communicating and using prediction information also depends on how the information is presented. The written and verbal information provided to the clinical team, patient and their family needs to be simple, clear, and consistent. Prediction information also needs to be integrated with the patient’s clinical records, which may be in paper-based or electronic systems, or a combination of both. The compatibility of the prediction information with existing systems will affect its accessibility and usefulness to the rehabilitation team. The PREP2 algorithm has several resources for communicating prediction information, including written information for the patient’s rehabilitation team and for the patient and their family.
The trialability of new processes also affects their implementation. A process that can be tested and then stopped if necessary is more likely to be implemented than one that requires ongoing use once initiated. Obtaining, communicating and using prediction information does not replace an existing process, and can therefore be stopped at any time without having to revert to previous practices. The relatively high trialability of communicating and using motor predictions therefore facilitates their implementation.
2.2.2Clinical setting
Characteristics of the clinical setting will also affect how readily predictions will be communicated and used. The clinical setting can support implementation by ensuring staff are provided with resources and training, as well as opportunities for practice and feedback. Training in the communication of predictions will need to be provided to all team members, including medical and nursing staff, in addition to allied health staff. Training will also need to be provided on an ongoing basis, as staff typically rotate through services, and new staff join the rehabilitation team on a regular basis. A positive learning climate, where staff feel safe to try new things despite a risk of failure, will also support staff as they learn to communicate and use predictions. Clear lines of communication are needed to ensure that predictions are shared within the clinical team, and handed over to other clinical teams caring for the patient. A qualitative systematic review found that patients want information to help them understand stroke recovery, but highlighted the need for consistency of information across the multi-disciplinary team (Luker et al., 2015). Therefore processes will need to be worked out in each clinical setting to ensure consistent communication of predictions.
2.2.3Characteristics of the health care professionals
Identifying which staff members are responsible for communicating predictions to the rest of the clinical team, and to the patient and their family, is essential. These staff members need specific training and support so they are well-equipped to effectively, confidently and accurately convey predictions. Unfamiliarity with providing individualised prognostic information is a major barrier for therapists considering using this type of information. Other healthcare professionals, specifically physicians, have a great deal more training and experience, particularly in areas such as oncology. For allied health professionals working in rehabilitation, giving individualised predictions is new territory. Therapists involved in this process may find negative predictions particularly challenging. Therapists tend to see themselves as allies and advocates for their patients, and providing a negative prediction may seem incompatible with their role. Their fears that negative predictions might demotivate the patient and be used to ration therapy need to be addressed as part of the implementation process. Concerns about the accuracy of predictions also need to be addressed. Therapists’ confidence in their ability to have potentially difficult conversations with their patient is likely to affect whether and how they communicate and use prediction information. Hence implementation strategies will be needed to support therapists and overcome these barriers.
2.3Outer setting
Implementation of biomarkers will not occur in isolation, the wider context needs to be considered. As yet, there is no external policy or incentive that includes biomarkers, as evidence is still preliminary. However, with stroke rehabilitation, clinical guidelines and registries have been key drivers in advancing care (Cadilhac et al., 2016; Royal College of Physicians Intercollegiate Stroke Working Party, 2016). Therefore it will be interesting to see what the external drive for biomarkers in stroke rehabilitation will be. The patients’ needs and push for this type of information is also likely to evolve as predictor information becomes more commonplace. Their expectations and requirements, as well as their tolerance to the measures needed to obtain prediction information, will also influence implementation.
3Conclusion
The potential benefit of applying implementation research is that it can reduce the often cited 17-year time lag between scientific evidence reporting and clinical implementation (Morris, Wooding, & Grant, 2011). The basic science of developing and combining robust biomarkers of motor recovery has now reached an exciting stage, where these biomarkers can start to be integrated into clinical practice and improve patient care. This review has used the CFIR to systematically explore the domains likely to influence implementation of biomarkers in stroke rehabilitation. Using the CFIR prior to implementation has allowed groups to identify potential barriers and utilise this information to refine and adapt both their implementation strategy and the innovation before implementation began (Kirk et al., 2016). In stroke rehabilitation, an effective clinical protocol was successfully up-scaled by developing an implementation strategy alongside the original clinical trial (Middleton et al., 2016). The cluster-randomised controlled trial ‘Quality in Acute Stroke Care’ (QASC) by Middleton and colleagues demonstrated an evidence-based protocol to improve management of fever, hyperglycaemia, and swallowing dysfunction in acute stroke units. The protocol reduced mortality and patient dependency. A prospective pre-test/post-test study of a corresponding implementation strategy resulted in the intervention being used in New South Wales (Middleton et al., 2016). The QASC study is an example in stroke care where an intervention was developed, evaluated, then implemented using a concurrently developed and theoretically underpinned implementation strategy (Dale et al., 2015; Middleton et al., 2016, 2011). This is a real-life example of all the stages of the MRC framework for the development and evaluation of complex interventions (Craig, Dieppe, Macintyre, Michie, Nazareth, & Petticrew, 2008). Given the complex nature of the processes required for biomarkers to become part of routine practice, further research into implementation is warranted and should be considered in parallel with further biomarker development.
The implementation processes for obtaining prediction information, and then communicating and using this information, will differ between sites depending on the characteristics of the clinical setting and people involved, as outlined above. In general, implementation is likely to involve an initial phase of adopting the new processes and then adapting them to suit the local setting. Engaged leadership at multiple levels of the organisation, appropriate resourcing, and active dissemination of information will be needed. While there are some prognostic tools (like the PREP2 algorithm) that have been validated, they do not yet have explicit implementation strategies alongside them that address these issues – and this is where the work needs to be done. More broadly, principled implementation strategies need to be embedded in the development of new stroke rehabilitation tools and treatments, to facilitate their eventual translation to clinical practice.
Conflict of interest
The authors have no interests to declare.
References
1 | Bembenek, J. P. , Kurczych, K. , Karli Nski, M. , & Czlonkowska, A. ((2012) ). The prognostic value of motor-evoked potentials in motor recovery and functional outcome after stroke - a systematic review of the literature. Functional Neurology, 27: (2), 79–84. |
2 | Boyd, L. A. , Hayward, K. S. , Ward, N. S. , Stinear, C. M. , Rosso, C. , Fisher, R. J. , & Cramer, S. C. ((2017) ). Biomarkers of stroke recovery: Consensus-based core recommendations from the Stroke Recovery and Rehabilitation Roundtable. International Journal of Stroke, 12: (5), 480–493, doi: 10.1177/1747493017714176 |
3 | Buma, F. E. , Lindeman, E. , Ramsey, N. F. , & Kwakkel, G. ((2010) ). Functional neuroimaging studies of early upper limb recovery after stroke: A systematic review of the literature. Neurorehabilitation and Neural Repair, 24: (7), 589–608, doi: 10.1177/1545968310364058 |
4 | Byblow, W. D. , Stinear, C. M. , Barber, P. A. , Petoe, M. A. , & Ackerley, S. J. ((2015) ). Proportional recovery after stroke depends on corticomotor integrity. Annals of Neurology, 78: (6), 848–859, doi: 10.1002/ana.24472 |
5 | Cadilhac, D. A. , Kim, J. , Lannin, N. A. , Kapral, M. K. , Schwamm, L. H. , Dennis, M. S. , & Meretoja, A. ((2016) ). National stroke registries for monitoring and improving the quality of hospital care: A systematic review. International Journal of Stroke, 11: (1), 28–40, doi: 10.1177/1747493015607523 |
6 | Chang, M. C. , Do, K. H. , & Chun, M. H. ((2015) ). Prediction of lower limb motor outcomes based on transcranial magnetic stimulation findings in patients with an infarct of the anterior cerebral artery. Somatosensory & Motor Research, 32: (4), 249–253, doi: 10.3109/08990220.2015.1091769 |
7 | Connell, L. A. , McMahon, N. E. , Harris, J. E. , Watkins, C. L. , & Eng, J. J. ((2014) ). A formative evaluation of the implementation of an upper limb stroke rehabilitation intervention in clinical practice: A qualitative interview study. Implementation Science, 9: , 90. 10.1186/s13012-014-0090-3 |
8 | Connell, L. A. , McMahon, N. E. , Tyson, S. F. , Watkins, C. L. , & Eng, J. J. ((2016) ). Case Series of a Knowledge Translation Intervention to Increase Upper Limb Exercise in Stroke Rehabilitation. Physical Therapy, 96: (12), 1930–1937, doi: 10.2522/ptj.20150694 |
9 | Craig, P. , Dieppe, P. , Macintyre, S. , Michie, S. , Nazareth, I. , & Petticrew, M. ((2008) ). Developing and evaluating complex interventions: The new Medical Research Council guidance. British Medical Journal, 337: (a1655). |
10 | Dale, S. , Levi, C. , Ward, J. , Grimshaw, J. M. , Jammali-Blasi, A. , D’Este, C. , & Middleton, S. ((2015) ). Barriers and enablers to implementing clinical treatment protocols for fever, hyperglycaemia, and swallowing dysfunction in the Quality in Acute Stroke Care (QASC) Project–a mixed methods study. Worldviews in Evidence Based Nursing, 12: (1), 41–50, doi: 10.1111/wvn.12078 |
11 | Damschroder, L. J. , Aron, D. C. , Keith, R. E. , Kirsh, S. R. , Alexander, J. A. , & Lowery, J. C. ((2009) ). Fostering implementation of health services research findings into practice: A consolidated framework for advancing implementation science. Implementation Science, 4: , 50. 10.1186/1748-5908-4-50 |
12 | Damschroder, L. J. , & Lowery, J. C. ((2013) ). Evaluation of a large-scale weight management program using the consolidated framework for implementation research (CFIR. Implementation Science, 8: , 51. 10.1186/1748-5908-8-51 |
13 | Doughty, C. , Wang, J. , Feng, W. , Hackney, D. , Pani, E. , & Schlaug, G. ((2016) ). Detection and Predictive Value of Fractional Anisotropy Changes of the Corticospinal Tract in the Acute Phase of a Stroke. Stroke, 47: (6), 1520–1526, doi: 10.1161/STROKEAHA.115.012088 |
14 | Favre, I. , Zeffiro, T. A. , Detante, O. , Krainik, A. , Hommel, M. , & Jaillard, A. ((2014) ). Upper Limb Recovery After Stroke Is Associated With Ipsilesional Primary Motor Cortical Activity: A Meta-Analysis. Stroke, 10.1161/STROKEAHA.113.003168 |
15 | Feigin, V. L. , Krishnamurthi, R. V. , Parmar, P. , Norrving, B. , Mensah, G. A. , Bennett, D. A. , G. B. D. S. P. E. Group, ((2015) ). Update on the Global Burden of Ischemic and Hemorrhagic Stroke in 1990-2013: The GBD 2013 Study. Neuroepidemiology, 45: (3), 161–176, doi: 10.1159/000441085 |
16 | Feng, W. , Wang, J. , Chhatbar, P. Y. , Doughty, C. , Landsittel, D. , Lioutas, V. A. , & Schlaug, G. ((2015) ). Corticospinal tract lesion load: An imaging biomarker for stroke motor outcomes. Annals of Neurology, 78: (6), 860–870, doi: 10.1002/ana.24510 |
17 | Greenhalgh, T. , Robert, G. , Bate, P. , Macfarlane, F. , & Kyriakidou, O. ((2005) ), Diffusion of Innovations in Service Organisations: A Systematic Literature Review, Malden, MA: Blackwell Publishing. |
18 | Grefkes, C. , & Ward, N. S. ((2014) ). Cortical reorganization after stroke: How much and how functional?. The Neuroscientist, 20: (1), 56–70, doi: 10.1177/1073858413491147 |
19 | Hannanu, F. F. , Zeffiro, T. A. , Lamalle, L. , Heck, O. , Renard, F. , Thuriot, A. , & Group, I.-H. S. ((2017) ). Parietal operculum and motor cortex activities predict motor recovery in moderate to severe stroke. Neuroimage Clinical, 14: , 518–529, doi: 10.1016/j.nicl.2017.01.023 |
20 | Heiss, W. D. , & Kidwell, C. S. ((2014) ). Imaging for prediction of functional outcome and assessment of recovery in ischemic stroke. Stroke, 45: (4), 1195–1201, doi: 10.1161/STROKEAHA.113.003611 |
21 | Hendricks, H. T. , Pasman, J. W. , van Limbeek, J. , & Zwarts, M. J. ((2003) ). Motor evoked potentials of the lower extremity in predicting motor recovery and ambulation after stroke: A cohort study. Archives of Physical Medicine and Rehabilitation, 84: (9), 1373–1379, doi: S0003999303002375 [pii] |
22 | Huijg, J. M. , Dusseldorp, E. , Gebhardt, W. A. , Verheijden, M. W. , van der Zouwe N. , Middelkoop, B. J. C. , & Crone, M. R. ((2014) ). Factors Associated With Physical Therapists’ Implementation of Physical Activity Interventions in the Netherlands. Physical Therapy, 10.2522/ptj.20130457 |
23 | Huijg, J. M. , Gebhardt, W. A. , Verheijden, M. W. , van der Zouwe, N. , de Vries, J. D. , Middelkoop, B. J. , & Crone, M. R. ((2015) ). Factors influencing primary health care professionals’ physical activity promotion behors: A systematic review. International Journal of Behoral Medicine, 22: (1), 32–50, doi: 10.1007/s12529-014-9398-2 |
24 | Kim, B. , & Winstein, C. ((2016) ). Can Neurological Biomarkers of Brain Impairment Be Used to Predict Poststroke Motor Recovery? A Systematic Review. Neurorehabilitation and Neural Repair, 31: (1), 3–24, doi: 10.1177/1545968316662708 |
25 | Kirk, M. A. , Kelley, C. , Yankey, N. , Birken, S. A. , Abadie, B. , & Damschroder, L. ((2016) ). A systematic review of the use of the Consolidated Framework for Implementation Research. Implementation Science, 11: , 72. 10.1186/s13012-016-0437-z |
26 | Kollen, B. , van de Port, I. , Lindeman, E. , Twisk, J. , & Kwakkel, G. ((2005) ). Predicting improvement in gait after stroke: A longitudinal prospective study/STR. Stroke, 36: , 50. |
27 | Langhorne, P. , Coupar, F. , & Pollock, A. ((2009) ). Motor recovery after stroke: A systematic review. Lancet Neurology, 8: (8), 741–754, 10.1016/S1474-4422(09)70150-4. |
28 | Luker, J. , Lynch, E. , Bernhardsson, S. , Bennett, L. , & Bernhardt, J. ((2015) ). Stroke Survivors’ Experiences of Physical Rehabilitation: A Systematic Review of Qualitative Studies. e. Archives of Physical and Medical Rehabilitation, 96: (9), 1610. 10.1016/j.apmr.2015.03.017 |
29 | Luker, J. , Murray, C. , Lynch, E. , Bernhardsson, S. , Shannon, M. , & Bernhardt, J. ((2017) ). Carers’ Experiences, Needs, and Preferences During Inpatient Stroke Rehabilitation: A Systematic Review of Qualitative Studies. Archives of Physical and Medical Rehabilitation, 10.1016/j.apmr.2017.02.024 |
30 | Luker, J. A. , Bernhardt, J. , Grimmer, K. A. , & Edwards, I. ((2014) ). A qualitative exploration of discharge destination as an outcome or a driver of acute stroke care. BMC Health Services Research, 14: , 193. 10.1186/1472-6963-14-193 |
31 | Middleton, S. , Lydtin, A. , Comerford, D. , Cadilhac, D. A. , McElduff, P. , Dale, S. , Committee, Q. W. G. A. S. ((2016) ). From QASC to QASCIP: Successful Australian translational scale-up and spread of a proven intervention in acute stroke using a prospective pre-test/post-test study design. e. BMJ Open, 6: (5), 011568. 10.1136/bmjopen-2016-011568 |
32 | Middleton, S. , McElduff, P. , Ward, J. , Grimshaw, J. M. , Dale, S. , D’Este, C. , & Group, Q. T. ((2011) ). Implementation of evidence-based treatment protocols to manage fever, hyperglycaemia, and swallowing dysfunction in acute stroke (QASC): A cluster randomised controlled trial. Lancet, 378: (9804), 1699–1706, doi: 10.1016/S0140-6736(11)61485-2 |
33 | Morris, Z. S. , Wooding, S. , & Grant, J. ((2011) ). The answer is 17 years, what is the question: Understanding time lags in translational research. Journal of the Royal Society of Medicine, 104: (12), 510–520, doi: 10.1258/jrsm.2011.110180 |
34 | Murray, E. , Treweek, S. , Pope, C. , MacFarlane, A. , Ballini, L. , Dowrick, C. , & May, C. ((2010) ). Normalisation process theory: A framework for developing, evaluating and implementing complex interventions. BMC Medicine, 8: , 63. 10.1186/1741-7015-8-63 |
35 | Nijland, R. H. , van Wegen, E. E. , Harmeling-van der Wel, B. C. , Kwakkel, G. , Early Prediction of Functional Outcome After Stroke, I. ((2013) ). Accuracy of physical therapists’ early predictions of upper-limb function in hospital stroke units: The EPOS Study. Physical Therapy, 93: (4), 460–469, doi: 10.2522/ptj.20120112 |
36 | Nijland, R. H. , van Wegen, E. E. , Harmeling-van der Wel, B. C. , Kwakkel, G. , & Investigators, E. ((2010) ). Presence of finger extension and shoulder abduction within 72 hours after stroke predicts functional recovery: Early prediction of functional outcome after stroke: The EPOS cohort study. Stroke, 41: (4), 745–750, doi: 10.1161/STROKEAHA.109.572065 |
37 | Persson, H. C. , Alt Murphy, M. , Danielsson, A. , Lundgren-Nilsson, A. , & Sunnerhagen, K. S. ((2015) ). A cohort study investigating a simple, early assessment to predict upper extremity function after stroke - a part of the SALGOT study. BMC Neurology, 15: , 92. 10.1186/s12883-015-0349-6 |
38 | Piron, L. , Piccione, F. , Tonin, P. , & Dam, M. ((2005) ). Clinical correlation between motor evoked potentials and gait recovery in poststroke patients. Archives of Physical Medicine and Rehabilitation, 86: (9), 1874–1878, doi: S0003-9993(05)00313-8 [pii] 10.1016/j.apmr.2005.03.007 |
39 | Puig, J. , Blasco, G. , Daunis, I. E. J. , Thomalla, G. , Castellanos, M. , Figueras, J. , & Pedraza, S. ((2013) ). Decreased corticospinal tract fractional anisotropy predicts long-term motor outcome after stroke. Stroke, 44: (7), 2016–2018, doi: 10.1161/STROKEAHA.111.000382 |
40 | Puig, J. , Blasco, G. , Schlaug, G. , Stinear, C. M. , Daunis, I. E. P. , Biarnes, C. , & Pedraza, S. ((2017) ). Diffusion tensor imaging as a prognostic biomarker for motor recovery and rehabilitation after stroke. Neuroradiology, 59: (4), 343–351, doi: 10.1007/s00234-017-1816-0 |
41 | Puig, J. , Pedraza, S. , Blasco, G. , Daunis, I. E. J. , Prados, F. , Remollo, S. , & Serena, J. ((2011) ). Acute damage to the posterior limb of the internal capsule on diffusion tensor tractography as an early imaging predictor of motor outcome after stroke. American Journal of Neuroradiology, 32: (5), 857–863, 10.3174/ajnr.A2400 |
42 | Rehme, A. K. , Volz, L. J. , Feis, D. L. , Eickhoff, S. B. , Fink, G. R. , & Grefkes, C. ((2015) ). Individual prediction of chronic motor outcome in the acute post-stroke stage: Behoral parameters versus functional imaging. Human Brain Mapping, 36: (11), 4553–4565, 10.1002/hbm.22936 |
43 | Royal College of Physicians Intercollegiate Stroke Working Party. ((2016) ), RCP National clinical guidelines for stroke: 5th edition, LondonL Royal College of Physicians. |
44 | Smith, M. C. , Barber, P. A. , & Stinear, C. M. ((2017) ). The TWIST algorithm predicts Time to Walk Independently after STroke. Neurorehabilitation and Neural Repair, in press, 10.1177/1545968317736820 |
45 | Smith, M. C. , Byblow, W. D. , Barber, P. A. , & Stinear, C. M. ((2017) ). Proportional Recovery From Lower Limb Motor Impairment After Stroke. Stroke, 48: (5), 1400–1403, doi: 10.1161/STROKEAHA.116.016478 |
46 | Stinear, C. M. ((2017) ). Prediction of motor recovery after stroke: Advances in biomarkers. Lancet Neurol, 16: (10), 826–836. |
47 | Stinear, C. M. , Barber, P. A. , Petoe, M. , Anwar, S. , & Byblow, W. D. ((2012) ). The PREP algorithm predicts potential for upper limb recovery after stroke. Brain; a Journal of Neurology, 135: (Pt 8), 2527–2535, doi: 10.1093/brain/aws146 |
48 | Stinear, C. M. , Byblow, W. D. , Ackerley, S. J. , Barber, P. A. , & Smith, M. C. ((2017) ). Predicting Recovery Potential for Individual Stroke Patients Increases Rehabilitation Efficiency. Stroke, 48: (4), 1011–1019, doi: 10.1161/STROKEAHA.116.015790 |
49 | Stinear, C. M., Byblow, W. D., Ackerley, S. J., Smith, M. C., Borges, V. M., & Barber, P. A. ((2017) a). PREP2: A biomarker-based algorithm for predicting upper limb function after stroke. Annals of Clinical and Translational Neurology, in press. |
50 | Stinear, C. M., Byblow, W. D., Ackerley, S. J., Smith, M. C., Borges, V. M., & Barber, P. A. ((2017) b). Proportional Motor} Recovery After Stroke: Implications for Trial Design. Stroke, 48: (3), 795–798, doi: 10.1161/STROKEAHA.116.016020 |
51 | van Almenkerk, S. , Smalbrugge, M. , Depla, M. F. , Eefsting, J. A. , & Hertogh, C. M. ((2013) ). What predicts a poor outcome in older stroke survivors? A systematic review of the literature. Disability and Rehabilitation, 35: (21), 1774–1782, doi: 10.3109/09638288.2012.756941 |
52 | Varsi, C. , Ekstedt, M. , Gammon, D. , & Ruland, C. M. ((2015) ). Using the Consolidated Framework for Implementation Research to Identify Barriers and Facilitators for the Implementation of an Internet-Based Patient-Provider Communication Service in Five Settings: A Qualitative Study. e. Journal of Medical Internet Research, 17: (11), 262. 10.2196/jmir.5091 |
53 | Veerbeek, J. M. , Kwakkel, G. , van Wegen, E. E. , Ket, J. C. , & Heymans, M. W. ((2011) ). Early prediction of outcome of activities of daily living after stroke: A systematic review. Stroke, 42: (5), 1482–1488, doi: 10.1161/STROKEAHA.110.604090 |