Governing-by-the-numbers/Statistical governance: Reflections on the future of official statistics in a digital and globalised society
Abstract
The growing importance of statistical evidence, data and information for political decisions is reflected in the handy and popular formulation ’Data for Policy’ (D4P). Under this cover, well-known guiding themes, such as the modernisation of the public sector, or evidence-informed policy-making, are led to new solutions with new technologies and infinitely rich data sources. Data for Policy means more to official statistics than just new data, techniques and methods. It is not least a matter of securing an important function and position for official statistics in the Policy for Data of the future. In order to justify this position, it is necessary to have a clear understanding of the tasks of official statistics for the functioning of (democratic) societies, with a view to how these tasks have to be reinterpreted under changing conditions (above all because of digitisation and globalisation).
“People are becoming more demanding, whether as consumers of goods and services in the market place, as citizens or as businesses affected by the policies and services which government provides. To meet these demands, government must be willing constantly to re-evaluate what it is doing so as to produce policies that really deal with problems; that are forward-looking and shaped by the evidence rather than a response to short-term pressures; that tackle causes not symptoms; that are measured by results rather than activity; that are flexible and innovative rather than closed and bureaucratic; and that promote compliance rather than avoidance or fraud. To meet people’s rising expectations, policy making must also be a process of continuous learning and improvement.”11
1.Introduction
“Data meet politics”, under this cover, well-known guiding themes, such as the modernisation of the public sector, or evidence-informed policy-making, are led to new solutions with new technologies and infinitely rich data sources by “using 21st century tools to address 21st century issues.” The possibilities for “cutting edge solutions”, “close to real time data” seem obvious if we succeed in using “advanced analytics, both timely and simple and clear enough for fast-paced policy decisions”.22 The question arises why, despite all these modern and innovative possibilities, there should still be a need for elaborate official statistics in the future. Why should a politician wait for official statistics when there was real-time data for the most difficult decisions? Why afford a statistical service to the cost of taxpayers when it seems so easy to analyse the data that all the machines mutually connected within the Internet of Things are generating all the time? As can be seen quickly, Data for Policy means more to official statistics than just new data, techniques and methods. It is not least a matter of securing an important function and position for official statistics in the Policy for Data of the future. In order to justify this position, it is necessary to have a clear understanding of the tasks of official statistics for the functioning of (democratic) societies, with a view to how these tasks have to be reinterpreted under changing conditions (above all because of digitisation and globalisation).
One might now come to the conclusion that these are all questions and topics with which the various actors in statistics have to come to terms, a purely practical matter. Why then do we need an article in a scientific journal? However, as will be explained below, it is very much a subject of scientific research, and not necessarily of those that are already being addressed in statistics, namely questions of survey methodology, sampling, error correction, etc. Rather, the scientific inputs in this field also and especially come from other disciplines, such as sociology or political science.
Furthermore, one may have the impression that the issues dealt with in the following, which deal with information quality, interaction with users and statistical governance, are relevant only to a small group of statisticians, especially those responsible for the strategic direction of official statistics. This impression is also misleading. The very basic topics, such as epistemology, form the foundation for a correctly understood and applied statistical methodology. In this respect, they belong not least to the elementary programme of statistical education and professional literacy.
The thesis is put forward that the questions and topics dealt with in this article are currently covered in many ways by methodological developments, scientific investigations and practical tests, but that these take place in partly very different communities and that mutual fertilisation is insufficient. For example, the Science and Technology Studies located in sociology or the discourse on Governing by the Numbers are little or not at all known in statistical communities. In mirror image, in scientific circles, for example in sociology, the knowledge and awareness of what quality standards have been implemented in official statistics in recent years is insufficient.
Especially under the current conditions of digitalisation, globalisation and the increasingly widespread scepticism towards experts and facts, it is therefore important to close the gaps between the discussions in different communities.
2.From evidence-based to data-driven decision-making
Since the 1990s at the latest, it has been part of the basic understanding of a modern and good governance that politics is based on evidence [2, 3]. Irrespective of the political colour of the rulers, a modernisation of the administration according to the New Public Management model was promoted. Rational, pertinent, legitimate and accountable decision-making requires that all available information be taken into account. If decisions are not supported by evidence, there are many dangers such as lack of transparency, subjectivity or even erroneous policies. In this respect, the demand for evidence-based policy-making (EBPM) is a tautology in times of enlightened, democratic societies that request efficiency. The origins and sources of EBPM can therefore also be found in the approaches to a rational and economic approach, be it in management theories at the enterprise level or in economic theory for politics.
More recently, the use of language has evolved to the extent that we are now talking about ‘data-driven policy-making’ [4] or more simply ‘data-for-policy (D4P)’ [5]. The background to this is the enormously rapid increase in the amount of data that should be used – provided the appropriate technologies and methodologies are in place – to further improve public administration in general and political decisions in particular.
Although this more recent orientation must be understood as a logical and consistent further development, it contains new elements and sets new priorities. First, the term ‘data’ takes the place of ‘evidence’, which is derived from the fact that data is regarded as the new fuel of the 21st century. Organisations and administrations should be data-centred, and decisions should be data-driven accordingly. In combination with algorithms, machine learning (so-called artificial intelligence) and the Internet of Things, which all rely on these data and use them as fuel, this results in a new dimension of decision making; instead of augmenting decisions, they are becoming entirely automated.
This makes it obvious that the question of the interaction of technologies, of political/social developments and of data/information (as tools of power and governance) in the era of digitalisation arises in a completely new and intensified form. Paradoxically, data/ information can also be misused and lead to the opposite of what was originally intended with EBPM, in a similar form to the Internet, which was supposed to promote democracy and also gives birth to authoritarian applications [6].
Of course, the developments described are of a general nature and affect all areas of the economy, public life and politics. However, the sole aim here is to show the effects on official statistics and possibly to draw conclusions for necessary measures and strategic directions. Official statistics in this sense is understood as the part of the public administration entrusted with providing society with solid statistics on the essential topics of population, social or environmental issues, and economy. As the more than two-hundred-year history of official statistics shows, there were not only steady developments, but also abrupt leaps in development, especially when technological, scientific and political driving forces were mutually reinforcing [7, 8]. Just as there is talk of a fourth industrial revolution, official statistics can also be seen in the midst of a period of rapid and fundamental change, in which raw materials (i.e. data), production processes and user expectations are shifting dramatically.
In this situation it is indeed possible to react with the adaptation of methods and processes to modified conditions in the usual continuous pattern. However, this is unlikely to be sufficient. Rather, in a period of fundamental societal and technological changes, it seems necessary to become clear again about the essence of what official statistics have been in the past, what they are today and what they should be in the future.
A crucial question in this context is whether the production of evidence on the one hand and policy decisions on the other are separate and independent processes. As can be seen from the many, sometimes dramatic examples (e.g. the start of the financial crisis 2009 through falsified Greek statistics, Brexit, to name but a few) [9, 10], this independence cannot be simply assumed. On the contrary, for the adequate use of EBPM or of D4P, it is essential to know the risks and side effects resulting from the fact that the processes of quantification, measuring and decision-making are systematically and permanently interrelated.33 Based on the sociological concept of ‘co-production’ from the Science and Technology Studies (STS), the different effects, feedback and pitfalls can be systematised and analysed. Particularly against the background of increasing uncertainty and scepticism towards experts in society, it is crucial that these experts and participants in political decision-making processes are conscious of the risks and side effects, are aware of their importance and take them into account when dosing and monitoring the efficacy of the ‘medicine’ called EBPM/D4P.
At the centre of this strategically important topic for official statistics is therefore the interrelation between, on the one hand, ‘Governing-by-the Numbers’ [11] and, on the other hand, ‘Informational Governance’ [12].
Some quite fundamental questions will have to be asked and discussed in the following:
• What are facts (also including related terms, such as data, information, evidence)?
• How can facts be produced with high quality?
• What is the role of official statistics as part of public administration?
• Is this role being changed due to the new constraints and challenges in the era of digitisation and globalisation?
• How can civil society’s role be strengthened and deepened in all processes of statistical production?
• Which adaptations of statistical governance are needed in order to preserve and protect the functioning of official statistics in the new informational (and political) ecosystem?
3.What are facts?
As a statistician, you are often surprised by the peculiar expectations or comments you are confronted with:
• On the one hand, there is an almost blind faith in the existence of facts as an absolute form of truth. “Look at the data!” “Let’s do a fact check!” “You can’t manage what you don’t measure!” belong to such widespread and popular mantras not seldom accompanied by a good portion of naivety.
• On the other hand, after a few sentences of conversation, you find yourself confronted with one of the common jokes about statistics and statisticians: “I only believe in statistics that I doctored myself.”44, “Lies, damned lies, and statistics”55 or “Not everything that can be counted counts, and not everything that counts can be counted.66 In contrast to the first group (the naïve positivists), a subtle mistrust and discomfort resonates, which feeds on professional ignorance and scepticism about the power of experts.
Due to a lack of statistical literacy and missing information about the quality of statistics, a dangerous mixture of feelings of dependence and distrust seems to emerge. Statistics are forced into the dichotomy of truth and lies, which are not only unsuitable, but also stand in the way of a proper handling of statistical information, based on a knowledge of its actual possibilities and limitations. More recently, it appears that this situation is not improving but worsening. There is a debate about facts and alternative facts, about news and fake news, without the core question being asked and answered in public what facts actually are.
In his book “Postfaktisch” Vincent F. Hendricks [13] presents a scale of information quality “in which true and different forms of false statements and strategies undermining truth face each other at opposite ends”. While he goes into detail on the various variants of misinformation, such as distorted statements, lies, fake news, it remains unclear what he understands by ‘true statements’. As a definition he offers “Verified Facts”. Irrespective of whether it makes sense and is helpful to deal with the concept of truth in this context, from the statistician’s point of view the question arises as to what facts are and how they should and can be verified.
It should be noted that statistical facts are the end product of processes that begin with the design of a methodology (translation of a question into a quantifiable variable, definition of the survey programme, etc.), which are secondly produced according to this methodological design, and which are finally communicated to those who wish to use the information for their respective questions. In other words, facts are manufactured products. Like other products, they can have a good design that functionally performs what is expected. As with other products, manufacturing errors can occur. As with other products, misunderstandings and errors can occur during delivery (here the communication). Due to this nature of statistical information, emotionally overloaded questions about truth and lies no longer arise. Rather, it is a matter of producing quality and communicating this quality in such a way that the users of the information can understand this and draw the right conclusions for themselves. However, all these are tasks and problems that occur in more or less the same form for all (industrial) products (that the user has not produced him/herself). Questions of quality management, transparency, labelling and certification naturally play a major role in this context.
These remarks are in danger of being misunderstood to mean that a kind of traditional deductive approach, which is supposedly the only one used in official statistics, is advocated. It should therefore be made clear that the tradition of official statistics is characterised by an interplay between theoretical model and empirical data evaluation, between deductive and inductive procedures [14]. This interplay is embedded in an iterative learning process that leads year after year to adjustments to new circumstances (be it information needs or data bases or methods). At the end of the day, however, official statistics are assessed by their compliance with international standards (which are themselves regularly revised), their comparability in terms of time and space, etc. An inductive reasoning (“data first”) to generate new questions will therefore not become the typical and frequently used approach in official statistics in the future either. Nevertheless, even more often than in the past, the use of secondary data could generate new questions and theories which could then be confirmed by deductive methods and models and eventually lead to statistical facts. This is an approach, labelled experimental statistics,77 which is currently pursued by official statistics.
Three dimensions are decisive for the quality of statistical information: first, statistical measurement quality; second, theoretical-methodological consistency; and third, relevance for information needs and decisions. Only if all three aspects are achieved satisfactorily, or better ’adequately’, can a statistical number, indicator, graph or map play its role: Because only then it is fit for purpose. The history of the last two hundred years also confirms that it were the forces behind these three spheres of influence (science, society, statistics) that drove the development of official statistics.
Finally, a look at the statistical community itself with the same questions: What are facts? What about the knowledge of the epistemological basics of statistics? Here it turns out that in the circle of professional statisticians reflexive approaches and the understanding of the product characteristics of statistical information are only of rather limited popularity.88 An awareness that the “map is not the territory”99 [15] and that this fundamentally shapes the view of the character, quality profile and purpose of statistics is not very pronounced. For example, the technical term “ground truth” is used for a kind of objective (
First findings can be summarised here:
• Basic statistical training should be grounded on a critical reflection of the subject of statistical information itself. What are facts? If this is clear, the concepts of quality management, communication of quality and a broad improvement of statistical literacy can be approached. Statistical competence and literacy must not be reduced to mathematical-technical skills. Rather, it also requires an understanding of the fundamentals of the humanities [17].
• In addition, it would be highly desirable if terminology were handled more consciously and precisely. Unfortunately, it is common today to use “data” very widely, without distinguishing between raw material (i.e. the data) and end product (i.e. the statistical information). This equalisation creates confusion and makes communication of information quality difficult.
4.From data to policy and retour
After taking this step and characterising statistics as products, we should now analyse its manufacturing method and the process chain through to delivery and use of the end products. If it is a long way from crude oil to the resulting intermediate and end products and the most diverse applications and uses, why should it be different in terms of data, information, knowledge and application? It is only when one understands that on this long journey the most diverse professions and expertise are required, which cooperate one after the other and with each other, that one is able to deal appropriately with the topic of quality.
The growing importance of statistical evidence, data and information for political decisions is reflected in the handy and popular formulation ‘Data for Policy’ (D4P).1111 However, this label is only suitable to a limited extent for characterising the network of relationships and mutual influence between data on the one hand and politics on the other. Even if the amount of data available to inform policy-making (including organisational, administrative decision making in a more general sense) is growing at an enormous rate in the era of digitalisation and globalisation, this raw material of data is not directly usable in politics. Hence, tailored processes are needed to distil, refine and process valuable statistical knowledge from the flood of raw data into digestible information for politics. The term ‘facts’ is used here as a generic term for such information. While statistical data represents the beginning of the processing, facts characterise its end.
With this distinction between data and facts, it is possible to break down the relationship between policy and data into different components that inform policy in different ways (see Fig. 1).
Figure 1.
Data, facts and policy.
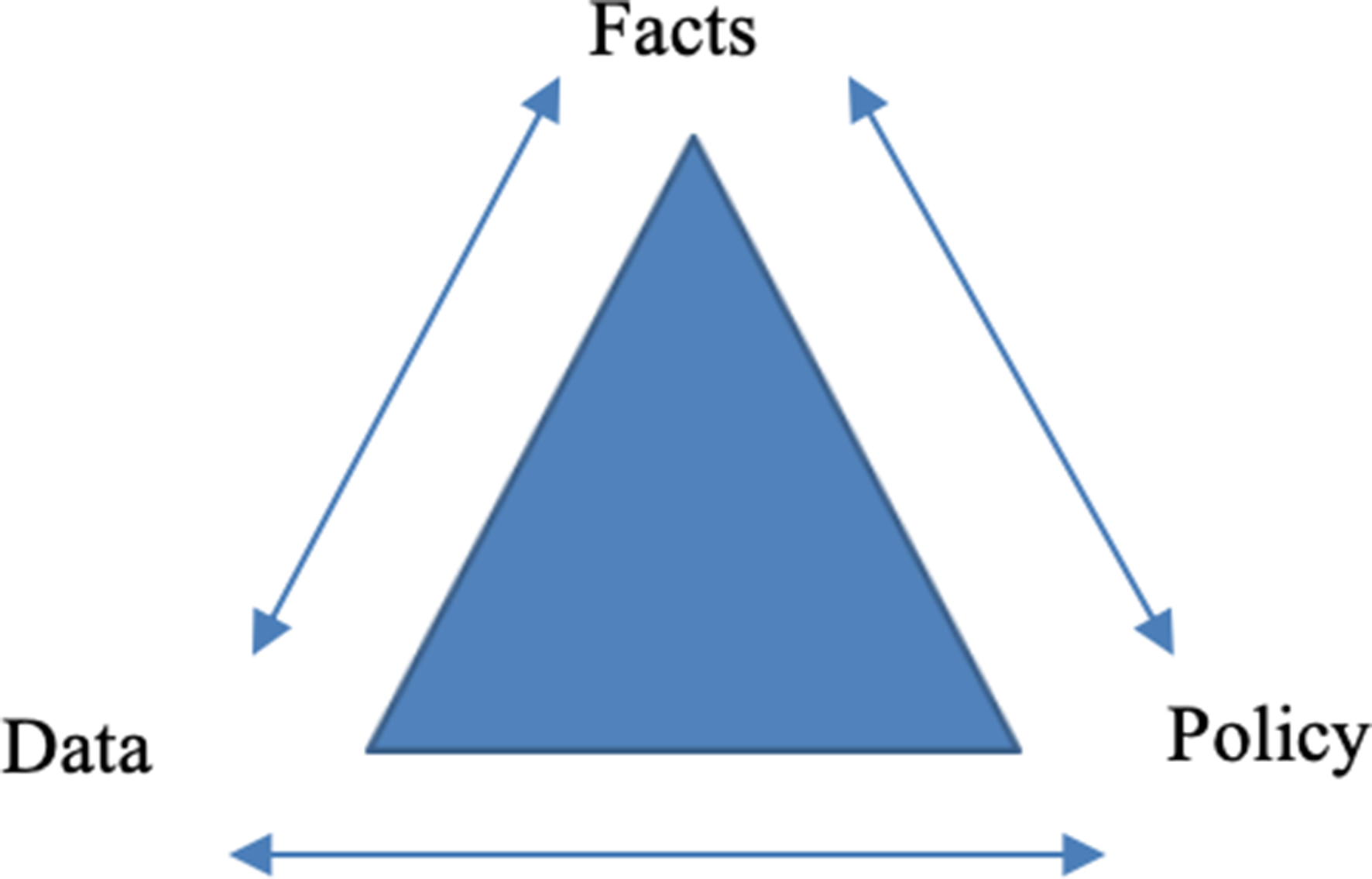
Within this broader relationship, the following fields must be distinguished from each other:1212
Data to facts (D2F): This is the outcome of data analysis, in which statisticians, data scientists, and empirically working researchers/analysts from different disciplines engage. Such facts can be the result of standard statistical processes on the one hand, but on the other hand also, for example, unique research-based evaluations of microdata.
Facts to policy/politics (F2P): This is the field of work of specialists who prepare and use the information content of facts for policy advice. Journalists, researchers and policy analysts focussing on future-oriented models are among them, just as policy-makers engaged in evidence-informed politics.
Policy/politics to facts (P2F): On the one hand, this is about the statistical design (i.e. conceptual form, choice of variables, determination of the work programme etc.) for matters that are of importance to society and are supposed to represent the general information needs; those aspired facts are the outputs of the statistical process in form of indicators, accounts, indices, maps, graphs, etc. On the other hand, it also includes questions of knowledge creation governance (who participates in the design process, who ultimately decides on the selection of the statistical programme, how much money and time is available, etc.).
Facts to data (F2D): This is the scientific and technical conception of the generation or selection of suitable data sources (inputs) to quantify facts (output); questions of authorisation, confidentiality, accessibility and ownership of the data are included.
Policy/politics to data (P2D): In many ways,evidence-informed policies necessitate new data and politics sets the framework conditions for the generation of such new data, including for their protection, for the infrastructure of research institutions or data centres, for the design of legal conditions, such as copyrights. Politics also influences the economic framework conditions under which industries develop innovatively and competitively (or not) in the digital era.
Data to policy/politics (D2P): New data and innovative methods of data-science can be used for experimental statistics which can be helpful in the policy-making process to guide priorities and identify future policy issues. Such a role of “big” data and data scientists as an institution of analysis directly and immediately delivering to politics undoubtedly has its limits and risks. Appropriate governance (reporting, committee work, etc.) for sense-making of such new statistical knowledge needs to be established; complementarity with more standardised statistical production methods needs to be emphasised.
5.Quality: Open, smart and trusted statistics, relevant for the society1414
5.1How can facts be produced with high quality?
Over the past twenty years, a modern quality management system has been established in official statistics based on codified principles, which are implemented in statistical production and adherence to which is checked/certified by external reviewers. Internationally agreed standards and guidelines regarding methodology have already existed before in many of the important statistical areas.
What is crucial is that there is no answer to the question of quality that would be the same and equally correct for all statistics. Rather, a specific solution will have to be found for different areas and different phases in the policy cycle, with different characteristics in the quality profile; this may correspond to a desire for the highest possible accuracy, for high frequency and speed, or for high coherence and consistency. For this reason, the official statistics portfolio contains very different products. To illustrate this with examples, compare the national accounts with the social statistics or the highly aggregated Consumer Price Index with the detailed statistical information in the agricultural sector. What quality entails is above all a result of years, sometimes decades, of development, iterative adaptation, inclusion of new information needs, consideration of resource limitations, etc.; the GDP and its quality can be only assessed against this background.
Despite modern quality management, official statistics are of course not immune to deficiencies and errors. Errors can occur in production, the design of the statistics can be inadequate, the communication may be misleading, the skills of staff may be insufficient. Above all, however, there may be a lack of user confidence in the quality of the facts, whether caused by previous mistakes or due to general scepticism towards government bodies, the media or experts. Mistakes can in principle be countered with three strategies: 1. you can cover them up, 2. you can try to avoid them, or 3. you can try to mitigate their negative effects. Of course, strategy 1 is completely out of the question from the very start; it is destructive to confidence in official statistics and unacceptable. The highest priority must be given to the avoidance of errors. In all cases in which errors have nevertheless occurred, the damage to users must be limited by all means (e.g. transparent, interactive communication, learning loops).
Irrespective of the fact that excellent communication of the facts and figures produced is part of the core business of official statistics, it is becoming increasingly clear that product quality must also be declared and explained in such a way that potential users of the information can orient themselves to it. It is not enough for quality to be high, users must understand and appreciate this. If differences in quality between different products or between different suppliers are not discernible or if they do not count, then everything becomes equally good or equally bad and distrust arises towards statistics in general.
For this reason, it is important to actively pursue the topics of branding (quality marking of a supplier), labelling (quality marking of a product) and certification and to implement them as quickly as possible. It is also necessary to obtain, through market research, the necessary evidence on how statistical products are used by users, where they see their unmet information needs and what contributes to building trust or suspicion.
The basic ideas of a comprehensive, systemic quality management are based on thinkers like Russell L. Ackoff, Peter Drucker and above all W. Edwards Deming. Deming, who was a statistician, by the way, emphasised the importance of managers not interpreting their role purely technically or economically. If they want to be successful, i.e. to produce excellent quality, they must understand their company, its employees, the interrelationships and backgrounds and much more in a profound knowledge. When producing statistics, it is therefore also important to have in-depth professional knowledge of the managers and quality of management.
Interim summary: Quality of statistical information can be achieved through total quality management (TQM1515), which includes all production processes as well as professional marketing. Management of quality, however, requires one thing most of all: quality of management.
5.2Official statistics as part of public administration
We are now turning to a special form of statistics, the so-called official statistics, that is to say statistics produced in offices and made available by them. In order to understand whether and to what extent this is only a subset of applied statistical methodology or whether the framework conditions, the mandate, the form of decision-making and other factors have a significant influence on the quality of the statistics produced, these parameters and their interaction with quality must be analysed.
Official statistics are part of the public administration that provides services that are of fundamental importance for a society. Which services are involved, where the border between the private and public sectors lies and how to ensure that these services are provided with the requested efficiency and effectiveness are questions and topics for which there is more than just one correct answer. Rather, different forms and solutions of governance have emerged over the course of history and for different political cultures. Contrary to the trend observed internationally in recent decades towards the privatisation of health and education sectors, transport and other network infrastructure, periodically recurring discussions about the possible privatisation of official statistics (at least so far) have quickly disappeared into nowhere. For this reason, it can be assumed that official statistics belong to the core of inalienable public services.
But what exactly are the characteristics of this public service? It is clear that we are talking about information, namely information that is held as a public good in an infrastructure for utilisation by citizens, entrepreneurs, teachers, researchers, politicians, or in other words by everyone. If, however, the nature of this area is such that many purposes are involved, how can the information provided be “fit for (multiple) purpose”?
The answer to this question lies firstly in the fact that the various stakeholders and constituencies of civil society are involved in a decision-making process that is concerned with the design of the statistics programme, individual surveys and variables. Secondly, the official statistics programme is a solution to a complex decision-making problem that is regularly and iteratively reviewed and then revised and adapted to new circumstances. The statistics programme today is therefore emergent from a long series of such iterations, definitions of conventions and standards etc. in the past.
Such a solution is comparatively easy to imagine as long as it concerns the society of a country and the corresponding official statistics of that country. In a globalised world, however, it is increasingly an issue that such national optima of the programme no longer meet the requirements; there is a lack of comparability, efficiency and traceability.
This thought leads us to another complex of issues that revolve around the question of how decisions are made regarding the elements of the statistics programme: Who determines the statistical priorities, conventions and standards, how methods, variables are selected, questionnaires are designed, which interests and interest groups are taken into account and how are formal decisions finally made? All these are important elements of statistical governance, which leave their mark on the end products at least as much as the statistical sciences do.
As Alain Desrosières and Theodore Porter have pointed out, (official) statistics, like the nation-state so familiar to us today, were born as a child in the course of the Enlightenment. For two hundred years statistics has been married to the nation-state and has lived through many ups and downs, good and bad times, dictatorships and democracies with the various forms of nation-states. Using the example of the birth of the Italian nation, Silvana Partriarca very clearly highlighted the interrelation between “numbers and nationhood” [19]. It becomes clear that the production of statistics is closely linked to the making of the state and that statistics are an essential prerequisite for any form of government. On the other hand, the governance of a state has an enormous influence on official statistics, their mode of production, their quality, their independence and their proximity (or distance) to citizens.
This mutual relationship also applies to forms which are not nationally organised, such as the European Union, for example, for which there have been corresponding statistical services and institutions at all stages of development. For this reason, in today’s era of globalisation, it is necessary to work out in what way statistics are conceptually geared to the needs, interests and possibilities of individual countries (e.g. the National Accounts) and to what extent they can be used (or not used) as a basis for the development of a new statistical system in those cases where global questions need more internationally aligned statistical answers. At present, it is not possible to foresee the solution to such complex political developments. The extent to which nation-state, multilateral or supranational movements will prove their worth, develop and manifest themselves is unclear today.1616 However, a lesson can be drawn from the common history of the nation state and national statistics: Also for (still unknown) future patterns and structures of political action, be they more global or more regional and local, there will be a need for statistics specially tailored to them. These statistics do not have to be identical in every case and automatically with those currently produced in the individual countries.
Interim summary:
• Governance, political framework conditions and political culture are important quality factors for statistical quality. Independent, strong and innovative statistical institutions can only flourish where good governance principles are sought and where the rule of law applies.
• Global phenomena partly require new statistical approaches and creative solutions, which are detached from national data, methods and frameworks in favouring genuinely international concepts and data sources.
5.3New opportunities and challenges in the era of digitisation and globalisation
5.3.1Three revolutions in the digital age
The digital age is not just a gradual evolution of previous phases of information and communication technology. Rather, a profound change is taking place in society, which fundamentally modifies personal behaviour in everyday life, and leads to completely new mixtures of risks and opportunities, of winners and losers and of consumers and producers concerning data or information [16]. It is spoken of as a data revolution,1717 to clarify the extent of the current structural change; however, technological changes do not happen in a vacuum, but are continually influenced by, and influence themselves social and political conditions, both of which are witnessing major changes. Overall, the following three developments are of prime importance for the future of official statistics:
First: Zettabytes and yottabytes
The era of the data revolution has started, significantly changing the picture with regard to both the production and consumption of data. On one hand, the availability of enormous amounts of data gives the statistical business a completely new push in a direction that is not yet sufficiently understood – although there is growing awareness for the synergies and potentials of close cooperation between statistics and other disciplines of data science [21, 22].
In recent years, the quantity of digital data created, stored, and processed in the world has grown exponentially. Every second, governments and public institutions, private businesses, associations, and even citizens generate series of digital imprints which, given their size, are referred to as ‘Big Data’.1818 The wealth of information is such that it has been necessary to invent new units of measurement, such as zettabytes or yottabytes, and sophisticated storage devices purely to deal with the constant flow of data. The world can now be considered as an immense source of data. Broad consensus reigns with regard to the wonderful opportunities which ‘Big Data’ can bring in relation to the statistics acquired from traditional sources such as surveys and administrative records. These opportunities include:
• Much faster and more frequent dissemination of data.
• Responses of greater relevance to the specific requests of users, since the gaps left by traditional statistical production are filled.
• Refinement of existing measures, development of new indicators, and the opening of new avenues for research.
• A substantial reduction in the burden on persons or businesses approached and a decrease in the non-response rate.
• Last, but not least, access to ‘Big Data’ could considerably reduce the costs of statistical production, at a time of severe cutbacks in resources and expenditure.
However, ‘Big Data’ also threatens a number of challenges:
• These data are not the result of a statistical manufacturing designed in accordance with standard practice and executed by supervised production processes, which causes quality risks as a result of a loosing of control.
• They do not fit current methodologies, classifications and definitions, and are therefore difficult to harmonise and convey in the existing statistical structures.
• Complex aggregates, such as the Gross Domestic Product (GDP) or the Consumer Price Index (CPI) aim at measuring macro-economic indicators [24] for the nation as a whole; their substitution by big data sources seems to be out of reach.
• In addition to this, ‘Big Data’ raises many major legal issues: security and confidentiality of data, respect for private life, data ownership, etc.
All of the above mean that, at least for now, ‘Big Data’ can only be used to a limited degree to supplement, rather than replace, sources of traditional data in certain statistical fields.
Second: Evidence and decisions
On the other hand, the demand for ‘evidence-based decision making’, (new public) management, and other applications of a neoliberal governance model [25] create a powerful driving force on the demand side of statistics. It can be recognised as an ‘ingredient of rationality’ [26] to take into account the consequences of a decision. It is a long way from this ‘Enlightenment’ viewpoint to a form of governance in which the availability of evidence is considered a prerequisite for any decision. ‘During the past hundred years or so, political governance underwent a massive “quantitative turn.” This quantitative turn is here understood as systematic effort to delineate and measure the objects and results of governance quantitatively for the purpose of demonstrating competitive edge and superiority at the individual and/or collective level.’ [27]. Now, from a statistical point of view, it seems almost desirable that this quantitative turn has led to a greater demand and supply of statistics, if there were not a number of side effects, which could endanger the quality of statistical information or could even be a threat to official statistics. If it is true that ‘measurement is a religion in the business world’ [28], this religion not only has a significant impact on the behaviour of managers, civil servants, and public and private sector employees, but rather, it also creates a hunger for data that is not matched by the appetite for good quality. In such an “audit society” [3], there is a great danger that the existence of data and information is assumed to be normal. That these informational products must be produced, that they can have indifferent quality, and that producing them costs time and money, is quickly pushed into the background when it comes down to having any data whatsoever available. Paradoxically, this same information society complains that the burden of statistics is too high. In all of this, it becomes clear that significant risks to statistics can arise because expectations concerning their quantity are too high, while those concerning their quality are too low. It is difficult to sustain a high-quality profile of products in a fast food culture.
All these trends, which have emerged in recent decades, are being accelerated by new technologies. Decisions which were ‘augmented’ by the use of evidence, might now become ‘automated’. The ‘Internet of Things [29], Artificial Intelligence (AI), and the growing importance of algorithms are posing new questions in areas other than technological ones:1919 ‘Society must grapple with the ways in which algorithms are being used in government and industry so that adequate mechanisms for accountability are built into these systems. There is much research still to be done to understand the appropriate dimensions and modalities for algorithmic transparency, how to enable interactive modelling, how journalism should evolve, and how to make machine learning and software engineering sensitive to, and effective in, addressing these issues.’ [31].
Third: Facts and alternatives
Information and facts are not neutral. Just as other manufactured products, they open manifold possibilities of ‘dual’ use and of risks which must be anticipated by responsible information producers in their policies and production processes. One of the key questions that, again, has to be asked, is related to the role that sciences have played in the past, and in how far this role needs to be critically assessed and revised [32, 33].
While uncertainties and risks are constantly growing in the eyes of citizens, and while the impact of globalisation becomes more and more visible, it appears as if people have had enough of experts [34]. It also appears as if ‘post-truth-politics’ would gain credibility and support, opening opportunities for populist and nationalist activists of all kinds. The trust of the population in their governments, and in official institutions in general, is rapidly decaying, and this lack of trust is naturally extended to the producers of official statistics.
Citizens ask themselves what use statistical indicators serve, and for whose benefit. Knowledge is power. Is statistical evidence used to stimulate political dialogue (opening up), to shorten it, or, in the worst case, to suppress it (closing down)[24, 35]? Depending on how these questions are answered, statistics will win or lose citizens’ trust. The closeness of official statistics to politics and their embeddedness in public administration can have both positive and negative consequences, depending on the perception of their use in political decision making and their professional independence.
In this context, a profound epistemological shift is needed since complexity and irreversibility undermine the idea that science (and statistics) can provide single, objective, and exhaustive answers. In the late modernity of risk societies [33], there is the epistemic and methodological necessity to empower people – citizens and policy makers – with the appropriate insight, to enable them to make the best possible decisions for achieving sustainability and pursuing resilience in a complex world.
5.3.2Answers to a dramatically changing environment
The continuous, bottom-up improvement of processes, technologies, and data sources that has characterised the last decades of official statistics is not enough in such an era of dramatic changes. The completely new, competitive situation requires official statistics to provide innovative strategic answers that go beyond traditional statistical methods and technologies. The core of this will be to maintain (or, if already lost, to win back) trust in official statistics, both as an institution and as an information infrastructure, in the face of scepticism towards politics and state institutions.
In the age of Big Data, AI, and algorithms, a need exists for ethical guidance and legal frameworks under new conditions: ‘In the world being opened up by data science and artificial intelligence, a version of the basic principle of the partnership between humans and technology still holds. Be guided by the technology, not ruled by it’ [36]. What might facilitate the perceived new search for orientation and balance is the stock of ethical and governance principles that is available, emerging from two centuries of history in official statistics.
For some years, especially in the field of environmental data, a new form of cooperation between science and citizens, called ‘citizen science’, is developing.2020 Citizen science projects actively involve citizens (as contributors, collaborators etc.) in scientific endeavour that generates new knowledge or understanding.2121 Although citizen science is still relatively young, it hits the point, which is becoming increasingly important for official statistics. The past distinction between producers of data and consumers makes less and less sense. Consequently, the question arises of how to actively involve citizens in the production of statistics throughout the entire production chain, from design to communication. In the past, citizens (as well as companies and many other partners of statistics),2222 were either passive respondents in surveys and/or simply consumers of ready-made statistical information. The answer to this question is anything but trivial. At its core are the same problems and difficulties as the issue of using Big Data for official statistics in general: control of procedures, quality assurance, interpretability of information, and neutrality/impartiality.
5.3.3Launching a new, scientific debate
It seems to be both necessary and urgent to launch a scientific debate in professional communities and initiate a period of reflection. Scientific research and development are essential to the quality of measurements and their results, whether they are based on statistical survey methodologies, or driven by data science concepts. Apparently, this relates in the first place to the relevant technical disciplines. However, this should be supplemented by going beyond pure methodologies, by taking on board aspects from other fields, such as sociology, historical, or legal disciplines. There are many different strands of science contributing research on processes of quantification, and the impact of quantification within social contexts [40]. Those scientific inputs should address questions and issues such as:
• Phases in the history of official statistics having the potential to explain the interaction between knowledge generation and society; the making of states; statistics under authoritarian, liberal, and neoliberal regimes.
• Official statistics as part of a knowledge base for life.
• Historical, cultural, and governance systems of countries; differences between statistical authorities, and their performance across the globe compared to in Europe; international/supranational governance in statistics.
• Creation of knowledge; measurement in science and practice; limits of measurement; facts and (science) fiction; statistics and theories, such as economic theory; epistemology, falsification/veri-fication of theories.
• Use, misuse, and abuse of evidence; the power of knowledge and how to share it; relationship to conceptual frames in politics.
• Public value in the context of public administration; participation of citizens via effective and efficient mechanisms.
• (New) Enlightenment; knowledge for the empowerment of citizens; citizen science; statistical literacy; education; participation in decision making; fostering the democratic process.
• Communication of data and metadata, and quality for users with unequal pre-knowledge and statistical literacy.
• Framing of indicators as a co-design process that activates the interest of civil society.
• Co-production of statistics; turning users of statistics into co-producers (‘prosumers’).
• Quality of information, institutions, products, and processes; how to decide on conventions about methodologies and programs of work; quality assurance.
• Professional ethics (for individuals), and good governance (for institutions).
• Professional profiles: survey methodologist, data scientist, accountant, data architect, social science engineer, etc.
5.4Strengthening civil society’s role
5.4.1Bridging the gap
How can we best bridge the gap between the public (the ‘citizen’) and statisticians? Is it enough to focus on improving the communication of statistical results? Is the problem to be solved purely one of language? Or do we need to start further upstream in the sequence of processes of measurement/quantification2323 (design, production, communication), and address the production of statistics, as well as the process of knowledge creation by users? Does the communication of the future perhaps require more participation? If so, who should participate and how should this be done in practice?
In the following, some approaches will be pursued that focus primarily on mainstreaming users and their interests throughout the production process. Most importantly, however, it is a question of fostering a greater involvement of civil society; that is to say, the general public are, on the whole, somewhat distanced from official statistics and valuable statistical information, so a bridge must be built in order to overcome that distance. Providing better information to users and non-users, and being able to counter their misjudgements and prejudices with facts, is probably the part of the statistical mission that has the greatest added social value. According to the legacy of Hans Rosling,2424 that mission is about education and providing information that is orientated towards the layperson. However, it should also be about co-design and co-production, through which the participation of the public in statistical results should be the aim.
Of course, the involvement of users and their interests has always played a significant role in official statistics. During the development and revision of both the statistics programme and of individual statistics, user advisory councils are consulted, scientific colloquia are organised, and, finally, legal decision-making processes are followed. The critical aspect here is that it is essentially a very narrow selection of experts and stakeholders who are involved in such consultation processes.
The dissemination of statistical information has undergone a complete transformation in recent years. This has started with the fact that the term ‘dissemination’ is now largely shunned and has been replaced with ‘communication’. In place of a publication programme producing a single flagship Statistical Yearbook, a series of individual, specialised, and very wide-ranging (printed or online) books has emerged. These are geared towards online media and have social networks as integrated distribution channels. Statistical offices commonly have an internet presence and websites prepared for diverse user groups as standard. Interactive communication tools and mobile applications facilitate access, even for the layperson.
Nevertheless, there is more to do. With reference to the still relatively young discipline of ‘citizen science’,2525 we need to understand the circumstances that have led to the mistrust of the elite in Western society, and the way that statistics are (or are at least perceived to be) an instrument of both the political/administrative elite and the scientific elite. William Davies’ analysis [42] could be taken as a starting point for reflection on the challenges and opportunities brought by this rapidly changing environment. A few of his observations, all of which add up to a general mistrust of official statistics, are as follows:
• Naïve use of indicators by politicians or misunderstanding of indicators by a society with a poor level of statistical literacy can create:
– Incorrect opinions,
– Which may mislead voters, or,
– Compel politicians to take non-optimal measures.
• Advocating the objectivity and expertise of technocrats as a better choice than the regime of demagogues/politicians is associated with the following risks:
– High-level aggregated artefacts (e.g. GDP) may be too abstract in their design and meaning for the average layperson,
– Ex ante/top-down classifications are out oftouch with the identities of individuals,
– National policies are too distant from individuals and their private spheres,
– In our era of big data, data-driven logic (the inductive search for messages in the data) has replaced statistical logic (top-down design of classifications and variables to be surveyed),
– Social network bubbles undermine the existence of facts.
The public’s mistrust of elites and technocrats, and their sympathy for demagogues and populists, may not seem rational.2626 Nonetheless, it is a real, international, and serious phenomenon of our current time.
What are the consequences for official statistics, if confidence in public institutions is generally shrinking, if the authority of the state and its representatives is questioned, and if facts are no longer seen as being without alternative?
The circular flow of statistical processes (design, production, communication, use) needs to be reviewed, wherever possible, aiming to bring on board both stakeholders and civil society: in their design (e.g. the early involvement of the public regarding new indicators and data platforms during their planning stages; human-centred co-design), in their production (e.g. crowd-sourcing of data; co-production) and in their communication (which should be interactive, open, accessible etc.) and in their use (by collecting evidence through market research of the use/misuse/non-use of indicators, by creating user-specific feedback loops, and by improving statistical literacy).
In the future-orientated involvement of users the mental separation between the producers and the consumers of statistics needs to be removed. To achieve this, it is necessary to anchor the goal of involving civil society as deeply as possible in the production process. The most important thing to do first is make people aware of the importance and consequences of statistics and numbers in their own lives and societies. A more fare reaching objective would aim at consumers becoming co-producers (‘prosumers’); stakeholders becoming shareholders. Similar to the introduction of the primacy of existing data over new surveys in the 2000s, change needs to be achieved in a well-rehearsed and conservative-thinking sphere; patterns must be maintained by defining strategic goals. The strategic goal here is to intensify the partnership between civil society and statistics in all the stages of the latter: in the scientific and design phase, during production, and – most importantly – through communication. A small selection of examples shall illustrate this approach:
• Co-production of statistics – participatory data
– The potential of ‘big data’ arising from any possible sources is examined by official statistics. These data are generated for specific purposes or result from technical processes. In any case, the information content for statistics must first be distilled from the dataset. In this context, approaches and ideas from the field of citizen science,2727 which aim at an active participation of volunteers in the collection of data, should be further examined.
– This form of participatory filing and sharing of data and knowledge has gained momentum, especially in the areas of environment and sustainable development [44]. For example, the homepage of WeObserve states: ‘WeObserve is a Coordination and Support Action which tackles three key challenges that Citizens Observatories (COs) face: awareness, acceptability and sustainability. The project aims to improve the coordination between existing COs and related regional, European and international activities. The WeObserve mission is to create a sustainable ecosystem of COs that can systematically address these identified challenges and help to move citizen science into the mainstream’.2828
• Participation in indicator design
– In 2010, the UK Statistics Service was commissioned to develop and publish a set of National Statistics to understand and monitor well-being. After the programme was launched with a national debate on ‘What matters to you?’, to improve understanding of what should be included in measures of the nation’s well-being, and after a discussion paper had summarised the output of this phase, an online consultation2929 was opened up to the wider public. This sought views on a proposed set of domains (aspects of national well-being) and headline indicators. The online consultation was open for participation between November 2010 and January 2011.
– One of the challenges of such a process is to communicate in a plausible manner that there are ‘participatory parts’ and more ‘technical parts’. Nevertheless, such a public and open consultation can make an additional contribution to bringing the design of new indicators out of the sphere of experts and insiders by informing citizens as early as possible and taking account of their opinions.
– However, one must consider that consultation fatigue may arise among the addressees. A consultation by scientific experts in the field of co-design3030 is therefore necessary for the success of such a project.
• Market research
– In order to constantly develop the quality of indicators and other statistical products, it is necessary to obtain the most precise information possible about their use, misuse or non-use.3131 The application of professional methods of market research should provide evidence that is important for the product design of the future.
• Finally, it is self-evident that the efforts to improve the communication of statistics, which have already been considerably intensified in recent years, need to be sustained and refined. With respect to increase ‘datacy’ and statistical literacy, it is for example necessary to make further progress in communicating uncertainty.3232
5.5Necessary adaptations of statistical governance
5.5.1The data-information-knowledge nexus and official statistics
As an adequate approach for reacting to the rapidly transforming political landscape caused by the digital revolution, globalisation, the crisis of the nation-state and the changing position of science in society, Soma et al. [12] widen the concept of governance in order to introduce an ‘informational governance’,3333 outlining this ‘along four interrelated themes:
• Processes of information construction: how “governing through information” appears and influences institutional change,
• Information processing through new technology, for example, social media: how information construction through use of new technology affects diversification of future governance arrangements,
• Qualities of transparency and accountability: how “governing through information” appears and influences institutional change,
• Fourth, institutional change: how new institutional arrangements for governing are emerging in the Information Age as a matter of new ICT developments, globalization, as well as new roles of state and science.’
They further state that: ‘growing uncertainties and complexities are partly caused by difficulties in controlling information flows in the more globalised world. Because the state and science increasingly are lacking the authority to unilaterally solve controversies bound up with politics and struggles on knowledge claims, problems of definitions, trust and power are increasing’ [12]. Central to all considerations for statistical governance is the entirely reviewed role of citizens [39].
In essence, the situation of official statistics will continue to be determined by techniques (tools), ethics (patterns of behaviour) and politics (questions of institutional set-up or communication). However, in rapidly changing circumstances, it is important that official statistics services play their important social role by adequately adapting the rules, principles and resources that shape their working conditions. They should be enabled to act proactively in the sense of educating liberal democratic societies [50].
‘In January 2018, the European Commission set up a high-level group of experts (“the HLEG”) to advise on policy initiatives to counter fake news and disinformation spread online. The main deliverable of the HLEG was a report designed to review best practices in the light of fundamental principles, and suitable responses stemming from such principles’ [51]. A review of statistical governance requires a broad approach, similar to that chosen here for the media. Stakeholders of official statistics should jointly define a multi-dimensional approach to address the issues ahead and constructively continue the successfully established governance of statistics.
Table 1
Annex 1 data, facts, policy: Actors and activities
Data | Facts | Policy/politics | |
---|---|---|---|
Data |
Data to facts (D2F)
|
Data to policy/politics (D2P)
| |
Facts |
Facts to data (F2D)
|
Facts to policy/politics (F2P)
| |
Policy/ politics |
Policy/politics to data (P2D)
|
Policy/politics to facts (P2F)
|
In the rapidly evolving variety of data science disciplines, there is a considerable risk that existing knowledge and established structures will go unused in governance and that the wheel will be reinvented many times. Essentially, the goal of governance is to create and maintain trust in information and, where lost, to regain it. But if such fundamental principles are neglected by other information producers and if unrealistic expectations are created,3434 then it is difficult for users to recognise differences in quality.
The question therefore arises as to whether the statistical governance structures dating back to the discussions of the 1990s,3535 which focused on nationally organised public administrations of statistics with their risks, still meet today’s challenges. Rather, it seems sensible and necessary to subject these fundamental principles to a review and revision process.
Official statistics services are therefore required to take the initiative here, to contribute their knowledge and to play an active, coordinating and integrating role in the discussion between different disciplines.
Questions that need to be addressed and answered (amongst others) are:
• Informational governance for the data sciences: lessons to be learnt and transferred from statistics.
• Statistics and data science in public administration: who is responsible for what?
• Professional values and ethics, revision of the international ethical codes and governance standards, evaluation of the status quo, analysis, gaps, recommendations, ethics for all three core statistical processes (design, production, communication).
• Governance for different types of statistical products (indicators ‘with authority’; indicators, accounts, statistics and their quality profiles; experimental statistics).
• From formal, administrative legitimacy of official statistics to social acceptance as a reference point.
• Ethics for decision-makers and their scientific services.
• Statistical competence: intensified cooperation between the education system (including vocational training) and official statistics services.
• Official statistics services’ obligations and rights in the data economy (B2G, G2B, G2G).
• International statistical governance.
– Global conventions that go beyond today’s recommendations,
– A new regulatory framework for access to privately owned data for official statistics.
• International monitoring of governance issues and in particular professional independence in countries.
6.Conclusion
Official statistics fulfil an essential duty for the functioning of democratic societies. As in the past, statistics will also be utilised for the making of the future state(s), may it be for good or bad. As in the past, official statistics will continue to be required to reconcile three fundamental rights: the right to privacy of individual data, the right of access to information and the right to live in a civilized society provided with sound information. This is a great responsibility that official statistics must live up to by reflecting their production methods scientifically and at the same time fighting for their appropriate place in the informational ecosystem of globalised societies.
The label ‘Data for Policy’ is only suitable to a limited extent for characterising the network of relationships and mutual influence between data on the one hand and politics on the other. Even if the amount of data available to inform decision-making is growing at an enormous rate in the era of digitalisation and globalisation, this raw material of data is not directly usable in politics. Hence, tailored processes are needed to distil, refine and process valuable statistical knowledge from the flood of raw data into digestible information for politics.
Data are given, facts are made. While data represent the beginning of the processing, facts characterise its end. Terminology must be unambiguous; it must be chosen professionally as well as consciously and has great importance in communication. Unfortunately, it is common today to use ‘data’ very widely, without distinguishing between raw material (i.e. the data) and end product (i.e. the facts). This equalisation creates confusion and makes communication of information quality difficult. The worst thing, however, is that this fuzziness is detrimental to the profile of statistics in the informational ecosystem.
Basic statistical training should be grounded on a critical reflection of the subject of statistical information itself. What are facts? If (and only if) the answer to this question is clear, the concepts of quality management, communication of quality and a broad improvement of statistical literacy can be approached. Statistical competence and literacy must not be reduced to mathematical-technical skills. Rather, it also requires an understanding of the fundamentals of the humanities.
Quality of statistical information can be achieved through total quality management, which includes all production processes as well as professional marketing. Management of quality requires one thing most of all: quality of management.
Scientific research and development are essential to the quality of statistics, whether they are based on survey methodologies, or driven by data science concepts. Apparently, this relates in the first place to the relevant technical disciplines. However, it is equally necessary to take on board aspects from other fields, such as sociology, historical, or legal disciplines. There are many different strands of science contributing research on processes of quantification, and the impact of quantification within social contexts.
Participation of civil society needs to be anchored in the working culture of the statistical factory. The strategic goal here is to intensify the partnership between civil society and statistics in all the stages of the manufacturing: in the scientific and design phase, during production, and – most importantly – through communication.
The power of numbers will increase dynamically with new data sources and technologies. Data for policy call for a comprehensive policy for data at both national and especially international level. Official statistics can and must claim a decisive role in this Policy for Data. Building on existing legal and codified principles and rules, further developments should be actively pursued to meet the new challenges. A global organisation of professional statistics anchored in civil society should monitor the independence and integrity of statistics in individual countries and develop a suitable indicator.
The development of official statistics has been and continues to be influenced by new data, by new methods or by society’s new information needs. We are now seeing all three driving forces changing very rapidly, even dramatically. With a forward-looking strategy, official statistics should seize the existing opportunities to remain what they are: policy relevant, but not politically driven.
Notes
1 White Paper “Modernising Government”. 1. The Prime Minister and the Minister for the Cabinet Office. White Paper: Modernising Government. White Paper presented to the Parliament. London: The Prime Minister and the Minister for the Cabinet Office, United Kingdom; 1999 30 March 1999.
2 For all quotations see https://www.data4policy.eu/.
3 The interaction between risk assessment (assumed as a technical and independent process) and decision making (assumed as dependent on this evidence and building on it) is very clearly illustrated in the movie “Eye in the Sky”: “Seeking authorisation to execute the strike, (British Army Colonel) Powell orders her risk-assessment officer to find parameters that will let him quote a lower 45% risk of civilian deaths. He re-evaluates the strike point and assesses the probability …at 45–65%. She makes him confirm only the lower figure, and then reports this up the chain of command. The strike is authorised, and …a missile is fired” https://en.wikipedia.org/wiki/Eye_in_the_Sky_ (2015_film).
4 A quote attributed to W. Churchill (https://www.goodreads.com/ quotes/300097-i-only-believe-in-statistics-that-i-doctored-myself) although source research has shown that this assignment itself is a ‘fake’ (https://www.destatis.de/GPStatistik/servlets/MCRFileNode Servlet/BWMonografie_derivate_00000083/ 8055_11001.pdf).
7 See for example https://ec.europa.eu/eurostat/web/experimental-statistics.
8 See Hannah Fry “Maths and tech specialists need Hippocratic oath, says academic” https://www.theguardian.com/science/2019/ aug/16/mathematicians-need-doctor-style-hippocratic-oath-says-academic-hannah-fry?CMP=Share_iOSApp_Other.
9 As remarked by Alfred Korzybski, see for example https://fs. blog/2015/11/map-and-territory/.
10 Ian I. Mitroff: “The biggest downfall of Expert Agreement is that it assumes that one can gather data, facts, and observations on an issue or phenomenon without having to presuppose any prior theory about the nature of what one is studying. It assumes that data, facts, and observations are theory and value-free. It’s not just that one can’t interpret anything without a theory of some kind, but even more basic, one can’t collect any data in the first place without having presupposed some understanding and/or theory about the phenomenon that underlies the data, certainly why this particular set of data is important to collect and how they should be collected so that they accurately reflect the “true nature of phenomenon.”16. Mitroff II. Technology Run Amok- Crisis Management in the Digital Age. Cham: Palgrave Macmillan; 2019.
11 Or in even more demanding as in the motto of the World Statistics Day “Better Data, Better Lives” https://www.un.org/en/ events/statisticsday/, concretely applied for example in OECD’s “Better Life Index” https://www.un.org/en/events/statisticsday/.
12 See also the tabular summary in Annex 1.
14 The observations in this section are described in more detail in Radermacher, W. J., “Official Statistics 4.0- Verified Facts for people in the 21
15 The statistician W. E. Deming developed TQM based on his “System of Profound Knowledge” with four parts, all related to each other. These four parts are: system, variation, theory and psychology. See https://blog.deming.org/2012/10/demings-system-of-profound-knowledge/.
16 Jürgen Habermas: “The sceptics doubt this with the argument that there is no such thing as a European “people” that could constitute a European state. On the other hand, peoples only emerge with their state constitutions. Democracy itself is a legally mediated form of political integration. Certainly, this in turn depends on a political culture shared by all citizens. But if one considers that in the European states of the 19th century national consciousness and civic solidarity have only gradually been generated with the help of national historiography, mass communication and conscription, there is no reason for defeatism.” 20. Habermas J. Der europäische Nationalstaat unter dem Druck der Globalisierung. Blätter für deutsche und internationale Politik. 1999; 1999(4): 425–36.
17 See for example the data revolution group, established by the UN (http://www.undatarevolution.org/).
18 For a more elaborated definition of ‘Big Data’ see Steve MacFeely “Big Data and Official Statistics”. 23. MacFeely S. Big Data and Official Statistics. In: Kruger Strydom S, Strydom M, editors. Big Data Governance and Perspectives in Knowledge Management. Hershey, PA: IGI Global; 2018. p. 25–54.
19 See the report ‘For a meaningful artificial intelligence – Towards a French and European Strategy’ 30. Villani C. FOR A MEANINGFUL ARTIFICIAL INTELLIGENCE- TOWARDS A FRENCH AND EUROPEAN STRATEGY. Mission assigned by the Prime Minister Eìdouard Philippe. Paris: AI for Humanity- French Strategy for Artificial Intelligence; 2018. p. 151.
20 See for example Haklay, Citizen Science and Policy: A European Perspective“ 37. Haklay M. Citizen, Science and Policy: A European Perspective. case study series. 2015; 4:[61 p.]. Available from: https://www.wilsoncenter.org/publication/citizen-science-and-policy-european-perspective.
21 See 38. ECSA. ECSA Policy Paper #3 Citizen Science as part of EU Policy Delivery- EU Directives2016:[4 p.]. Available from: https://ecsa.citizen-science.net/documents.
22 See 39. Soma K, Onwezen MC, Salverda IE, van Dam RI, Roles of citizens in environmental governance in the Information Age – four theoretical perspectives. Current opinion in Environmental Sustainability. 2016; 2016 [18]:122–30.
23 ‘However, till very recently, very few studies have questioned the figures they used, as if these figures were simply measuring a pre-existing reality. To prevent this “realist epistemology”, Alain DesrosieÌres, who is the founder of a new way of thinking about statistics, proposed to talk not about “measurement” but about “quantifying process”: “The use of the verb ‘to measure’ is misleading because it overshadows the conventions at the foundation of quantification. The verb ‘quantify’, in its transitive form (‘make into a number’, ‘put a figure on’, ‘numericize’), presupposes that a series of prior equivalence conventions has been developed and made explicit […]. Measurement, strictly understood, comes afterwards […]. From this viewpoint, quantification splits into two moments: convention and measurement.”’ 41. Eyraud C, Stakeholder involvement in the statistical value chain: Bridging the gap between citizens and official statistics. In: Eurostat, editor. Power from Statistics: data, information and knowledge- outlook report- 2018 edition. Luxembourg: Publication Office of the European Union; 2018. p. 103–6.
24 Hans Rosling was a physician and statistician who, with his passion and his gift for explanation, managed to portray statistics completely new ways and use completely new dimensions of communication; he died in February 2017 (https://www.theguardian.com/ global-development/2017/feb/07/hans-rosling-obituary and https:// www.gapminder.org/).
25 See Haklay [2015], Citizen Science and Policy: A European Perspective 37. Haklay M. Citizen Science and Policy: A European Perspective. case study series. 2015; 4:[61 p.]. Available from: https://www.wilsoncenter.org/publication/citizen-science-and-policy-european-perspective.
26 See for example Chris Arnade’s blog ‘Why Trump voters are not “complete idiots”’ 43. Arnade C. Why Trump voters are not “complete idiots” 2016 [Available from]: https://medium.com/ @Chris_arnade/trump-politics-and-option-pricing-or-why-trump-voters-are-not-idiots-1e364a4ed940#.faldoe9vg.
27 1. Principle of citizen science Citizen science projects actively involve citizens in scientific endeavour that generates new knowledge or understanding.https://ecsa.citizen-science.net/sites/default/ files/ecsa_ten_principles_of_citizen_science.pdf.
28 See https://www.weobserve.eu/ 45. WeObserve. An Ecosystem of Citizen Observatories for Environmental Monitoring: WeObserve; 2018 [Available from: https://www.weobserve.eu/.
29 http://webarchive.nationalarchives.gov.uk/20120104115644/http://www.ons.gov.uk/ons/about-ons/consultations/open-consultations/measuring-national-well-being/index.html.
30 46. Joost G, Unteidig A. Design and Social Change: The Changing Environment of a Discipline in Flux. In: Jonas W, Zerwas S, Anshelm Kv, editors. Transformation Design Perspectives on a New Design Attitude: Birkhäuser; 2015, 47. Gericke K, Eisenbart B, Waltersdorfer G. Staging design thinking for sustainability in practice: guidance and watch-outs. In: König A, editor. Sustainability science. New York: Routledge; 2018. p. 147–66, 48. Hisschemoller M, Cuppen E. Participatory assessment: tools for empowering, learning and legitimating? In: Jordan AJ, Turnpenny JR, editors. The tools of policy formulation: Edgar Elgar; 2015. p. 33–51.
31 Lehtonen, The multiple roles of sustainability indicators in informational governance: Between intended use and unanticipated influence 49. Lehtonen M, Sébastien L, Bauler T. The multiple roles of sustainability indicators in informational governance: Between intended use and unanticipated influence. Current Opinion in Environmental Sustainability. 2016; 2016(18):1–9.
32 See for example Eurostat’s project “COMmunicating UNcertainty In Key Official Statistics” (https://ec.europa.eu/eurostat/ cros/content/communicating-uncertainty-key-official-statistics_en) or UK Government Statistical Service’s “Communicating quality, uncertainty and change” (https://gss.civilservice.gov.uk/policy-store/communicating-quality-uncertainty-and-change/).
33 ‘The concept of informational governance has emerged to capture these new challenges of environmental governance in the context of the Information Age. The logic of informational governance stems from the observation that information is not only a source for environmental governance arrangements, but also that it contributes to transformation of environmental governance institutions. Such societal transformation refers to how the raise of information technology, flows and networks leads to a fundamental restructuring of governance processes, structures, practices and power relations. ’ 12. Soma K, MacDonald BH, Termeer CJ, Opdam P. Introduction article: informational governance and environmental sustainability. Current Opinion in Environmental Sustainability. 2016; 2016 (18): 131–9.
34 Wigglesworth, Can big data revolutionise policymaking by governments? 52. Wigglesworth R, Can big data revolutionise policymaking by governments? FinancialTimes. 2018 31.01.2018.; Heubl, Night light images paint accurate picture of China GDP 53. Heubl B. Night light images paint accurate picture of China GDP. NIKKEI ASIAN REVIEW. 2018 March 24, 2018.
35 See in particular William Seltzer’s paper “Politics and Statistics: Independence, Dependence, or Interaction?” 54. Seltzer W. Politics and Statistics: Independence, Dependence, or Interaction? In: Analysis UDoEaSIaP, editor. New York: UN; 1994.
References
[1] | The Prime Minister and the Minister for the Cabinet Office. White Paper: Modernising Government. White Paper presented to the Parliament. London: The Prime Minister and the Minister for the Cabinet Office, United Kingdom; 30 March (1999) . |
[2] | Rose N. Governing by numbers: Figuring out democracy. Accounting, Organization and Society. (1991) ; 16: (7): 673-92. |
[3] | Power M. The Audit Society, (1994) . |
[4] | van Veenstra AF, Kotterink B. Data-driven policy making: The policy lab approach. In: (eds.) PPea, Editor. Electronic Participation ePart 2017 Lecture Notes in Computer Science. Cham: Springer; (2017) 10429. |
[5] | Verhulst SG, Zeynep E, Crowcroft J. Data & Policy: A new venue to study and explore policy-data interaction. Data & Policy. (2019) ; 1: (e1): 1-5. |
[6] | Turner F. Machine politics - the rise of the internet and a new age of authoritarianism. Harper’s Magazine. (2019) ; 25-33. |
[7] | Desrosières A. The politics of large numbers – A history of statistical reasoning. Cambridge Massachusetts: Harvard University Press. (1998) . |
[8] | Porter TM. Trust in numbers: The pursuit of objectivity in science and public life. Princeton, N. J.; Chichester: Princeton University Press. (1995) . |
[9] | Prévost J-G. Politics and policies of statistical independence. In: Prutsch MJ, Editor. Science, Numbers and Politics. Cham: Palgrave Macmillan. (2019) ; 153-80. |
[10] | Doorn Mv. How Wrong Ideas about Knowledge Can Ruin Decisions. Medium – the Understanding Project [Internet]. (2019) . Available from: https://medium.com/the-understanding-project/how-wrong-ideas-about-knowledge-ruin-decisions-ab6f1ed197ab. |
[11] | Bartl W, Papilloud C, Terracher-Lipinsky A. Governing by numbers-key indicators and the politics of expectations. Historical Social Reasearch. (2019) ; 44: (2): 1-339. |
[12] | Soma K, MacDonald BH, Termeer CJ, Opdam P. Introduction article: Informational governance and environmental sustainability. Current Opinion in Environmental Sustainability. (2016) ; 2016: (18): 131-9. |
[13] | Hendricks VF, Vestergaard M. Postfaktisch- die neue wirklichkeit in zeiten von bullshit, fake news und verschwöruungstherien. München: Karl Blessing Verlag. (2018) . |
[14] | Box GEP. Science and statistics. Journal of the American Statistical Association. (1976) ; 71: (356): 791-9. |
[15] | Wuppuluri S, Doria FA. The map and the territory: Exploring the foundations of science. Thought and Reality: Springer International Publishing. (2018) . |
[16] | Mitroff II. Technology run amok- crisis management in the digital age. Cham: Palgrave Macmillan. (2019) . |
[17] | Vestberg H. Why we need both science and humanities for a Fourth Industrial Revolution education2018 09.08.2019. Available from: https://www.weforum.org/agenda/2018/09/why-we-need-both-science-and-humanities-for-a-fourth-industrial-revolution-education/. |
[18] | Radermacher WJ. Official statistics 4.0 – Verified Facts for People in the 21st Century. Heidelberg: Springer Nature Switzerland AG; Imprint Springer. (2019) ; (forthcoming). |
[19] | Patriarca S. Numbers and nationhood-writing statistics in nineteenth-century italy. Cambride: Cambride University Press. (1996) . |
[20] | Habermas J. Der europäische Nationalstaat unter dem Druck der Globalisierung. Blätter für deutsche und internationale Politik. (1999) ; 1999: (4): 425-36. |
[21] | Cao L. Data science: A comprehensive overview. ACM Comput Surv. (2017) ; 50: (3): 1-42. |
[22] | Cao L. Data science: Challenges and directions. COMMUNICATIONS OF THE ACM. (2017) ; 80: (8): 59-68. |
[23] | MacFeely S. Big data and official statistics. In: Kruger Strydom S, Strydom M, Editors. Big Data Governance and Perspectives in Knowledge Management. Hershey, PA: IGI Global. (2018) ; 25-54. |
[24] | Lehtonen M. Indicators: Tools for informing, monitoring or controlling? In: Jordan AJ, Turnpenny JR, Editors. the Tools of Policy Formulation- Actors, Capacities, Venues and Effects. Cheltenham: Edward Elgar Publishing. (2015) ; 76-99. |
[25] | Davies W. The limits of neoliberalism- authority, sovereignty and the logic of competition. London: SAGE Publications. (2016) . |
[26] | Peruzzi A. Complexity: Between rhetoric and science. In: Maggino F, Editor. Complexity in Society: From Indicators Construction to Their Synthesis: Springer International Publishing. (2017) ; 3-50. |
[27] | Sangolt L. A century of quantification and “Cold calculation”. Trends in the Pursuit of Efficiency, Growth and Pre-eminence. In: Sangolt L, Editor. Between Elightenment and Disaster- Dimensions of the Political Use of Knowledge. Brussels: P. I. E. Peter Lang. (2010) ; 75-110. |
[28] | Ryan L. Forbes/Leadership [Internet]: Forbes. 2014. [cited 2018]. Available from: https://www.forbes.com/sites/lizryan/2014/02/10/if-you-cant-measure-it-you-cant-manage-it-is-bs/#3c96ed027b8b. |
[29] | De Clerck J-P. i-SCOOP [Internet]2017. Available from: https://www.i-scoop.eu/internet-of-things-guide/internet-of-things/. |
[30] | Villani C. FOR A meaningful artificial intelligence- towards a french and european strategy. Mission Assigned by the Prime Minister Eìdouard Philippe. Paris: AI for Humanity- French Strategy for Artificial Intelligence. (2018) ; 151. |
[31] | Diakopoulos N. Accountability in algorithmic decision-making. COMMUNICATIONS OF THE ACM. (2015) ; 59: (2): 56-62. |
[32] | Benessia A, Funtowicz S, Giampietro M, Guimaraes Pereira A, Ravetz J, Saltelli ASR et al. The rightful place of science: Science on the verge. Tempe, AZ: Consortium for Science, Policy and Outcomes. (2016) . |
[33] | Beck U. Risk society towards a new modernity. London: Sage; (1998) . 260 S.p. |
[34] | Mance H. Britain has had enough of experts, says gove. Financial Times. (2016) . |
[35] | Fukuda-Parr S. Global goals as a policy tool: Intended and unitended consequences. In: Fukuda-Parr S, Ely Yamin A, Editors. the MDGs, Capabilities and Human Rights. New York: Routledge. (2015) ; 14-27. |
[36] | Lohr S. Civility in the Age of Artificial Intelligence. ODBMSorg [Internet]. 2016 Nov. 17, 2015]. Available from: http://www.odbms.org/2016/02/civility-in-the-age-of-artificial-intelligence/. |
[37] | Haklay M. Citizen Science and Policy: A European Perspective. case study series. 2015; 4: [61 p.]. Available from: https://www.wilsoncenter.org/publication/citizen-science-and-policy-european-perspective. |
[38] | ECSA. ECSA Policy Paper #3 Citizen Science as part of EU Policy Delivery- EU Directives2016: [4 p.]. Available from: https://ecsa.citizen-science.net/documents. |
[39] | Soma K, Onwezen MC, Salverda IE, van Dam RI. Roles of citizens in environmental governance in the information age – four theoretical perspectives. Current Opinion in Environmental Sustainability. (2016) ; 2016: (18): 122-130. |
[40] | Diaz-Bone R, Didier E, editors. Conventions and Quantification – Transdisciplinary Perspectives on Statistics and Classifications, (2016) . |
[41] | Eyraud C. Stakeholder involvement in the statistical value chain: Bridging the gap between citizens and official statistics. In: Eurostat, Editor. Power From Statistics: Data, Information and Knowledge – Outlook Report – 2018 ed. Luxembourg: Publication Office of the European Union. (2018) ; 103-6. |
[42] | Davies W. How statistics lost their power – and why we should fear what comes next. The Guardian. (2017) . |
[43] | Arnade C. Why Trump voters are not “complete idiots” 2016 [Available from]: https://medium.com/@Chris_arnade/trump-politics-and-option-pricing-or-why-trump-voters-are-not-idiots-1e364a4ed940#.faldoe9vg. |
[44] | König A, editor. Sustainability Science. New York: Routledge; (2018) . |
[45] | WeObserve. An Ecosystem of Citizen Observatories for Environmental Monitoring: WeObserve; (2018) [Available from]: https://www.weobserve.eu/. |
[46] | Joost G, Unteidig A. Design and social change: The changing environment of a discipline in flux. In: Jonas W, Zerwas S, Anshelm Kv, Editors. Transformation Design Perspectives on a New Design Attitude: Birkhäuser. (2015) . |
[47] | Gericke K, Eisenbart B, Waltersdorfer G. Staging design thinking for sustainability in practice: Guidance and watch-outs. In: König A, editor. Sustainability science. New York: Routledge. (2018) ; 147-66. |
[48] | Hisschemoller M, Cuppen E. Participatory assessment: Tools for empowering, learning and legitimating? In: Jordan AJ, Turnpenny JR, Editors. the Tools of Policy Formulation: Edgar Elgar. (2015) ; 33-51. |
[49] | Lehtonen M, Sébastien L, Bauler T. The multiple roles of sustainability indicators in informational governance: Between intended use and unanticipated influence. Current Opinion in Environmental Sustainability. (2016) ; 2016: (18): 1-9. |
[50] | Stapleford TA. Price indexes, political judgments, and the challenge of democratic control. Ottawa Group – International Working Group on Price Indices- Fourteenth Meeting; May 20th to 22nd 2015; Tokyo, Japan: Statistics Japan. (2015) . |
[51] | HLEG. A multi-dimensional approach to disinformation- Final report of the High Level Expert Group on Fake News and Online Disinformation. Luxembourg: European Commission, Directorate-General for Communication Networks CaT. 12 March (2018) . |
[52] | Wigglesworth R. Can big data revolutionise policymaking by governments? FinancialTimes. 31.01.2018. |
[53] | Heubl B. Night light images paint accurate picture of China GDP. Nikkei Asian Review. March 24, (2018) . |
[54] | Seltzer W. Politics and statistics: Independence, dependence, or interaction? In: UN Dept. of Econ. and Soc. Information and Policy Analysis, Working Paper No.6. New York: UN. (1994) . |