An investigation of worker decision-making optimization for smart manufacturing
Abstract
The transition to smart factories, characterized by computer-integrated facilities and advanced technologies, has heightened the demand for specific and advanced skills in workers. This multidisciplinary project brings together insights from cognitive psychology research and qualitative field research to present a framework that identifies factors at the individual and organizational system levels for success in smart manufacturing. The cognitive psychology research indicated that augmenting human cognition, particularly memory, significantly improved performance in training scenarios. The field research resulted in a multi-factor framework to address individual and organizational decision-making factors. A proposal is presented to apply the research findings to designing a training paradigm for smart manufacturing workers and facilities to be adaptable for a variety of situations.
1.Introduction
According to the U.S. Bureau of Labor Statistics [37], in 2022, manufacturing productivity increased even as US manufacturers decried a workforce “skills gap” and challenges in trying to find the “right talent” [25]. If these circumstances remain unchanged, an anticipated 2.1 million manufacturing jobs “could go unfilled by 2030” [25]. These factors must be considered in the context of the ongoing development of smart factories consisting of computer-integrated facilities, machines, and processes: Advanced Technology Services [1] has named 2022 “the year of smart factories.” In contrast to early industrial revolution requirements for low-skilled human labor, the “right talent” shortage highlights how the skill demand for workers is more specific and advanced for smart factories [20]. However, technical skills are only part of the requisite knowledge and skill base needed for worker success in smart manufacturing. Additional skills such as decision-making, critical thinking, and interpersonal skills have been cited as important for worker success [31]. And, a significant recognition that we are moving from Industry 4.0 to Industry 5.0, where the smart factory is more human-centric even while continuing to advance in IoT and AI, calls for an increased understanding of the necessary training for workers in manufacturing as one that focuses on continual resilience and adaptability. We hypothesize that worker success will increase with the development of cognitive skills based on familiarity with integrating complexity. This approach assumes a willingness to work with complex temporal and spatial environments in their entirety, rather than the more traditional approaches emphasizing simplification, factorization, and separation. The project described here is a multi-disciplinary project that focuses on essential decision-making skills for workers in smart manufacturing.
Decision-making is ubiquitous in everyday life and in the research literature, spanning disciplinary areas that include cognitive psychology, group dynamics, organizational communication, management sciences, political science, and more. In a smart work environment, decision-making includes the interaction of humans, machines, and software. Examples of this can be seen in areas such as AI medical diagnosis, AI hiring decisions in human resources, and AI decision-making control during air flights [28]. While AI has enhanced organizational processes, it has also disrupted them through flawed decision-making. A recent report on AI in manufacturing emphasizes the need to re-skill workers in the continuous development of domain expertise, e.g., knowing the industry from the local factory floor to the more global industry operations such as logistics, innovation potential, vertical and horizontal integration [24]. Domain knowledge enables the worker to work with AI, not just to use it as a tool. Rather than reject AI decision-making (an untenable and unrealistic stance) or simply call for improved AI decision-making (a partial solution), this project focuses on how to better prepare humans for smart work in the manufacturing industry. Effective decision-making in the smart workplace calls for humans to not only be self-aware of their own decision-making but also to be aware of any nonhuman decision-making that is taking place. The decision-making skills of workers must include technical knowledge and interactional knowledge to be attuned to cues from humans and nonhumans.
In the following sections, the background for the project is provided, and research results will be presented. The research is reported based on units of analysis, starting with the individual in describing research findings related to how to augment cognition. Those research results are followed by a description of findings from field research and interviews that address smart work as embedded in multiple contexts. After our report, a proposal is outlined that describes how to manage disruptions caused by AI by changing the work training paradigm. This project focuses on smart manufacturing, but the findings and proposal could be applied to other industries that utilize AI and smart technologies.
2.Augmenting human cognition to improve training methods
In order to be prepared for smart work environments, individuals must be cognitively equipped to navigate such environments successfully. Current models of human–machine interface (HMI) systems emphasize the necessity of considering human cognition in the design of efficient HMI systems (e.g., [30]). Human cognition is inherent in any HMI design; however, efficient HMI systems should consider the limitations of human cognition and augment human cognition appropriately. Human cognition is limited in many ways, but two of the most well-documented flawed human cognitive abilities (which can have profound consequences) are human memory and perception. Human memory has been extensively documented to be error-prone, lacking in detail, and incredibly malleable (e.g., [2,23]). Decades of research by Elizabeth Loftus and colleagues have demonstrated that false memories can be easily “implanted”, and although they are false, the individual will confidently believe that the memory is real, vivid, and detailed. Loftus and colleagues have created false memories in their research participants for benign events, such as becoming sick from eating strawberry ice cream during childhood, and for emotionally dramatic events, such as being lost in a shopping mall as a child.
Human perception is another cognitive ability that is quite flawed. Perceiving external stimuli in real-world environments is challenging because the physical signal is complex and often transient, and the human perceptual system has capacity limits and is thus error-prone. Some of the most compelling demonstrations of the limitations of the human perceptual system have been provided by demonstrations of change blindness and change deafness. Change blindness occurs when human observers miss above-threshold, and often obvious, changes occurring in the environment. For example, Simons and Levin [32] demonstrated that people fail to notice that the person they were giving directions to changed to a completely different person when their interaction was interrupted by two individuals carrying a door. The phenomenon occurs in other sensory modalities. For example, demonstrations of change deafness have shown that listeners miss large changes in acoustic stimuli, such as the sound of a dog barking and changing to a piano tune [12].
Given that the workplace will continue to embed technology at all levels, one promising approach to preparing workers for smart environments is to augment the limitations of human cognition. This approach has received some attention in the scientific literature on improving education. For example, research has demonstrated instruction is more effective if the content is organized in ways that are helpful to the learner [26], when the content is logically sequenced [9], and when superfluous distractions have been minimized, such as the amount of space between graphics and key concepts [22]. In the context of workplace training, however, attempts to enhance training through augmentation has predominantly centered on the creation of external, supportive AI systems, rather than addressing internal cognitive functions. Thus, one purpose of this project was to examine which cognitive abilities should be augmented to maximize the performance and efficiency of workers in smart work environments. This research has the potential to guide initiatives aimed at equipping workers for successful integration into smart environments and for successful interaction with artificial intelligence.
Based on the limitations of human memory and perception described above, the purpose of the following experiment was to examine whether human performance benefits more from augmenting memory, augmenting perception, or augmenting both cognitive abilities.
2.1.Participants
Forty-one undergraduate students with normal or corrected-to-normal vision completed the experiment. Participants were compensated with course credit for their time.
2.2.Stimuli
A set of 15 videos were downloaded from YouTube and edited using Microsoft Movie Maker. Each video was approximately 5 minutes in duration, and each video demonstrated how to make and use a novel device (example stimuli and assessments can be found at this link). Quizzes were created for each video. The quizzes were 10-item open-ended questions regarding the processes that occurred in the videos. There were 4 separate conditions. In all conditions, the audio was removed from the video. No additional editing was performed in the No Augmentation condition. In the Augmented Perception condition, descriptive text boxes were added to explain each step, and red arrows and circles were added to highlight the relevant components of the video. In the Augmented Memory condition, descriptive text boxes were added to explain each step of the video and study guides with screenshots, and the text boxes were provided to participants between the video and the quiz. In the Augmented Both condition, descriptive text boxes were added to explain each step, red arrows and circles were added to highlight the relevant components of the video, and study guides with screenshots and text boxes were provided to participants between the video and the quiz.
2.3.Procedure
Participants were assigned to one of the four conditions (No Augmentation, Augmented Perception, Augmented Memory, or Augmented Both) using a Latin square to counterbalance. In all conditions, participants watched a video demonstrating how to make and use a novel device. After each video, there was a two-minute pause. In the None and Augmented Perception conditions, participants were instructed to sit quietly during the two-minute period. In the Augmented Memory and Augmented Both conditions, participants were provided with a study guide to review. After the two-minute pause, all participants completed a 10-question assessment on the creation and functionality of the novel mechanism in the video. This was repeated six times, and each participant watched six randomly selected videos (from the stimulus set of 15 videos) and completed the corresponding assessment.
2.4.Results and discussion
Performance on the assessments was determined by calculating the number of correct responses (out of 10 questions). As shown in Fig. 1, augmenting human cognition resulted in more accurate performance on the assessments than no augmentation. Accuracy was the highest in the Augmented Memory condition (
Fig. 1.
Mean proportion of correct responses on the assessment as a function of condition.
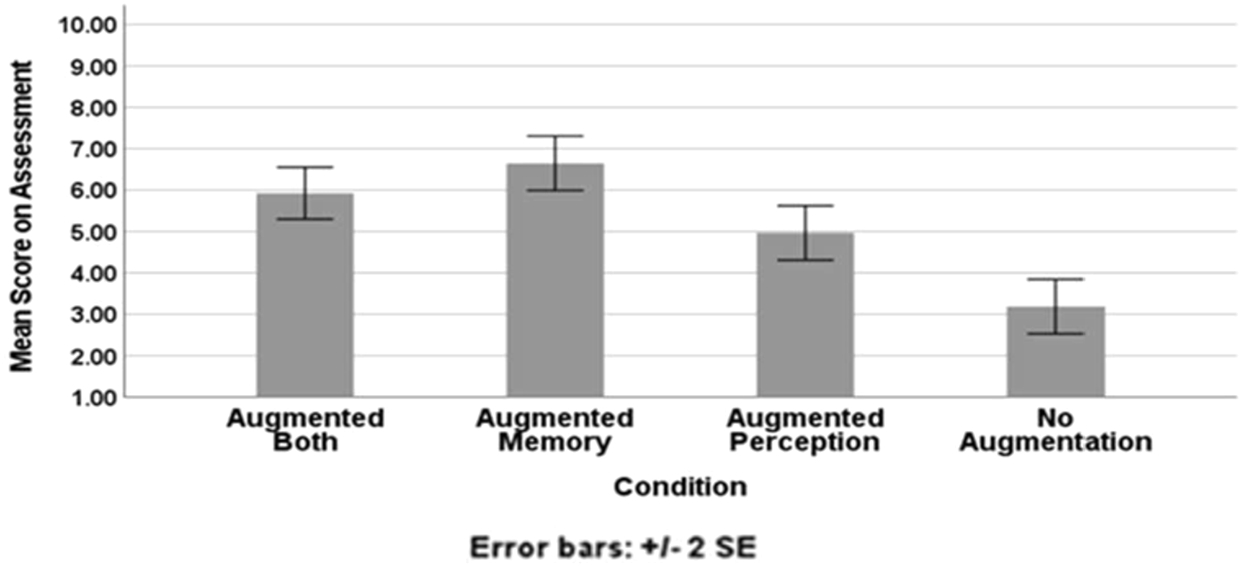
One of the major issues in the current economic landscape is the challenge of finding qualified workers for jobs. Manufacturing faces particular challenges in that the nature of manufacturing has been shifting with the increasing adoption of ‘smart’ manufacturing. As such, the skill demand in workers is more specific and advanced [20]. A promising, converging approach to assist workers with new skill acquisition is to enhance the underlying supportive cognitive processes that assist with the development and execution of new skills. The results of this experiment demonstrate that augmenting human cognition substantially improves the retention of information regarding how to create and use a novel device. Specifically, augmenting human memory resulted in the most accurate performance on the assessments, which indicates that augmenting human memory during smart-factory training sessions has the potential to maximize the acquisition of complex and novel information. Gajdzik and Wolniak [10] argue it is imperative that modern education systems prevent the ‘obsolescence of competence’ as the workforce prepares for smart manufacturing, and many of the required skills depend upon an ability to work with complex and novel information.
Manufacturing environments have relied upon outdated training materials and methodologies that lack effectiveness and fail to integrate insights from psychological research. This has often led to suboptimal employee performance and retention rates. The results of this experiment have the potential to inform the development of improved, research-driven training materials that will improve employee performance and retention. The potential benefits of this research include training methods aligned with cognitive principles, a significant improvement in the effectiveness of employee learning and skill acquisition, a deeper understanding of how augmenting cognitive processes can lead to the creation of more engaging and personalized training experiences, and enhanced employee satisfaction and competency levels, which will in turn positively impact overall productivity and efficiency within manufacturing environments. One potential challenge in adopting new technologies or processes could be that implementing research-driven training methodologies may require initial investments in resources and training infrastructure. Nevertheless, empowering manufacturing environments with advanced, research-driven training solutions, has the potential to create a workforce that is highly skilled, motivated, and engaged, ultimately driving greater success and competitiveness in the industry.
3.Identifying contributing factors for smart decision-making preparedness through field research
The purpose of conducting field research was to gather information on current in situ circumstances related to how manufacturing workers are trained and their abilities (or challenges) in adapting to a smart manufacturing environment.
3.1.Participants and procedures
A cross-country comparative case study approach was taken in gathering data from the US and Germany to identify factors that may be in common or different across these contexts to inform better the analysis of differences that may impact worker training and adaptation. In addition to visiting smart manufacturing facilities, visits were conducted to training sites (i.e., a technical college, and an applied sciences university). Visits typically consisted of tours and extended interviews with supervisors, managers, and training personnel. Six sites were visited, and approximately 24 interviews were conducted starting from Spring through the Fall of 2021. Drawing broadly from a grounded theory approach [11], the qualitative data were analyzed using an inductive and iterative approach to identify characteristics related to smart manufacturing worker decision-making. Similar to Bokrantz et al. [3], the researchers used this approach to aid in conceptualization development to derive categories and themes as they emerged from the data through multiple readings [35]. At regular intervals, the research team met to discuss findings from the field research and interview, created labels to describe decision-making characteristics, and from there, summarized the findings through a matrix. This analysis resulted in the team revising their description of worker decision-making to include context and systemic factors. Given the relatively small number of interviews and sites that the analysis is based on, our results are intended to be presented as exploratory.
3.2.Results and discussion
Initially, the project focused on human workers and their decision-making skills. However, after conducting site visits and interviews, the research team revised the decision-making framework to take into consideration the meaning of the work context as well as the structures and systems set in place in a factory that affect worker decision-making opportunities and expectations. The result of this analysis was the development of a multi-factor matrix that addresses worker characteristics as well as organizational characteristics. In other words, the characteristics of decision-making broadened out in terms of the unit of focus (i.e., individuals and organizational systems), and in terms of temporality (i.e., preceding, synchronous, and anticipated events).
Table 1 identifies factors related to individual worker decision-making. The research team identified that before even entering into a smart factory, workers had preconceptions about what ‘factory work’ involved (i.e., anticipatory socialization). Workers who assumed a ‘Third Industrial revolution’ model of factory work of low-skilled workers tended to have more difficulties in adapting to the cognitive and skill demands of the smart factory. In the field research, the team identified a variety of training approaches that ranged from a technical college or school training prior to employment to apprenticeship-type programs where technical education and working occurred together to on-site training. While supervisors recognized the importance of training, they also highlighted the importance of what they described as ‘soft skills’ and ‘attitude.’ The area of decision-making expectations identified how there are various realms in which workers would make decisions. One challenge noted when explicitly asking about areas where workers made decisions is that the idea of ‘decision’ and ‘decision-making’ tended to be associated with positions at the strategic, management, or supervisory levels. However, researchers have made the case for the importance of worker decision-making on the factory floor [8,13].
Table 1
Essential smart manufacturing decision-making factors for workers
Factor | Description | Practical application |
Anticipatory socialization | Establishing vocational expectations | Inform potential employees of details and expectations of smart manufacturing, possibly through high school or technical school preparation |
Training | How workers are trained for their specific job position | Develop training paradigms that augment memory to maximize worker retention of information |
Dispositions | Worker attitudes including adaptability and flexibility, openness to continuous learning | Identify and support workers who demonstrate positive dispositions; develop a work culture that supports curiosity throughout the organizational hierarchy |
Decision-making expectations | Types of decisions and decision-making processes that workers participate in | Identify formal and informal decision and decision-making areas for workers |
Horizontal collaboration | Worker inclusion in problem-solving and decision making | Expand task-time expectations to allow for worker collaboration; develop physical spaces to allow for worker-to-worker discussions |
The facilitation of factory floor decision making represents more than a broadening of decision-making to include workers in problem solving. Such a logistical move is founded on assumptions that the production process may be improved by decisions that are made during the production cycle by those directly involved with production. As well, having factory floor participation added to the traditional C-suite structure would provide an opportunity for balancing between global and local optima–local optimization seeks to solve an isolated problem, while global optimization addresses the condition of the system as a whole. In the production process, finding a local optima may have implications for the supply chain system as a whole, and it is useful to ensure that local optima do not disrupt the global logistics of the system. A Pareto optimal condition exists, where one cannot improve the local without causing harm to the global process. Dynamic decision-making models allow for embedded decision making that recognizes conditional dependencies and allows variables, conditions and decisions to change with the environment as well as with facility internal conditions. Thus, worker decision making can improve the production process up to the point where it will not have deleterious effects on the entire logistical system.
One step in this process is to identify formal and informal decision-making opportunities. Horizontal collaboration is an extension of decision-making expectations but rather than focusing on the decision, highlights the social and power dynamics of decision-making. Horizontal collaboration specifically highlights the degree to which worker-to-worker interactions are embedded as a part of decision-making. The research team noted that there was increased horizontal collaboration when workers were directly involved in problem-solving and when physical and temporal arrangements supported group collaboration. In addition to the above factors, the team identified key challenges or outcomes. These included hiring gaps and retention of employees – topics that relate to worker decision-making outside of the immediate work context in deciding whether to seek employment in smart manufacturing and whether to stay in a smart manufacturing position or site once employed.
Table 2
Essential smart manufacturing decision-making factors for organizational system
Factors | Description |
Supply chain analysis and feedback | Analysis of supply chain data to anticipate issues |
Consumer analysis and feedback | Analysis of consumer demands to anticipate production needs |
Outsourcing of production materials/resources | Relationship to materials needed for production |
Facility information integration | Availability of systemic production analysis to all employees |
Smart technology integration | The degree or extent to which smart manufacturing or computer-integrated technology is utilized throughout a facility |
Use of predictive analytics | Use of data to anticipate future production demands |
The above factors revolve around the individual. The research team also identified factors to characterize the decision-making of a factory site as an organizational system (see Table 2). The first three factors relate to organizational inputs or ways in which the organization, as a system, utilizes technology to scan the environment. These three factors involve monitoring the supply chain, monitoring consumer feedback, and monitoring resources. In relating these factors to smart decision-making, in each of the areas, the team noted how advanced technologies such as data analytics were relevant for gathering this input. However, observed organizations were inconsistent in their gathering and use of this information. The next two factors, facility information integration and smart technology integration relate to the use of technology within the factory ecosystem. In facility information integration, the team identified how there were varying ways in which information was made available to employees working within the factory. For example, it was common for factory floors to have large monitors with information about factory production levels for any worker to see. However, there were also low-tech means of making information available such as displays with whiteboards and poster boards. The factor of smart technology integration refers to the extent to which computer integration is infused throughout the factory. The team visited sites where the factory was close to being a ‘lights out’ facility with few humans and many robots to sites where there were some areas of production that were computer-integrated whereas other areas of the factory floor resembled third rather than fourth industrial revolution technologies. Finally, the team observed differences in the use of predictive analytics to anticipate future demands, and again, there were variations in the extent to which companies utilized predictive analytics.
Qualitative research has been utilized for investigating smart manufacturing previously. In some instances, these projects focus on the methodology and advocacy of particular research approaches [15,34]. Marrucci et al.’s [21] work is similar to this project in utilizing a comparative, qualitative analysis that collected data from a range of smart manufacturing companies. Their work identified a variety of factors that impacted technological adoption such as technological infrastructure and competence, integration capabilities, organizational agility, environmental dynamism, and so forth. While the aforementioned project utilized qualitative data, this was gathered through a survey methodology. Utilizing in situ interviewing enabled the researchers of this project to develop categories that included human interaction and other social-contextual factors in the analysis such as anticipatory socialization, worker dispositions, and consumer analysis. In this respect, this project provides an empirical bridge from Industry 4.0 to Industry 5.0 [see [18,39]].
4.General discussion
4.1.Summary of results
Current manufacturing environments struggle to find adequately skilled personnel to fill various job roles. This challenge will become more pronounced as manufacturing continues to integrate’smart’ manufacturing technologies. The experiment described earlier directly addressed this challenge by providing an empirically-based and promising approach to enhancing the fundamental cognitive processes that facilitate the acquisition and mastery of new skills. The results of this experiment demonstrate that enhancing memory results in the most improvement during training on how to build and use a novel device. However, augmenting perception and both cognitive processes (i.e., memory and perception) improved information retention and understanding during training. The present results indicate that strategies to enhance human cognition during training sessions in smart factories hold significant potential for optimizing the acquisition of knowledge, which will improve worker performance.
As the economic landscape grapples with the demand for specialized expertise, particularly in sectors undergoing radical transformations, like manufacturing, the strategy of enhancing underlying cognitive processes is an essential component of worker training. This approach not only aids in information retention but also has the potential to improve worker performance, thereby contributing to a more adept and skilled workforce.
In considering ‘smart decision-making’ in advanced manufacturing, this concept can apply to individual humans and to the organizational ecosystem in that each has opportunities to utilize computer-integrated technologies to aid in decision-making. The field research analysis identified how decision-making situations rely on preceding inputs (i.e., anticipatory socialization; dispositions; training for individuals; analysis of consumers, supplies, and production inputs for factories), and internal processes (i.e., decision-making expectations and horizontal integration for individuals; facility information integration and smart technology integration for factories). Our results demonstrate that attention must be given to the alignment of organizational structure and worker preparation. A smart production process requires smart workers who have the ability to communicate effectively with workplace technology. Further, decision making in manufacturing can utilize parallel production processes, separating the sequential components of production and allowing them to occur simultaneously to improve efficiency and reduce downtime.
4.2.The future of the workforce and the workplace
Citrix, a cloud computing and virtual technology company, has created a matrix that aligns worker futures with the production process [7]. The matrix describes four alternative scenarios based on two axes: (1) whether workers will be augmented with technological supports, or replaced; and (2) whether the work, itself, will be centralized in large organizations, or spread across platforms connecting smaller, more agile businesses. The scenarios below in Table 3 are combinations of these two major axes.
Table 3
Scenarios of workers as based on augmentation and organizational centralization
Distributed organizations | Centralized organizations | |
Augmented workers | Freelance frontiers | Powered productives |
Replaced workers | Platform plugins | Automation corporations |
We believe this to be an important breakthrough in smart factory analysis as it does not consider either the workforce or the factory owners in isolation from each other. Findings from the study indicate that businesses prefer the powered productive scenario in which corporations continue to grow using technology to continuously improve the production process with potentially augmented employees. Alternatively, employees see the future more as that represented by platform plugins. This latter scenario presents a contingent workforce that is flexible, adaptable and not committed in the long term to a specific employer. Even though this platform model provides more opportunities for small businesses to find niche areas due to technology, this remains a pessimistic model for most employees, since it allows for worker replacement and/or a decline in long-term employer-employee relationships. A powered productive scenario is a more positive interpretation of successful human machine integration, where production processes are enhanced for all workers through continuous introduction of technology and an increasingly adaptive workforce.
A lack of a shared vision may impact the approach to workforce training and development and the very organizational framework of a factory floor. It is incumbent upon employers and business leaders to act in ways that minimize the ‘dystopian’ threat perceived by workers when anticipating the introduction of technology to the workplace. The digital disconnect identified in Work 2035 can be ameliorated by increasing opportunities for transparent employer-employee interactions around technology implementation. Trenerry et al. [36] recognize that research on employee-related factors during digital transformation in the workplace is only now beginning to emerge. They identify factors at the individual, group, and organizational levels that may assist in the stability of a digital transformation.
The different manifestations of workforce attributes and organizational approaches to the smart factory process that were reported through field visits may be a result of the different ‘mixes’ of the sites. U.S. manufacturers consistently identified worker recruitment and retention as ongoing challenges. During the site visits, it was apparent that digital transformations were heavily focused on worker adaptation to the new technology, and this adaptation was managed through training on the new technology, emphasizing operating skills rather than adaptability, attitudes, and problem-solving skills. Employers recognized that some workers simply were not able to adapt to the digitization, resulting in termination or a lack of promotability within the manufacturer. Both instances can be interpreted as reflecting a disconnect between the expectations of the worker and those of the employer.
Recent research indicates that by 2027 in the United States, 60 percent of workers will either be independent contractors (IC) or will have worked as independent contractors [5]. As employers react to IC growth, they will increasingly pay for results rather than labor. This means that our traditional employee model 4 may be outdated in just a few years, and employees will have to retool to think of themselves as entrepreneurs, not hourly workers, in line with the platform plugin model presented previously, or in a more extreme scenario, the freelance frontier. It also suggests that even though employers may prefer a model that maintains a centralized factory structure, this option may not be possible. Field visits indicated that each employer had differing definitions of what it meant to be a smart factory, and different approaches to the use of technology in the process. Complexity in decision making is mirrored in the management of complexity in decision making frameworks. Complexity that is managed by a strict and rigid organizational design can suppress the advantages of a complex analysis. While streamlined processes can make short term gains, they disregard the opportunities that big data analytics, applied in production and supply chain logistics, can provide for stable and efficient production. A centralized design that encourages human–machine integration, and that provides for increased technology training of workers may be a successful model for managing complexity.
4.3.Guidelines for training implementation
As stated, worker training must consider the specific industry and factory floor environment in implementing a training program. The perception of smart manufacturing varies by industry; its complexity manifests in different technology utilization and employee requirements. We suggest the following components for a preliminary assessment of manufacturing employees and their working environment: 1. Develop and pilot a user journey identifying the DM process (user experience of a production process) for both workers and C suite organizations; 2. Design and implement experiments assessing cognitive load in various scenarios representing complexity, time compression, and external agents in the environment; 3. Develop an assessment tool to connect DM process with dialogue characteristics in the production process; 4. Develop and implement an application based training in optimization techniques.
User journeys are an important consideration in smart manufacturing since they recognize that manufacturing processes differ within and between industries, and they are produced from the perspective of the workers, themselves. Based on the concept of user experience first introduced by Norman [27], user journey maps include affective factors along with traditional assessments of efficiency and productivity. Because our research focuses on reducing and managing cognitive load in the 5th industry worker, it is important to begin with an assessment of the worker experience, recognizing that this assessment will vary depending on the industry, the factory, and the functional responsibilities of the worker.
Assessment of cognitive load of workers can then be interpreted in the user journey’s context. We recommend doing these assessments on site, as they will be more accurate representations of workers’ involvement in the production and decision-making process.
The third component to training preparation utilizes expert decision-making routines in smart factories and investigates how planned human–human and human–machine dialogic routines can enhance decision-making processes. An integrative approach is emphasized to examine the interplay between individual cognitive abilities and cognitive load with interactional skills, focusing on language-use and systems thinking.
The final component of the training is a training protocol designed to assist the worker to shift from traditional, linear decision-making approaches to an optimization routine that can help in reducing cognitive stress, even while increasing both short- and long-term efficiency in the production process. Table 4 illustrates the differences between traditional and optimized decision making, and directly relates to the other components of language interaction and the reduction of cognitive stress. Optimized decision making is based on a move to embrace complexity rather than taking a reductionist approach to making complex challenges ‘fit’ into our simplified understanding.
Table 4
Transitioning from traditional to optimized decision making
Traditional decision-making | Optimized decision-making | |
Directionality | Linear, assumes consistency in a relationship | Nonlinear, assumes relationships between variables change due to external and internal factors |
Feedback | Post choice feedback provides input to decision maker, but does not offer the ability to change the system providing the impact. | Feedback is endogenous to the choice process (embedded choice); decision maker can use feedback to modify systemic behavior |
Short- and long-term objectives | Decision-making is separated between short- and long-term objectives. Short-term objectives cumulate to affect long-term outcomes. | Short- and long-term objectives are considered simultaneously, either through the involvement of actors with different objectives, or the system input of impacts of short-term on long-term systems. |
Teamwork | Teamwork is direct and immediate. Teamwork is less affected by individuals with different objectives. | Teamwork may be direct and indirect; can come from individuals, machines, or systems with different objectives and timelines. |
Stress | Stress is primarily found in the decision-making stage, rather than implementation. | Stress can be in both the decision-making stage and the implementation stage since they can occur simultaneously. Reduced stress can result from knowledge of long-term systemic impact. And the ability to interact with correct terminology and timing |
Efficiency | Efficiency is hampered by linear processes. Follow through on multiple options is inhibited. | Efficiency is increased, since it allows for input throughout the process, not only at the end point of decision-making. |
4.4.Smart factory timeline
The literature on becoming a smart manufacturer is varied, and often cites pillars or components rather than provide a specific progression model. Osterreider, Budde, and Friedli [29] point to the four-layer concept of the smart factory-control, cloud and intelligence, data, and physical-as representing the complexity of ‘becoming smart’, and create a categorization of the literature that mirrors this complexity. Smart factories, especially those that are small to medium sized, will have to make choices as to the layers upon which they will focus; even within those layers there are multiple options for factory development. Indeed, this lack of a specific path to smart factory production was reflected in the site visits, where a large variance in worker training, data analytics on the factory floor, and the number and type of robotics was observed. The one attribute that most had in common was the lack of attention to predictive analytics, which seemed to be last on the list. Interestingly, one factory floor had the capacity and technology for predictive analytics in real time, but did not offer the line workers the ability to interact with the data and adjust. Future research can combine these differing factory choices with environmental variables that might explain the variance among industries, such as the type of production process, the availability of workers, or the mindset of the employers.
Predictive analytics – the industry 4.0 readiness study commissioned by the IMPULS Foundation of the German Engineering Federation (VDMA) identified a five-step process to identify the readiness of an industry to enter into smart manufacturing [14]. The fifth and final level is centered on skill acquisition and development of employees. Others have indicated that big data analytics requires a human machine interaction that allows for human interpretation and decision-making of the predictive and prescriptive information provided by data analysis. It is possible that predictive analytics is impossible to implement without some sort of employee readiness, thus explaining why it is the final implementation.
5.Future directions
The results of this multi-disciplinary project indicate that decision making in a smart factory, or in any organization where there is human machine interaction, needs to move from a traditional linear and simplification approach to one that embraces complexity and seeks to optimize solutions both temporally and spatially. The experimental results pointed to augmented memory as being the most fruitful approach to preparing workers. Augmented memory is a method that provides various contextual references to assist in skill acquisitions. Future research would benefit from optimization, using multiple external contexts to effectively augment memory.
Another promising direction for future research is an assessment of cognitive load. Cognitive workload is the level of mental activity a person exerts while doing a specific task, and it can be measured with EEG (electroencephalogram). The EEG data have the potential to show at what stage and in what condition participants exert more mental effort, which will inform how to better prepare workers to succeed in manufacturing environments. There will also be a need for empirical investigation of convergent approaches to assist workers with new skill acquisition. For example, enhancing the underlying supportive perceptual and motor processes may facilitate the development and execution of new skills. Several perceptual and motor processes play a role in the acquisition of novel and complex skills. For instance, specific patterns of eye movements have consistently been linked to successful problem-solving, and guiding eye movements so that they mimic these patterns has been found to double the success rate of solving a problem (see [33] for a review). Language appears to be particularly essential to successful performance on novel tasks. For example, language reduces the time it takes to find a visual target in a complex array of distractor items [6]. Language can enhance perception by bringing sub-threshold visual stimuli into conscious awareness [19], and verbal labels have been found to facilitate learning new associations between actions and effects [17]. Conversely, inhibiting internal dialogue via an articulatory suppression task, such as requiring participants to say “tick, tick, tick…” in response to a metronome, substantially reduces the ability to form new associations between stimuli [17]. The advantage that language provides is most apparent early in the learning process and is less influential in affecting performance once a task becomes more practiced [38]. This trend is consistent with current cognitive models that frame the initial stage of learning as language-based, which later transitions into a more process-based cognitive operation [4].
Our approach shows the value of a multi-disciplinary, integrative approach. The design of training materials for workers should be based on empirically-supported methods for enhancing information retention and application. Training materials for workers should be grounded in empirically supported methods to ensure their effectiveness and long-term impact. By relying on empirical data, organizations can identify the most efficient and successful approaches to convey information, promote understanding, and foster the development of essential skills among their workforce. In an ever-evolving work landscape, where adaptability and continuous learning are crucial, relying on empirically supported methods for augmenting human cognition ensures that training materials are not only relevant but also contribute to the overall growth and success of both individual employees and the organization as a whole.
The approach advanced here looks at how to enable workers to equip them for dealing with the mechanization and digitization in smart manufacturing through the development of thinking skills that are more appropriate to a future that is codefined by machines and artificial intelligence. To this end, this project has explored ways to manage cognitive stress, look to optimization and embedded decision-making, and identify organizational frameworks that work with complexity rather than minimizing it. Indeed, our approach seeks to develop smarter thinkers through integrating complexity into our thought processes. Results from implementing training with various industry sites have implications for policies and standards in smart factory processes. Our work directly informs the third building block of the Singapore Economic Development Boards’ Smart Industry Readiness Index (SIRI) Framework. Developed in 2019, the International Centre for Industrial Assessment reports that SIRI has now been used in more than 50 countries with hundreds of manufacturers participating in the SIRI assessment. SIRI identifies three major blocks-process, technology, and organization; results from implementation of our trainings can inform all three of these blocks.
However, the proposed model could be applied to other contexts, including organizational, community, society. People need to think smarter across the board. Smart manufacturing has moved more quickly than smart cities, perhaps due to the difference between private and public investment, and in the resultant different decision model for the private and public sector. Technology can be understood as instrumental and only supportive of an existing political and social order, or it can be considered as generative of entirely new and radically different ways of political and social decision making [16]. The path that is taken may depend upon the willingness of both government leaders and citizens to adopt new ways of decision making. And the success of digital transformation in the political realm will also depend on the ability of citizens to make decisions effectively within a new e-environment.
Digital platforms and technology enhanced organizations also have the potential to transform the community. Organizations can use the learning approaches outlined here to enable community development based on technology-based peer to peer networks, use generative AI to work on urban planning, and combine environmental sustainability with economic prosperity. The quadruple helix concept uniting universities, government, business, and citizens can establish a platform for smart communities, but in order for it to be transformative, participants must be comfortable with complexity in decision-making, and have trust in the processes that are often more common in machines than individuals.
Acknowledgements
This project was sponsored through a National Science Foundation planning grant through the Future of Work at the Human–Technology Frontier program (NSF Award 2026283).
Conflict of interest
The authors have no conflict of interest to report.
References
[1] | Advanced Technology Services, Top 8 manufacturing trends for 2022. n.d. https://www.advancedtech.com/blog/manufacturing-trends/. |
[2] | D.M. Bernstein, C. Laney, E.K. Morris and E.F. Loftus, False beliefs about fattening foods can have healthy consequences, Proceedings of the National Academy of Sciences 102: ((2005) ), 13724–13731. doi:10.1073/pnas.0504869102. |
[3] | J. Bokrantz, A. Skoogh, C. Berlin, T. Wuest and J. Stahre, Smart maintenance: An empirically grounded conceptualization, International Journal of Production Economics 223: ((2020) ), 107534. doi:10.1016/j.ijpe.2019.107534. |
[4] | M. Brass, B. Liefooghe, S. Braem and J. De Houwer, Following new task instructions: Evidence for a dissociation between knowing and doing, Neuroscience and Biobehavioral Reviews 81: ((2017) ), 16–28. doi:10.1016/j.neubiorev.2017.02.012. |
[5] | C. Brooke, The history and future of the gig economy (Infographic), Business2Community, (2019) . Available from: https://www.business2community.com/infographics/the-history-and-future-of-the-gig-economy-infographic-02260584. |
[6] | K.W. Cho, I can see what you are saying: Auditory labels reduce visual search times, Acta Psychologica 170: ((2016) ), 19–31. doi:10.1016/j.actpsy.2016.06.003. |
[7] | Citrix, Work 2035. Fieldwork by Citrix, (2020) . https://www.citrix.com/content/dam/citrix/en_us/documents/analyst-report/work-2035.pdf. |
[8] | M. DiGiovanni, Developing knowledge workers on the factory floor: The case of ABB-Alamo, Development and Learning in Organizations: An International Journal 18: (4) ((2004) ), 10–12. |
[9] | F.E. Fontana, O. Furtado Jr., O. Mazzardo and J.D. Gallagher, Whole and part practice: A meta-analysis, Perceptual and Motor Skills 109: ((2015) ), 517–530. doi:10.2466/pms.109.2.517-530. |
[10] | B. Gajdzik and R. Wolniak, Smart production workers in terms of creativity and innovation: The implication for open innovation, Journal of Open Innovation: Technology, Market, and Complexity 8: (2) ((2022) ). doi:10.3390/joitmc8020068. |
[11] | B. Glaser and A. Strauss, The Discovery of Grounded Theory, Aldine, Hawthorne, NY, (1967) . |
[12] | M.K. Gregg and A.S. Samuel, Change deafness and the organizational properties of sounds, Journal of Experimental Psychology: Human Perception and Performance 34: ((2008) ), 974–991. |
[13] | L. Hannola, A. Richter, S. Richter and A. Stocker, Empowering production workers with digitally facilitated knowledge processes–a conceptual framework, International Journal of Production Research 56: (14) ((2018) ), 4729–4743. doi:10.1080/00207543.2018.1445877. |
[14] | IW Consult and Institute for Industrial Management, Industrie 4.0-readiness study, IMPULS Foundation of the German Engineering Federation (n.d.). https://www.industrie40-readiness.de/?lang=en. |
[15] | J.I. Iyana, M. Alias, M. Zulkipli and F. Muhammad-Sukki, Using illustrations to make decisions on the most appropriate qualitative research methodology: The industry 4.0 scenario, International Journal of Qualitative Methods 19: ((2020) ). doi:10.1177/1609406920907247. |
[16] | P. James, Civic technology: A chutes and ladders analysis of transformative potential, Journal of Smart Cities and Societies 1: (1) ((2022) ), 3–15. doi:10.3233/SCS-210117. |
[17] | J. Kray, R. Eenshuistra, H. Kerstner, M. Weidema and B. Hommel, Language and action control, Psychological Science 17: (9) ((2006) ), 737–741. doi:10.1111/j.1467-9280.2006.01774.x. |
[18] | J. Leng, W. Sha, B. Wang, P. Zheng, C. Zhuang, Q. Liu, T. Wuest, D. Mourtzis and L. Wang, Industry 5.0: Prospect and retrospect, Journal of Manufacturing Systems 65: ((2022) ), 279–295. doi:10.1016/j.jmsy.2022.09.017. |
[19] | G. Lupyan and E.J. Ward, Language can boost otherwise unseen objects into visual awareness, Proceedings of the National Academy of Sciences – PNAS 110: (35) ((2013) ), 14196–14201. doi:10.1073/pnas.1303312110. |
[20] | E.G. Margherita and A.M. Braccini, Managing the fourth industrial revolution: A competence framework for smart factory, in: The Fourth Industrial Revolution: Implementation of Artificial Intelligence for Growing Business Success, Springer International, (2021) , pp. 389–402. doi:10.1007/978-3-030-62796-6_23. |
[21] | A. Marrucci, R. Rialti and M. Balzano, Exploring paths underlying industry 4.0 implementation in manufacturing SMEs: A fuzzy-set qualitative comparative analysis, Management Decision ((2023) ). doi:10.1108/MD-05-2022-0644. |
[22] | R.E. Mayer, Applying the science of learning: Evidence-based principles for the design of multimedia instruction, American Psychologist 63: ((2008) ), 760–769. doi:10.1037/0003-066X.63.8.760. |
[23] | G.A. Mazzoni, E.F. Loftus and I. Kirsch, Changing beliefs about implausible autobiographical events: A little plausibility goes a long way, Journal of Experimental Psychology: Applied 7: ((2001) ), 51–59. |
[24] | D. McCauley, Taking AI to the Next Level in Manufacturing, in MIT Technology Review Insights, (2024) , https://www.technologyreview.com/2024/04/09/1090880/taking-ai-to-the-next-level-in-manufacturing. |
[25] | NAM News Room, 2.1 million manufacturing jobs could go unfilled by 2030, (2021) . https://www.nam.org/2-1-million-manufacturing-jobs-could-go-unfilled-by-2030-13743/?stream=workforce. |
[26] | R.A. Noe and J.A. Colquitt, Planning for training impact: Principles of training effectiveness, in: Creating, Implementing, and Managing Effective Training and Development, (2002) , pp. 53–79. |
[27] | D. Norman, J. Miller and A. Henderson, What you see, some of what’s in the future, and how we go about doing it, in: Proceedings of CHI 1995, (2017) . |
[28] | T. Olavsrud, 7 famous analytics and AI disasters, in CIO, (2022) , https://www.cio.com/article/3586802/5-famous-analytics-and-ai-disasters.html. |
[29] | P. Osterreider, L. Budde and T. Friedli, The smart factory as a key construct of industry 4.0: A systematic literature review, International Journal of Production Economics 221: ((2019) ). doi:10.1016/j.ijpe.2019.08.011. |
[30] | H. Ryu, N. Kim, J. Lee and D. Shin, An affordance-based model of human action selection in a human–machine interaction system with cognitive interpretations, International Journal of Human–Computer Interaction 32: ((2016) ), 402–414. doi:10.1080/10447318.2016.1157678. |
[31] | J. Schwartz, D. Denny, D. Mallon, Y. Van Durme, M. Hauptmann, R. Yan and S. Poynton, Beyond reskilling: Investing in resilience for uncertain futures, in: Deloitte Insights, (2020) , https://www2.deloitte.com/us/en/insights/focus/human-capital-trends/2020/reskilling-the-workforce-to-be-resilient.html. |
[32] | D.J. Simons and D.T. Levin, Failure to detect changes to people during a real-world interaction, Psychonomic Bulletin and Review 5: ((1988) ), 644–649. doi:10.3758/BF03208840. |
[33] | M.J. Spivey and R. Dale, Eye movements both reveal and influence problem solving, in: The Oxford Handbook of Eye Movements, Oxford University, (2011) , online edition. doi:10.1093/oxfordhb/9780199539789.013.0030. |
[34] | T. Stock, M. Obenaus, S. Kunz and H. Kohl, Industry 4.0 as enabler for a sustainable development: A qualitative assessment of its ecological and social potential, Process Safety and Environmental Protection 118: ((2018) ), 254–267. doi:10.1016/j.psep.2018.06.026. |
[35] | D.R. Thomas, A general inductive approach for qualitative data analysis, (2003) . Available from: https://www.academia.edu/download/84611396/Inductive_20Content_20Analysis.pdf, accessed on 25 May 2024. |
[36] | B. Trenerry, S. Chng, Y. Wang, Z.S. Suhaila, S.S. Lim, H.Y. Lu and P.H. Oh, Preparing workplaces for digital transformation: An integrative review and framework of multi-level factors, Frontiers in Psychology 12: ((2021) ). doi:10.3389/fpsyg.2021.620766. |
[37] | U.S. Bureau of Labor Statistics, U.S. manufacturing output, hours worked, and productivity recover from COVID-19. TED: The economics daily, (2022) . https://www.bls.gov/opub/ted/2022/u-s-manufacturing-output-hours-worked-and-productivity-recover-from-covid-19.htm. |
[38] | W. Van’t and C. Jarrold, The role of language in novel task learning, Cognition 194: ((2020) ), 104036–104036. doi:10.1016/j.cognition.2019.104036. |
[39] | X. Xu, Y. Lu, B. Vogel-Heuser and L. Wang, Industry 4.0 and industry 5.0 – inception, conception and perception, Journal of manufacturing systems 61: ((2021) ), 530–535. doi:10.1016/j.jmsy.2021.10.006. |