May Bradykinesia Features Aid in Distinguishing Parkinson’s Disease, Essential Tremor, And Healthy Elderly Individuals?
Abstract
Background:
Bradykinesia is the hallmark feature of Parkinson’s disease (PD); however, it can manifest in other conditions, including essential tremor (ET), and in healthy elderly individuals.
Objective:
Here we assessed whether bradykinesia features aid in distinguishing PD, ET, and healthy elderly individuals.
Methods:
We conducted simultaneous video and kinematic recordings of finger tapping in 44 PD patients, 69 ET patients, and 77 healthy elderly individuals. Videos were evaluated blindly by expert neurologists. Kinematic recordings were blindly analyzed. We calculated the inter-raters agreement and compared data among groups. Density plots assessed the overlapping in the distribution of kinematic data. Regression analyses and receiver operating characteristic curves determined how the kinematics influenced the likelihood of belonging to a clinical score category and diagnostic group.
Results:
The inter-rater agreement was fair (Fleiss K = 0.32). Rater found the highest clinical scores in PD, and higher scores in ET than healthy elderly individuals (p < 0.001). In regard to kinematic analysis, the groups showed variations in movement velocity, with PD presenting the slowest values and ET displaying less velocity than healthy elderly individuals (all ps < 0.001). Additionally, PD patients showed irregular rhythm and sequence effect. However, kinematic data significantly overlapped. Regression analyses showed that kinematic analysis had high specificity in differentiating between PD and healthy elderly individuals. Nonetheless, accuracy decreased when evaluating subjects with intermediate kinematic values, i.e., ET patients.
Conclusion:
Despite a considerable degree of overlap, bradykinesia features vary to some extent in PD, ET, and healthy elderly individuals. Our findings have implications for defining bradykinesia and categorizing patients.
1INTRODUCTION
Bradykinesia (movement slowness) and associated motor features (hypokinesia, i.e., reduced movement amplitude, hesitations/halts and sequence effect) characterize the motor phenotype of Parkinson’s disease (PD) and atypical parkinsonism [1–8]. Nevertheless, bradykinesia can be also observed in various neurological conditions [9] and in elderly healthy subjects [10–12].
Although the clinical examination has been deemed the gold standard for evaluating bradykinesia, it is hindered by limited reliability, coupled with inter and intra-rater variability [5, 9, 13–17]. Interpreting objective bradykinesia data, obtained through kinematic techniques is an especially challenging task, particularly when utilized for diagnostic purposes [5, 18, 19]. In a recent study, we utilized a kinematic motion system to objectively evaluate finger tapping movements in patients suffering from PD and ET, as well as healthy controls (HC) [20]. Despite showing distinct motor traits between PD, ET, and HC at the group level, the examination of individual data revealed a significant overlap in kinematic measures across the three cohorts. Consequently, we faced difficulty in precisely predicting the accurate diagnosis upon considering individual bradykinesia features.
In light with the marked overlap characterizing the features of bradykinesia and also considering that a broad spectrum of neurological conditions may underlie this motor disorder, we recently proposed a dual-axes approach to bradykinesia [7]. Axis I concerns the phenomenology of bradykinesia and its associated features, while axis II concerns the possible bradykinesia etiology [7]. Accordingly, we posited that certain combinations of bradykinesia features could offer hints about specific etiologies. For instance, bradykinesia combined with sequence effect and other features strongly points to parkinsonism. Conversely, isolated bradykinesia (movement slowness alone) is a non-specific finding that may manifest in various neurological conditions [7]. Further studies, however, are needed to better address the relationship between bradykinesia phenomenology (Axis I) and its etiology (Axis II).
In this study, we analyzed the kinematics of three groups commonly encountered in the movement disorder outpatient clinic: patients with PD, ET, and elderly individuals who may exhibit subtle parkinsonian signs. Also, we investigated whether clinical and kinematic evaluations of bradykinesia could aid in classifying individuals without a priori diagnosis. The study’s results were interpreted in the context of our recently published viewpoint on bradykinesia [7].
2Materials and Methods
2.1Participants
One hundred and ninety subjects were enrolled in the study, including 44 PD patients (17 F, mean age±standard deviation - SD: 67.84±8.69 years), 69 ET patients (31 F, mean age±SD: 68.42±11.16 years), and 77 HC (44 F, mean age±SD: 66.1±8.14 years) (Table 1). Participants were consecutively recruited at the outpatient clinic of the Department of Human Neurosciences, Sapienza University of Rome. The diagnosis of PD and ET was based on clinical criteria [2, 21, 22]. PD patient with various phenotypes of the disease were enrolled, including patients with an akinetic-rigid form, as well as with tremor dominant phenotype [23, 24]. Pharmacological therapies possibly acting on the central nervous system were discontinued in patients. In detail in PD, dopaminergic therapy discontinuation was performed at least 24 hours before the experimental evaluation [25–28]. In ET patients, therapy discontinuation was obtained by dose reduction in the week prior to evaluation, with drug withdrawal 24 hours earlier for propranolol and benzodiazepines and 48 hours earlier for primidone and topiramate [20, 29–31]. Demographic data and clinical information were collected (Table 1). Cognitive performance was tested in participants by using the Montreal Cognitive Assessment (MoCA) [32]. The study protocol underwent review and gained approval from the local Institutional Review Board, and all participants provided their written informed consent to undergo the experimental procedures.
Table 1
Participants’ demographic and clinical features
PD (44) | ET (69) | HC (77) | |
Gender | 17 F (38.6%) | 31 F (44.9%) | 44 F (57%) |
Age (y) | 67.84±8.69 | 68.42±11.16 | 66.1±8.14 |
Age at onset (y) | 61.64±9.52 | 55.4±18.1 | – |
Disease duration (y) | 3.62±2.49 | 14.98±14.1 | – |
Familial history | 0 Y (0%) | 35 Y (50.73%) | – |
MoCA score | 26.56±2.51 | 27.42±2.61 | 27.21±2.82 |
FTMTRS | – | 19.41±13.32 | – |
UPDRS part III | 34.85±12.71 | – | – |
PD, Parkinson’s disease; ET, essential tremor; HC, healthy controls; F, females; M, males; Y, yes; MoCA, Montreal cognitive assessment; FTMTRS, Fahn-Tolosa-Marin Tremor Rating Scale; Movement Disorder Society-sponsored revision of the Unified Parkinson’s Disease Rating Scale (MDS-UPDRS). Data are indicated as mean±standard deviation (SD). Percentages are indicated in brackets.
2.2Video recordings and evaluation
Participants were video recorded while repeatedly performing 15 s of opening and closing the index finger and the thumb (finger tapping) as fast as possible and with the widest range of motion [16, 20, 25–28, 33–35]. Subjects performed finger tapping by holding their arm at roughly shoulder height, with the forearm semi-flexed and the wrist in line with the forearm [20, 25–28, 35]. PD patients performed the finger tapping with the most affected hand [20, 25, 27]. In light of previous investigations indicating no significant impact of handedness on motor performance, finger tapping assessments were performed with the dominant hand by both ET and HC [20, 25, 27]. The camera only captured the hand performing finger taps, disregarding facial and other body movements, assuring that the presence of other neurological indications, such as hypomimia or change in body posture, would not influence the assessment [16, 17, 36]. Finger tapping videos were randomized for participants’ diagnosis, and a blinded clinical evaluation was performed offline based on video recordings. In detail, seven neurologists with expertise in movement disorders independently scored the tapping performance from 0 to 4, according to item 3.4 (finger tapping) of the Movement Disorder Society-sponsored revision of the Unified Parkinson’s Disease Rating Scale (MDS-UPDRS) [33, 34]. As per the MDS-UPDRS protocol, raters were requested to clinically assess movement velocity (VelC), hesitations/ halts (RhythmC), and decrementing amplitude (sequence effect or Seq_EffC) while executing the tapping test. A rating of 0 denotes a normal motor performance, whereas a score of 1 indicates slightly impaired movements. Similarly, score 2 represents mild abnormal movements, while score 3 depicts a moderate tapping abnormality. Lastly, score 4 is indicative of a severe movement abnormality [33, 34]. Besides the overall score, we asked evaluators to distinguish, using a binary system (yes or no), if the MDS-UPDRS score assigned to a specific video was due to a) decreased movement speed, and/or b) modified rhythm, and/or c) sequence effect [16].
2.3Kinematic recordings and analysis
We recorded the kinematics of repetitive finger tapping using a 3-D optoelectronic system (SMART motion system, BTS, Milan, Italy), consisting of three infrared cameras that detected the movement in three-dimensional space of reflective markers placed on the body segments to be analyzed. Specifically, three markers were placed on the hand and two additional markers were placed on the distal phalanx of the index finger and thumb of the dominant hand in the ET and HC groups and on the most affected hand in the PD group. The cameras had a sampling rate of 120 Hz [25, 27, 29, 35, 37–40]. Motion analysis was conducted offline using specialized software (SMART Analyzer, BTS Engineering, Italy). Using an automatic algorithm, this software accurately identified several kinematic parameters, as described elsewhere [25, 27, 35, 37]. In line with the clinical evaluation approach, we narrowed our focus for further analysis on kinematic data to movement velocity (VelK), expressed in degrees/s, movement rhythm (RhythmK), which is determined by the coefficient of variation computed by the ratio of standard deviation to mean value of inter-tap intervals. Higher values indicate a less regular repetition of movements. We also considered the amplitude decrement (Seq_EffK), expressed in degrees per number of movement [25, 27, 35, 37]. It should be noted that the researcher responsible for the kinematic recordings and analyses (LA) was also blinded to the participants’ diagnoses.
2.4Statistical analysis
We analyzed gender distribution and familial history differences among PD, ET, and HC participants using the Chi-square test. Additionally, the Kruskal-Wallis test was performed to investigate potential variations in demographic and clinical characteristics between the three groups, and post-hoc comparisons were conducted using Dunn’s test.
Fleiss’ K was employed to calculate the inter-raters’ consensus in the blinded video evaluation, whereby a value above 0.21 indicated a satisfactory level of agreement between raters in accordance with Landis and Koch’s (1977) criteria [41]. Kruskal-Wallis testing was utilized to compare clinical scores obtained from the blinded video evaluation of the three participant groups (mean of the 7 raters scores), and post-hoc comparisons were carried out with Dunn’s test. The frequencies of observance for each motor abnormality— VelC, RhythmC, and Seq_EffC— were compared between the groups through the Chi-square test. In addition, finger tapping kinematics were compared between PD, ET, and HC using one-way ANOVA, with kinematic variables analyzed in separate ANOVAs. Post-hoc analyses were performed using the Bonferroni test.
The possible correlations between clinical data, video scores, and kinematic parameters were assessed using Spearman’s rank correlation coefficient. To evaluate the overlapping distribution of the kinematic data, clinical evaluation scores were analyzed and presented through stratified density plots. Furthermore, ordinal and multinomial logistic regression analyses were conducted to determine the impact of each kinematic variable on the probability of belonging to a clinical score category (0, 1, 2, or 3-4) and a diagnostic group (PD, ET, or HC). To obtain a comprehensive assessment, a combined kinematic score (CKS) was developed by considering all the kinematic variables. The CKS was calculated by taking the linear combination of the coefficients derived from the ordinal logistic model. The resulting score accurately reflected the participant’s overall kinematic performance.
To ensure consistency, we normalized the CKS values to a range of 0 to 1 before proceeding, with a higher score reflecting more severe alterations. ROC curves were utilized for visualization and determination of the predictive power of each kinematic variable and the CKS for a particular diagnostic category. The area under the ROC curve (AUC) was used as a measure of discriminating capability for the model. We chose the optimal cut-off point based on the Youden index.
We set the level of significance at 0.05 and performed data analysis using R (version 4.2.2) and STATISTICA® (TIBCO Software Inc., Palo Alto, California, US).
3Results
3.1Demographic and clinical results
The analysis revealed no clear differences in gender ratio and age between PD, ET, and HC, and MoCA scores were statistically comparable among the three groups of participants (p > 0.05). As expected, the age of disease onset was significantly higher in PD than in ET (p = 0.009), while disease duration showed an opposite trend, being longer in ET than in PD (p < 0.001). Furthermore, while 50.73% of ET cases had a positive family history of tremor, no PD patients reported a similar family history of parkinsonism or other neurological conditions (Table 1).
3.2Video evaluation
Through our blinded finger tapping video evaluation, a fair global agreement was reached amongst raters (Fleiss K = 0.34). In particular, the Fleiss K was 0.3 for the PD group, 0.31 for ET, and 0.27 for HC. Kruskal-Wallis ANOVA indicated that there was a significant variance in the finger tapping scores between the three groups of participants [H(2)=74.28, p < 0.001]. Post-hoc comparisons showed higher scores in PD as opposed to ET and HC (p = 0.012 and < 0.001, respectively), as well as in ET as opposed to HC (p < 0.001) (Fig. 1A). Intriguingly, raters assigned an MDS-UPDRS finger tapping score of≥1 to 149 out of 190 subjects, and details on finger tapping scores for each group can be found in Fig. 1B.
Fig. 1
Blinded video evaluation results. A) Finger tapping blinded video rating scores in healthy controls (HC), patients with Parkinson’s disease (PD), and essential tremor (ET), according to the Movement Disorder Society-sponsored revision of the Unified Parkinson’s Disease Rating Scale (MDS-UPDRS). Horizontal lines denote median values. Asterisks indicate p < 0.05 in the post hoc comparisons. B) Number of subjects (percentage are in brackets) within the HC, ET, and PD groups who obtained specific clinical scores (ranging from 0 to 4) at the blinded video evaluations. C) Percentage of subjects within the HC, ET, and PD groups showing specific movement abnormalities at the blinded video evaluation, including reduced velocity (RV), altered rhythm (AR), and sequence effect (SE) (PD group: RV vs. AR: p = 0.58; AR vs. SE: p = 0.026; RV vs. SE: p = 0.026; ET group: RV vs. AR: p = 0.043; AR vs. SE: p = 0.19; RV vs. SE: p = 0.003; HC group: RV vs. AR: p = 0.23; AR vs. SE: p = 0.43; RV vs. SE: p = 0.42). Note that movement slowness was the most prominent abnormality and it differed between PD and ET, being much lower in HC (RV: PD vs. ET: p = 0.012, PD vs. HC: p = 0.002; ET vs. HC: p < 0.001). Irregular movement rhythm differentiates controls from patients, but not PD from ET (AR: PD vs. ET: p = 0.55, PD vs. HC: p = 0.03; ET vs. HC: p = 0.03). The SE was more prevalent in PD than in ET and HC, but it did not differ between ET and HC (SE: PD vs. ET: p < 0.001, PD vs. HC: p < 0.001; ET vs. HC: p = 0.15).
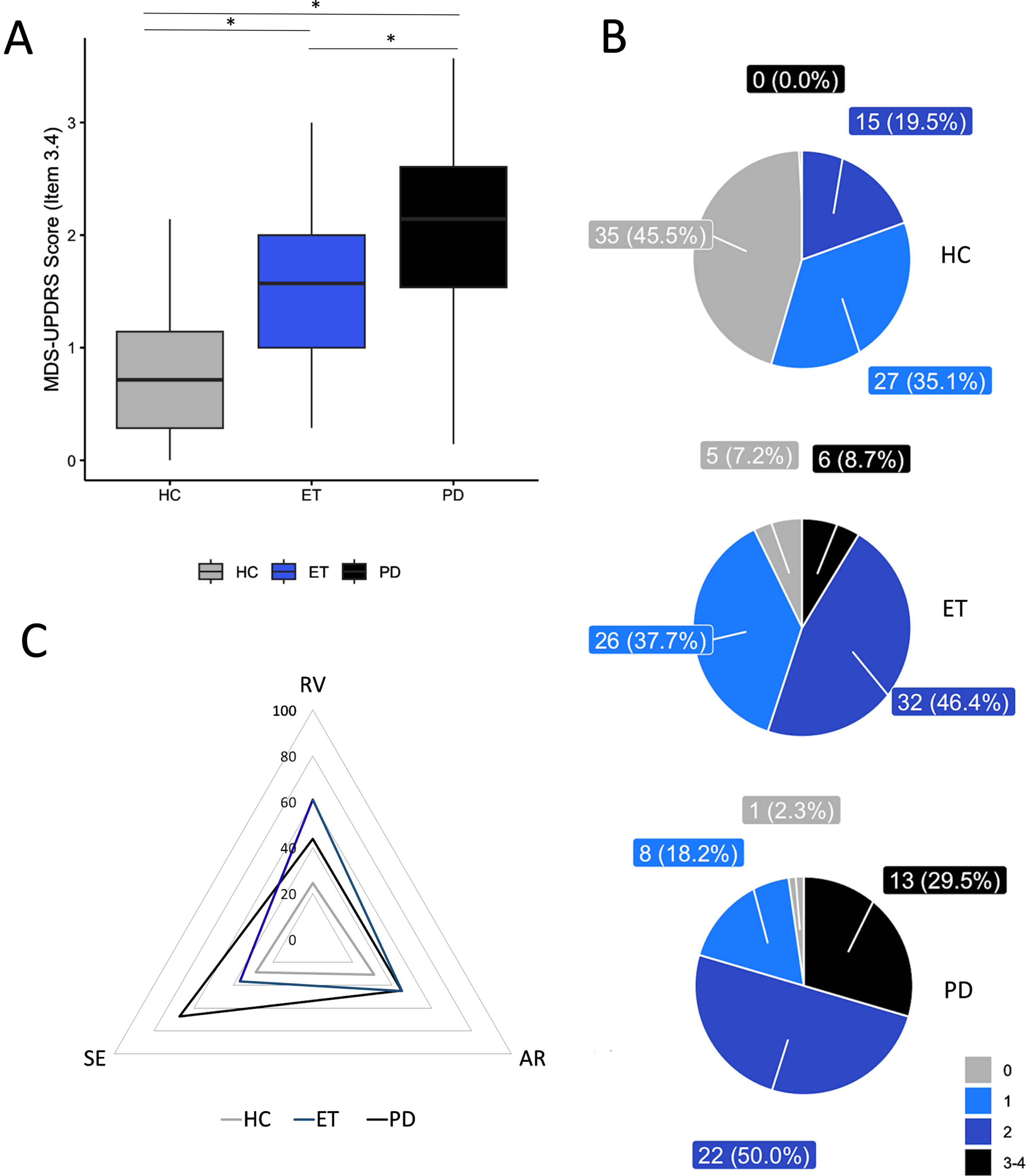
When considering the specific motor abnormalities responsible for the MDS-UPDRS finger tapping score (Fig. 1C), we observed that in PD patients, 43.8% had reduced VelC, 44.8% had altered RhythmC, and 67.2% had Seq_EffC. In ET patients, 60.9% had reduced VelC, 44.9% had altered RhythmC, and only 36.6% showed Seq_EffC (Fig. 1). In HC, we observed equal prevalence of the three motor abnormalities (reduced VelC - 24.48%, altered RhythmC- 30.98%, and Seq_EffC- 28.75%), with no significant difference between any of them. In summary, movement slowness, which was identified in both PD and ET patients by the raters, proved to be the most prominent abnormality in movement. It was also observed that this abnormality differed between PD and ET, in addition to being much lower in the control group (all ps < 0.01, see Fig. 1 Legend for more details). On the other hand, although an irregular movement rhythm could differentiate controls from patients, it was not able to distinguish between PD and ET (p = 0.55). The sequence effect was found to be more prevalent in individuals with PD than in those with ET and HC (both ps < 0.001). However, there was no significant difference observed between the occurrence of the sequence effect in ET and HC (p = 0.15).
3.3Kinematic analysis of finger tapping
The kinematic parameters of finger tapping in the three groups are shown in Table 2. As expected, the ANOVAs disclosed a significant effect of the factor ‘GROUP’ for VelK (F2,187 = 26.61, p < 0.001), RhythmK (F2,187 = 10.04, p < 0.001), and Seq_EffK (F2,187 = 6.91, p = 0.001). VelK was lower in PD as compared to both ET and HC (p = 0.005 and p < 0.001, respectively), as well as in ET as compared to HC (p < 0.001). RhythmK was more irregular in PD than in ET and HC (p = 0.005 and p < 0.001, respectively), with no difference between ET and HC (p = 0.48). Finally, the Seq_EffK was higher in PD than in ET and HC (p < 0.001 and p = 0.03, respectively), with no difference between ET and HC (p = 0.43).
Table 2
Finger tapping kinematics
PD (44) | ET (69) | HC (77) | p* | p** | p*** | |
N MOV | 50 (19.75) | 38.13(18.5) | 50.91 (19.6) | <0.001 | 1 | <0.001 |
CV (RhythmK) | 0.12 (0.03) | 0.1 (0.043) | 0.08 (0.06) | 0.005 | <0.001 | 0.48 |
MOVEMENT AMPLITUDE | 39.21(20.81) | 45.59 (18.34) | 49.24 (12.98) | 0.064 | <0.001 | 0.053 |
MOVEMENT VELOCITY (VelK) | 766.618 (410.57) | 925.634 (308.39) | 1053.36 (155.32) | 0.005 | <0.001 | <0.001 |
AMPLITUDE SLOPE (Seq_EffK) | -0.18 (0.25) | -0.1 (-0.27) | -0.11 (0.16) | <0.001 | 0.003 | 0.43 |
VELOCITY SLOPE | -4.33 (-5.02) | -4.56 (-5.67) | -4.94 (-4.83) | 1 | 1 | 1 |
PD, Parkinson’s disease; ET, essential tremor; HC, healthy controls; N MOV, number of movements; CV, coefficient of variation (RhythmK). Movement amplitude is expressed in degrees; movement velocity (VelK) is expressed in degrees/sec. Amplitude slope is (Seq_EffK) expressed in degrees/n mov. Velocity slope is expressed in (degrees/sec)/n mov. Data are indicated as median (interquartile range). *p values from post-hoc comparisons between PD and ET; **p values from post-hoc comparisons between PD and HC; ***p values from post-hoc comparisons between ET and HC. Significant values are indicated in bold.
In summary, kinematic analysis of finger tapping revealed that subjects with PD exhibit bradykinesia, often accompanied by sequence effect an irregular rhythm, whereas the primary motor abnormality observed in subjects with ET and HC was an isolated bradykinesia.
3.4Correlation analysis
We found a correlation between age and the median clinical score at the blinded video rating in participants (R = 0.23, p = 0.001). The analysis also showed an inverse correlation between video rating global scores and VelK values (r=-0.51, p < 0.001). Conversely, a direct relation was observed between video rating global scores and RhythmK values (r = 0.51, p < 0.001). This means that the patients who obtained the highest scores at the blinded video evaluation also showed the slower and the more irregular movement at the kinematic analysis. Notably, we did not find any correlation between clinical scores and the Seq_EffK (r=-0.06, p = 0.38). Also, no correlations were found between kinematic values and tremor severity in ET (r = 0.12, p = 0.34), nor between other demographic, clinical, and kinematic data.
3.5Stratified density plots
In Fig. 2, the distribution of kinematic parameter values in the participants is presented, based on the median score (ranging from 0 to 4) given by the 7 raters. Supplementary Figures 1, 2, and 3 depict the distribution of kinematic parameter based on the clinical score given by each rater. The density plots reveal a substantial overlap among the kinematic data curves, which suggests that a specific clinical score is associated with a broad range of kinematic values. Figure 2 also illustrates the distribution of kinematic parameters among the three groups of participants (PD, ET, and HC), displaying the lowest VelK values in the PD group (left-hand graph), with substantial overlap observed in both ET and HC groups. Notably, the kinematic data of RhythmK and Seq_EffK demonstrated even greater overlap across all three groups (middle and right-hand graphs).
Fig. 2
Stratified density plots. Upper part: Density plots were used to evaluate the overlapping in the distribution of the kinematic data based on the median score (ranging from 0 to 4) given by the 7 raters. Note the marked overlapping between data curves, indicating that a given clinical score reflected a wide range of kinematic values in participants. Velocity is expressed as degrees/s (VelK). The coefficient of variation (CV), computed by the standard deviation/mean value of the inter-tap intervals, expresses movement rhythm (with higher CV values representing a lower regularity of repetitive movements) (RhythmK). Amplitude slope is expressed in (degrees/s)/n.mov (Seq_EffK). Lower part: distribution of kinematic parameters among the three groups of participants (HC: healthy controls, ET: essential tremor, PD: Parkinson’s disease). Note that the PD group had the lowest VelK values (left-hand graph). However, VelK greatly overlapped in ET and HC. The overlapping of kinematic data between the three groups was even greater for the RhythmK and Seq_EffK (middle and right-hand graph).
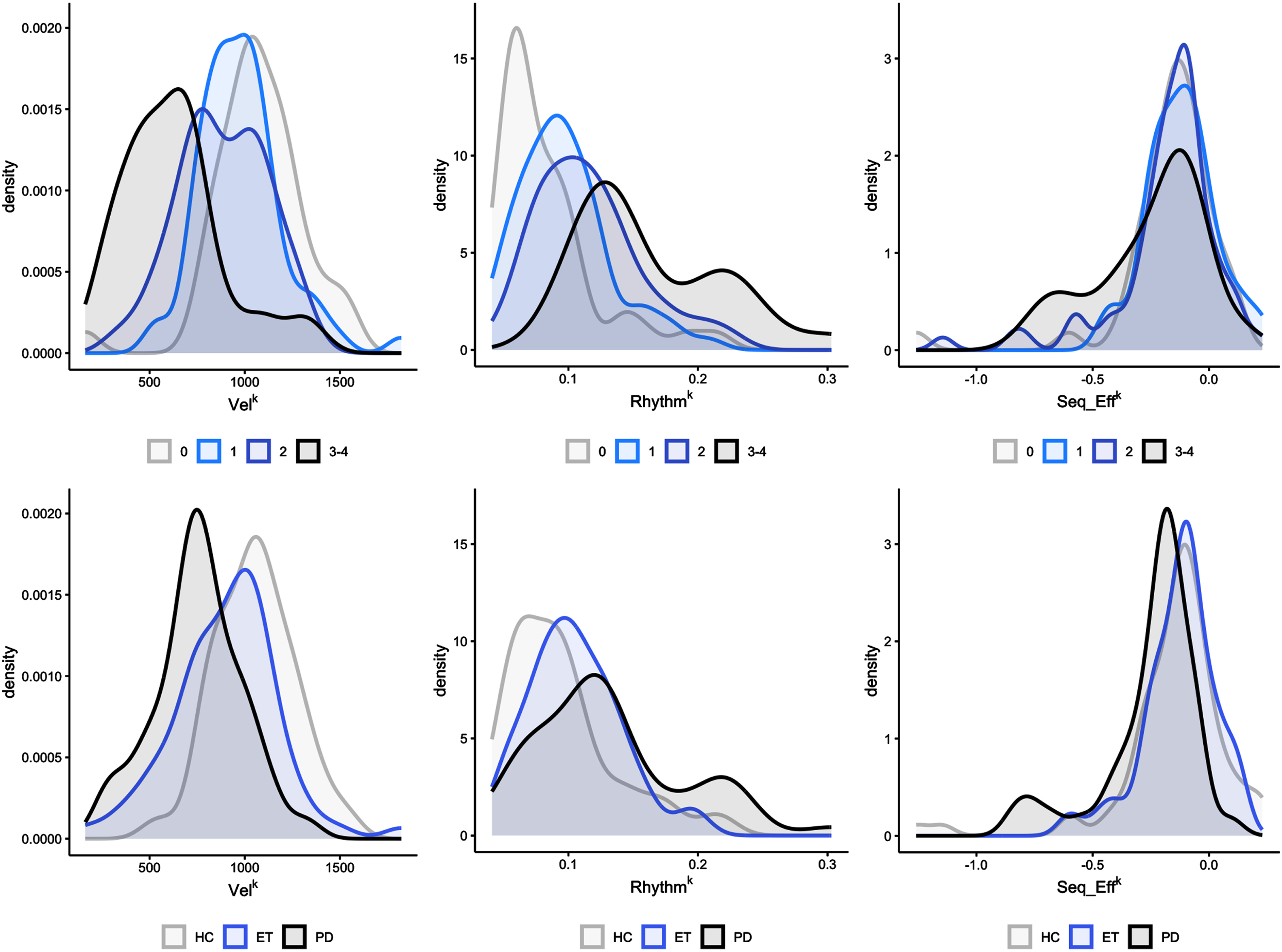
Fig. 3
Ordinal and multinomial logistic regression analysis results. A) The figure depicts the probability of belonging to a specific clinical score category (0, 1, 2, or 3-4) based on individual kinematic parameters, i.e., movement velocity (VelK), coefficient of variation (RhythmK), and amplitude slope (Seq_EffK), and on the combined kinematic score (CKS). Note that subjects with high CKS, i.e., greater than 0.6, had a high probability of being classified as bradykinetic, i.e., with Movement Disorder Society-sponsored revision of the Unified Parkinson’s Disease Rating Scale (MDS-UPDRS) clinical scores higher than 2. The opposite occurred for subjects with low CKS score (<than 0.2), which had a high probability of being classified as normal (MDS-UPDRS clinical scores = 0). Intermediate CKS values, however, correspond to a low probability of being correctly classified as bradykinetic or not bradykinetic. B) The figure depicts the probability of belonging to a specific diagnostic group (HC, healthy controls; ET, essential tremor; PD, Parkinson’s disease) based on individual kinematic parameters, and on the CKS. Note that subjects with CKS values greater than 0.7 had a high probability of belonging to the PD group, and a very low probability of belonging to the HC group. The opposite was observed for subjects with low CKS score values (lower than 0.2), which had a high probability of being normal subjects and a very low probability of belonging to the PD group. At intermediate CKS values corresponded comparable probabilities of belonging to the PD, ET, and HC groups.
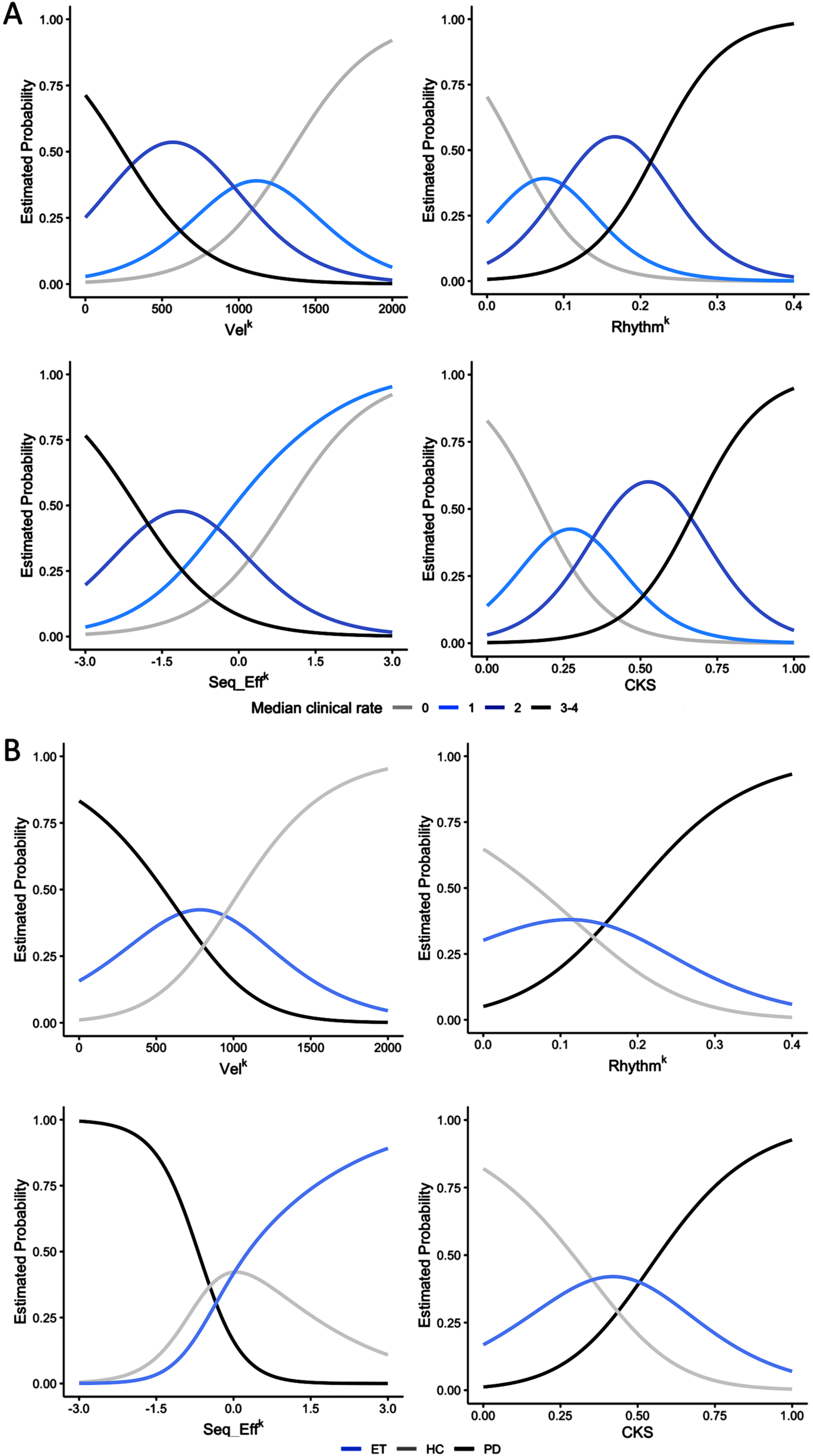
3.6Ordinal and multinomial logistic regression analyses, ROC curves
The results of the ordinal and multinomial logistic regression analyses are presented in Fig. 3, which depicts the probability of being assigned to a specific clinical score category (0, 1, 2, or 3-4, Fig. 3A) or diagnostic group (PD, ET, and HC, Fig. 3B) based on individual kinematic parameters and CKS values. Notably, individuals with high CKS scores (>0.6) were more likely to be classified as clinically bradykinetic, with MDS-UPDRS clinical scores exceeding 2 (Fig. 3A). Conversely, those with low CKS scores (<0.2) had a high probability of being clinically classified as normal, with MDS-UPDRS clinical scores of 0. Intermediate CKS values were associated with a low probability of proper bradykinetic classification (Fig. 3A). Similarly, subjects with CKS values greater than 0.7 had a high probability of being in the PD group and a very low probability of being in the HC group (Fig. 3B). Conversely, individuals with low CKS scores (less than 0.2) had a high probability of being normal and a very low probability of being in the PD group (Fig. 3B). Again, intermediate CKS values were associated with equal probabilities of belonging to the PD, ET, and HC groups, reflecting more limited possibilities for proper diagnostic classification. ROC curves (Fig. 4) demonstrated that CKS cutoffs of 0.7 and 0.2 were highly specific for differentiating PD from HC [sensitivity: 0.136 (0.052, 0.274), specificity: 0.986 (0.951, 0.998), and sensitivity: 0.247 (0.156, 0.358), specificity: 0.938 (0.877, 0.975), respectively]. It is worth noting that the area under the curve (AUC) of the VelK parameter was comparable to that of the CKS, highlighting the crucial role of this kinematic parameter in assessing bradykinesia.
Fig. 4
Receiver operating characteristic (ROC) curves. ROC curves were used to graphically represent and to determine the capacity of the kinematic variables and of the combined kinematic score (CKS) to evaluate the clinical score category and the diagnostic group in participants. The value of area under the ROC curve (AUC) was considered to measure for how well the model can discriminate between subjects.
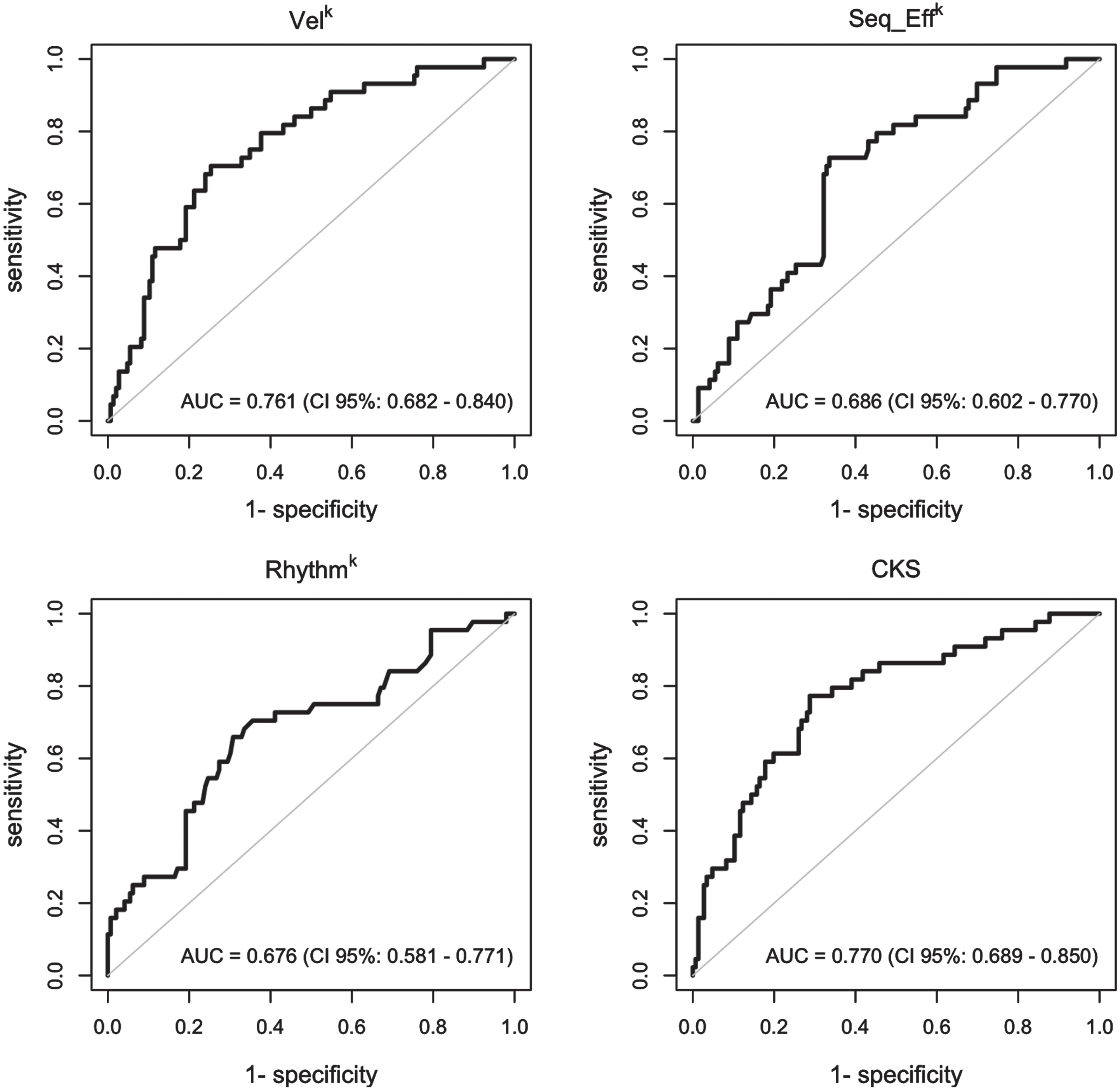
4DISCUSSION
Our study aimed to determine whether a comprehensive clinical and kinematic assessment of repetitive finger tapping could facilitate the detection of bradykinesia and accurately predict diagnoses across a range of PD, ET, and healthy elderly individuals. Our findings support previous evidence indicating that bradykinesia is a prominent feature of PD, but is also present in ET and, to a lesser extent, in otherwise HC [9, 20]. We also noted that the features of bradykinesia differ depending on the group of subjects it occurs in. Specifically, bradykinesia with sequence effect and altered movement rhythm is more common in PD whereas bradykinesia alone is frequently observed in ET and HC. Alongside clinical examination, kinematic analysis is crucial for accurate bradykinesia detection and patient categorization in most cases. However, our study also uncovered a complex relationship between bradykinesia phenomenology and the underlying etiology, and in approximately 30% of cases the diagnosis was not achievable.
Demographic characteristics, such as age and sex distribution, of the PD, ET, and HC groups were similar, dampening the impact of these factors on our results. Cognitive performance was also comparable among the three groups, thus avoiding a potential confounding factor. Even though the diagnosis of PD and ET was determined by clinical criteria and without the use of DaTscan examination, all patients were consistently monitored in our outpatient clinic for several years, significantly mitigating the possibility of misdiagnosis bias [2, 22]. To ensure the accuracy of our assessments, we took additional measures. First, all patients discontinued therapies 48 hours before the experimental evaluation to avoid any potential impact on finger tapping performance. Second, bradykinesia assessments were blinded to participants’ diagnosis. Third, a blinded assessment of both videos and kinematic recordings was conducted to ensure that the evaluation of finger movement remained unbiased. Again, to avoid any external influence on the assessments, the video frames focused only on the subject’s hand [16, 17], thus eliminating the possibility that additional neurological symptoms, such as hypomimia or altered body posture, would influence the assessment. In this study, we specifically tested finger tapping movements which allowed us to rule out the possibility that bradykinesia in ET could be attributed to tremors, as tremors may have a greater impact on proximal arm movements [20]. In this regard, we also observed no correlation between tremor severity and finger tapping velocity in ET patients. Finally, while the level of agreement among evaluators during the blinded examination of video recordings was merely “fair”, confirming previous observations [17], the findings obtained from the kinematic analysis were consistent with the clinical outcome.
To our knowledge, this is the first study assessing bradykinesia through a blinded analysis on simultaneous video and kinematic recordings of finger tapping recordings in a broad sample of PD, ET, and HC. Our findings showed that nearly 80% of the participants were classified as bradykinetic, based on an MDS-UPDR finger tapping score of≥1. However, upon further analysis, it was observed that individuals with PD exhibited more severe bradykinesia scores compared to those with ET and HC, while those with ET demonstrated higher scores than those in the HC group. Though it is not surprising that the PD group exhibited higher bradykinesia scores, our study’s sample consistently displayed a greater proportion of bradykinetic ET cases than those reported in prior research, which ranged from less than 2% to 20% [42–50]. There are various factors that could account for discrepancies in findings between our study and previous research. For example, in our analysis we utilized a MDS-UPDRS score threshold of≥1; additionally, it is worth noting the absence of blinding techniques during bradykinesia evaluation in earlier investigations. It is important to note that only one previous study has specifically evaluated finger tapping movements [48], which are considered the most effective test for detecting bradykinesia in clinical practice [2, 5, 21, 25, 33, 34]. Also, Jim
One additional finding of our study is the significant overlap of kinematic data, indicating that a given clinical score may reflect a diverse range of kinematic values. Thus, kinematic and clinical assessments were not always consistent, so an individual with low clinical scores (i.e., no/minimal bradykinesia) may actually have low amplitude and velocity values or an altered kinematic rhythm, and vice versa. Further analysis revealed a marked overlap of kinematic parameters among the three groups (PD, ET, and HC). To estimate the probability of being assigned to a specific clinical score group and diagnostic group based on individual kinematic parameters, we conducted ordinal and multinomial logistic regression analyses, considering both the individual kinematic parameters as well as the CKS, which aggregates various kinematic movements into a single value. Consistent with expectations, the CKS values of PD patients were found to be the highest among the three groups. We found that kinematics could differentiate between individuals with moderate/severe bradykinesia and those with normal motor function, e.g., eukinesia [7]. Additionally, both movement velocity and the CKS exhibited high specificity in distinguishing PD patients from HC. However, it was unlikely that the present methodology could correctly classify participants with intermediate kinematic values. In summary, by utilizing a combination of kinematic parameters, we were able to accurately predict with high precision whether a subject belonged to the PD or HC group. Nevertheless, approximately 30% of our sample exhibited mild to moderate kinematic abnormalities that precluded us from accurately diagnosing their condition using the methodology presented. As a result, a comprehensive evaluation and characterization of bradykinesia features, whether considered independently or in conjunction, proved inadequate in establishing the underlying etiology in all cases [7]. The present finding indicates that there can be a complex relationship between the phenomenology of bradykinesia and its etiology. In these conditions, it is crucial to evaluate other clinical symptoms and signs to achieve an accurate diagnosis. It is important to note that in our study, we specifically examined abnormalities in voluntary movement. Nonetheless, as discussed in our viewpoint, including an evaluation of spontaneous movement, as well as other symptoms could greatly improve the diagnostic accuracy [7].
It should be noticed that we only enrolled patients with mild PD and ET. Based on previous evidence, we can hypothesize patients in more advanced disease stages may display different bradykinesia features [5, 27, 29]. Studies in PD, for example, have shown that advanced patients do not show the sequence effect, but have a markedly reduction of movement amplitude (marked hypokinesia) [5, 27]. Only one study evaluated bradykinesia in patients with ET using a longitudinal approach, showing that a similar rate of decrease in movement velocity and amplitude (brady-hypokinesia) can be observed in patients during disease progression [29]. Further studies of larger samples of patients at different disease stages and other longitudinal works are needed to support these findings. Finally, future study will assess the possible effect of aging on bradykinesia comparison between PD, ET, and HC.
In conclusion, this paper provides evidence supporting previous observations that bradykinesia can occur in conditions other than PD and demonstrates that its features vary depending on the underlying condition. Overall, the features of bradykinesia can provide valuable information for distinguishing PD from ET and healthy aging, but the interpretation should always consider the context of the individual and the presence of other symptoms or signs. Therefore, a comprehensive clinical assessment that integrates subjective and objective measures is necessary for accurate diagnosis and follow-up.
ACKNOWLEDGMENTS
The authors wish to thank Dr. Laura Centonze (laura.centonze@uniroma1.it) from the Department of Human Neurosciences, Sapienza University of Rome, for her support in the English-language editing of the manuscript.
FUNDING
This work was supported by the Italian Ministry of Health (Current Research 2023).
CONFLICT OF INTEREST
The authors have no conflict of interest to report.
DATA AVAILABILITY
The data supporting the findings of this study are available on request from the corresponding author. The data are not publicly available due to privacy or ethical restrictions.
SUPPLEMENTARY MATERIAL
[1] The supplementary material is available in the electronic version of this article: https://dx.doi.org/10.3233/JPD-230119.
REFERENCES
[1] | Armstrong MJ , Litvan I , Lang AE , Bak TH , Bhatia KP , Borroni B , Boxer AL , Dickson DW , Grossman M , Hallett M , Josephs KA , Kertesz A , Lee SE , Miller BL , Reich SG , Riley DE , Tolosa E , Tröster AI , Vidailhet M , Weiner WJ ((2013) ) Criteria for the diagnosis of corticobasal degeneration. Neurology 80: , 496–503. |
[2] | Postuma RB , Berg D , Stern M , Poewe W , Olanow CW , Oertel W , Obeso J , Marek K , Litvan I , Lang AE , Halliday G , Goetz CG , Gasser T , Dubois B , Chan P , Bloem BR , Adler CH , Deuschl G ((2015) ) MDS clinical diagnostic criteria for Parkinson’s disease. Mov Disord 30: , 1591–1601. |
[3] | Höglinger GU , Respondek G , Stamelou M , Kurz C , Josephs KA , Lang AE , Mollenhauer B , Müller U , Nilsson C , Whitwell JL , Arzberger T , Englund E , Gelpi E , Giese A , Irwin DJ , Meissner WG , Pantelyat A , Rajput A , van Swieten JC , Troakes C , Antonini A , Bhatia KP , Bordelon Y , Compta Y , Corvol J-C , Colosimo C , Dickson DW , Dodel R , Ferguson L , Grossman M , Kassubek J , Krismer F , Levin J , Lorenzl S , Morris HR , Nestor P , Oertel WH , Poewe W , Rabinovici G , Rowe JB , Schellenberg GD , Seppi K , van Eimeren T , Wenning GK , Boxer AL , Golbe LI , Litvan I , for the Movement Disorder Society-endorsed PSP Study Group ((2017) ) Clinical diagnosis of progressive supranuclear palsy: The movement disorder society criteria: MDS Clinical Diagnostic Criteria for PSP. Mov Disord 32: , 853–864. |
[4] | McKeith IG , Boeve BF , Dickson DW , Halliday G , Taylor J-P , Weintraub D , Aarsland D , Galvin J , Attems J , Ballard CG , Bayston A , Beach TG , Blanc F , Bohnen N , Bonanni L , Bras J , Brundin P , Burn D , Chen-Plotkin A , Duda JE , El-Agnaf O , Feldman H , Ferman TJ , Ffytche D , Fujishiro H , Galasko D , Goldman JG , Gomperts SN , Graff-Radford NR , Honig LS , Iranzo A , Kantarci K , Kaufer D , Kukull W , Lee VMY , Leverenz JB , Lewis S , Lippa C , Lunde A , Masellis M , Masliah E , McLean P , Mollenhauer B , Montine TJ , Moreno E , Mori E , Murray M , O’Brien JT , Orimo S , Postuma RB , Ramaswamy S , Ross OA , Salmon DP , Singleton A , Taylor A , Thomas A , Tiraboschi P , Toledo JB , Trojanowski JQ , Tsuang D , Walker Z , Yamada M , Kosaka K ((2017) ) Diagnosis and management of dementia with Lewy bodies: Fourth consensus report of the DLB Consortium. Neurology 89: , 88–100. |
[5] | Bologna M , Paparella G , Fasano A , Hallett M , Berardelli A ((2020) ) Evolving concepts on bradykinesia. Brain 143: , 727–750. |
[6] | Wenning GK , Stankovic I , Vignatelli L , Fanciulli A , Calandra-Buonaura G , Seppi K , Palma J-A , Meissner WG , Krismer F , Berg D , Cortelli P , Freeman R , Halliday G , Höglinger G , Lang A , Ling H , Litvan I , Low P , Miki Y , Panicker J , Pellecchia MT , Quinn N , Sakakibara R , Stamelou M , Tolosa E , Tsuji S , Warner T , Poewe W , Kaufmann H ((2022) ) The Movement Disorder Society Criteria for the Diagnosis of Multiple System Atrophy. Mov Disord 37: , 1131–1148. |
[7] | Bologna M , Espay AJ , Fasano A , Paparella G , Hallett M , Berardelli A ((2023) ) Redefining bradykinesia. Mov Disord 38: , 551–557. |
[8] | Herz DM , Brown P ((2023) ) Moving, fast and slow: Behavioural insights into bradykinesia in Parkinson’s disease. Brain–10.1093/brain/awad069. |
[9] | Paparella G , Fasano A , Hallett M , Berardelli A , Bologna M ((2021) ) Emerging concepts on bradykinesia in non-parkinsonian conditions. Eur J Neurol 28: , 2403–2422. |
[10] | Bennett DA , Beckett LA , Murray AM , Shannon KM , Goetz CG , Pilgrim DM , Evans DA ((1996) ) Prevalence of parkinsonian signs and associated mortality in a community population of older people. N Engl J Med 334: , 71–76. |
[11] | Buchman AS , Wilson RS , Shulman JM , Leurgans SE , Schneider JA , Bennett DA ((2016) ) Parkinsonism in older adults and its association with adverse health outcomes and neuropathology. J Gerontol A Biol Sci Med Sci 71: , 549–556. |
[12] | Buchanan SM , Richards M , Schott JM , Schrag A ((2021) ) Mild parkinsonian signs: A systematic review of clinical, imaging, and pathological associations. Mov Disord 36: , 2481–2493. |
[13] | Hasan H , Athauda DS , Foltynie T , Noyce AJ ((2017) ) Technologies assessing limb bradykinesia in Parkinson’s disease. J Parkinsons Dis 7: , 65–77. |
[14] | Merola A , Sturchio A , Hacker S , Serna S , Vizcarra JA , Marsili L , Fasano A , Espay AJ ((2018) ) Technology-based assessment of motor and nonmotor phenomena in Parkinson disease. Expert Rev Neurother 18: , 825–845. |
[15] | Ricciardi L , De Angelis A , Marsili L , Faiman I , Pradhan P , Pereira EA , Edwards MJ , Morgante F , Bologna M ((2020) ) Hypomimia in Parkinson’s disease: An axial sign responsive to levodopa. Eur J Neurol 27: , 2422–2429. |
[16] | Marsili L , Duque KR , Gregor N , Abdelghany E , Abanto J , Duker AP , Hagen MC , Espay AJ , Bologna M ((2023) ) Bradykinesia in neurodegenerative disorders: A blinded video analysis of pathology-proven cases. Mov Disord 38: , 496–501. |
[17] | Williams S , Wong D , Alty J , Relton SD ((2023) ) Parkinsonian hand or clinician’s eye? Finger tap bradykinesia interrater reliability for 21 movement disorder experts. J Parkinsons Dis 13: , 525–536. |
[18] | Heldman DA , Espay AJ , LeWitt PA , Giuffrida JP ((2014) ) Clinician versus machine: Reliability and responsiveness of motor endpoints in Parkinson’s disease. Parkinsonism Relat Disord 20: , 590–595. |
[19] | Růžička E , Krupička R , Zárubová K , Rusz J , Jech R , Szabó Z ((2016) ) Tests ofmanual dexterity and speed in Parkinson’s disease: Not all measurethe same. Parkinsonism Relat Disord 28: , 118–123. |
[20] | Bologna M , Paparella G , Colella D , Cannavacciuolo A , Angelini L , Alunni-Fegatelli D , Guerra A , Berardelli A ((2020) ) Is there evidence of bradykinesia in essential tremor? Eur J Neurol. |
[21] | Berardelli A , Wenning GK , Antonini A , Berg D , Bloem BR , Bonifati V , Brooks D , Burn DJ , Colosimo C , Fanciulli A , Ferreira J , Gasser T , Grandas F , Kanovsky P , Kostic V , Kulisevsky J , Oertel W , Poewe W , Reese J-P , Relja M , Ruzicka E , Schrag A , Seppi K , Taba P , Vidailhet M ((2013) ) EFNS/MDS-ES/ENS [corrected] recommendations for the diagnosis of Parkinson’s disease. Eur J Neurol 20: , 16–34. |
[22] | Bhatia KP , Bain P , Bajaj N , Elble RJ , Hallett M , Louis ED , Raethjen J , Stamelou M , Testa CM , Deuschl G , the Tremor Task Force of the International Parkinson and Movement Disorder Society ((2018) ) Consensus Statement on the classification of tremors. from the task force on tremor of the International Parkinson and Movement Disorder Society: IPMDS Task Force on Tremor Consensus Statement. Mov Dis 33: , 75–87. |
[23] | Nicoletti A , Mostile G , Nicoletti G , Arabia G , Iliceto G , Lamberti P , Marconi R , Morgante L , Barone P , Quattrone A , Zappia M ((2016) ) Clinical phenotype and risk of levodopa-induced dyskinesia in Parkinson’s disease. J Neurol 263: , 888–894. |
[24] | Kang GA , Bronstein JM , Masterman DL , Redelings M , Crum JA , Ritz B ((2005) ) Clinical characteristics in early Parkinson’s disease in a central California population-based study. Mov Disord 20: , 1133–1142. |
[25] | Bologna M , Guerra A , Paparella G , Giordo L , Alunni Fegatelli D , Vestri AR , Rothwell JC , Berardelli A ((2018) ) Neurophysiological correlates of bradykinesia in Parkinson’s disease. Brain 141: , 2432–2444. |
[26] | Guerra A , Colella D , Giangrosso M , Cannavacciuolo A , Paparella G , Fabbrini G , Suppa A , Berardelli A , Bologna M ((2022) ) Driving motor cortex oscillations modulates bradykinesia in Parkinson’s disease. Brain 145: , 224–236. |
[27] | Bologna M , Leodori G , Stirpe P , Paparella G , Colella D , Belvisi D , Fasano A , Fabbrini G , Berardelli A ((2016) ) Bradykinesia in early and advanced Parkinson’s disease. J Neurol Sci 369: , 286–291. |
[28] | Guerra A , Colella D , Cannavacciuolo A , Giangrosso M , Paparella G , Fabbrini G , Berardelli A , Bologna M ((2023) ) Short-term plasticity of the motor cortex compensates for bradykinesia in Parkinson’s disease. Neurobiol Dis 182: , 106137. |
[29] | Angelini L , Paparella G , De Biase A , Maraone A , Panfili M , Berardelli I , Cannavacciuolo A , Di Vita A , Margiotta R , Fabbrini G , Berardelli A , Bologna M ((2023) ) Longitudinal study of clinical and neurophysiological features in essential tremor. Eur J Neurol 30: , 631–640. |
[30] | Paparella G , Angelini L , De Biase A , Cannavacciuolo A , Colella D , Di Bonaventura C , Giallonardo AT , Berardelli A , Bologna M ((2021) ) Clinical and kinematic features of valproate-induced tremor and differences with essential tremor. Cerebellum 20: , 374–383. |
[31] | Paparella G , Ferrazzano G , Cannavacciuolo A , Cogliati Dezza F , Fabbrini G , Bologna M , Berardelli A ((2018) ) Differential effects of propranolol on head and upper limb tremor in patients with essential tremor and dystonia. J Neurol 265: , 2695–2703. |
[32] | Nasreddine ZS , Phillips NA , Bédirian V , Charbonneau S , Whitehead V , Collin I , Cummings JL , Chertkow H ((2005) ) The Montreal Cognitive Assessment, MoCA: A brief screening tool for mild cognitive impairment. J Am Geriatr Soc 53: , 695–699. |
[33] | Goetz CG , Tilley BC , Shaftman SR , Stebbins GT , Fahn S , Martinez-Martin P , Poewe W , Sampaio C , Stern MB , Dodel R , Dubois B , Holloway R , Jankovic J , Kulisevsky J , Lang AE , Lees A , Leurgans S , LeWitt PA , Nyenhuis D , Olanow CW , Rascol O , Schrag A , Teresi JA , van Hilten JJ , LaPelle N , Movement Disorder Society UPDRS Revision Task Force ((2008) ) Movement Disorder Society-sponsored revision of the Unified Parkinson’s Disease Rating Scale (MDS-UPDRS): Scale presentation and clinimetric testing results. Mov Disord 23: , 2129–2170. |
[34] | Antonini A , Abbruzzese G , Ferini-Strambi L , Tilley B , Huang J , Stebbins GT , Goetz CG , Barone P , MDS-UPDRS Italian Validation Study Group, Bandettini di Poggio M , Fabbrini G , Di Stasio F , Tinazzi M , Bovi T , Ramat S , Meoni S , Pezzoli G , Canesi M , Martinelli P , Maria Scaglione CL , Rossi A , Tambasco N , Santangelo G , Picillo M , Morgante L , Morgante F , Quatrale R , Sensi M , Pilleri M , Biundo R , Nordera G , Caria A , Pacchetti C , Zangaglia R , Lopiano L , Zibetti M , Zappia M , Nicoletti A , Quattrone A , Salsone M , Cossu G , Murgia D , Albanese A , Del Sorbo F ((2013) ) Validation of the Italian version of the Movement Disorder Society–Unified Parkinson’s Disease Rating Scale. Neurol Sci 34: , 683–687. |
[35] | Paparella G , Ceccanti M , Colella D , Cannavacciuolo A , Guerra A , Inghilleri M , Berardelli A , Bologna M ((2021) ) Bradykinesia in motoneuron diseases. Clin Neurophysiol 132: , 2558–2566. |
[36] | Duker AP ((2013) ) Video recording in movement disorders: Practical issues. Continuum (Minneap Minn) 19: , 1401–1405. |
[37] | Bologna M , Latorre A , Di Biasio F , Conte A , Belvisi D , Modugno N , Suppa A , Berardelli A , Fabbrini G ((2016) ) The effect of L-dopa/carbidopa intestinal gel in Parkinson disease assessed using neurophysiologic techniques. Clin Neuropharmacol 39: , 302–305. |
[38] | Bologna M , Guerra A , Colella D , Cioffi E , Paparella G , Di Vita A , D’Antonio F , Trebbastoni A , Berardelli A ((2020) ) Bradykinesia in Alzheimer’s disease and its neurophysiological substrates. Clin Neurophysiol 131: , 850–858. |
[39] | Colella D , Guerra A , Paparella G , Cioffi E , Di Vita A , Trebbastoni A , Berardelli A , Bologna M ((2021) ) Motor dysfunction in mild cognitive impairment as tested by kinematic analysis and transcranial magnetic stimulation. Clinical Neurophysiol 132: , 315–322. |
[40] | De Biase A , Paparella G , Angelini L , Cannavacciuolo A , Colella D , Cerulli Irelli E , Giallonardo AT , Di Bonaventura C , Berardelli A , Bologna M ((2022) ) Tremor and movement slowness are two unrelated adverse effects induced by valproate intake. Mov Disord Clin Pract 9: , 1062–1073. |
[41] | Landis JR , Koch GG ((1977) ) The measurement of observer agreement for categorical data. Biometrics 33: , 159–174. |
[42] | Hornabrook RW , Nagurney JT ((1976) ) Essential tremor in Papua, New Guinea. Brain 99: , 659–672. |
[43] | Geraghty JJ , Jankovic J , Zetusky WJ ((1985) ) Association between essential tremor and Parkinson’s disease. Ann Neurol 17: , 329–333. |
[44] | Cleeves L , Findley LJ , Koller W ((1988) ) Lack of association between essential tremor and Parkinson’s disease. Ann Neurol 24: , 23–26. |
[45] | Lou JS , Jankovic J ((1991) ) Essential tremor: Clinical correlates in 350 patients. Neurology 41: , 234–238. |
[46] | Koller WC , Busenbark K , Miner K ((1994) ) The relationship of essential tremor to other movement disorders: Report on 678 patients. Essential Tremor Study Group. Ann Neurol 35: , 717–723. |
[47] | Tallón-Barranco A , Vázquez A , Javier Jiménez-Jiménez F , Ortí-Pareja M , Gasalla T , Cabrera-Valdivia F , Benito-León J , Molina JA ((1997) ) Clinical features of essential tremor seen in neurology practice: A study of 357 patients. Parkinsonism Relat Disord 3: , 187–190. |
[48] | Jiménez-Jiménez FJ , Rubio L , Alonso-Navarro H , Calleja M , Pilo-de-la-Fuente B , Plaza-Nieto JF , Benito-León J , García-Ruiz PJ , Agúndez JA ((2010) ) Impairment of rapid repetitive finger movements and visual reactiontime in patients with essential tremor. Eur J Neurol 17: , 152–159. |
[49] | Fekete R , Li J ((2013) ) Clinical differentiation of essential tremor and Parkinson’s disease. Clin Med Insights Case Rep 6: , CCRep.S11903. |
[50] | Erro R , Pilotto A , Esposito M , Olivola E , Nicoletti A , Lazzeri G , Magistrelli L , Dallocchio C , Marchese R , Bologna M , Tessitore A , Misceo S , Gigante AF , Terranova C , Moschella V , di Biase L , Di Giacopo R , Morgante F , Valentino F , De Rosa A , Trinchillo A , Malaguti MC , Brusa L , Matinella A , Di Biasio F , Paparella G , De Micco R , Contaldi E , Modugno N , Di Fonzo A , Padovani A , Barone P; TITAN Study Group ((2022) ) The Italian tremor Network (TITAN): Rationale, design and preliminary findings. Neurol Sci 43: , 5369–5376. |
[51] | Tarakad A , Jankovic J ((2018) ) Essential tremor and Parkinson’s disease: Exploring the relationship. Tremor Other Hyperkinet Mov (N Y) 8: , 589. |
[52] | Thenganatt MA , Jankovic J ((2016) ) The relationship between essential tremor and Parkinson’s disease. Parkinsonism Relat Disord 22: Suppl 1, S162–165. |