Effect of robot-assisted gait training on motor dysfunction in Parkinson’s patients:A systematic review and meta-analysis
Abstract
BACKGROUND:
Robot-assisted gait training (RAGT) has been reported to treat motor dysfunction in patients with Parkinson’s disease (PD) in the last few years. However, the benefits of RAGT for treating motor dysfunction in PD are still unclear.
OBJECTIVES:
To investigate the efficacy of RAGT for motor dysfunction in PD patients.
METHODS:
We searched PubMed, Web of Science, Cochrane Library, Embase, CNKI, Wanfang, Chinese Biomedical Literature Database (CBM), and Chinese VIP Database for randomized controlled trials investigating RAGT to improve motor dysfunction in PD from the databases’ inception dates until September 1, 2022. The following outcome indexes were employed to evaluate motor dysfunction: the Berg Balance Scale (BBS), Activities-specific Balance Confidence Scale (ABC), 10-Meter Walk Test gait speed (10-MWT), gait speed, stride length, cadence Unified Parkinson Disease Rating Scale Part III (UPDRS III), 6-Minute Walk Test (6MWT), and the Timed Up and Go test (TUG). The meta-analysis was performed using the proper randomeffect model or fixed-effect model to evaluate the difference in efficacy between the RAGT and the control groups. The Cochrane Risk of Bias Tool was used for the included studies and Grading of Recommendations, Assessment, Development, and Evaluations (GRADE) was used to interpret the certainty of the results.
RESULTS:
The results consisted of 17 studies comprising a total of 670 participants. Six hundred and seven PD patients with motor dysfunction were included: 335 in the RAGT group and 335 in the control group. This meta-analysis results established that when compared with the control group, robot-assisted gait training improved the BBS results of PD patients (MD: 2.80, 95%CI: 2.11–3.49,
CONCLUSION:
Even though RAGT can improve balance function, walking function, and gait performance and has demonstrated positive results in several studies, there is currently insufficient compelling evidence to suggest that it can improve all aspects of lower motor function.
1.Introduction
Parkinson’s disease (PD) is the second most common neurodegenerative disease, causes deterioration of motor activities, and occurs due to impairment of the dopaminergic nigrostriatal system [1, 2]. The probability of an individual developing PD is directly correlated with age [3]. PD diagnoses are predicted to increase from six million in 2015 to an estimated twelve million by 2040 by which time around one percent of the global population will be over sixty years old [4, 5, 6]. The established symptoms of PD affect an individual’s motor functions and include diagnoses of bradykinesia, cogwheel rigidity, resting tremor, a slow shuffling gait, postural instability, and imbalance [7] all of which have a severe impact on a patient’s quality of life, reduce their productivity, and place strain on medical resources and healthcare providers [8].
Existing PD management strategies aim to provide symptomatic relief and decelerate the disease’s progression [9]. The current treatment paradigm for PD requires the employment of anti-Parkinson medications, which often fail to adequately treat the disease and provide negligible or no long-term improvement of the patient’s condition [10]. Additionally, as the disease develops, the patient will require increased doses of medication which can increase the risk of physical complications such as dyskinesia [11]. Adjunctive treatments and complementary therapy, such as exercise, can diminish the side effects induced by anti-PD therapeutics and have been proven to effectively improve motor disorders such as balance, gait, risk of falls and physical function while reducing the risk of falls [12, 13]. Over the last few years, robot-assisted gait training (RAGT) has been widely used as a new physical therapy technique for neurological disorders such as stroke, spinal cord injury, and PD [14, 15, 16]. The fundamental principles of RAGT concern the promotion of neuroplasticity and cortical reorganization to enhance the walking function in PD patients; this is achieved via the implementation of task-specific activities and high-intensity repetitive training aimed at improving lower extremity motor function [17]. RAGT reinforces multisensory stimulation in walking training and focuses on achieving the correct performance of gait movements to enhance walking and gait function [18]. Recent studies have demonstrated that RAGT improves bradykinesia, motivation, freezing, rigidity, gait, leg agility and posture demonstrating the potential to be a feasible and dependable rehabilitation paradigm [19, 20, 21]. By contrast, the results of a prior meta-analysis contradict these findings and posit that RAGT does not appear to be a more effective approach than the control intervention of conventional rehabilitation training [22]. Additionally, certain studies suggested that RAGT did not demonstrate superiority over conventional rehabilitation training designed to improve gait function [20], walking function or balance function aspects [23]. Currently, there is insufficient high-quality evidence-based medical data to determine whether RAGT treatment positively affects motor dysfunction symptoms in PD patients.
This systematic review and meta-analysis of randomized controlled trials (RCTs) was conducted to investigate and identify the effectiveness of RAGT on motor function in PD patients, further analyzing the efficacy on balance function, walking function and gait dysfunction.
2.Materials and methods
This systematic review was conducted and reported according to the Preferred Reporting Items for Systematic Reviews and Meta-Analysis (PRISMA) statement and followed the recommendations of the Cochrane Collaboration Handbook [24]. The PROSPERO register number is CRD42022359363.
2.1Search strategy
This study performed the search strategy according to PRISMA criteria. This study conducted a comprehensive systematic search of the following databases: PubMed, Embase, Web of Science, Cochrane Library, Chinese National Knowledge Infrastructure (CNKI), Chinese Biomedical Databases (CBMs), Chinese VIP, and Wanfang Database from inception to September 1, 2022. The search formula included words such as “Parkinson disease”, “robot-assisted gait training” “RAGT”, and “randomized controlled trials”. The search parameters necessitated a specific approach for combinations of the Medical Subject Headings (MeSH) and free words which required the implementation of unambiguous, pre-defined keywords for each database. The literature search was limited to RCTs and involved screening the reference lists for relevant and potential articles. The Supplement details the specific search strategy for all databases.
2.2Selection criteria
All references were managed using EndNote X9 (Thomson ResearchSoft, Stamford, CT, USA), and any duplicates were removed. Two reviewers (QX and QC) independently screened the records by title and abstract, eliminating irrelevant studies. The full text of the remaining articles was carefully examined and compared against the eligibility criteria. If discrepancies occurred between the two reviewers, the third experienced reviewer (WS) was invited to resolve the issue via discussion.
Two independent reviewers (QX and QC) nominated studies that fulfilled the following inclusion criteria: (1) the study design is RCT; (2) the eligible population must consist of PD patients experiencing motor dysfunction; (3) the experimental group required the use of RAGT (or RAGT combined with conventional rehabilitation e.g., conventional exercise training and treadmill training) while the control group used only conventional rehabilitation such as conventional exercise training and treadmill training. The following were not included in our systematic review: (1) poor quality studies with design defects; (2) studies with insufficient data; (3) animal experiments, case reports, reviews, non-RCTs or duplicate publications; (4) the subjects combined with various organ dysfunction or other serious diseases.
2.3Data extraction and outcome measures
Original data, including the first author, year of publication, sample size, age, sex, intervention treatment measured outcomes and intervention period, were independently extracted from the articles by two authors (GC and JL) and recorded in a standardized form. The means and standard deviations (M
2.4Risk of bias assessment
The risk of bias in all articles was evaluated using the Cochrane Collaboration recommendations [25]. The following information was assessed: random sequence generation, allocation concealment, blinding of participants and personnel, blinding of outcome assessments, incomplete outcome data, selective reporting and other bias. The methodological quality of all articles was independently assessed by two reviewers (LY and DZ). Any disagreements in the risk bias assessment were settled by consulting a third reviewer (XJ).
2.5Level of evidence
Two reviewers (QX and SW) assessed the evidence level by using the Grading of Recommendations, Assessment, Development and Evaluation (GRADE) approach [26]. Evidence was evaluated as very low, low, moderate or high on the basis of the following five items: risk of bias, inconsistency, imprecision, indirectness of evidence and publication bias. When a disagreement occurred in the assessment of the risk of bias and quality level, a third reviewer (JZ) adjudicated the disagreement.
2.6Statistical analysis
Review Manager version 5.3 (The Cochrane Collaboration, Copenhagen, Denmark) was used to perform the meta-analysis. The continuous variables were analyzed in combination with the Mean Difference (MD) and 95% Confidence Interval (CI). The heterogeneity of each study was evaluated using the Cochrane Q test and I2 test; if case I250%, heterogeneity was adjudged to exist between studies. A random-effects model was used in cases of significant heterogeneity. Otherwise, a fixed-effects model was used. Whenever significant heterogeneity is present, the sensitivity analysis was performed by sequentially excluding studies to explore the effect of each selected study on overall estimates, and the stability of the results of I2 and the changes in the combined effect size after excluding studies were analyzed. We reported the results of a narrative and descriptive summary if insufficient data were pooled. Publication bias was assessed by visual inspection using the Begg test.
Figure 1.
Flow diagram of the study selections.
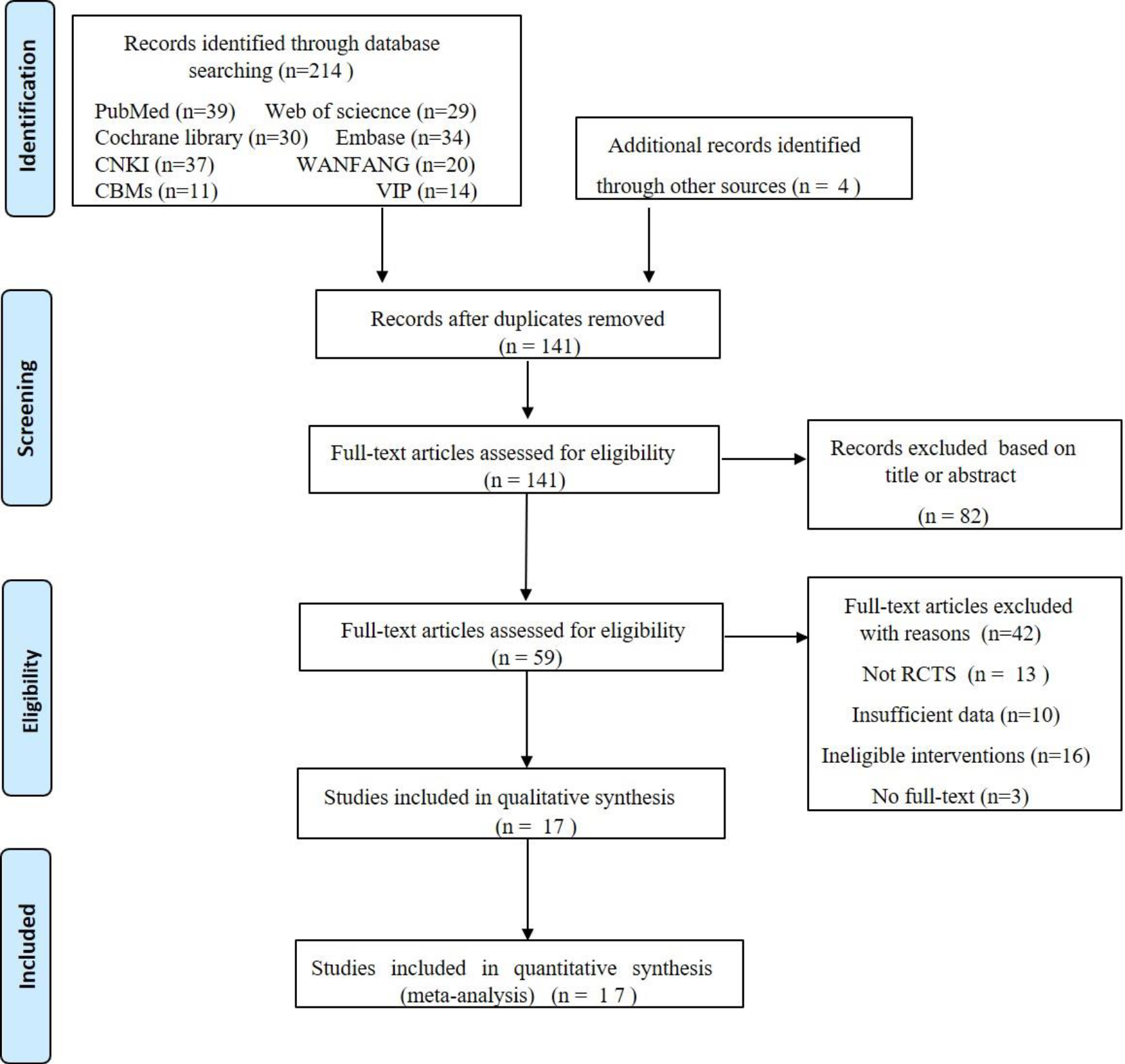
3.Results
3.1Search strategy results
In Fig. 1, we describe the studies filtering process in detail. Initially, the computerized literature search identified 218 relevant studies which, following the elimination of duplicate studies, we reduced to 141. After reading the titles and abstracts, we excluded 82 studies because they were repetitive studies unrelated to our research topic, including conference papers, reviews meta-analyses, case reports, or studies of other diseases. We downloaded and read the full texts of the remained 59 studies. Forty-two studies were excluded for the following reasons: (1) Not RCTs (
3.2Baseline characteristics and quality assessment of the included studies
3.2.1Baseline characteristics
A total of 17 randomized controlled trial studies [20, 23, 27, 28, 29, 30, 31, 32, 33, 34, 35, 36, 37, 38, 39, 40, 41] were included in this meta-analysis. All studies were RCTs published from 2012 [27, 28, 29] to 2022 [20, 38, 39]. A total of 670 participants were included, with the number of participants in each study varying from 15 [38] to 96 [36], and the mean age of participants was from 65.2 [37] years to 77.7 [34] years. The frequency of treatment in each study ranged from 10 times in 12 weeks [38] to six times a week [34], with the duration of RAGT being 4 week [20, 23, 27, 28, 29, 30, 31, 32, 34, 35, 36, 39, 40], 8 week [33, 37] or 12 week [38]. The interventions considered for the experimental groups were diverse and included RAGT, RAGT with conventional exercise training and RAGT with treadmill training. The control groups received conventional exercise training or treadmill training. Detailed information regarding the trials and patients included in this study is presented in Table 1.
Table 1
Characteristics of the included studies
Included studies | No. of patients (T/C) | Age (year) (T/C) | Sex (M/F) (T/C) | Study group | Control group | Outcome | Intervention time | Robot type |
---|---|---|---|---|---|---|---|---|
Carda et al. [27] | 15/15 | 67.87 |
| RAGT | Treadmill training | (3)(7)(8)(9) | 4 w 3 times/week, 30 min/session | Lokomat (Hocoma AG, Volketswil, Switzerland) |
Picelli et al. [28] | 18/18 | 68.1 | 10/8–6/12 | RAGT | Conventional exercise training | (3)(6)(7)(9) | 4 w 3 times/week, 45 min/session | Gait-Trainer GT1 (RehaStim, Berlin, Germany) |
Picelli et al. 2012b [29] | 16/15 | 68.3 | 20–14 | RAGT | Conventional exercise training | (1)(2)(3)(7)(8) | 4 w 3 times/week, 40 min/s session | Gait-Trainer GT1 (RehaStim, Berlin, Germany) |
Picelli et al. 2013 [30] | 20/20 | 68.5 | 9/11– 6/14 | RAGT | Treadmill training | (1)(3)(6)(7) | 4 w 3 times/week 45 min/session | Gait-Trainer GT1 (RehaStim, Berlin, Germany) |
Sale et al. [31] | 10/10 | 70.27 | 6/4–5/5 | RAGT | Treadmill training | (4)(5)(6) | 4 w 5 times/week, 45-minute sessions | G-EO system device (Reha Technology AG; Olten, Switzerland) |
Picelli et al. [23] | 33/33 | 68.2 | 26/7–22/11 | RAGT | Conventional exercise training | (1)(2)(7)(8) | 4 w 3 times/week, 45 min/session | Gait-Trainer GT1 (RehaStim, Berlin, Germany) |
Chen et al. [33] | 20/20 |
|
| RAGT | Conventional exercise training | (1)(4)(5)(8) | 8 w 5 times/week, 30 min/session | Lokomat (Hocoma AG, Volketswil, Switzerland) |
Galli et al. [32] | 25/25 | 68.8 | 14/11– 12/13 | RAGT | Treadmill training | (4)(6)(7) | 4 w 5 times/week, 45 min/session | G-EO system device (Reha Technology AG; Olten, Switzerland) |
Furnari et al. [34] | 19/19 | 71.5 | 11/8–10/9 | RAGT | Conventional exercise training | (7) | 4 w 6 times/week, 60 min/session | Lokomat (Hocoma, Zurich, Switzerland) |
Xia et al. [35] | 15/15 | 70.3 | 8/7– 7/8 | RAGT | Conventional exercise training | (3)(4)(5)(6) (7)(8) | 4 w 3 times/week, 20 min/session | Lokomat (Hocoma AG, Volketswil, Switzerland) |
Capecci et al. [36] | 48/48 | 68.1 | 19/29–24/24 | RAGT | Treadmill training | (3)(7)(8)(9) | 4 w 5 times/week 45 min/session | G-EO system device (Reha Technology AG; Olten, Switzerland) |
Table 1, continued | ||||||||
---|---|---|---|---|---|---|---|---|
Included studies | No. of patients (T/C) | Age (year) (T/C) | Sex (M/F) (T/C) | Study group | Control group | Outcome | Intervention time | Robot type |
Ma et al. [37] | 20/20 | 65.2 | 8/12– 7/13 | RAGT | Conventional exercise training | (1)(4)(7)(9) | 8w 5 times/week, 50 min/session |
|
Spina et al. [41] | 11/11 | 68.6 | 5/6– 4/7 | RAGT | Conventional exercise training | (1)(3) | 4 w 5 times/week 45 min/session | Robotic device hunova® (Movendo Technology, Genoa, Italy) |
Lin et al. [40] | 16/16 | 66.7 | 8/8– 7/9 | RAGT | Conventional exercise training | (1)(4)(8) | 8w 5 times/week, 50 min/session | Flexbot lower limb rehabilitation robot (Shanghai Jinghe Robotics Co., Ltd. China) |
Kawashima et al. [38] | 7/8 | 77.0 | 3/4– 1/7 | RAGT | Overground gait training | (1)(3)(4)(6)(7) | 12w 10 times/ 12 weeks 30 min/session | Exoskeleton Stride Management Assist (Honda R&D, Tokyo, Japan). |
Kim et al. [20] | 22/22 | 68.7 | 6/16–7/15 | RAGT | Treadmill training | (1)(3)(8) | 4 w 3 times/week 45 min/session | Treadmill-based exoskeleton robot, the Walkbot-S (P&S Mechanics, Seoul, Korea). |
Ding et al. [39] | 20/20 | 71.35 | 13/7–12/8 | RAGT | Conventional exercise training | (1)(2)(7)(8) | 4 w 5 times/week 60 min/session | G-EO system device (Reha Technology AG; Olten, Switzerland) |
NOTE: –
Figure 2.
Risk of bias summary.
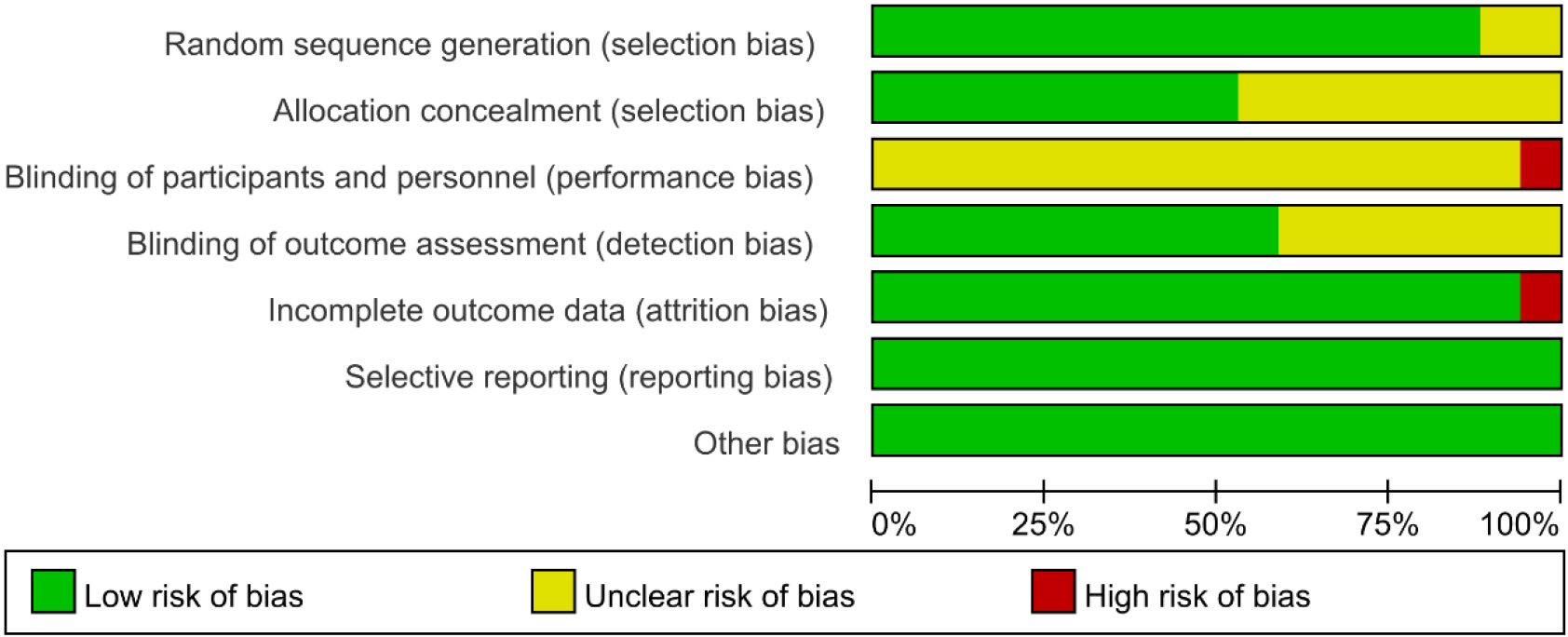
Figure 3.
Risk of bias graph (presented as percentages).
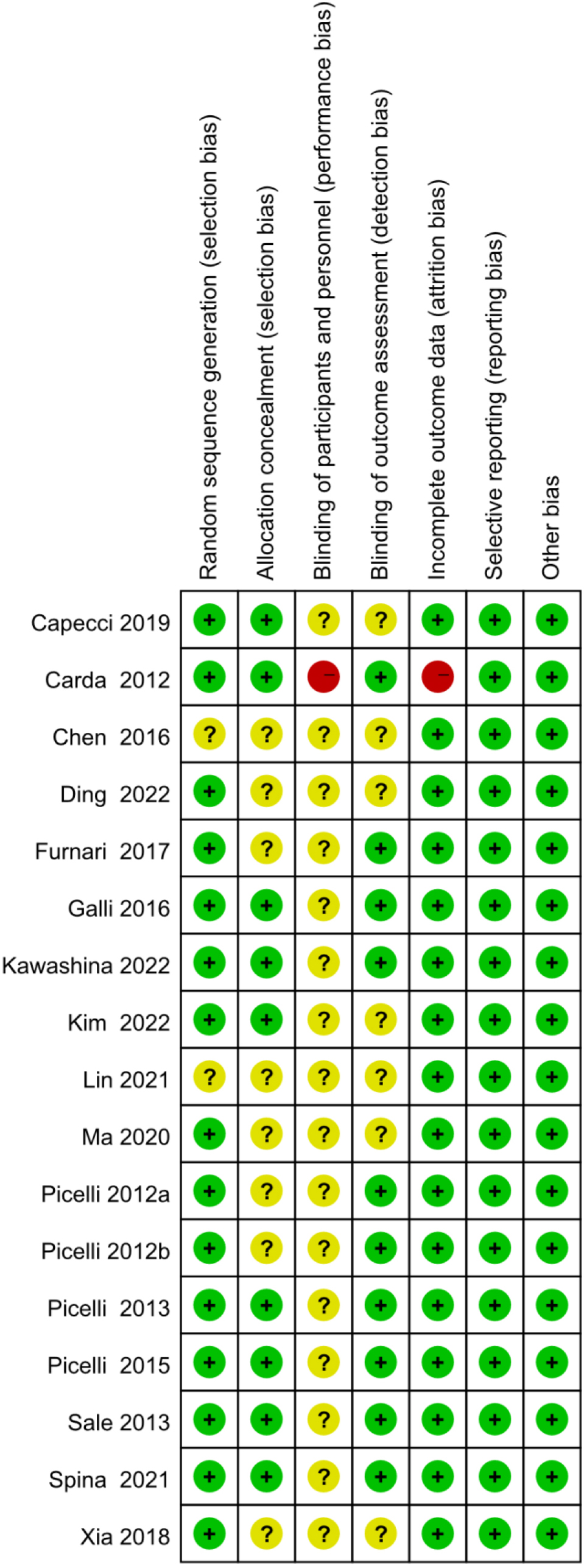
3.2.2Quality assessment of the included studies
Methodological quality assessment was performed according to Preferred Reporting Items for Systematic Reviews and Meta-analysis guidelines. The quality assessment results for the included studies are shown in Figs 2 and 3. The method of randomization was described by all 17 studies, and fourteen of them detailed the randomization methods [20, 23, 27, 28, 29, 30, 31, 32, 35, 36, 37, 38, 39, 41]. Allocation concealment methods were described in nine of the studies [23, 27, 30, 31, 32, 36, 41]. In one of the studies [27], the blinding of participants and personnel were described as unblind, and thus, we judged blinding a high risk of bias. Nine studies clearly reported the blinding of outcome assessment [23, 27, 28, 29, 30, 31, 32, 34, 41]. However, incomplete outcome data reason was not mentioned in one study [27], and thus, we judged incomplete outcome data a high risk of bias. In addition, all studies were at low risk of selective reporting and other forms of bias [20, 23, 27, 28, 29, 30, 31, 32, 33, 34, 35, 36, 37, 38, 39, 40, 41].
Figure 4.
Forest plot of the comparison of the BBS between the experimental and control groups.
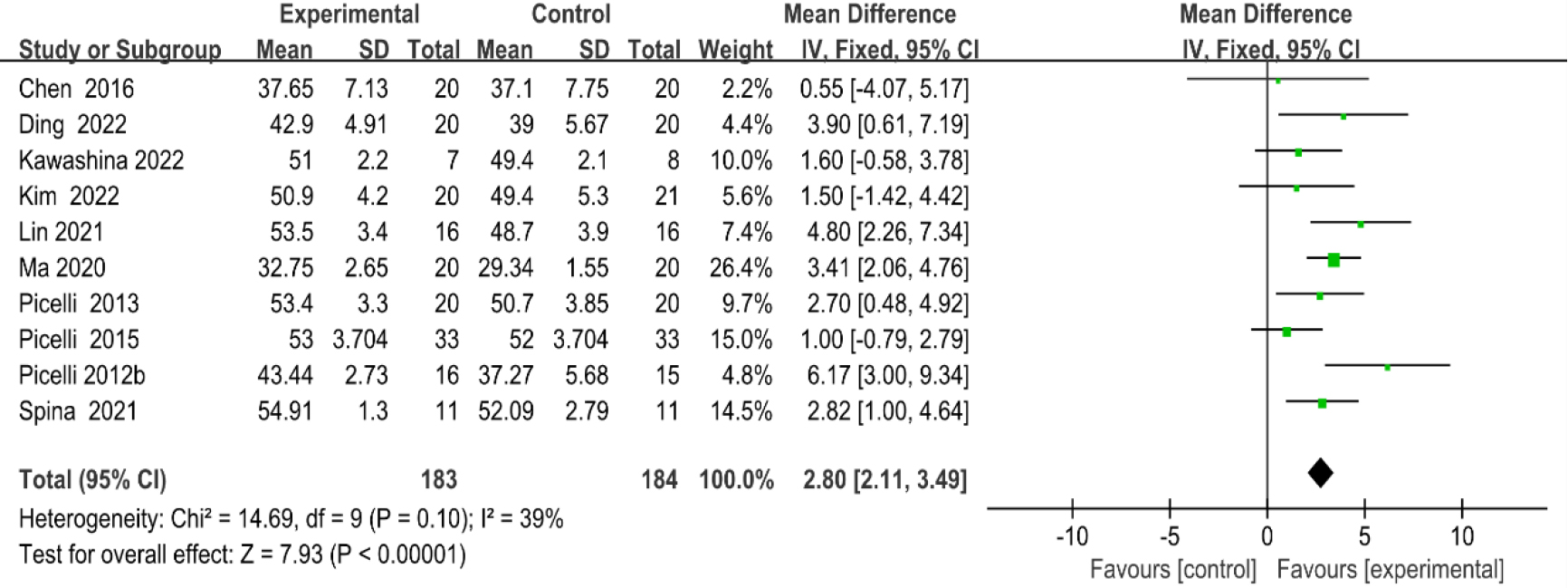
3.3Meta-analysis
3.3.1Berg balance scale
As shown in Fig. 4, ten studies [20, 23, 29, 30, 33, 37, 38, 39, 40, 41] provided data regarding BBS. There were 367 cases which consisted of 183 cases in the RAGT group and 184 cases in the non-RAGT group. The analyses of heterogeneity revealed no obvious heterogeneity between the results of each study (P
Figure 5.
Forest plot of the comparison of the ABC between the experimental and control groups.
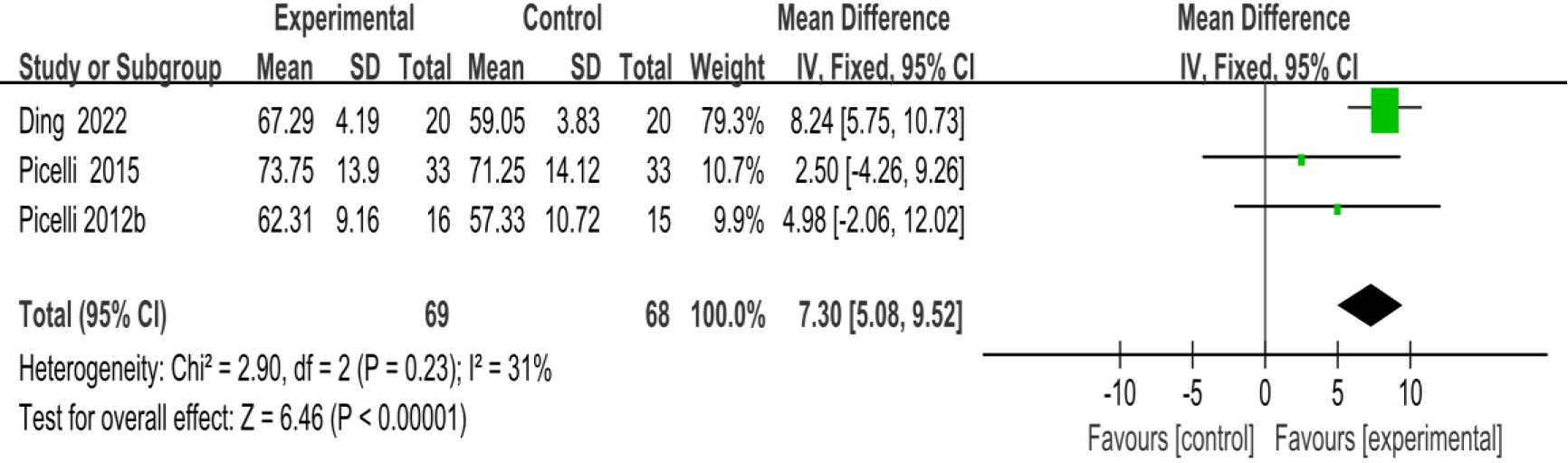
3.3.2Activities-specific balance confidence scale
As shown in Fig. 5, three studies [23, 29, 39] provided data concerning ABC. There were 137 cases which consisted of 69 cases in the RAGT group and 68 cases in the non-RAGT group. The analyses of heterogeneity revealed no obvious heterogeneity between the results of each study (
Figure 6.
Forest plot of the comparison of the 10-MWT between the experimental and control groups.
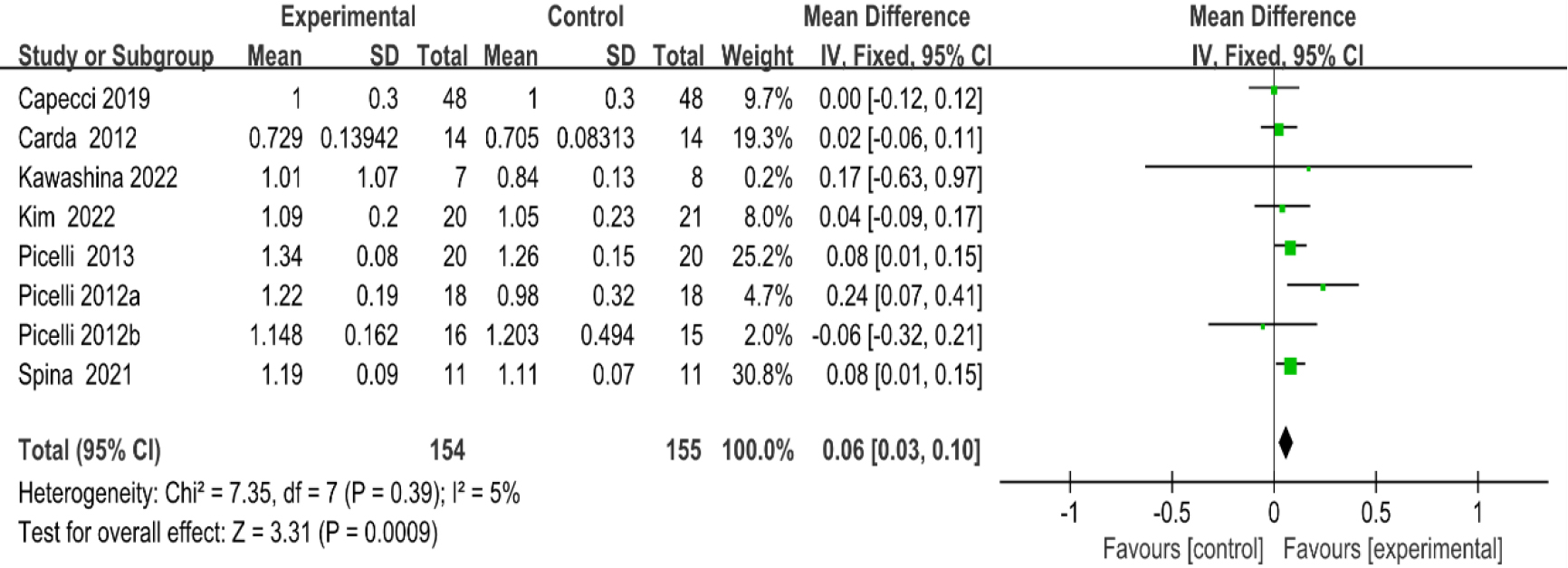
3.3.310-m walk test
As shown in Fig. 6, eight studies [20, 27, 28, 29, 30, 35, 36, 38, 41] provided data concerning 10-MWT. There were 309 cases which consisted of 154 cases in the RAGT group and 155 cases in the non-RAGT group. The analyses of heterogeneity revealed no obvious heterogeneity between the results of each study (
3.3.4Gait speed
As shown in Fig. 7, seven studies [31, 32, 33, 35, 37, 38, 40] provided data concerning gait speed. There were 227 cases which consisted of 113 cases in the RAGT group and 114 cases in the non-RAGT group. The analyses of heterogeneity revealed no obvious heterogeneity between the results of each study (
3.3.5Stride length
As shown in Fig. 8, five studies [28, 30, 31, 33, 35] provided data concerning stride length. There were 166 cases which consisted of 83 cases in the RAGT group and 83 cases in the non-RAGT group. The analyses of heterogeneity revealed no obvious heterogeneity between the results of each study (
3.3.6Cadence
As shown in Fig. 9, five studies [28, 30, 31, 32, 35] provided data concerning cadence. There were 176 cases which consisted of 88 cases in the RAGT group and 88 cases in the non-RAGT group. The analyses of heterogeneity revealed no obvious heterogeneity between the results of each study (
Table 2
Summary of findings of certainty of the evidence
Outcome | No. of studies | No. of participants | Risk of bias | Inconsistency | Indirectness | Imprecision | Publication bias | SMD/MD (95% CI) | Certainty |
---|---|---|---|---|---|---|---|---|---|
BBS | 10 | 367 | Serious | No | No | Serious | No | 2.80 (2.11–3.49) | Low |
ABC | 3 | 137 | Serious | No | No | Serious | No | 7.30 (5.08–9.52) | Low |
10MWT | 8 | 309 | Serious | No | No | Serious | No | 0.06 (0.03–0.10) | Low |
Gait speed | 7 | 227 | Serious | No | No | Serious | No | 3.67 (2.58–4.76) | Low |
Stride length | 5 | 166 | Serious | No | No | Serious | No | 5.53 (3.64–7.42) | Low |
Cadence | 5 | 176 | Serious | No | No | Serious | No | 4.52 (0.94–8.10) | Low |
UPDRS III | 11 | 474 | Serious | No | No | Serious | No | Low | |
TUG | 9 | 372 | Serious | No | No | Very serious | Yes | Very low | |
6MWT | 5 | 240 | Serious | Very serious | No | Serious | No | 13.87 (11.92–15.82) | Very low |
Figure 7.
Forest plot of the comparison of the Gait speed between the experimental and control groups.
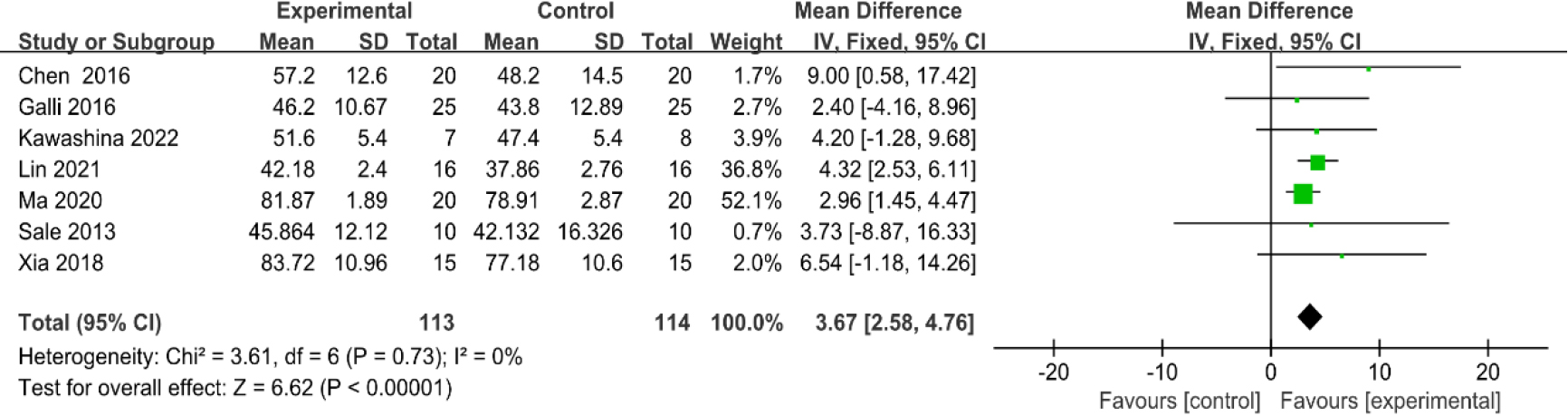
Figure 8.
Forest plot of the comparison of the stride length between the experimental and control groups.
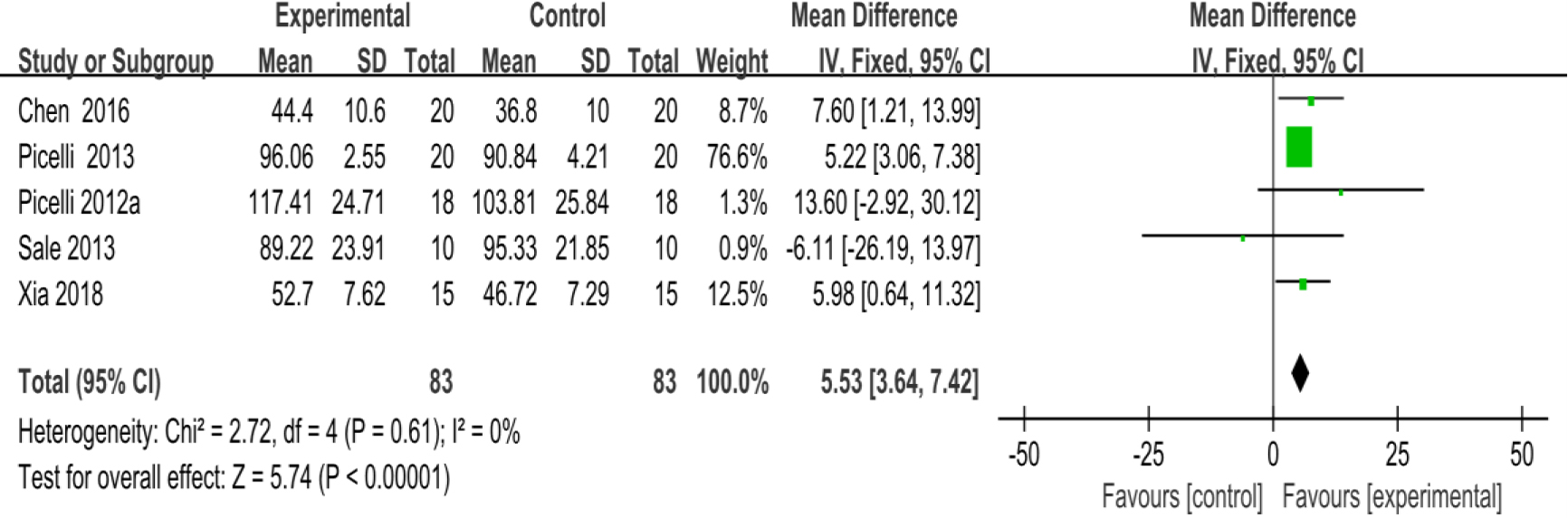
3.3.7Unified parkinson disease rating scale part III (UPDRS III)
As shown in Fig. 10, eleven studies [23, 27, 28, 29, 30, 32, 34, 35, 36, 38, 39] provided data concerning UPDRS III. There were 474 cases which consisted of 237 cases in the RAGT group and 237 cases in the non-RAGT group. The analyses of heterogeneity revealed no obvious heterogeneity between the results of each study (
3.3.8Timed up and go (TUG) test
As shown in Fig. 11, nine studies [20, 23, 27, 29, 33, 35, 36, 39, 40] provided data concerning TUG test. There were 372 cases which consisted of 186 cases in the RAGT group and 186 cases in the non-RAGT group. The analyses of heterogeneity showed no obvious heterogeneity between the results of each study (
3.3.96-minute walk test (6MWT)
Five studies [27, 28, 30, 36, 37] provided data concerning 6MWT. There were 240 cases which consisted of 120 cases in the RAGT group and 120 cases in the non-RAGT group, respectively. The analyses of heterogeneity demonstrated significant heterogeneity between the results of each study (
3.3.10Publication bias
Funnel plots were drawn for the studies on BBS with the more and important outcome indicators in the included studies. Most studies were distributed in the 95% CI range of the inverted funnel plot and had a bit symmetrical, suggesting that there was a certain publication bias in the funnel plot (Fig. 13).
3.3.11Quality of evidence (GRADE)
The evidence level according to GRADE ranged from very low to low, and a moderate or high level of evidence was not observed. Table 2 displays the details of the GRADE assessment.
Note: Risk of bias: No/serious/very serious: Most of the information comes from studies with low/moderate/high risk of bias. Inconsistency: Serious: I2
4.Discussion
Figure 9.
Forest plot of the comparison of the cadence between the experimental and control groups.
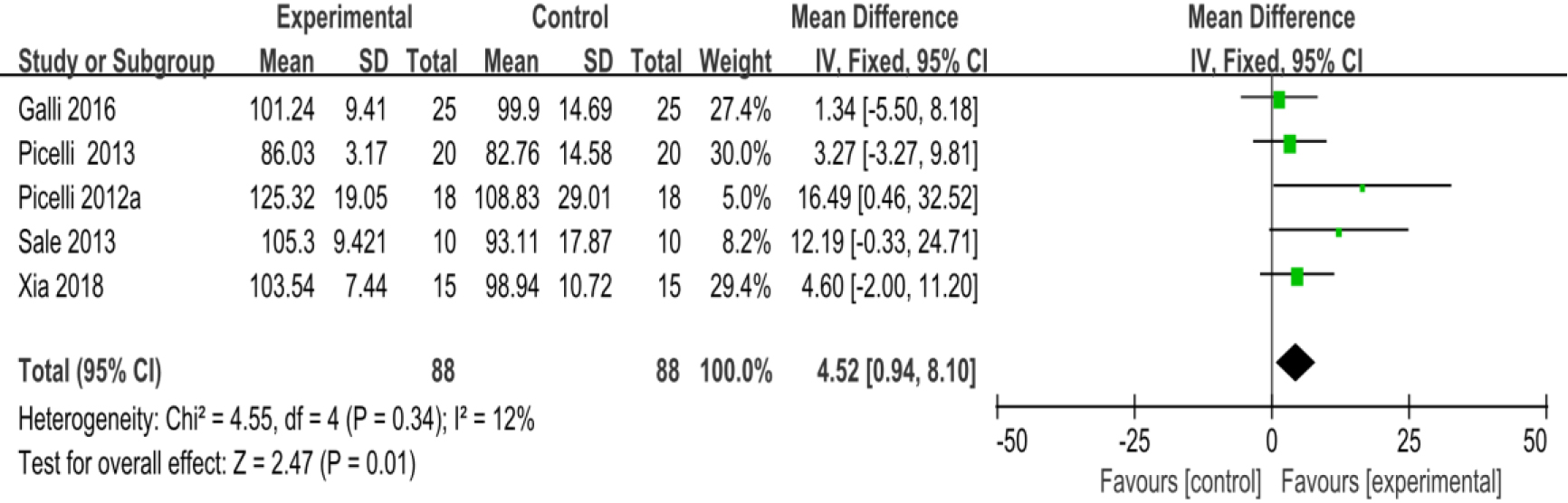
Figure 10.
Forest plot of the comparison of the UPDRS III between the experimental and control groups.
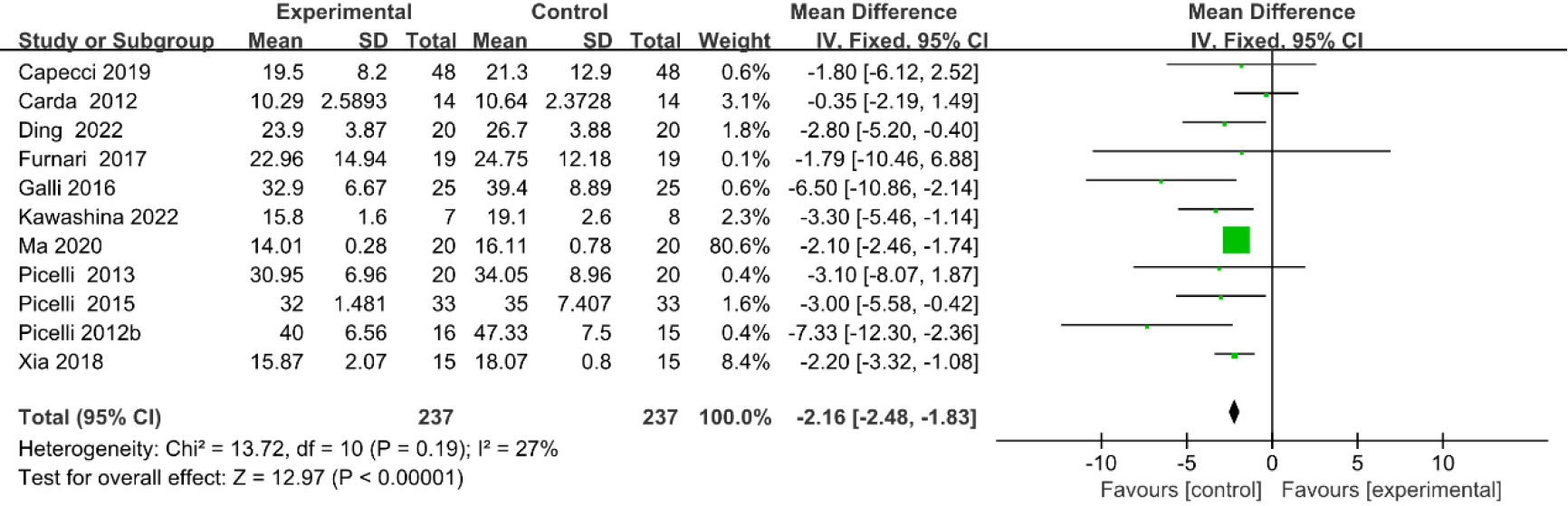
Figure 11.
Forest plot of the comparison of the TUG between the experimental and control groups.
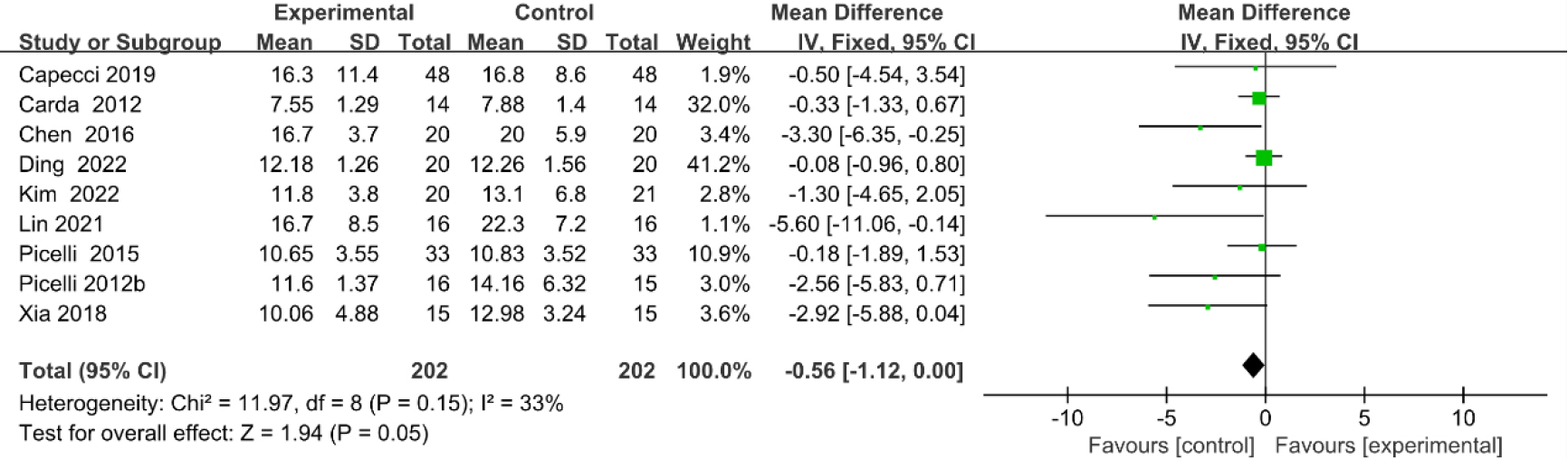
With the onset and development of PD, postural instability deteriorates and gait difficulties become exacerbated, increasing the risk of falls and severely impacting a patient’s well-being and health [42]. The current treatment program involves the use of exercise and adaptive physical activity to address balance instability, gait disturbances, and the static postural changes resulting from PD [43]. These strategies are, currently, the most beneficial approaches for improving PD motor dysfunction [44], while RAGT represents an opportunity to establish a new physical therapy paradigm for the treatment of PD.
Figure 12.
Forest plot of the comparison of the 6MWT between the experimental and control groups.
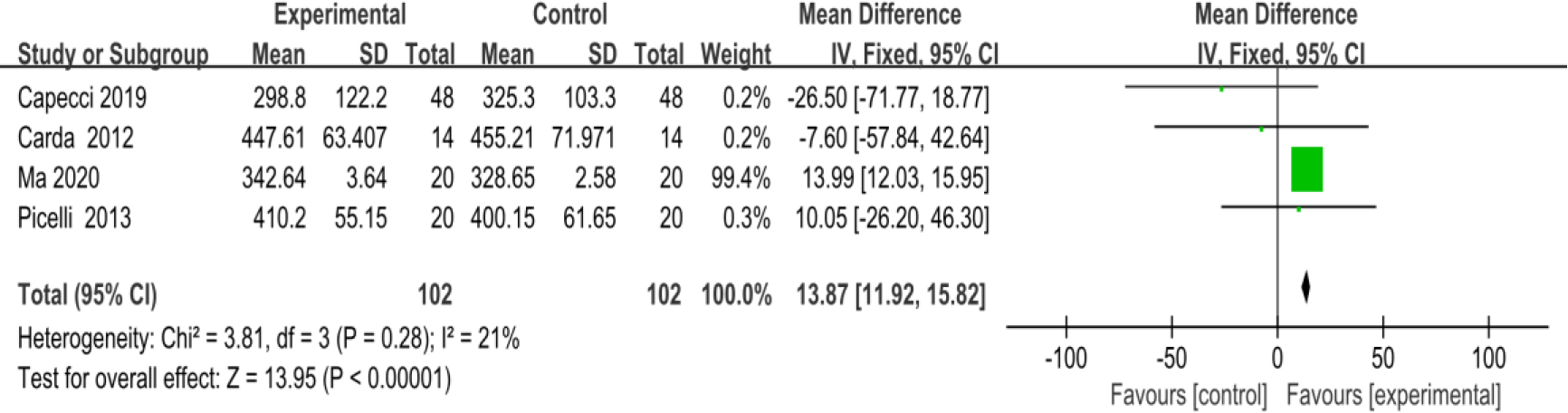
Figure 13.
Funnel plot for evaluating the publication bias of BBS.
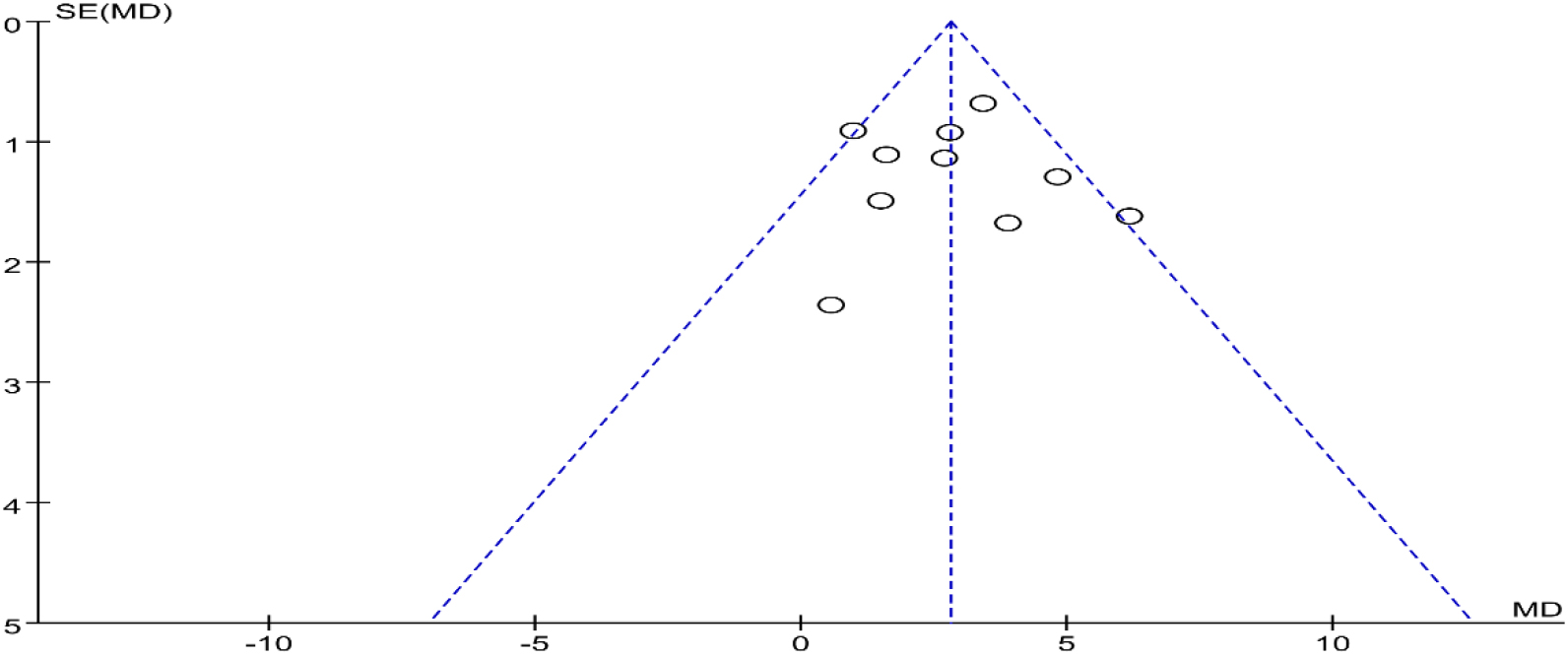
This systematic review and meta-analysis involved 17 RCTs with high quality ratings in the literature to comprehensively assess the effectiveness of RAGT in treating lower extremity motor dysfunction in PD patients. This study found that RAGT therapy achieved superior results compared to conventional rehabilitation training based on improved walking function, balance function and gait performance, even though there was no significant difference in certain balance function assessments such as the TUG test. However, the certainty of the evidence was low to very low by GRADE which may have some impact on the reliability of the results of this study.
The results of the meta-analysis established that, when compared with the control group, the RAGT intervention demonstrated significant statistical significance in the BBS test for assessing the risk of falls and the ABC test for measuring balance confidence and achieved improved efficacy in balance function [45, 46]. Spina et al. noted that robot-assisted balance training significantly improves balance function in mild PD patients in comparison with traditional balance training [41]. RAGT interventions contain the appropriate level of intensity, repetition, difficulty, and complexity, to promote the neuroplastic changes required to improve motor skills and strengthen the lower leg muscles [18]. Additionally, RAGT may exert a proprioceptive cueing effect by providing an external rhythm that compensates for the defective internal rhythm of the basal ganglia [47] allowing for earlier and more targeted balance training to improve balance and promote overall motor function recovery, which could effectively prevent accidental falls [36]. This study did not detect any significant statistical difference when analyzing the TUG test; this may be attributed to the condition of the PD patient, specifically the motor retardation, which occurs during turning and requires a higher dynamic balance function [48]. Primarily, the TUG test is employed to assess a senior citizen’s fall risk, and there is still a lack of evidence concerning patients with neurological disorders such as PD [49].
This meta-analysis has important implications for clinical practice. UPDRS III motor aspect scores are the optimum measure of the severity of PD symptoms, while 6MWT and 10MWT are used to assess walking function [50]. The amalgamated meta-analysis results demonstrate that RAGT was more statistically significant than the control group when examining UPDRS III, 6MWT, and 10MWT scores and can significantly improve walking function. Capecci et al. [36] noted that repetitive intensive gait training is an effective treatment for PD patients, particularly those with a severe walking disability This meta-analysis also demonstrated that RAGT was more effective than conventional rehabilitation in improving parameters such as gait speed, stride length, and cadence, which significantly improves the gait of PD patients. Even though an earlier pooled meta-analysis conducted by Alwardat et al. [22] did not detect any significant changes regarding stride time and cadence, RAGT has been shown to significantly improve gait function for PD patients in some recent clinical studies [20, 38]. RAGT devices can facilitate sensory feedback via proprioception provide safe protection for walking training and reduce the workload of the physical therapist [51]. RAGT provides repetition of gait-like movements and these mechanisms of action could stimulate the motor area and sensory area of the cerebral hemisphere which has a positive influence on the central pattern generators at the spinal level; this process facilitates neuromuscular regulation, enhances the contraction and inhibition patterns of the lower limb muscles, improves proprioceptive reflexes, and promotes co-activation of ankle dorsiflexion and plantar flexors [52, 53, 54]. Via a combination of these mechanisms, the gait of the PD patients demonstrated notable improvement suggesting that RAGT is an effective form of physical therapy for the treatment of gait disorders [55].
This systematic review and meta-analysis have several limitations: (1) the majority of the included literature were small-sample trials; (2) this study principally reported the short-term efficacy of RAGT in PD patients, and thus its long-term follow-up efficacy in PD patients needs to be further proved; (3) there is no standardization for RAGT equipment, such as the Gait Trainer GT, exoskeleton, or Lokomat, and exercise prescriptions concerning the devices employed for RAGT, which may result in discrepancies in treatment outcomes. Therefore, more high-quality, large-sample, multi-center, and largely sampled RCTs are needed to further verify the impact of RAGT on motor function in PD patients.
RAGT has excellent potential for clinical application and is worthy of clinical promotion. The results of this study suggested that RAGT can improve balance, walking, and gait performance and significantly improve lower extremity function in PD patients. As a new physical therapy technique, RAGT can promote the activation of neuroplasticity through repetitive, high-intensity, and individualized walking training, which has been gradually applied to the clinical treatment of PD patients, relieving the work pressure of rehabilitation therapists while providing excellent clinical efficacy.
5.Conclusions
This systematic review and meta-analysis indicate that RAGT may be more effective than conventional rehabilitation treatments for improving balance function, walking function and gait disorders. Due to the certainty of the evidence being low to very low by GRADE more high-quality clinical studies with large sample sizes are needed to explore the long-term effects of RAGT on lower extremity motor dysfunction in PD patients. Additionally, this research supports that RAGT can be widely recommended as an addition to the current physical therapy model adopted for PD patients.
Author contributions
All authors contributed to the conception and design of the study. Material preparation, data collection, and analysis were performed by JZ, QC, SW, QX, LY and DZ. The manuscript was written and revised by XJ HL and HB. All authors read and approved the final manuscript.
Ethical approval
Not applicable.
Funding
The authors received no financial support for the research, authorship, and/or publication of this article.
Informed consent
Not applicable.
Supplementary data
The supplementary files are available to download from http://dx.doi.org/10.3233/BMR-220395.
Acknowledgments
None to report.
Conflict of interest
None of the authors have any competing interests to report.
References
[1] | Pajares M, A IR, Manda G, et al. Inflammation in Parkinson’s Disease: Mechanisms and herapeutic Implications. Cells. (2020) ; 9: (7): 1687. |
[2] | Simon DK, Tanner CM, Brundin P. Parkinson Disease Epidemiology, Pathology, Genetics, and Pathophysiology. Clinics in Geriatric Medicine. (2020) ; 36: (1): 1-12. |
[3] | Hallett PJ, Engelender S, Isacson O. Lipid and immune abnormalities causing age-dependent neurodegeneration and Parkinson’s disease. Journal of Neuroinflammation. (2019) ; 16: (1): 153. |
[4] | Ascherio A, Schwarzschild MA. The epidemiology of Parkinson’s disease: risk factors and prevention. The Lancet Neurology. (2016) ; 15: (12): 1257-72. |
[5] | de Lau LM, Breteler MM. Epidemiology of Parkinson’s disease. The Lancet Neurology. (2006) ; 5: (6): 525-35. |
[6] | Dorsey ER, Bloem BR. The Parkinson Pandemic-A Call to Action. JAMA Neurology. (2018) ; 75: (1): 9-10. |
[7] | Reich SG, Savitt JM. Parkinson’s Disease. The Medical Clinics of North America. (2019) ; 103: (2): 337-50. |
[8] | Hayes MT. Parkinson’s Disease and Parkinsonism. The American Journal of Medicine. (2019) ; 132: (7): 802-7. |
[9] | Raza C, Anjum R, Shakeel NUA. Parkinson’s disease: Mechanisms, translational models and management strategies. Life Sciences. (2019) ; 226: : 77-90. |
[10] | Mirelman A, Bonato P, Camicioli R, et al. Gait impairments in Parkinson’s disease. The Lancet Neurology. (2019) ; 18: (7): 697-708. |
[11] | Mizuno Y, Shimoda S, Origasa H. Long-term treatment of Parkinson’s disease with levodopa and other adjunctive drugs. Journal of Neural Transmission (Vienna, Austria: 1996). (2018) ; 125: (1): 35-43. |
[12] | Feng YS, Yang SD, Tan ZX, et al. The benefits and mechanisms of exercise training for Parkinson’s disease. Life Sciences. (2020) ; 245: : 117345. |
[13] | Xu X, Fu Z, Le W. Exercise and Parkinson’s disease. International Review of Neurobiology. (2019) ; 147: : 45-74. |
[14] | Hobbs B, Artemiadis P. A Review of Robot-Assisted Lower-Limb Stroke Therapy: Unexplored Paths and Future Directions in Gait Rehabilitation. Frontiers in Neurorobotics. (2020) ; 14: : 19. |
[15] | Okawara H, Tashiro S, Sawada T, et al. Neurorehabilitation using a voluntary driven exoskeletal robot improves trunk function in patients with chronic spinal cord injury: a single-arm study. Neural Regeneration Research. (2022) ; 17: (2): 427-32. |
[16] | Calafiore D, Negrini F, Tottoli N, et al. Efficacy of robotic exoskeleton for gait rehabilitation in patients with subacute stroke: a systematic review. European Journal of Physical and Rehabilitation Medicine. (2022) ; 58: (1): 1-8. |
[17] | Esquenazi A, Packel A. Robotic-assisted gait training and restoration. American Journal of Physical Medicine & Rehabilitation. (2012) ; 91: (11 Suppl 3): S217-27; quiz S28-31. |
[18] | Yun SJ, Lee HH, Lee WH, et al. Effect of robot-assisted gait training on gait automaticity in Parkinson disease: A prospective, open-label, single-arm, pilot study. Medicine. (2021) ; 100: (5): e24348. |
[19] | Picelli A, Capecci M, Filippetti M, et al. Effects of robot-assisted gait training on postural instability in Parkinson’s disease: a systematic review. European Journal of Physical and Rehabilitation Medicine. (2021) ; 57: (3): 472-7. |
[20] | Kim H, Kim E, Yun SJ, et al. Robot-assisted gait training with auditory and visual cues in Parkinson’s disease: A randomized controlled trial. Annals of Physical and Rehabilitation Medicine. (2022) ; 65: (3): 101620. |
[21] | Zampogna A, Mileti I, Martelli F, et al. Early balance impairment in Parkinson’s Disease: Evidence from Robot-assisted axial rotations. Clinical neurophysiology: Official Journal of the International Federation of Clinical Neurophysiology. (2021) ; 132: (10): 2422-30. |
[22] | Alwardat M, Etoom M, Al Dajah S, et al. Effectiveness of robot-assisted gait training on motor impairments in people with Parkinson’s disease: a systematic review and meta-analysis. International Journal of Rehabilitation Research Internationale Zeitschrift fur Rehabilitationsforschung Revue Internationale de Recherches de Readaptation. (2018) ; 41: (4): 287-96. |
[23] | Picelli A, Melotti C, Origano F, et al. Robot-assisted gait training is not superior to balance training for improving postural instability in patients with mild to moderate Parkinson’s disease: a single-blind randomized controlled trial. Clinical Rehabilitation. (2015) ; 29: (4): 339-47. |
[24] | Page MJ, Moher D, Bossuyt PM, et al. PRISMA 2020 explanation and elaboration: updated guidance and exemplars for reporting systematic reviews. BMJ (Clinical research ed). (2021) ; 372: : n160. |
[25] | Cumpston MS, McKenzie JE, Welch VA, et al. Strengthening systematic reviews in public health: guidance in the Cochrane Handbook for Systematic Reviews of Interventions, 2nd edition. Journal of public health (Oxford, England). (2022) ; 44: (4): e588-e592. |
[26] | Schünemann HJ, Oxman AD, Brozek J, et al. GRADE: assessing the quality of evidence for diagnostic recommendations. ACP Journal Club. (2008) ; 149: (6): 2. |
[27] | Carda S, Invernizzi M, Baricich A, et al. Robotic gait training is not superior to conventional treadmill training in parkinson disease: a single-blind randomized controlled trial. Neurorehabilitation and Neural Repair. (2012) ; 26: (9): 1027-34. |
[28] | Picelli A, Melotti C, Origano F, et al. Robot-assisted gait training in patients with Parkinson disease: a randomized controlled trial. Neurorehabilitation and Neural Repair. (2012) ; 26: (4): 353-61. |
[29] | Picelli A, Melotti C, Origano F, et al. Does robotic gait training improve balance in Parkinson’s disease? A randomized controlled trial. Parkinsonism & Related Disorders. (2012) ; 18: (8): 990-993. |
[30] | Picelli A, Melotti C, Origano F, et al. Robot-assisted gait training versus equal intensity treadmill training in patients with mild to moderate Parkinson’s disease: a randomized controlled trial. Parkinsonism & Related Disorders. (2013) ; 19: (6): 605-610. |
[31] | Sale P, De Pandis MF, Le Pera D, et al. Robot-assisted walking training for individuals with Parkinson’s disease: a pilot randomized controlled trial. BMC Neurology. (2013) ; 13: : 50. |
[32] | Galli M, Cimolin V, De Pandis MF, et al. Robot-assisted gait training versus treadmill training in patients with Parkinson’s disease: a kinematic evaluation with gait profile score. Functional Neurology. (2016) ; 31: (3): 163-170. |
[33] | Chen D, Su X. Efficacy of lower limb robot gait training on Parkinson ’s patients. Chinese Medical Device Information. (2016) ; 22: (23): 50-2+5. |
[34] | Furnari A, Calabrò RS, De Cola MC, et al. Robotic-assisted gait training in Parkinson’s disease: a three-month follow-up randomized clinical trial. The International Journal of Neuroscience. (2017) ; 127: (11): 996-1004. |
[35] | Xia M, Zhan ZT, Cai GE, et al. Three-dimensional gait analysis of patients with Parkinson ’s disease before and after automatic lower limb robot training. Chinese Journal of Neurology. (2018) ; 51: (7): 504-509. |
[36] | Capecci M, Pournajaf S, Galafate D, et al. Clinical effects of robot-assisted gait training and treadmill training for Parkinson’s disease. A Randomized Controlled Trial. Annals of Physical and Rehabilitation Medicine. (2019) ; 62: (5): 303-312. |
[37] | Ma HL, He Q, Wu ZJ, et al. Effect of lower limb rehabilitation robot combined with audio-visual training on gait of Parkinson’s disease patients. Chinese Journal of Community Physicians. (2020) ; 36: (35): 158-159. |
[38] | Kawashima N, Hasegawa K, Iijima M, et al. Efficacy of Wearable Device Gait Training on Parkinson’s Disease: A Randomized Controlled Open-label Pilot Study. Internal Medicine (Tokyo, Japan). (2022) ; 61: (17): 2573-80. |
[39] | Ding W, Liang C, Su M. Effect of lower limb rehabilitation robot on balance function in patients with Parkinson ’s disease. Chinese Journal of Rehabilitation Medicine. (2022) ; 37: (04): 494-500. |
[40] | Lin ZL, Fu XW, Yu CW, et al. Effect of Flexbot lower extremity rehabilitation robot combined with virtual reality training on balance function and walking ability of patients with Parkinson’s disease. Zhejiang Medical Journal. (2021) ; 43: (04): 405-408+413. |
[41] | Spina S, Facciorusso S, Cinone N, et al. Effectiveness of robotic balance training on postural instability in patients with mild Parkinson’s disease: A pilot, single blind, randomized controlled trial. Journal of Rehabilitation Medicine. (2021) ; 53: (2): jrm00154. |
[42] | Intzandt B, Beck EN, Silveira CRA. The effects of exercise on cognition and gait in Parkinson’s disease: A scoping review. Neuroscience and Biobehavioral Reviews. (2018) ; 95: : 136-69. |
[43] | Sierra MS, Garcia AD, Otálora S, et al. Assessment of a Robotic Walker in Older Adults With Parkinson’s Disease in Daily Living Activities. Frontiers in Neurorobotics. (2021) ; 15: : 742281. |
[44] | Debû B, De Oliveira Godeiro C, Lino JC, et al. Managing Gait, Balance, and Posture in Parkinson’s Disease. Current Neurology and Neuroscience Reports. (2018) ; 18: (5): 23. |
[45] | Lima CA, Ricci NA, Nogueira EC, et al. The Berg Balance Scale as a clinical screening tool to predict fall risk in older adults: a systematic review. Physiotherapy. (2018) ; 104: (4): 383-94. |
[46] | Opara J, Małecki A, Małecka E, et al. Motor assessment in Parkinson‘s disease. Annals of Agricultural and Environmental Medicine: AAEM. (2017) ; 24: (3): 411-5. |
[47] | Nieuwboer A, Rochester L, Müncks L, et al. Motor learning in Parkinson’s disease: limitations and potential for rehabilitation. Parkinsonism & Related Disorders. (2009) ; 15: (Suppl 3): S53-8. |
[48] | Graff K, Szczerbik E, Kalinowska M, et al. Using the TUG Test for the Functional Assessment of Patients with Selected Disorders. International Journal of Environmental Research And Public Health. (2022) ; 19: (8): 4602. |
[49] | Nightingale CJ, Mitchell SN, Butterfield SA. Validation of the Timed Up and Go Test for Assessing Balance Variables in Adults Aged 65 and Older. Journal of Aging and Physical Activity. (2019) ; 27: (2): 230-3. |
[50] | Martínez-Martín P, Rodríguez-Blázquez C, Mario A, et al. Parkinson’s disease severity levels and MDS-Unified Parkinson’s Disease Rating Scale. Parkinsonism & Related Disorders. (2015) ; 21: (1): 50-4. |
[51] | Takakusaki K. Neurophysiology of gait: from the spinal cord to the frontal lobe. Movement Disorders: Official Journal of the Movement Disorder Society. (2013) ; 28: (11): 1483-91. |
[52] | Toole T, Maitland CG, Warren E, et al. The effects of loading and unloading treadmill walking on balance, gait, fall risk, and daily function in Parkinsonism. Neuro Rehabilitation. (2005) ; 20: (4): 307-22. |
[53] | Bello O, Marquez G, Fernandez-Del-Olmo M. Effect of Treadmill Walking on Leg Muscle Activation in Parkinson’s Disease. Rejuvenation Research. (2019) ; 22: (1): 71-8. |
[54] | Guo Y, Yang J, Liu Y, et al. Detection and assessment of Parkinson’s disease based on gait analysis: A survey. Frontiers in Aging Neuroscience. (2022) ; 14: : 916971. |
[55] | Carmignano SM, Fundaro C, Bonaiuti D, et al. Robot-assisted gait training in patients with Parkinson’s disease: Implications for clinical practice. A Systematic Review. Neuro Rehabilitation. (2022) ; 51: (4): 649-663. |