Effect of Genetic Risk on the Relationship Between rs-fMRI Complexity and Tau and Amyloid PET in Alzheimer’s Disease
Abstract
Reduced functional magnetic resonance imaging (fMRI)-complexity in Alzheimer’s disease (AD) progression has been demonstrated and found to be associated with tauopathy and cognition. However, association of fMRI-complexity with amyloid and influence of genetic risk (APOE ɛ4) remain unknown. Here we investigate the association between fMRI-complexity, tau-PET, and amyloid-PET as well as influence of APOE genotype using multivariate generalized linear models. We show that fMRI-complexity has a strong association with tau but not amyloid deposition and that the presence of an APOE ɛ4 allele enhances this effect. Thus fMRI-complexity provides a surrogate marker of impaired brain functionality in AD progression.
INTRODUCTION
Nonlinear statistical analyses of neural signals from resting state functional magnetic resonance imaging (rs-fMRI) that characterize the signal complexity within a brain area have been proposed as measures for the information processing capacity of brain areas and networks,1–4 or indices of pathological brain function.5–7 One of the most commonly used metrics is SampleEntropy (SampEn)8 and its extension to multiple temporal frequencies, MultiScale Entropy (MSE).9 SampEN and MSE both capture the randomness and predictability of a stochastic process. SampEn is calculated as the negative log of the likelihood that a pattern match at a specific length can be observed also at a length plus one. A match thereby is defined by a specific sensitivity threshold. MSE is an extension of SampEn to multiple frequency scales, which are formed by coarse sampling of the original time series to generate new time series with reduced frequency. Such fMRI-complexity analyses have demonstrated progressive decline in complexity from cognitively normal subjects (CN) to patients with mild cognitive impairment (MCI) and patients with Alzheimer’s disease (AD).10–12 fMRI-complexity is also associated with overall cognitive function and hippocampal fMRI-complexity specifically with memory deficits.13,14 In sum, complexity was demonstrated to be related to worsening cognitive function with AD progression.
Amyloid has been shown to vary across as well as within diagnostic groups. Amyloid load can also be relatively high in individuals without cognitive impairments. Accordingly, recent hypotheses refer to amyloid as a prerequisite for AD but tau as the trigger that leads to cognitive decline as a result of neurotoxicity and hence loss of synapses, dendrites and ultimately neurons.15,16 Furthermore, it has been shown that tau deposition is related to cognitive impairments in different domains in a region-specific manner and that these relations are only weakly related to amyloid burden.17 Hence, there is increasing evidence for the relevance of tau pathology as the more critical pathophysiological index of neurodegeneration and subsequent cognitive decline than amyloid,18 and AD therapy targeting tau being studied as potential interventions.19 Since fMRI-complexity captures changes related to alterations in neuronal signaling, which is the basis for brain function, and these alterations in neuronal signaling and decline in cognition are strongly associated with tauopathy, it is reasonable to hypothesize that fMRI-complexity has a closer association to tau than amyloid. In a recent paper we showed that fMRI complexity in precuneus and medial temporal lobe is negatively associated with tau-PET uptake and that the relation between fMRI-complexity and cognitive function is mediated by tau deposition.20 However, the effects of amyloid deposition or genetic risk (APOE ɛ4) on the relation between fMRI-complexity and tau remains unknown.
APOE ɛ4 has strong links to amyloid pathology and APOE ɛ4 is generally associated with greater risk of dementia.21 Recently however, APOE ɛ4 has also been demonstrated as a critical regulator of tau pathology independent of amyloid in human18 and animal studies.22 It was demonstrated that the APOE ɛ4 allele is associated with more severe tau pathogenesis and tau related neuronal degeneration independent of amyloid pathology, particularly in medial temporal lobe.23 APOE ɛ4 influences early regional tau PET burden, above and beyond effects related to cross-sectional amyloid PET burden.24–26 Carriers of APOE ɛ4 also show accelerated amyloid-related tau spreading even at lower amyloid levels.27 Finally, animal models demonstrate that the APOE ɛ4 genotype affects tau pathogenesis, neuroinflammation, and tau-mediated neurodegeneration independently of amyloid-β pathology.22 Therefore, we hypothesize that the association between fMRI-complexity and tau-PET in areas of early AD pathology (posterior cingulate, precuneus, (para)hippocampal gyrus, and entorhinal cortex) is significantly modified by APOE status.
In this study, we investigate the association between fMRI-complexity, tau-PET and amyloid-PET as well as influence of APOE status.
METHODS
Data used in the preparation of this article were obtained from the Alzheimer’s Disease Neuroimaging Initiative (ADNI) database (http://adni.loni.usc.edu). From the ADNI phase 3 (ADNI3), we identified participants that had amyloid PET (florbetapir, 18F-AV-45), tau PET (flortaucipir, 18F-AV-1451), an fMRI resting state scan and an anatomical scan (MPRAGE) at the same timepoint. We collected the tabulated SUVR values for amyloid and tau from the ADNI database for ROIs within the FreeSurfer Desikan-Killiany atlas. We also downloaded the ADNIMERGE table, containing demographic information (age, sex, number of APOE ɛ4 alleles), and diagnostic labels (CN/MCI/AD). Raw structural and functional MRI data was downloaded for each identified subject. The rs-fMRI data were preprocessed using Matlab and SPM12, and included motion realignment, regression of white matter, cerebrospinal fluid, and head motion related signal fluctuations, coregistration to anatomical scan and normalization into MNI standard space. FMRI-complexity was then calculated by Multiscale Sample Entropy (MSE) (LOFT complexity toolbox)28 with pattern sensitivity threshold r = 0.5, pattern matching length m = 2 and temporal scale 6 (for low signal fluctuations at 0.05 Hz). MSE maps were then parcellated into regions of interest using the same FreeSurfer Desikan-Killiany atlas resulting in ROI specific average MSE values. Finally, we defined a set of bilateral ROIs associated with early AD pathology: posterior cingulate, precuneus, (para)hippocampal gyrus, and entorhinal cortex. For each ROI we thus had indices for amyloid, tau and fMRI-complexity (see Supplementary Figure 1 for example of ROI specific values for diagnostic groups). A multivariate generalized linear model was used to investigate the adjusted independent effects of amyloid and tau in relation to fMRI-complexity (Model1). Furthermore, we were interested whether such effects were modified by genetic risk, e.g., APOE ɛ4 status (carriers versus non-carriers). These effects were tested using the interaction terms in the multivariate model. Age and gender were controlled for in all models. We also tested a second model that accounted for diagnostic group as there likely is an association between disease severity and physiological markers of the disease (Model2). Data normality was visualized by histogram. Model integrity was assessed by residual plots. SAS 9.4 was used for statistical analyses. The global α level was set to 0.05, with Bonferroni correction for multiple comparisons (number of ROIs tested). Thus, the adjusted α for overall association and interaction test is 0.0125 and for strata specific (APOE ɛ4 carrier or non-carrier) effect is 0.006.
RESULTS
The final cohort consisted of 127 subjects (Table 1). For Model1 (not including information for diagnostic group), we observed statistically significant negative associations between complexity and tau in parahippocampal gyrus (–0.07 95% CI: (–0.13, –0.02); p = 0.01) and precuneus (–0.05 95% CI: (–0.08, –0.01); p = 0.01) with a trend in posterior cingulate cortex (–0.06 95% CI: (–0.12, 0); p = 0.07) (Table 2A). No statistically significant relationships with amyloid were found. APOE ɛ4 status was found as a statistically significant effect modifier on the association between tau and complexity (p = 0.006) in posterior cingulate gyrus (Table 2B). This strata specific effect showed that APOE ɛ4 carriers have a statistically significantly stronger negative association between tau and complexity of –0.26 95% CI: (–0.42, –0.1); p = 0.002 compared to non-carriers with the effect of 0.09 95% CI: (–0.09, 0.28), p: 0.33. A similar trend was found in precuneus with effect in APOE ɛ4 carriers of –0.14 95% CI: (–0.24, –0.05); p = 0.004 and non-carriers of –0.02 95% CI: (–0.13, 0.08), p: 0.68 with an interaction test p = 0.1. For amyloid no statistically significant interaction effect was found nor was there a main effect (Table 2B). For Model2 that accounts for diagnostic group, the main effects between fMRI-complexity and tau disappeared, but the interaction effect with APOE ɛ4 in posterior cingulate cortex remained. Since the data were slightly skewed to the left, we conducted sensitivity analysis with cubed data transformation, which resulted in a close-to-perfect bell shape distribution and no extreme outlier was observed in Cook’s distance plot. All statistical significance remained after data transformation.
Table 1
Cohort Demographics
CN | MCI | AD | |
N | 79 | 42 | 6 |
age (mean/SD) | 71.1/5.6 | 68.6/7.8 | 69.7/10.0 |
gender (F/M) | 47/32 | 17/25 | 2/4 |
APOE ɛ4 (0/1/2 alleles) | 54/20/5 | 24/16/2 | 3/1/2 |
Table 2
Test statistics of Multivariate Generalized Linear Model. A, B) Main and interaction effect for multivariate model without accounting for diagnostic groups. C, D) Main and interaction effects for multivariate model including diagnostic group. The adjusted α for overall association and interaction test is 0.0125 and for strata specific effect (by APOE ɛ4 carries or non-carries) is 0.006. Results with statistical significance after correction for multiple testing are highlighted in bold
A) Overall | Amyloid | Tau | |||||
test statistic | p | test statistic | p | ||||
Entorhinal | 0.01 95% CI: (–0.02, 0.03) | 0.64 | –0.01 95% CI: (–0.05, 0.03) | 0.6 | |||
Parahippocampus | 0.01 95% CI: (–0.01, 0.03) | 0.27 | –0.07 95% CI: (–0.13, –0.02) | 0.01 | |||
Posterior Cingulate | 0.01 95% CI: (–0.01, 0.02) | 0.6 | –0.06 95% CI: (–0.12, 0) | 0.07 | |||
Precuneus | 0.00 95% CI: (–0.02, 0.01) | 0.83 | –0.05 95% CI: (–0.08, –0.01) | 0.01 | |||
B) APOE ɛ4 | Interaction Test | Amyloid | Tau | ||||
carrier | Amyloid p | Tau p | test statistic | p | test statistic | p | |
Entorhinal | no | 0.75 | 0.17 | 0.01 95% CI: (–0.05, 0.06) | 0.85 | –0.07 95% CI: (–0.19, 0.04) | 0.21 |
yes | 0.02 95% CI: (–0.05, 0.09) | 0.57 | 0.04 95% CI: (–0.08, 0.16) | 0.5 | |||
Parahippocampus | no | 0.02 | 0.64 | –0.02 95% CI: (–0.07, 0.04) | 0.54 | –0.19 95% CI: (–0.35, –0.03) | 0.02 |
yes | 0.08 95% CI: (0.02, 0.15) | 0.01 | –0.13 95% CI: (–0.3, 0.03) | 0.12 | |||
Posterior Cingulate | no | 0.05 | <0.006 | –0.03 95% CI: (–0.08, 0.02) | 0.29 | 0.09 95% CI: (–0.09, 0.28) | 0.33 |
yes | 0.05 95% CI: (–0.01, 0.11) | 0.09 | –0.26 95% CI: (–0.42, –0.1) | <0.002 | |||
Precuneus | no | 0.23 | 0.1 | –0.02 95% CI: (–0.07, 0.02) | 0.28 | –0.02 95% CI: (–0.13, 0.08) | 0.68 |
yes | 0.02 95% CI: (–0.03, 0.07) | 0.53 | –0.14 95% CI: (–0.24, –0.05) | <0.004 | |||
C) Overall (with DDX) | Amyloid | Tau | |||||
test statistic | p | test statistic | p | ||||
Entorhinal | 0.00 95% CI: (–0.02, 0.03) | 0.75 | 0.01 95% CI: (–0.04, 0.05) | 0.7 | |||
Parahippocampus | 0.01 95% CI: (–0.01, 0.03) | 0.22 | –0.03 95% CI: (–0.09, 0.03) | 0.41 | |||
Posterior Cingulate | 0.01 95% CI: (–0.01, 0.03) | 0.35 | –0.02 95% CI: (–0.08, 0.04) | 0.51 | |||
Precuneus | 0.00 95% CI: (–0.01, 0.02) | 0.83 | 0.00 95% CI: (–0.03, 0.04) | 0.89 | |||
D) APOE ɛ4 (with DDX) | Interaction Test | Amyloid | Tau | ||||
carrier | Amyloid p | Tau p | test statistic | p | test statistic | p | |
Entorhinal | no | 0.9 | 0.24 | 0.00 95% CI: (–0.05, 0.06) | 0.88 | –0.04 95% CI: (–0.15, 0.08) | 0.56 |
yes | 0.01 95% CI: (–0.06, 0.08) | 0.78 | 0.07 95% CI: (–0.06, 0.2) | 0.31 | |||
Parahippocampus | no | 0.04 | 0.55 | –0.01 95% CI: (–0.06, 0.04) | 0.72 | –0.11 95% CI: (–0.27, 0.05) | 0.19 |
yes | 0.08 95% CI: (0.01, 0.14) | 0.02 | –0.04 95% CI: (–0,22, 0.14) | 0.67 | |||
Posterior Cingulate | no | 0.05 | <0.01 | –0.02 95% CI: (–0.07, 0.03) | 0.44 | 0.13 95% CI: (–0.05, 0.32) | 0.15 |
yes | 0.06 95% CI: (0.00, 0.11) | 0.05 | –0.18 95% CI: (–0.35, –0.02) | 0.03 | |||
Precuneus | no | 0.29 | 0.09 | –0.06 95% CI: (–0.06, 0.03) | 0.45 | 0.07 95% CI: (–0.04, 0.17) | 0.21 |
yes | –0.03 95% CI: (–0.03, 0.06) | 0.47 | –0.04 95% CI: (–0.14, 0.05) | 0.36 |
Supplementary Figure 1 exemplifies amyloid, tau and fMRI-complexity values for each diagnostic group in precuneus and parahippocampal gyrus.
Fig. 1
Visualization of A) main effect on the association between fMRI-complexity and tau-PET SUVR and B) APOE ɛ4 presence enhanced this association. There were no associations with amyloid-PET SUVR.
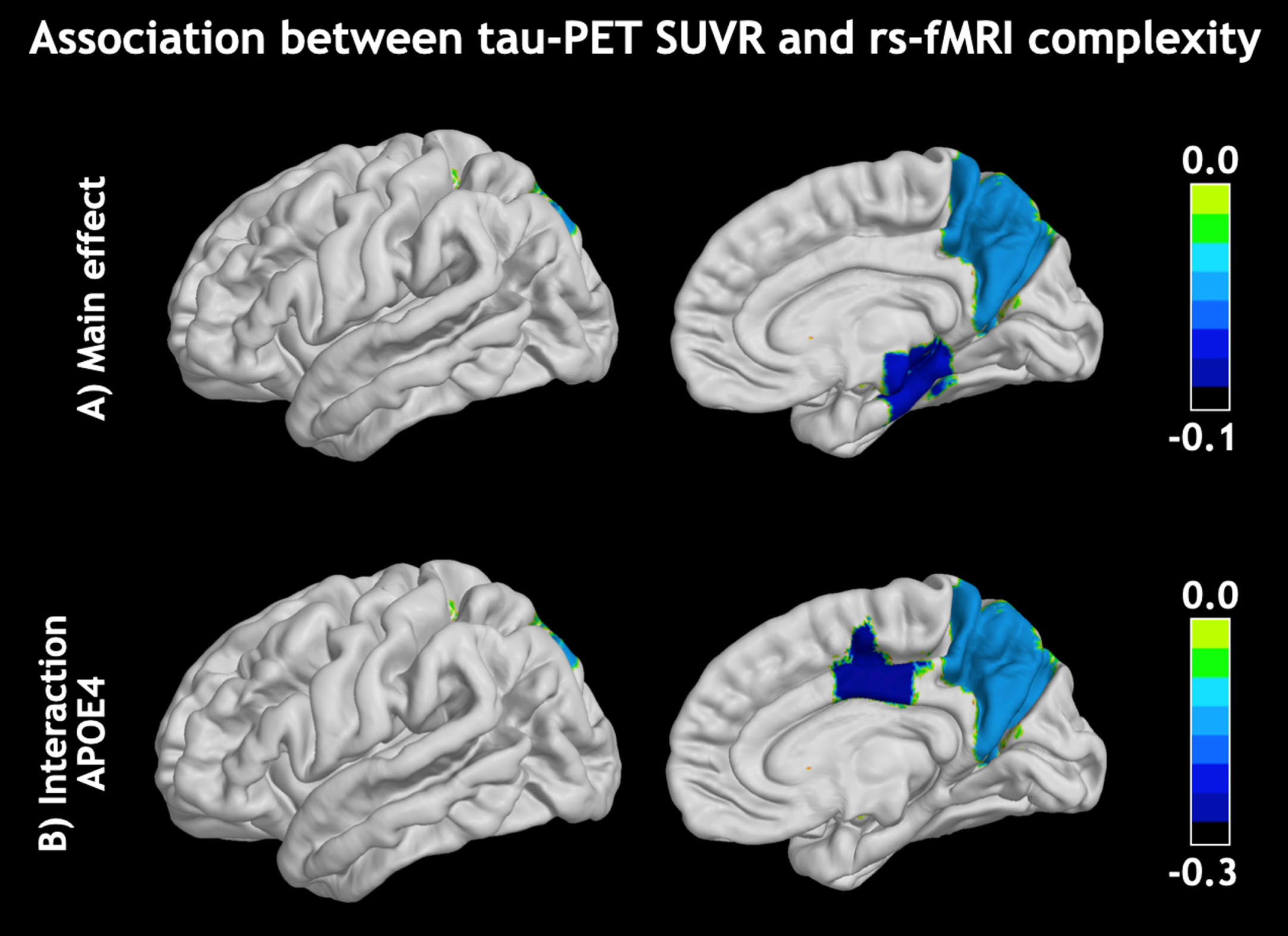
DISCUSSION
The observations of a negative association between fMRI-complexity and tau-PET in medial temporal and parietal cortices from this study confirmed previously reported statistically significant inverse relationships in these areas. While the cohort was largely overlapping with the ADNI cohort in our previous paper, here we used a different cortical parcellation and thus present evidence that the reported relationship is robust to atlas selection and small alterations in the subject sample. Furthermore, while fMRI complexity is robustly found to be associated with tau, we could for the first time demonstrate that this is independent of amyloid, and that fMRI-complexity shows no association with amyloid deposition in areas affected early in the progression of AD. Hence, fMRI-complexity appears to be indicative of dysfunction of neuronal signaling in the presence of tauopathy. This aligns with the notion that tau-PET imaging correlates more closely with neuronal injury and associated cognitive decline. It is conceivable that fMRI-complexity assesses dynamic fluctuations of rs-fMRI signals that possess clinically meaningful information and is sensitive to alterations in these dynamics in the process of tau related synaptic neurodegeneration.29,30 In addition to the general effect we observed, we further found that the increased risk conferred by the APOE ɛ4 allele had a strong modifying effect leading to a stronger negative relationship between fMRI-complexity and tau-PET values in posterior cingulate cortex (PCC) and precuneus. The PCC has been discussed as an early epicenter of AD and that changes in PCC gray matter31 and glucose metabolism32 predicts AD progression. Additionally, postmortem tissue analysis in PCC showed reduced synaptic numbers already during prodromal stages of AD.33 Following this line of thought, we propose that fMRI-complexity might be a novel marker to assess tau-related alterations in neuronal signaling and subsequent cognitive deficits in the progression of AD. This hypothesis also considers why there were no association or interactions with amyloid, since while the presence of amyloid increases risk for cognitive decline it is the occurrence of tau that leads to synaptic deficits, neurodegeneration and consequently cognitive decline. Furthermore, amyloid can largely vary across diagnostic groups since even with high amyloid load there might not be severe cognitive impairment. However, longitudinal studies will be needed to investigate whether subjects with higher genetic risk and/or amyloid burden show faster decrease of fMRI-complexity which the current cross-sectional observations indicate. Our data also showed that amyloid is more variable and a less robust predictor of diagnosis than tau or fMRI-complexity (Supplementary Figure 1).
When including information about diagnostic group in the statistical model, the main effects disappeared which suggests a complete mediation effect of diagnostic group on the relation between fMRI-complexity and tau. This finding is not surprising, since with increased load of amyloid and specifically tau there is likely higher cognitive impairment and thus more severe diagnosis. We believe that the initial model is important as it compares purely physiological markers which all are capturing different aspects of AD pathology that are used to classify diagnostic groups: fMRI-complexity, amyloid and tau are all to some degree associated with diagnostic group.
To conclude, we observed a significant inverse relationship between fMRI-complexity and tau-PET markers, specifically in medial temporal lobe and precuneus and that this relationship was modified by genetic risk. Therefore, fMRI-complexity might provide a surrogate index of the information processing capacity of regional neuron populations and is sensitive to tau-related neuronal injury and cognitive decline in the course of AD pathogenesis.
AUTHOR CONTRIBUTIONS
Kay Jann (Conceptualization; Data curation; Formal analysis; Funding acquisition; Software; Visualization; Writing – original draft); Steven Cen (Formal analysis; Writing – review & editing); Mariella Santos (Data curation; Formal analysis); Leon Aksman (Data curation; Writing – review & editing); Dilmini Wijesinghe (Data curation; Software; Writing – review & editing); Ru Zhang (Data curation; Formal analysis; Writing – review & editing); Kirsten Lynch (Data curation; Visualization; Writing – review & editing); John M. Ringman (Supervision; Writing – review & editing); Danny J. Wang (Conceptualization; Funding acquisition; Supervision; Writing – review & editing).
ACKNOWLEDGMENTS
Data collection and sharing for this project was funded by the Alzheimer’s Disease Neuroimaging Initiative (ADNI) (National Institutes of Health Grant U01 AG024904) and DOD ADNI (Department of Defense award number W81XWH-12-2-0012). ADNI is funded by the National Institute on Aging, the National Institute of Biomedical Imaging and Bioengineering, and through generous contributions from the following: AbbVie, Alzheimer’s Association; Alzheimer’s Drug Discovery Foundation; Araclon Biotech; BioClinica, Inc.; Biogen; Bristol-Myers Squibb Company; CereSpir, Inc.; Cogstate; Eisai Inc.; Elan Pharmaceuticals, Inc.; Eli Lilly and Company; EuroImmun; F. Hoffmann-La Roche Ltd and its affiliated company Genentech, Inc.; Fujirebio; GE Healthcare; IXICO Ltd.; Janssen Alzheimer Immunotherapy Research & Development, LLC.; Johnson & Johnson Pharmaceutical Research & Development LLC.; Lumosity; Lundbeck; Merck & Co., Inc.; Meso Scale Diagnostics, LLC.; NeuroRx Research; Neurotrack Technologies; Novartis Pharmaceuticals Corporation; Pfizer Inc.; Piramal Imaging; Servier; Takeda Pharmaceutical Company; and Transition Therapeutics. The Canadian Institutes of Health Research is providing funds to support ADNI clinical sites in Canada. Private sector contributions are facilitated by the Foundation for the National Institutes of Health (http://www.fnih.org). The grantee organization is the Northern California Institute for Research and Education, and the study is coordinated by the Alzheimer’s Therapeutic Research Institute at the University of Southern California. ADNI data are disseminated by the Laboratory for Neuro Imaging at the University of Southern California.
FUNDING
This project was funded by NIA R01AG066711 (Jann/Wang).
CONFLICT OF INTEREST
Kay Jann is an Editorial Board Member of this journal but was not involved in the peer-review process of this article nor had access to any information regarding its peer-review.
All other authors have no conflict of interest to report.
DATA AVAILABILITY
Data is publicly available and managed by the ADNI consortium.
SUPPLEMENTARY MATERIAL
[1] The supplementary material is available in the electronic version of this article: https://dx.doi.org/10.3233/JAD-240459.
REFERENCES
1. | McDonough IM and Nashiro K. Network complexity as a measure of information processing across resting-state networks: Evidence from the Human Connectome Project. Front Hum Neurosci (2014) ; 8: : 409. |
2. | Smith RX , Yan L and Wang DJ. Multiple time scale complexity analysis of resting state FMRI. Brain Imaging Behav (2014) ; 8: : 284–291. |
3. | Wang DJJ , Jann K , Fan C , et al. Neurophysiological basis of multi-scale entropy of brain complexity and its relationship with functional connectivity. Front Neurosci (2018) ; 12: : 352. |
4. | Wang Z , Li Y , Childress AR , et al. Brain entropy mapping using fMRI. PLoS One (2014) ; 9: : e89948. |
5. | Fernández A , Gómez C , Hornero R , et al. Complexity and schizophrenia. Prog Neuropsychopharmacol Biol Psychiatry (2013) ; 45: : 267–276. |
6. | Sun J , Wang B , Niu Y , et al. Complexity analysis of EEG, MEG, and fMRI in mild cognitive impairment and Alzheimer’s disease: A Review. Entropy (Basel) (2020) ; 22: : 239. |
7. | Takahashi T . Complexity of spontaneous brain activity in mental disorders. Prog Neuropsychopharmacol Biol Psychiatry (2013) ; 45: : 258–266. |
8. | Richman JS and Moorman JR. Physiological time-series analysis using approximate entropy and sample entropy. Am J Physiol Heart Circ Physiol (2000) ; 278: : H2039–H2049. |
9. | Costa M , Goldberger AL and Peng CK. Multiscale entropy analysis of complex physiologic time series. Phys Rev Lett (2002) ; 89: : 068102. |
10. | Zheng H , Onoda K , Nagai A , et al. Reduced dynamic complexity of BOLD signals differentiates mild cognitive impairment from normal aging. Front Aging Neurosci (2020) ; 12: : 90. |
11. | Wang Z and Alzheimer’s Disease Neuroimaging Initiative. Brain entropy mapping in healthy aging and Alzheimer’s disease. Front Aging Neurosci (2020) ; 12: : 596122. |
12. | Niu Y , Wang B , Zhou M , et al. Dynamic complexity of spontaneous BOLD activity in Alzheimer’s disease and mild cognitive impairment using multiscale entropy analysis. Front Neurosci (2018) ; 12: : 677. |
13. | Grieder M , Wang DJJ , Dierks T , et al. Default mode network complexity and cognitive decline in mild Alzheimer’s disease. Front Neurosci (2018) ; 12: : 770. |
14. | Liu CY , Krishnan AP , Yan L , et al. Complexity and synchronicity of resting state blood oxygenation level-dependent (BOLD) functional MRI in normal aging and cognitive decline. J Magn Reson Imaging (2013) ; 38: : 36–45. |
15. | Wu M , Zhang M , Yin X , et al. The role of pathological tau in synaptic dysfunction in Alzheimer’s diseases. Transl Neurodegener (2021) ; 10: : 45. |
16. | Bloom GS . Amyloid-β and tau: The trigger and bullet in Alzheimer disease pathogenesis. JAMA Neurol (2014) ; 71: : 505–508. |
17. | Bejanin A , Schonhaut DR , La Joie R , et al. Tau pathology and neurodegeneration contribute to cognitive impairment in Alzheimer’s disease. Brain (2017) ; 140: : 3286–3300. |
18. | van der Kant R , Goldstein LSB and Ossenkoppele R. Amyloid-β-independent regulators of tau pathology in Alzheimer disease. Nat Rev Neurosci (2020) ; 21: : 21–35. |
19. | Giacobini E and Gold G. Alzheimer disease therapy–moving from amyloid-β to tau. Nat Rev Neurol (2013) ; 9: : 677–686. |
20. | Jann K , Boudreau J , Albrecht D , et al. FMRI complexity correlates with tau-PET and cognitive decline in late-onset and autosomal dominant Alzheimer’s disease. J Alzheimers Dis (2023) ; 95: : 437–451. |
21. | Gharbi-Meliani A , Dugravot A , Sabia S , et al. The association of APOE ɛ4 with cognitive function over the adult life course and incidence of dementia: 20 years follow-up of the Whitehall II study. Alzheimers Res Ther (2021) ; 13: : 5. |
22. | Shi Y , Yamada K , Liddelow SA , et al. ApoE4 markedly exacerbates tau-mediated neurodegeneration in a mouse model of tauopathy. Nature (2017) ; 549: : 523–527. |
23. | La Joie R , Visani AV , Lesman-Segev OH , et al. Association of APOE4 and clinical variability in Alzheimer disease with the pattern of tau- and amyloid-PET. Neurology (2021) ; 96: : e650–e661. |
24. | Young CB , Johns E , Kennedy G , et al. APOE effects on regional tau in preclinical Alzheimer’s disease. Mol Neurodegener (2023) ; 18: : 1. |
25. | Therriault J , Benedet AL , Pascoal TA , et al. Association of apolipoprotein E ɛ4 with medial temporal tau independent of amyloid-β. JAMA Neurol (2020) ; 77: : 470–479. |
26. | Baek MS , Cho H , Lee HS , et al. Effect of APOE ɛ4 genotype on amyloid-β and tau accumulation in Alzheimer’s disease. Alzheimers Res Ther (2020) ; 12: : 140. |
27. | Steward A , Biel D , Dewenter A , et al. ApoE4 and connectivity-mediated spreading of tau pathology at lower amyloid levels. JAMA Neurol (2023) ; 80: : 1295–1306. |
28. | Kay J . Complexity Toolbox 2.0, https://www.github.com/kayjann/complexity ((2022) ). |
29. | Pooler AM , Noble W and Hanger DP. A role for tau at the synapse in Alzheimer’s disease pathogenesis. Neuropharmacology (2014) ; 76: (Pt A): 1–8. |
30. | Tracy TE and Gan L. Tau-mediated synaptic and neuronal dysfunction in neurodegenerative disease. Curr Opin Neurobiol (2018) ; 51: : 134–138. |
31. | Lee PL , Chou KH , Chung CP , et al. Posterior cingulate cortex network predicts Alzheimer’s disease progression. Front Aging Neurosci (2020) ; 12: : 608667. |
32. | Zhang Q , Fan C , Wang L , et al. Glucose metabolism in posterior cingulate cortex has supplementary value to predict the progression of cognitively unimpaired to dementia due to Alzheimer’s disease: An exploratory study of. Geroscience (2024) ; 46: : 1407–1420. |
33. | Scheff SW , Price DA , Ansari MA , et al. Synaptic change in the posterior cingulate gyrus in the progression of Alzheimer’s disease. J Alzheimers Dis (2015) ; 43: : 1073–1090. |