Amyloid-β Deposition Predicts Grocery Shopping Performance in Older Adults Without Cognitive Impairment
Abstract
Background:
A screening tool sensitive to Alzheimer’s disease (AD) risk factors, such as amyloid-β (Aβ) deposition, and subtle cognitive changes, best elicited by complex everyday tasks, is needed.
Objective:
To determine if grocery shopping performance could differentiate older adults at elevated risk of developing AD (OAer), older adults at low risk of developing AD (OAlr), and young adults (YA), and if amount of Aβ deposition could predict grocery shopping performance in older adults (OA).
Methods:
Twenty-one OAer (78±5 years), 33 OAlr (78±5 years), and 28 YA (31±3 years) performed four grocery shopping trials, with the best and worst performances analyzed. Measures included trial time, number of correct items, number of grocery note fixations, and number of fixations and percentage of time fixating on the correct shelving unit, correct brand, and correct shelf. Linear mixed effects models compared measures by performance rank (best, worst) and group (OAer, OAlr, YA), and estimated the effect of Aβ deposition on measures in OA.
Results:
Relative to their best performance, OAer and OAlr exhibited more correct shelving unit fixations and correct brand fixations during their worst performance, while YA did not. Within OA’s worst performance, greater Aβ deposition was associated with a smaller percentage of time fixating on the correct shelving unit, correct shelf, and correct brand. Within OA, greater Aβ deposition was associated with more grocery note fixations.
Conclusions:
OA with elevated Aβ deposition may exhibit subtle working memory impairments and less efficient visual search strategies while performing a cognitively demanding everyday task.
INTRODUCTION
Alzheimer’s disease (AD) results in a progressive loss of ability to perform everyday tasks [1] that leads to a loss of independence that is both costly and feared [2, 3]. Amyloid-β (Aβ), currently considered the primary pathological hallmark of Alzheimer’s disease (AD), accumulates decades before clinically detectable cognitive symptoms arise [4, 5]. However, current diagnostic methods are limited and often do not detect the disease until pathology is advanced [6–8]. New screening methods that can identify elevated risk and likelihood of developing AD are needed to facilitate early treatment and prevention of cognitive and functional impairments arising from AD.
During the asymptomatic (i.e., preclinical) phase of AD, Aβ deposition can occur up to 20 years before cognitive symptoms are detectable [9]. The cognitive functions often used to diagnose AD, including global cognition and self-reported performance of cognitively demanding, complex everyday tasks, such as using transportation and handling personal finances, do not typically decline until 6-7 years before diagnosis of dementia [10]. In contrast, subtle declines affecting executive function and information processing speed can occur 16–17 years before diagnosis of dementia with an etiology of AD, and changes in gait speed and variability can be observed up to 10 years before detected cognitive decline [10–16]. The substantial delay between the onset of Aβ deposition and detectable cognitive and behavioral symptoms [10, 17] highlights a critical need for objective screening tools that are sensitive to the presence of Aβ deposition and subtle changes in cognitive functions to enable intervention before significant impairments become manifest.
Measures obtained from eye movements during performance of a visually-demanding and complex everyday task may be particularly sensitive to subtle cognitive and perceptual deficits because eye movements are closely linked to cognition [18], and visuospatial processing may be impacted in those with preclinical AD 10 years before a diagnosis of dementia [19]. Fixation duration can indicate attentional filtering, working memory load, and when information processing becomes more effortful [18], as well as provide information about strategies that individuals utilize to solve problems [20]. Notably, individuals with dementia due to AD exhibit shorter gaze durations than healthy older adults, especially during the encoding portion of a visuospatial short-term memory task, suggesting that those with AD may exhibit visuospatial working memory performance deficits due to poor encoding mechanisms [21]. Additionally, performance of an allocentric spatial processing task, which relies on intact encoding of information about the location of objects in relation to other objects external to the self, is correlated with risk of developing dementia in healthy adults [22]. Encoding may be impacted in those with AD because of impaired feature binding [23], which involves integrating characteristics, such as shape, size, location and color of an object, to accurately perceive the object and differentiate it from other objects viewed over time [24]. Further, performance of a virtual reality spatial navigation task can differentiate individuals with mild cognitive impairment (MCI) at increased risk of developing dementia due to AD, based on biomarker status, relative to individuals with MCI at low risk of developing dementia [25]. In spite of evidence that visuospatial processing and navigation may be impacted by AD, to date, the visual search strategies of older adults who are at elevated risk of developing AD, based on Aβ deposition, have not been explored.
Here, we implemented a grocery shopping task in a subset of older adults from the Brain Networks and Mobility (B-NET, NCT03430427) study [26] who had additional data collected to measure Aβ deposition in the brain to quantify objective performance of a complex, everyday task. We selected grocery shopping as a complex everyday task because it places high demands on visual search and working memory [27, 28], hypothesizing that subtle deficits in these cognitive domains due to impaired feature binding could impact the ability to recognize and remember the unique features of each grocery item. Grocery shopping requires coordination of multiple cognitive domains, limiting an individual’s ability to compensate for subtle declines in cognition [7, 8]. Virtual reality versions of grocery shopping have shown that recalling items from a grocery list, finding relevant items in the store and selecting the correct items are associated with measures of learning, attention, working memory, and executive function [27, 28], which are often affected early in AD [10, 29, 30]. Further, Zygouris et al. [28] determined that performance time and accuracy during a tablet-based virtual reality grocery shopping task could differentiate between those with amnestic mild cognitive impairment (MCI), multidomain MCI, and healthy controls [28]. Although virtual grocery shopping tasks can detect subtle cognitive deficits, a major limitation is that performance is impacted by an individual’s familiarity with technology [27]. While some older adults frequently utilize computers and smartphones, others rarely, if ever, use these same technologies [31]. Relatedly, individuals with cognitive deficits associated with schizophrenia often take longer or shorter amounts of time to perform a virtual grocery shopping task, relative to the same task in a real-world grocery store [32], and these differences are likely due to familiarity with using computers or playing video games [33, 34]. Thus, objective metrics quantified from performance of a challenging and ecologically valid grocery shopping task may be able to detect early declines in cognitive functions while avoiding confounds related to familiarity with technology.
The purpose of this study was to identify if eye movements obtained from a grocery shopping task could: 1) differentiate older adults at elevated risk of developing AD (OAer), OA at low risk of developing AD (OAlr), and young adults (YA), and 2) be predicted by Aβ deposition in older adults (OA). We hypothesized that from YA to OAlr to OAer, each group would exhibit progressively less efficient working memory and visual search, as evidenced by a greater number of fixations on the grocery list and relevant grocery items (e.g., the correct shelving unit, brand, and shelf associated with a target grocery item) and a smaller percentage of the total trial time fixating on relevant grocery items (e.g., as individuals spent a larger percentage of the total trial time fixating on grocery items that were dissimilar to a target grocery item, such as the wrong shelving unit, brands, or shelves). We also hypothesized greater Aβ deposition in OA would predict a greater number of fixations on the grocery list and relevant grocery items and a smaller percentage of the total trial time fixating on relevant grocery items.
METHODS
Participants
The current study involves individuals who were recruited to participate in an extra study visit, as part of the B-NET study, which is a longitudinal, observational study. Those who participated in the extra study visit were already enrolled in the B-NET study and had already performed all baseline screening assessments as part of the larger B-NET study, excluding those with evidence of impaired global cognition. All individuals were recruited from Forsyth County, NC and the surrounding region. The inclusion criteria for the larger B-NET study were: being a community-dwelling OA over 70 years of age or a YA aged 25-35 years and the ability to communicate with study personnel. The exclusion criteria were: diagnosis of a neurologic disease, prior traumatic brain injury with residual effects, history of brain or spinal cord tumor, serious or uncontrolled chronic disease, having an amputation or musculoskeletal impairments that prevented performing all study procedures, dependence on a walker or another person to ambulate, significant uncorrectable hearing and/or vision impairment, an unwillingness or inability to have an MRI brain scan, plans to relocate during the study period and an unwillingness to return for planned study visits, participation in a structured exercise or cognitive intervention, and evidence of impaired global cognition (i.e., cognitive impairment). Cognitive impairment was determined by the study neuropsychologist and primarily based on performance on the Montreal Cognitive Assessment (MoCA), as described in our associated work [35]. A MoCA score of < 21 was considered to be evidence of suspected mild cognitive impairment or dementia and exclusionary. Intermediate MoCA scores (21 to 25) were reviewed by the study neuropsychologist, with additional consideration of other baseline cognitive screening assessments, to determine cognitive status (i.e., no or suspected cognitive impairment). Individuals who were determined to have suspected cognitive impairment were excluded from participation in the larger B-NET study. The inclusion criteria for the extra study visit were: the ability to communicate in English with study personnel, the ability to walk quickly for at least two minutes at a time without assistance, and, additionally for the OA, a willingness to have an Aβ positron emission tomography (PET) scan. Exclusion criteria for the extra study visit were: recent surgery or hospitalization within 6 months prior to the supplemental visit and uncorrected hearing and/or vision impairments. The extra study visit and the larger B-NET study were approved by the Institutional Review Boards at the involved institutions, and all participants gave written, informed consent prior to participating in any study procedures.
Quantification of Aβ deposition
Global brain Aβ deposition was quantified by Pittsburgh Compound-B (PiB) PET imaging in OA participants. Brain MRI data were first obtained for all OA participants on a 3T Siemens Skyra scanner via a high-resolution 32-channel head coil. MRI sequences included T1-weighted 3D volumetric MPRAGE (1×1×1 mm isotropic voxels, matrix 192×240×256, TR = 2300 ms, TE = 2.98 ms), and the scans were processed using FreeSurfer v5.3 (https://surfer.nmr.mgh.harvard.edu) to generate target and reference regions of interest for amyloid PET scan processing. Then [11C]Pittsburgh compound-B (PIB) [36] was utilized to assess fibrillar Aβ brain deposition on PET. Participants were injected with an i.v. bolus of ~ 10 mCi (370 MBq) (+/–10%) [11C]PiB over 5–10 s, followed by 40-min uptake. Prior to the PET scan, a computed tomography (CT) scan was performed for attenuation correction. Emission images were acquired continuously for 40–70 min post-injection (6×5-min frames) on a 64-slice GE Discovery MI DR PET/CT scanner. PET images were reconstructed as multi-frame images, motion correction was applied to PET images, and then the corrected PET images were averaged into a 3D image. Participant CT scans were transformed to MRI space, and the transformation was then applied to coregister the PET images (in the same native space as CT) to the MRI scans. A voxelwise 40–70 min standardized uptake volume ratio (SUVR, cerebellar grey matter reference) image was then generated. Global brain Aβ deposition was calculated as PiB SUVR, averaged from a cortical meta-region of interest sensitive to early AD [37, 38], using FreeSurfer-segmented regions (Supplementary Table 1). This meta-ROI has been demonstrated to be sensitive to preclinical Aβ pathology and predictive of future cognitive and functional decline [37, 38], and was appropriate for characterizing early neuropathology in the OA B-NET cohort and relating to functional outcomes explored in the current study. This global PiB SUVR measure served as a biomarker of Aβ burden. Rather than use a single cut-off score, Aβ+ was determined based on a threshold of > =1.21 SUVR and, for those initially classified as exhibiting intermediate Aβ deposition (i.e., 1.21–1.4 PiB SUVR), using expert visual raters to adjudicate Aβ positivity status [39]. Aβ–was determined based on a threshold of <1.21 SUVR. After quantifying Aβ deposition, OA were classified as either at elevated risk of developing AD (OAer, i.e., having elevated Aβ deposition [Aβ+]) or at low risk of developing AD (OAlr, i.e., having insignificant Aβ deposition [Aβ–]). The MRI and PET scans, Aβ deposition quantification, and assignment of OA to coded groups, based on their Aβ+ or Aβ–status were completed by personnel from the larger B-NET study. Thus, participants and research personnel involved in collecting data for the current study were blinded to which OA were Aβ+ and which were Aβ–, respectively. OA were analyzed as two separate OAer and OAlr groups for the first study aim and as a single OA group, with Aβ deposition included as a continuous variable, for the second study aim.
Descriptive assessments
Demographic data, including age, gender, race/ethnicity, and years of formal education completed, were collected as part of the larger study during the baseline visit, in conjunction with the cognitive screening assessments. Older adult participants also completed a PET scan, the timing of which was variable relative to the current study visit due to difficulties during the COVID-19 pandemic.
During the current study visit, participants from the OAer, OAlr, and YA groups all completed the same procedures. Participants first completed a series of cognitive assessments, including the Coding subtest from the Wechsler Adult Intelligence Scale 4th edition [40] to assess information processing speed and the Comprehensive Trail Making Test (CTMT) [41] to assess general executive function (Total Composite Index, CTMT TCI), inhibition of distraction (Inhibitory Control Index, CTMT ICI), and set-shifting ability (Set-Shifting Index, CTMT SSI). Participants then completed a functional mobility assessment, the expanded Short Physical Performance Battery (eSPPB Total) [42], to assess balance, gait speed, narrow walking gait speed, and repeated chair stands. Participants also completed several self-reported questionnaires, including the Physical Activity Scale for the Elderly (PASE) [43] to assess self-reported physical activity, the Activities-specific Balance Confidence Scale (ABC) [44] to assess balance confidence, and the Mobility Assessment Tool-short form (MAT-sf) [45] to assess self-perceived walking ability. In addition to these initial assessments, after completing all four grocery shopping trials, participants completed a grocery shopping questionnaire that was developed for this specific study. The questionnaire assessed their grocery shopping habits before and during the COVID pandemic and their familiarity with the grocery items and specific brands utilized in the grocery shopping task.
Grocery shopping task
Following the initial assessments, participants completed a series of four grocery shopping trials. The grocery shopping setup was comprised of two 1.7 m shelving units, with closed, opaque sides, that faced each other and were stocked with canned goods, mimicking part of a grocery store aisle (Fig. 1A). One shelving unit contained 36 canned beans, with 18 Bush’s brand beans and 18 Goya brand beans (Fig. 1B), and the other shelving unit contained 36 canned soups, with 18 Progresso brand soups and 18 Campbell’s brand soups (Fig. 1C). The 18 items from each brand were divided into three subvarieties, with six cans in each subvariety. Within the Bush’s beans, there were six different cans of chili beans, six different cans of reduced sodium beans, and six different cans of traditional beans, and within the Goya beans, there were six different cans of beans in sauce, six different cans of low sodium beans, and six different cans of traditional beans. Within the Progresso soups, there were six different cans of rich and hearty soups, six different cans of light soups, and six different cans of traditional soups, and within the Campbell’s soups, there were six different cans of chunky soups, six different cans of well yes! soups, and six different cans of traditional soups. Each of the subvarieties was placed on a different shelf, and the three shelves from each brand were grouped together.
Fig. 1
Grocery shopping setup, including A) a schematic of the grocery shopping trial setup, B) the beans shelving unit, and C) the soups shelving unit.
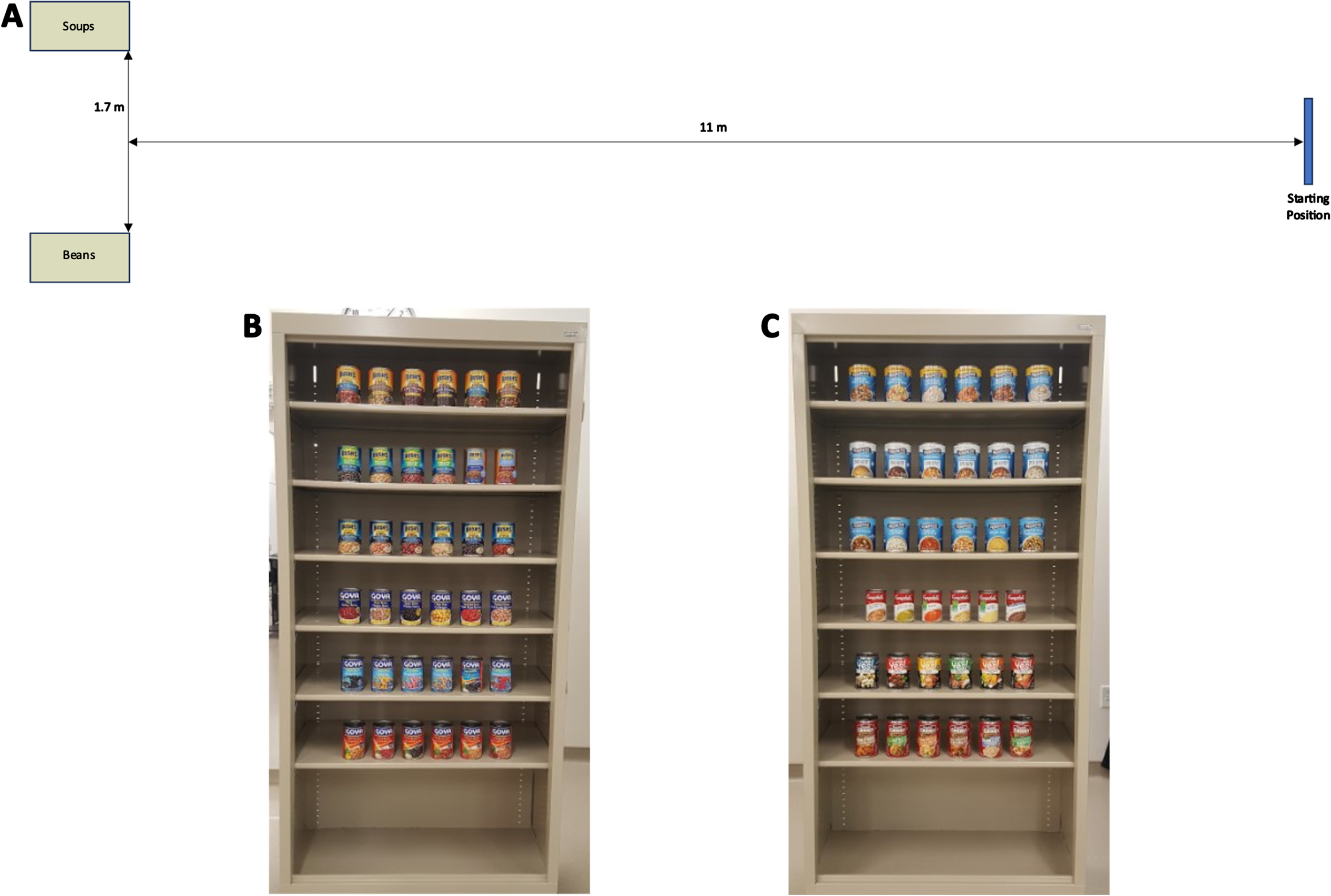
Participants completed four grocery shopping trials, two in which they were asked to retrieve a can of beans, and two in which they were asked to retrieve a can of soup. Participants began each trial 11 m away from the two grocery shopping shelving units (Supplementary Figure 1). At the start of each trial, they were instructed to “walk towards the grocery shelves, pick up a specific item that I’ll name in a second, and then bring it back and place it here [in a specific location at the starting position]. I want you to do this task at your preferred, comfortable walking speed, just like you would if you were walking in an aisle at the grocery store.” They were then asked if they had any questions, those questions were answered, and then they were finally instructed to “remember, for this trial I want you to walk at your preferred, comfortable speed, and I want you to go and get the [specific item named and a grocery notecard with the name of the grocery item printed on it was handed to the participant]. You may start walking whenever you’re ready.” Target items were placed at one of four quadrants on the shelving unit: upper left, upper right, lower left, lower right. During the four grocery shopping trials, participants were asked to find items located at different diagonal positions on each shelving unit to ensure that they had to search for items in different locations on each of the four trials. For example, if a participant was first asked to find an item that was located in the upper left quadrant on the soups shelving unit, they were subsequently asked to find another soup item that was located in the lower right quadrant. Then, they were asked to find bean items that were located in the upper right and lower left quadrants of the beans shelving unit during the last two trials. Each of the two soups trials and each of the two beans trials were always grouped together, but the order in which participants were asked to find soups or beans and in which participants were asked to find grocery items in each of the four quadrants was counterbalanced across participants. This counterbalanced order was maintained across the OAer, OAlr, and YA groups to minimize an order or location effect on performance.
During the grocery shopping trials, gaze data were recorded using Tobii Pro Glasses 3 (firmware version 1.23.1 + pumpa, Stockholm, Sweden) wireless eye-tracking glasses, which did not restrict head movement, and Tobii Pro Glasses 3 Controller software (version 1.16.4, Stockholm, Sweden) installed on a laptop computer in an environment with bright, consistent lighting. The glasses and the controller software recorded a digital video of the participant’s viewpoint (25 Hz, 1920×1080 pixel resolution) via a camera embedded into the nose bridge of the glasses, binocular gaze coordinate data (100 Hz, accuracy of 0.6° visual angle) via two cameras embedded into each glasses lens as horizontal and vertical gaze position in pixels relative to the participant viewpoint video, where (0,0) corresponded to the top-left of the participant viewpoint video, and audio via a microphone embedded into the glasses frame. Snap-on corrective lenses (Tobii Pro, Stockholm, Sweden) were used to substitute for prescription glasses, as needed. The built-in Tobii Pro single-point calibration procedure was utilized with the calibration card provided by Tobii Pro, prior to the start of recording gaze data. To validate each calibration, the participant was asked to direct their gaze to various targets in the environment. In the case that one or more gaze points were offset from the targets in the environment, the glasses were recalibrated.
After data collection, Tobii Pro Lab software (version 1.207, Stockholm, Sweden) was utilized to superimpose the gaze coordinate data on top of the participant’s viewpoint video during each trial, and then classify the gaze location in each frame to pre-defined areas of interest (AOIs). The AOIs were layered, including 36 individual can AOIs on the shelving unit that contained the prespecified grocery item (i.e., 36 different soup can AOIs during soups trials and 36 different beans can AOIs during beans trials), 6 shelf AOIs corresponding to a subvariety within each brand (i.e., three for each brand on the correct shelving unit), 2 brand AOIs (i.e., encompassing all three subvariety shelves for each brand on the correct shelving unit), and 1 correct shelving unit AOI (i.e., the shelving unit that contained the prespecified grocery item, which was the soups shelving unit for the soups trials and the beans shelving unit for the beans trials). By layering the AOIs, any gaze location on an individual can AOI on the correct shelving unit would also register as a gaze location on the corresponding shelf (subvariety), the brand, and the correct shelving unit. There was also a separate AOI for the grocery item notecard, for the wrong shelving unit (i.e., the shelving unit that did not contain the prespecified grocery item, which was the soups shelving unit during the beans trials and the beans shelving unit during the soups trials), and for all other locations external to the shelving unit (e.g., the walking path or surrounding environment in the lab). Within Tobii Pro Lab, short gaps in the data (≤25 ms) were filled through linear interpolation, noise in the data was filtered using a 5-sample moving median, the window length was set to 20 ms in the velocity calculator, the velocity-threshold identification fixation filter was set to 50°/s, adjacent fixations with a maximum time of 45 ms and angle of 1.0° between them were merged, and fixations shorter than 45 ms were discarded. Then a custom Matlab program (MathWorks, Natick, MA) was utilized to validate each fixation, such that the duration of each fixation lasted at least 45 ms, the angular velocity of each fixation did not exceed 50°/s, and the change in angle during each fixation did not exceed 1.0°. The minimum fixation duration of 45 ms reflects the minimum amount of time needed to process and identify visual stimuli [46]. The maximum angular velocity of 50°/s and maximum change in angle of 1.0° were used to obtain good separation of fixations from saccades in older participants [47]. In examining the data, 9.2% of samples were lost as a result of blinks or no tracking data being recorded. These lost samples seem to be randomly distributed across participants and time.
Grocery shopping measures
Two goal-related measures of task performance were computed from the grocery shopping trials of each participant. Trial Time quantified the amount of time that each participant used to select a grocery item for each of the four grocery shopping trials. It was computed from the recorded eye-tracking glasses audio, gaze, and digital video data as the elapsed time, in seconds, from when the participant walked near enough to the grocery shelving units to be able to see all of the cans on the shelves until the participant brought the selected item (correct or incorrect) back to the starting position. Number of Correct Items quantified errors in selecting the correct grocery item for each participant across all four trials. It was computed as the number of trials in which each participant selected and brought back the correct (prespecified) grocery item to the starting position.
Valid fixations and their corresponding AOIs were used to compute several measures related to how eye movements were used to perform the grocery shopping task from when the prespecified grocery item was announced at the end of each trial instruction until the participant selected the item (correct or incorrect) by turning to walk back to the starting position with the selected item in their hand. Grocery Note Fixations was the number of fixations on the notecard specifying the correct grocery item (i.e., grocery list) during each trial. The remaining measures were quantified in terms of both the number of fixations and the percentage of time spent fixating on relevant items during each trial. Correct Item Fixations was the number of fixations on the correct (i.e., prespecified) grocery item. Percentage of Time Fixating on Correct Item was quantified as the percentage of all fixation time spent fixating on the correct grocery item. Correct Shelf Fixations was the number of fixations on the correct shelf (i.e., belonging to the correct subvariety of soup or beans). Percentage of Time Fixating on Correct Shelf was the percentage of all fixation time spent fixating on the correct shelf. Correct Brand Fixations was the number of fixations on the correct brand (i.e., on the three shelves comprising the correct brand of soup or beans). Percentage of Time Fixating on Correct Brand was the percentage of all fixation time spent fixating on the correct brand. Correct Shelving Unit Fixations was the number of fixations on the correct shelving unit (i.e., on any of the shelves in the shelving unit containing the prespecified can of soup or beans). Percentage of Time Fixating on Correct Shelving Unit was the percentage of all fixation time spent fixating on the correct shelving unit. Selected Item Fixations was the number of fixations on the selected item (i.e., whether or not it was the correct item). Percentage of Time Fixating on Selected Item was the percentage of all fixation time spent fixating on the selected item.
For each participant, Trial Time and the correctness of the item selected was used to rank all four trial performances. The “best” and “worst” of the four performances were then analyzed, to account for the impact of familiarity with the different brands, subvarieties, and specific grocery items on the visual search process and to better highlight the impact of searching for an item that is unfamiliar. Additionally, this analysis allows for an examination of how Aβ deposition impacts grocery shopping performance measures when OA are performing to the best of their ability (i.e., are less challenged) or performing poorly (i.e., are more challenged). Analyzing the mean performance across all four trials, instead of analyzing the best and worst performances, would have lessened the impact of searching for unfamiliar items in the task performance measures if a participant was very familiar with one or two of the prespecified items and very unfamiliar with one or two of the other prespecified items. It also would have precluded examining the impact of Aβ deposition on the visual search process under more and less challenging conditions in OA. The Best Performance was defined as the trial with the shortest trial time to select the correct item or as the trial with the shortest trial time if all four selections were incorrect. The Worst Performance was defined as the trial with the longest trial time to select an incorrect item or as the longest trial time if all four selections were correct.
Statistical analysis
Analyses were conducted using R (R Core Team 2020), RStudio (Posit team 2023), and the tidyverse package [48]. Significance was set a priori at p≤0.05.
One-way ANOVAs and post hoc comparisons with Tukey corrections compared age, years of education, cognitive test scores, and functional mobility test scores between the three groups (YA, OAlr, OAer). T-tests compared the OAlr and OAer in Aβ deposition and in the number of days between PET scan and the behavioral study. Chi-square tests of independence compared sex and race proportions across the three groups. Chi-square tests of independence also compared the likelihood for each group that participants performed their best on a given trial (trial 1–4) and performed their worst on a given trial. Kruskal-Wallis rank sum tests compared familiarity with can brands across the three groups.
To determine the effect of group (OAer, OAlr, YA) on grocery shopping performance, a one-way ANCOVA and post hoc comparison with Tukey corrections compared the number of correct items across groups (OAer, OAlr, YA), covarying for years of education. Linear mixed effects models compared outcome measures within group (OAer, OAlr, and YA) and between performance rank (best and worst performances), covarying for years of education. The mixed models included random intercepts for participant, grocery item type (bean or soup) within participant, and trial order (first or second) within participant to account for individual-specific, grocery item type-specific, and trial-specific variability in the outcomes. The significance of main effects and interactions were determined with t-tests using Satterthwaite’s method [49]. Post hoc comparisons between groups, performance rank, or the interaction of the two were applied using Tukey corrections.
To determine the effect of Aβ deposition in OA on grocery shopping performance, a linear regression estimated the fixed effect of Aβ levels, covarying for years of education and time between the PET scan and current study visit, on the number of correct items. Linear mixed effects models estimated the effects on outcome measures by Aβ levels and performance rank (best and worst performances), covarying for years of education and time between the PET scan and current study visit. Mixed models included random intercepts for participant, grocery item type (bean or soup) within participant, and trial order (first or second) within participant to account for individual-specific, grocery item type-specific, and trial-specific variability in the outcomes. The significance of main effects and interactions were determined with t-tests using Satterthwaite’s method [49].
We applied the False Discovery Rate (FDR) correction for multiple hypothesis testing (using the Benjamini-Hochberg procedure) to the p-values of all hypothesis tests (n = 78). The FDR correction identified the most influential grocery shopping performance measures, but the results that were no longer significant after the correction were still reported and interpreted due to the exploratory and hypothesis-generating aspects of the novel grocery shopping task employed in this study.
RESULTS
Fifty-six OA and 29 YA participated in the extra study visit. One OA was excluded from the analysis because they could not be classified as Aβ+ or Aβ– after deciding against undergoing a PET scan. One OA from the OAer group was excluded from the analysis due to technical difficulties. Additionally, two OA in the OAer group did not complete the Coding subtest because of a hand tremor that was not caused by a neurological disease but would have regardless invalidated the test score. One YA was excluded from the analysis because they did not complete the grocery shopping trials due to technical difficulties. Therefore, 21 cognitively normal OAer, 33 cognitively normal OAlr, and 28 YA participants were included in the analysis. Data from the descriptive assessments, including age, gender, race/ethnicity, years of formal education completed, Aβ deposition, time between the PET scan and participation in the extra study visit, cognitive function, functional mobility, and self-report measures for the OAer, OAlr, and YA groups are summarized in Table 1. Both OA groups were older than the YA (both p < 0.001) and scored lower than the YA on the Expanded Short Physical Performance Battery (both p < 0.001), the Activities-Specific Balance Confidence Scale (both p < 0.01), and the Mobility Assessment Tool short form (both p < 0.001). AB deposition was higher in the OAer than in the OAlr group (p < 0.001). Sex (χ2(2) = 1.987, p = 0.370), race (χ2(6) = 12.262, p = 0.056), years of education (F(2,79) = 1.393, p = 0.254), cognitive test scores (WAIS IV Coding: F(2,77) = 0.648, p = 0.526; CTMT ICI percentile score: F(2,79) = 0.741, p = 0.480; CTMT set-shifting percentile score: F(2,79) = 0.325, p = 0.723; CTMT TCI percentile score: F(2,79) = 0.347, p = 0.708), and the Physical Activity Scale for the Elderly (F(2,79) = 1.174, p = 0.315) did not differ across the three groups. Answers for the grocery shopping questionnaire in the OAer, OAlr, and YA groups are summarized in Table 2, along with familiarity indices for can brands. We found that YA were significantly more familiar with Bush’s beans than were both OA groups (YA = 2.14, OAlr = 1.67, OAer = 1.43; Kruskal-Wallis χ2(2) = 12.069, p = 0.002). The three groups did not differ in the number of Bush’s beans they had to retrieve (YA = 16/28, OAlr = 19/33, OAer = 13/21; χ2(2) = 1.125, p = 0.570), nor did they differ in the proportion of Bush’s beans they got correct (YA = 16/16, OAlr = 16/19, OAer = 13/13; χ2(2) = 4.884, p = 0.087), nor did they differ in the proportion of Bush’s beans trials that were ranked their “worst” performances (YA = 4/16, OAlr = 5/19, OAer = 2/13; χ2(2) = 0.581, p = 0.748). Therefore, familiarity with the Bush’s brand did not appear to bias the results and we kept these trials in the analyses.
Table 1
Characteristics of participants in the YA, OAlr, and OAer groups. Values are Mean (SD) or Median (Q1–Q3)
YA (n = 28) | OAlr (n = 33) | OAer (n = 21) | |
Demographic Characteristics | |||
Age (y) | 31 (3) | 78 (5)* | 78 (5)* |
Female n (%) | 17 (60.7%) | 15 (45.5%) | 9 (42.9%) |
Race/Ethnicity n (%) | |||
Caucasian/White | 18 (64.3%) | 28 (84.9%) | 19 (90.5%) |
African American/Black | 3 (10.7%) | 4 (12.1%) | 2 (9.5%) |
Asian | 3 (10.7%) | 1 (3.0%) | 0 (0%) |
Multiracial/Other | 4 (14.3%) | 0 (0%) | 0 (0%) |
Education (y) | 16.9 (2.1) | 16.1 (2.6) | 15.7 (3.1) |
Aβ deposition (SUVR) | NA | 1.14 (1.12–1.18) | 1.74 (1.53–1.95)† |
Timing between PET scan and participation in current study visit (in months) | NA | 18 (14–20) | 18 (5–19) |
Timing between baseline demographic and cognitive assessments and participation in current study visit (in months) | 0 (0–0) | 28 (25–29) | 27 (16.5–28) |
Cognitive Assessments | |||
WAIS IV Coding (age-adjusted scaled score, max 19) | 12 (11–13.25) | 12 (11–13) | 12 (11–14) |
Comprehensive Trail Making Test Total Composite Index (age-adjusted percentile) | 58 (20.5–70) | 55 (34–77) | 58 (40–77) |
Comprehensive Trail Making Test Inhibitory Control Index (age-adjusted percentile) | 52.5 (18.25–67) | 47 (34–70) | 55 (40–73) |
Comprehensive Trail Making Test Set-Shifting Index (age-adjusted percentile) | 60.5 (30.75–80.25) | 63 (40–82) | 55 (40–73) |
Functional Mobility Assessment | |||
Expanded Short Physical Performance Battery (Total score, max 4) | 2.8 (0.2) | 2.3 (4)* | 2.3 (0.4)* |
Self-reported Questionnaires | |||
Physical Activity Scale for the Elderly (max, 360) | 166.2 (75.6) | 140.6 (62.2) | 148.7 (57.3) |
Activities-Specific Balance Confidence Scale (max. 100%) | 97.81 (96.17–98.75) | 88.75 (83.75–95.31)* | 87.50 (83.13–92.50)* |
Mobility Assessment Tool—short form (max, 80) | 73.13 (70.92–73.13) | 68.99 (63.34–70.31)* | 65.36 (62.01–70.31)* |
Best performances by trial order | |||
Trial 1 n (%) | 4 (14.3%) | 5 (15.2%) | 2 (9.52%) |
Trial 2 n (%) | 8 (28.6%) | 7 (21.2%) | 9 (42.9%) |
Trial 3 n (%) | 9 (32.2%) | 11 (33.3%) | 5 (23.8%) |
Trial 4 n (%) | 7 (25%) | 10 (30.3%) | 5 (23.8%) |
Worst performances by trial order | |||
Trial 1 n (%) | 8 (28.6%) | 11 (33.3%) | 11 (52.4%) |
Trial 2 n (%) | 7 (25%) | 8 (24.2%) | 7 (33.3%) |
Trial 3 n (%) | 6 (21.4%) | 8 (24.2%) | 0 (0%) |
Trial 4 n (%) | 7 (25%) | 6 (18.2%) | 3 (14.3%) |
Asterisks (*) indicate difference from YA, daggers (†) indicate difference from OAlr. Both OA groups were older than the YA and scored lower on the Expanded Short Physical Performance Battery, the Activities-Specific Balance Confidence Scale, and the Mobility Assessment Tool short form than the YA. Aβ deposition was higher in the OAer than in OAlr. Sex, race, years of education, cognitive test scores, and the Physical Activity Scale for the Elderly did not differ across the three groups. The distribution of best/worst performances were not related to trial order, and this did not differ by group.
Table 2
Grocery shopping habits in the YA, OAlr, and OAer groups. Values are n (%)
YA | OAlr | OAer | |
(n = 28) | (n = 33) | (n = 21) | |
Before COVID | |||
Did you use to grocery shop online before COVID? | |||
No | 23 (82) | 32 (97) | 19 (90) |
Very little | 3 (11) | 1 (3) | 2 (10) |
Less than half | 0 (0) | 0 (0) | 0 (0) |
About half | 1 (4) | 0 (0) | 0 (0) |
More than half | 0 (0) | 0 (0) | 0 (0) |
Most of it | 1 (4) | 0 (0) | 0 (0) |
In an average week, how much of the household grocery shopping did you do? | |||
All | 18 (64) | 17 (52) | 15 (71) |
More than half | 4 (14) | 5 (15) | 1 (5) |
About half | 1 (4) | 2 (6) | 1 (5) |
Less than half | 5 (18) | 8 (24) | 3 (14) |
None | 0 (0) | 1 (3) | 1 (5) |
In an average week, how often did you shop for groceries? | |||
Every day | 0 (0) | 0 (0) | 0 (0) |
2–4 times per week | 6 (21) | 8 (25) | 7 (33) |
Once a week | 15 (54) | 17 (53) | 10 (48) |
Once in two weeks | 5 (18) | 4 (12) | 4 (19) |
Once a month | 2 (7) | 3 (9) | 0 (0) |
In an average week, how much time did you spend grocery shopping, on average, during each shopping trip? | |||
Less than 30 minutes | 8 (29) | 12 (38) | 6 (29) |
30-60 minutes | 15 (54) | 18 (56) | 13 (62) |
1-2 hours | 5 (18) | 2 (6) | 2 (10) |
More than 2 hours | 0 (0) | 0 (0) | 0 (0) |
During the past year (COVID) | |||
Have you grocery shopped online during COVID? | |||
No | 11 (39) | 27 (82) | 15 (71) |
Very little | 7 (25) | 3 (9) | 5 (24) |
Less than half | 4 (14) | 1 (3) | 0 (0) |
About half | 4 (14) | 2 (6) | 1 (5) |
More than half | 0 (0) | 0 (0) | 0 (0) |
Most of it | 2 (2) | 0 (0) | 0 (0) |
In an average week, how much of the household grocery shopping did you do? | |||
All | 16 (57) | 16 (48) | 13 (62) |
More than half | 4 (14) | 7 (21) | 2 (10) |
About half | 1 (4) | 3 (9) | 3 (14) |
Less than half | 7 (25) | 6 (18) | 2 (10) |
None | 0 (0) | 1 (3) | 1 (5) |
In an average week, how often did you shop for groceries? | |||
Every day | 0 (0) | 0 (0) | 0 (0) |
2–4 times per week | 7 (25) | 5 (16) | 4 (19) |
Once a week | 12 (43) | 16 (50) | 10 (50) |
Once in two weeks | 8 (29) | 8 (25) | 6 (3) |
Once a month | 1 (4) | 3 (9) | 0 (0) |
In an average week, how much time did you spend grocery shopping, on average, during each shopping trip? | |||
Less than 30 minutes | 8 (29) | 11 (34) | 4 (19) |
30–60 minutes | 16 (57) | 18 (56) | 15 (71) |
1–2 hours | 4 (14) | 3 (9) | 2 (10) |
More than 2 hours | 0 (0) | 0 (0) | 0 (0) |
These days | |||
When you grocery shop, do you use a shopping list? | |||
Always | 6 (21) | 0 (0) | 4 (19) |
Often | 12 (43) | 10 (31) | 12 (57) |
Seldom | 5 (18) | 7 (22) | 4 (19) |
Never | 5 (18) | 3 (9) | 1 (5) |
When you grocery shop, do you buy items that you see in the store that are of interest (not on a list)? | |||
Always | 0 (0) | 3 (9) | 0 (0) |
Often | 6 (21) | 13 (41) | 11 (52) |
Seldom | 13 (46) | 14 (44) | 9 (43) |
Never | 9 (32) | 2 (6) | 1 (5) |
How often do you buy canned soup? | |||
Frequently | 2 (7) | 8 (24) | 5 (24) |
Occasionally | 15 (54) | 21 (64) | 15 (71) |
Never | 11 (39) | 4 (12) | 1 (5) |
How familiar are you with Campbell’s soup labels? | |||
Very familiar | 18 (64) | 24 (73) | 11 (52) |
Somewhat familiar | 5 (18) | 7 (21) | 10 (50) |
Not familiar | 5 (18) | 2 (6) | 0 (0) |
Average familiarity (1 to 3) | 1.54 | 1.33 | 1.48 |
How familiar are you with Progresso soup labels? | |||
Very familiar | 12 (43) | 13 (39) | 9 (43) |
Somewhat familiar | 8 (29) | 15 (45) | 10 (50) |
Not familiar | 8 (29) | 5 (15) | 2 (10) |
Average familiarity (1 to 3) | 1.86 | 1.76 | 1.67 |
How often do you buy canned beans? | |||
Frequently | 5 (18) | 10 (3) | 11 (52) |
Occasionally | 19 (68) | 17 (52) | 9 (43) |
Never | 4 (14) | 6 (18) | 1 (5) |
How familiar are you with Goya canned beans labels? | |||
Very familiar | 8 (29) | 3 (9) | 3 (14) |
Somewhat familiar | 12 (43) | 14 (42) | 8 (38) |
Not familiar | 8 (29) | 16 (48) | 10 (50) |
Average familiarity (1 to 3) | 2.00 | 2.39 | 2.33 |
How familiar are you with Bush’s canned beans labels? | |||
Very familiar | 6 (21) | 14 (42) | 14 (67) |
Somewhat familiar | 12 (43) | 16 (48) | 5 (24) |
Not familiar | 10 (36) | 3 (9) | 2 (10) |
Average familiarity (1 to 3) | 2.14 | 1.67* | 1.43* |
Familiarity indices were calculated for each of the 4 can brands. Asterisks (*) indicate difference from YA. On average, YA were more familiar with Bush’s than were both OAler (p = 0.040) and OAer (p = 0.005).
Effect of group (YA, OAlr, and OAer) on grocery shopping performance
Trial order (1 through 4) did not affect which trial was a person’s “best” performance (χ2(3) = 6.098, p = 0.107), and the association between trial order and “best” trial was not affected by group (χ2(6) = 3.063, p = 0.801) (Table 1). Likewise, trial order did not affect which trial was a person’s “worst” performance (χ2(3) = 7.561, p = 0.056), and the association between trial order and “worst” trial was not affected by group (χ2(6) = 8.113, p = 0.230) (Table 1). Of all models, the number of years of education was a significant covariate only in the Aβ regression model predicting the percentage of time fixating on the correct shelf (B = 0.9%, p = 0032).
The omnibus statistics are provided in Table 3. Significant interactions and main effects are shown in Figs. 2 and 3. There was a significant main effect of group on number of correct grocery items selected, and follow-up tests revealed that both OAlr and OAer selected fewer correct grocery items than YA did (p < 0.001 and p = 0.020 respectively, Fig. 2A). There were significant interactions between group and performance rank in trial time; number of fixations and percentage of time fixating on the correct shelving unit; number of fixations on the correct brand; number of fixations and percentage of time fixating on the correct shelf; and number of fixations on the selected item (Table 3). Follow-up testing revealed that during their worst performance, relative to their best, both OA groups exhibited longer trial times, (OAer, B = 18.6 s, p = 0.021; OAlr, B = 32.0 s, p < 0.001) (Fig. 2B), fixated more times on the correct shelving unit (OAer, B = 41 fixations, p = 0.031; OAlr, B = 76 fixations, p < 0.001) (Fig. 2D), and fixated more times on the correct brand (OAer, B = 36 fixations, p = 0.028; OAlr, B = 61 fixations, p < 0.001) (Fig. 3A). Meanwhile, YA’s best and worst performances did not differ in trial time, fixations on the correct shelving unit, or fixations on the correct brand. Follow-up testing also revealed that YA and OAlr both spent a greater percentage of time fixating on the correct shelving unit during their worst performances (OAlr, B = 21.3%, p < 0.001; YA, B = 19.4%, p < 0.001), while OAer’s best and worst performances did not differ in the percentage of time spent fixating on the correct shelving unit (Fig. 2E). YA and OAer spent a smaller percentage of time fixating on the correct shelf (OAer, B = –18.9%, p < 0.001; YA, B = –11.9%, p = 0.003) (Fig. 3D), but OAlr did not. Finally, only OAlr fixated more times on the selected item (OAlr, B = 6 fixations, p < 0.001) (Fig. 2F) and on the correct shelf (OAlr, B = 19 fixations, p < 0.001) (Fig. 3C) during their worst trial.
Table 3
Effect of group (YA, OAlr, and OAer) and rank (best and worst performances) on grocery shopping performance measures
Group | Rank | Group*Rank | Education | ||||||
F | p | F | p | F | p | F | p | B (SE) | |
Number of correct items | 11.900 | <0.001 | — | — | 0.140 | 0.603 | — | ||
Trial time | 12.457 | <0.001 | 55.271 | <0.001 | 4.817 | 0.010 | 0.187 | 0.666 | –0.2458 (0.568) |
Number of grocery note fixations | 3.0779 | 0.051 | 3.7784 | 0.056 | 1.4817 | 0.234 | 2.9021 | 0.092 | –0.4745 (0.279) |
Number of correct shelving unit fixations | 8.227 | <0.001 | 59.2834 | <0.001 | 5.0522 | 0.008 | 0.0803 | 0.777 | –0.3572 (1.261) |
Percentage of time fixating on correct shelving unit | 11.4709 | <0.001 | 73.0933 | <0.001 | 6.4254 | 0.002 | 0.0836 | 0.773 | 0.1152 (0.398) |
Number of selected item fixations | 5.8085 | 0.004 | 20.3924 | <0.001 | 3.1213 | 0.049 | 0.4738 | 0.493 | 0.1232 (0.179) |
Percentage of time fixating on selected item | 0.123 | 0.885 | 19.7117 | <0.001 | 0.1347 | 0.874 | 0.2021 | 0.654 | 0.0907 (0.202) |
Number of correct brand fixations | 7.3551 | 0.001 | 54.102 | <0.001 | 3.7967 | 0.025 | 0.4003 | 0.528 | –0.6881 (1.088) |
Percentage of time fixating on correct brand | 4.9727 | 0.009 | 10.0848 | 0.002 | 2.0872 | 0.130 | 0.1826 | 0.670 | –0.2078 (0.486) |
Number of correct shelf fixations | 3.8962 | 0.022 | 11.7866 | 0.001 | 4.698 | 0.010 | 0.1672 | 0.683 | 0.2034 (0.498) |
Percentage of time fixating on correct shelf | 1.5576 | 0.217 | 51.7025 | <0.001 | 4.1539 | 0.019 | 1.8665 | 0.176 | 0.5054 (0.370) |
Number of correct item fixations | 2.9892 | 0.054 | 2.03 | 0.157 | 2.2308 | 0.112 | 1.2536 | 0.265 | 0.214 (0.191) |
Percentage of time fixating on correct item | 0.5954 | 0.554 | 65.2094 | <0.001 | 0.3244 | 0.724 | 1.2576 | 0.265 | 0.2424 (0.216) |
Fig. 2
Overall grocery shopping performance, fixations on the correct shelving unit, and fixations on the selected item during participants’ best and worst performances. Significant interactions between group and performance rank are indicated by a colored asterisk(s) and bracket at the respective group. A main effect of group is indicated by a black asterisk(s) and bracket that spans across the groups that differ. A main effect of performance rank is indicated by a black asterisk(s) and the words “performance rank” in the top right of the plot. Level of significance is indicated as follows: ***p<0.001, **p<0.01, *p<0.05.
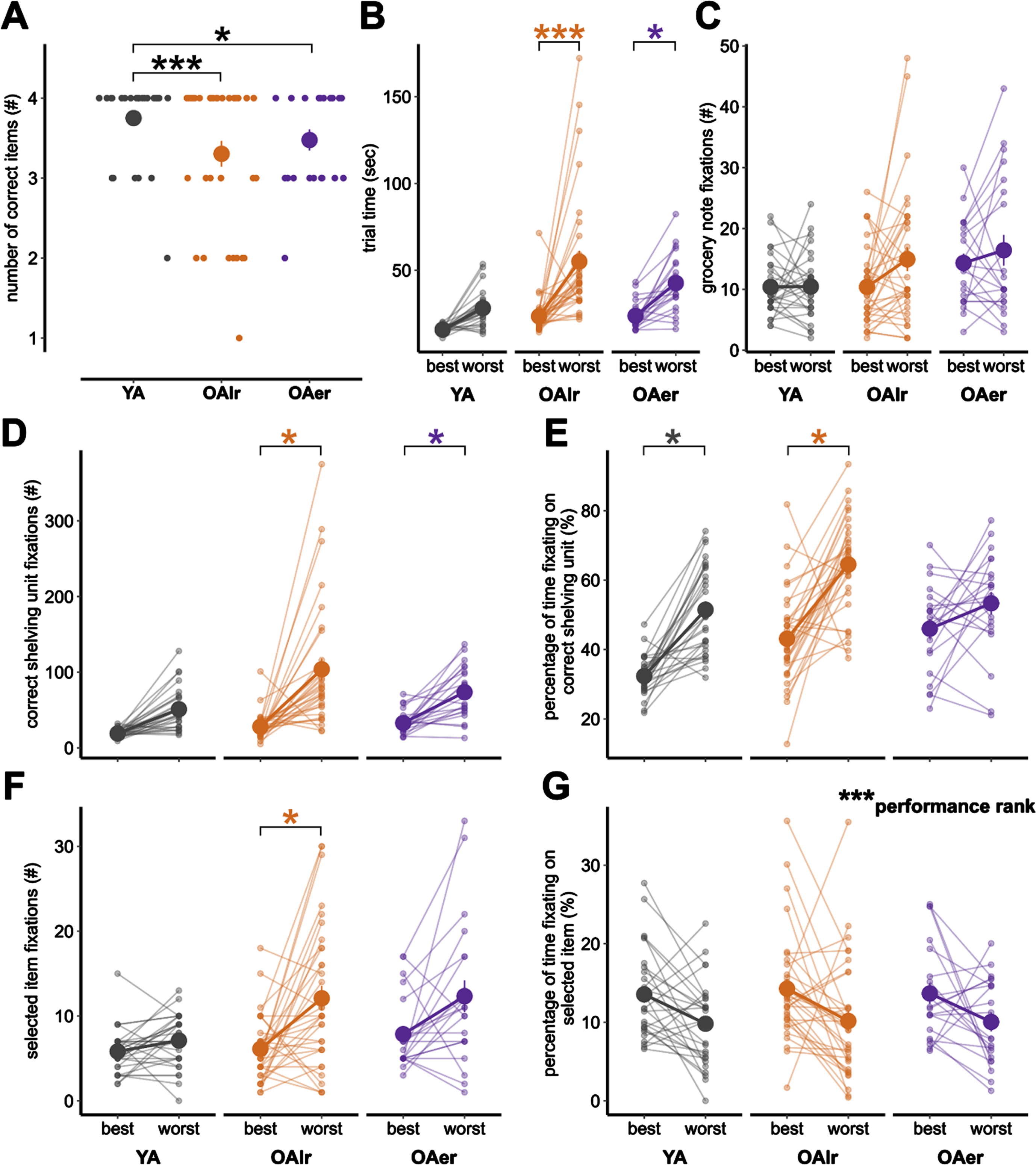
Fig. 3
Fixations on the correct brand, shelf, and item during grocery shopping during participants’ best and worst performances. Significant interactions between group and performance rank are indicated by a colored asterisk(s) and bracket at the respective group. A main effect of group is indicated by a black asterisk(s) and bracket that spans across the groups that differ. A main effect of performance rank is indicated by a black asterisk(s) and the words “performance rank” in the top right of the plot. Level of significance is indicated as follows: ***p<0.001, **p<0.01, *p<0.05.
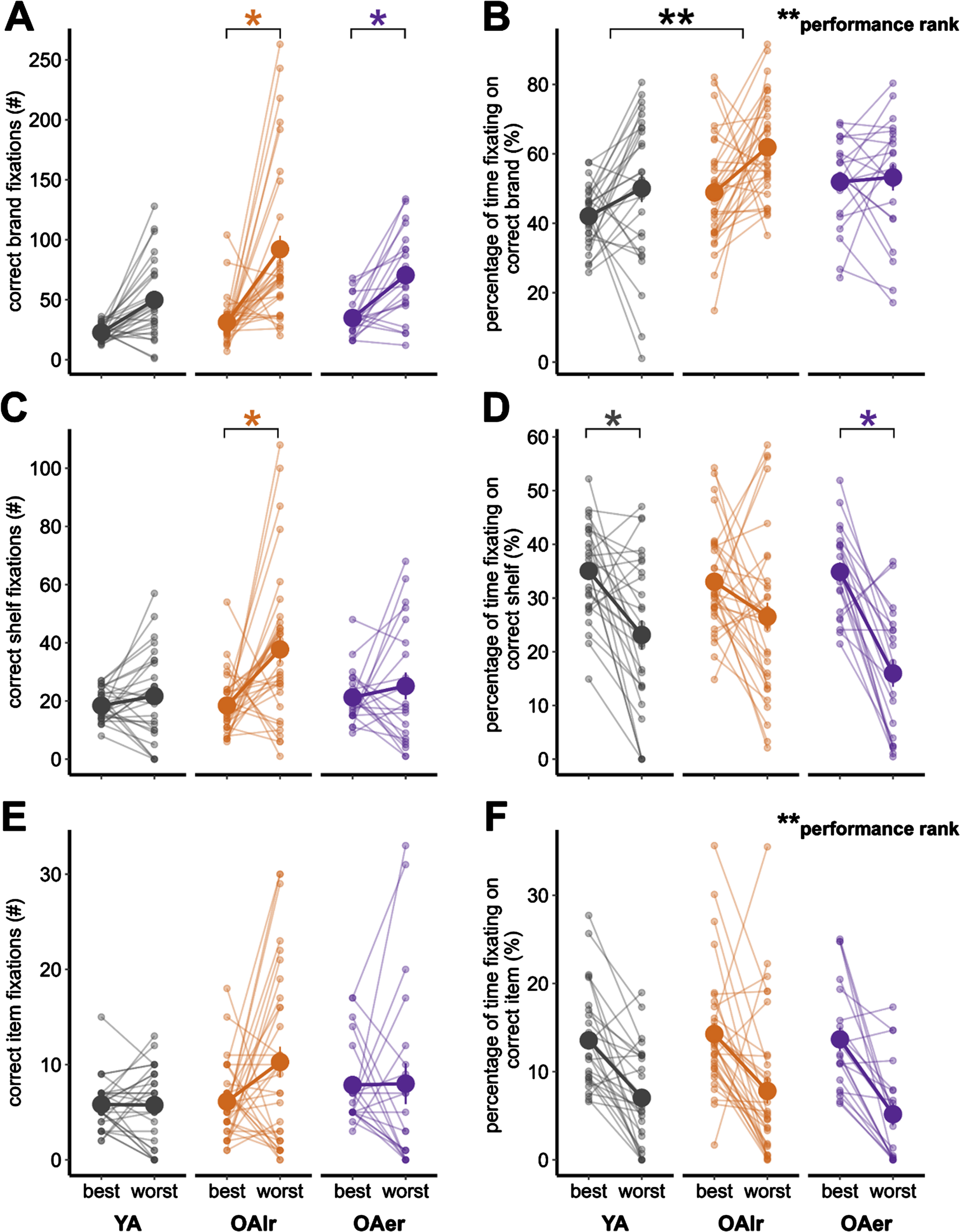
There was a significant main effect of group on percentage of time spent fixating on the correct brand (Table 3). Follow-up tests revealed that OAlr spent a greater percentage of time fixating on the correct brand than did YA (B = 9%, p = 0.011) (Fig. 3B). There were significant main effects of performance rank on percentage of time fixating on the correct brand, selected item, and correct item (Table 3). Follow-up tests revealed that participants spent a greater percentage of time fixating on the correct brand (Fig. 3B), but a smaller percentage of time fixating on the correct items (Fig. 3F) and selected items (Fig. 2G) during their worst performances relative to their best (correct brand, B = 7%, p = 0.003; correct item, B = –7%, p < 0.001; selected item, B = –4%, p < 0.001).
The results that did not survive the FDR corrections were the interactions between group and performance rank on the number of fixations on the correct brand, the number of fixations on the selected item, and the percentage of time fixating on the correct shelf. The following results remained significant: the main effects of group on the number of correct grocery items selected and on the percentage of time spent fixating on the correct brand; the main effects of performance rank on the percentage of time fixating on the correct brand, selected item, and correct item; and the interaction between group and performance rank on trial time, the number of fixations on the correct shelving unit, the percentage of time fixating on the correct shelving unit, and the number of fixations on the correct shelf. Figures with FDR-corrected significance indicated are provided as Supplementary Figures 2 and 3.
Effect of Aβ deposition in OA on grocery shopping performance
The omnibus statistics are provided in Table 4. Significant interactions and main effects are shown in Figs. 4 and 5. There was not a significant effect of Aβ deposition on the number of correctly selected grocery items in OA (Fig. 4A). There were significant interactions between Aβ deposition and performance rank in the percentage of time fixating on the correct shelving unit, the correct brand, and the correct shelf (Table 4). Follow-up tests revealed that greater Aβ deposition was associated with a decrease in the percentage of time fixating on the correct shelving unit during OA’s worst performances (B = –12.9% per 1 PiB SUVR, p = 0.016) (Fig. 4E). Similarly, follow-up tests revealed that during OA’s worst performances greater Aβ deposition was linked to a decrease in the percentage of time fixating on the correct brand (B = –13. 1% per 1 PiB SUVR, p = 0.043) (Fig. 5B), and on the correct shelf (B = –11.7% per 1 PiB SUVR, p = 0.028) (Fig. 5D). Aβ deposition did not relate to the percentage of time fixating on the correct shelving unit, brand, or shelf during OA’s best performances.
Fig. 4
Overall grocery shopping performance, fixations on the correct shelving unit, and fixations on the selected item during OA’s best and worst performances as a function of Aβ deposition. Significant interactions between Aβ deposition and performance rank are indicated by a colored asterisk(s) for the respective best or worst performance. A main effect of group is indicated by a black asterisk(s) and the words “Aβ deposition” in the top right of the plot. A main effect of performance rank is indicated by a black asterisk(s) and the words “performance rank” in the top right of the plot. Level of significance is indicated as follows: ***p<0.001, **p<0.01, *p<0.05.
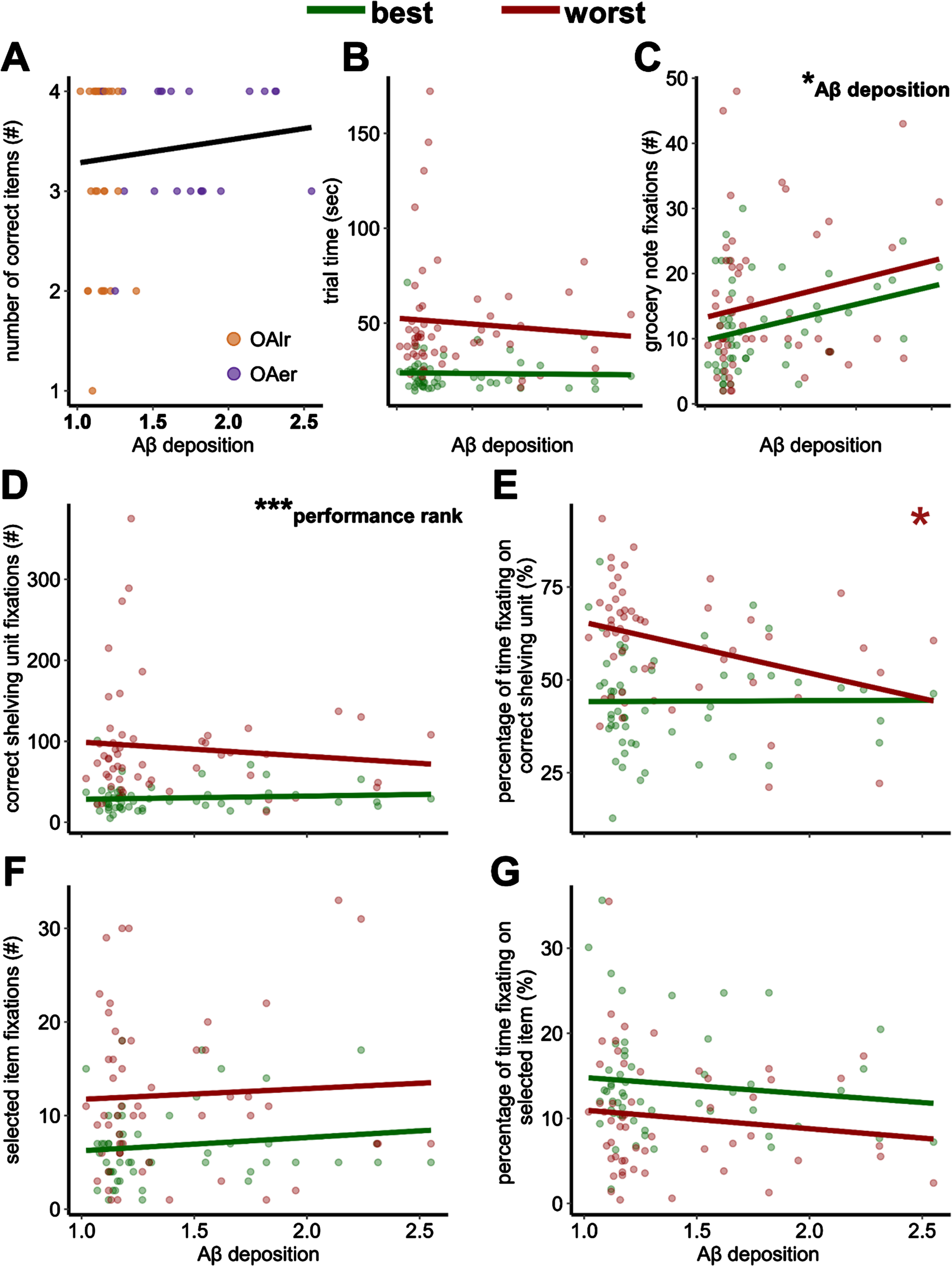
Fig. 5
Fixations on the correct brand, shelf, and item during OA’s best and worst grocery shopping performances as a function of Aβ deposition. Significant interactions between Aβ deposition and performance rank are indicated by a colored asterisk(s) for the respective best or worst performance. A main effect of group is indicated by a black asterisk(s) and the words “Aβ deposition” in the top right of the plot. A main effect of performance rank is indicated by a black asterisk(s) and the words “performance rank” in the top right of the plot. Level of significance is indicated as follows: ***p<0.001, **p<0.01, *p<0.05.
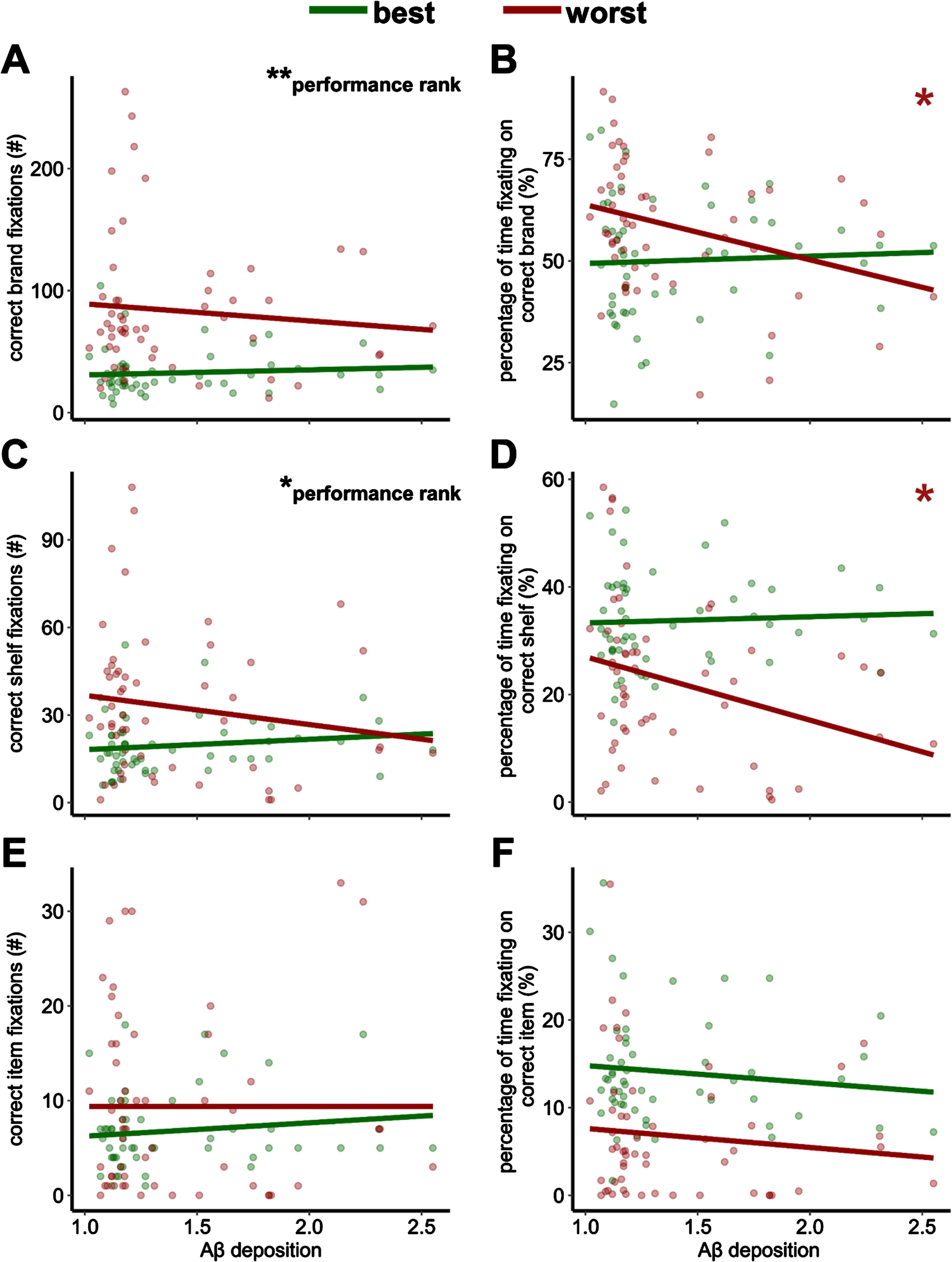
Table 4
Effect of Aβ deposition in OA on grocery shopping performance measures
Aβ | Rank | Aβ*rank | Time between visits | Education | |||||||||
F | p | B (SE) | F | p | F | p | F | p | B (SE) | F | p | B (SE) | |
Number of correct items | 0.603 | 0.441 | 0.236 (0.300) | — | — | 0.040 | 0.843 | 0.002 (0.015) | 0.079 | 0.780 | –0.012 (0.041) | ||
Trial time | 0.204 | 0.655 | –2.588 (8.046) | 3.370 | 0.086 | 0.005 | 0.942 | 0.561 | 0.459 | 0.235 (0.313) | 0.005 | 0.944 | –0.063 (0.895) |
Number of grocery note fixations | 5.332 | 0.025 | 5.833 (3.187) | 0.275 | 0.603 | 0.009 | 0.925 | 0.963 | 0.331 | 0.121 (0.123) | 2.431 | 0.125 | –0.557 (0.357) |
Number of correct shelving unit fixations | 0.155 | 0.694 | 5.957 (17.667) | 6.736 | 0.011 | 0.777 | 0.381 | 0.992 | 0.322 | 0.611 (0.614) | 0.010 | 0.921 | –0.171 (1.724) |
Percentage of time fixating on correct shelving unit | 1.936 | 0.168 | 2.756 (4.835) | 16.759 | <0.001 | 6.135 | 0.016 | 0.441 | 0.508 | 0.116 (0.175) | 0.105 | 0.747 | 0.162 (0.499) |
Number of selected item fixations | 0.676 | 0.415 | 1.600 (2.343) | 1.584 | 0.214 | 0.011 | 0.917 | 0.076 | 0.784 | 0.023 (0.084) | 0.429 | 0.515 | 0.156 (0.239) |
Percentage of time fixating on selected item | 1.252 | 0.267 | –2.228 (2.344) | 1.224 | 0.273 | 0.034 | 0.855 | 0.004 | 0.950 | 0.005 (0.083) | 0.252 | 0.617 | 0.119 (0.237) |
Number of correct brand fixations | 0.138 | 0.711 | 5.149 (14.752) | 6.507 | 0.012 | 0.758 | 0.386 | 0.518 | 0.473 | 0.368 (0.512) | 0.149 | 0.700 | –0.554 (1.434) |
Percentage of time fixating on correct brand | 2.017 | 0.161 | 1.961 (5.337) | 7.850 | 0.007 | 4.309 | 0.043 | 0.102 | 0.751 | 0.061 (0.190) | 0.0002 | 0.999 | –0.007 (0.537) |
Number of correct shelf fixations | 0.353 | 0.554 | 3.967 (6.666) | 5.659 | 0.019 | 2.087 | 0.151 | 0.368 | 0.545 | 0.140 (0.231) | 0.716 | 0.399 | 0.548 (0.648) |
Percentage of time fixating on correct shelf | 3.234 | 0.076 | 1.219 (4.105) | 0.643 | 0.425 | 5.040 | 0.028 | 0.0002 | 0.999 | –0.002 (0.143) | 4.748 | 0.033 | 0.871 (0.400) |
Number of correct item fixations | 0.196 | 0.659 | 1.518 (2.596) | 0.743 | 0.392 | 0.147 | 0.703 | 0.153 | 0.697 | 0.035 (0.090) | 1.535 | 0.220 | 0.315 (0.254) |
Percentage of time fixating on correct item | 0.927 | 0.340 | –2.043 (2.435) | 3.269 | 0.076 | 0.024 | 0.878 | 0.203 | 0.654 | 0.041 (0.090) | 1.876 | 0.177 | 0.351 (0.256) |
There was a significant main effect of Aβ deposition on the number of grocery note fixations (Table 4). Follow-up tests revealed that greater Aβ deposition was associated with an increase in fixations on the grocery note (B = 5.8% per 1 PiB SUVR, p = 0.025) (Fig. 4C). There were significant main effects of performance rank on the number of fixations on the correct shelving unit, brand, and shelf (Table 4). Follow-up tests revealed that during their worst performances, OA fixated more times on the correct shelving unit (B = 62 fixations, p < 0.001) (Fig. 4D), the correct brand (B = 51 fixations, p < 0.001) (Fig. 5A), and the correct shelf (B = 13.3 fixations, p < 0.001) (Fig. 5C). Finally, the number of years of education was a significant covariate of the percentage of time fixating on the correct shelf (B = 0.9%, p = 0.033).
The results that did not survive the FDR corrections were the main effect of Aβ deposition on the number of grocery note fixations, the main effect of performance rank on the number of fixations on the correct shelf, and the interactions between Aβ deposition and performance rank on the percentage of time fixating on the correct brand and the correct shelf. The following results remained significant: the main effects of performance rank on the number of fixations on the correct shelving unit and the correct brand, and the interaction between Aβ deposition and performance rank on the percentage of time fixating on the correct shelving unit. Figures with FDR-corrected significance indicated are provided as Supplementary Figures 4 and 5.
DISCUSSION
The purpose of this study was to identify if grocery shopping performance could differentiate OAer, OAlr, and YA, as well as if grocery shopping performance could be predicted by Aβ deposition in OA. This study has three main findings. The first main finding is that both OA groups exhibited age-related declines in efficiency of search strategies, relative to YA, during their worst grocery shopping performances, and OAer may have failed to discern the shelf organization as well as OAlr did. The second main finding is that greater Aβ deposition in OA was associated with less efficient search strategies during the worst grocery shopping performance. The third main finding is that greater Aβ deposition in OA was associated with evidence of poor working memory during both the best and worst grocery shopping performances.
In partial support of our hypothesis, in comparison to YA, both OA groups exhibited age-related deficits in efficiency of search strategies during their worst grocery shopping performances, relative to their best. Although the results did not confirm the hypothesized trend in fixation patterns across groups (i.e., a progressively greater number of fixations on the grocery list and relevant grocery items and a smaller percentage of the total trial time fixating on relevant grocery items from YA to OAlr to OAer across the best and worst performances), the differences exhibited by both OA groups from their best to worst performances indicate deficits in search strategy efficiency that were not similarly exhibited by YA. More specifically, in comparison to their best performance, both the OAer and OAlr groups exhibited a greater number of fixations on the correct shelving unit and on the correct brand during their worst performance, while YA did not exhibit a different number of fixations on either of these AOIs between their best and worst performances. These results are in agreement with the findings of previous studies that determined OA exhibit a greater number of fixations than YA during driving-related visual search tasks [50–52]. Because the number of fixations during a search task is an indicator of how challenging the task is for an individual [50], the increased number of fixations exhibited by the OA groups from their best to their worst performances, relative to no differences exhibited by YA, suggests that both OA groups were more challenged than YA when trying to find certain grocery items and exhibited age-related declines in search efficiency. OA may have chosen a less efficient visual search process or failed to extract the maximum amount of information possible from each fixation due to a decrease in the size of the useful field of view with increasing age [52, 53].
Additionally in partial support of our hypothesis, OAer may have failed to discern the shelf organization as well as OAlr did, as exemplified by differences from the best to the worst performances. While all three groups increased the percentage of time that they fixated on the correct brand during their worst, relative to their best performance, OAlr increased their time more than YA did (13% and 8%, respectively), and OAer only negligibly increased their time (increased by 1%). Further, relative to their best performance, OAer exhibited a longer trial time but decreased the percentage of time that they fixated on the correct shelf during their worst trial, while OAlr also exhibited a longer trial time but did not exhibit a change in percentage of time fixating on the correct shelf and increased the number of fixations on the correct shelf between their best and worst performances. These results provide evidence that OAer may have failed to discern the shelf organization as well as OAlr did. Although OAlr were inefficient in their search and maybe brought back the wrong item during their worst performance, they seem to have picked up on the shelf organization and zeroed in on the correct shelf, spending much of the trial time at least in the correct vicinity of the correct item when searching for a harder to find grocery item. In contrast, OAer only negligibly increased the percentage of time that they fixated on the correct brand and spent a smaller percentage of time fixating on the correct shelf, suggesting that they not only searched inefficiently when searching for a harder to find grocery item but also potentially failed to discern the organization of the grocery shelves and spent much of the trial time not scanning in the vicinity of the correct item. These interpretations are supported by previous research that determined, relative to healthy OA controls, those with AD fixated on salient visual cues less often and on non-salient visual cues more often during a wayfinding virtual reality, spatial task [54] and exhibited less focused visual exploration and fewer fixations on informative regions during a clock reading task [55]. Further, a recent study established that from healthy controls to those with MCI to those with dementia due to AD, each group, respectively, spent more time looking at distractors than target stimuli during a visual search task, exhibiting reduced search efficiency as cognitive impairment increased [56]. Thus, subtle deficits in visual search efficiency, similar to those exhibited by individuals with MCI and dementia with an etiology of AD due to a decline in visuospatial attention as a result of Aβ deposits in the visual system of the brain [56], are likely present in those at elevated risk of developing AD due to Aβ deposition.
In further support of Aβ deposition impacting grocery shopping performance and consistent with our hypothesis, in the OA analysis, greater Aβ deposition was associated with increasingly less efficient search strategies during the worst grocery shopping performance. Specifically, during an OA’s worst performance, greater Aβ deposition predicted a smaller percentage of time spent fixating on the correct shelving unit, the correct brand, and the correct shelf. Despite less relative time spent fixating on the correct region of the prespecified grocery item during their worst performance, all OA, regardless of Aβ deposition, exhibited a greater number of fixations on those same AOIs during their worst, relative to their best, performance. Further, the trial time did not differ between the two performances in OA. The fact that OA with greater Aβ deposition spent a smaller percentage of time fixating on these AOIs with a greater number of fixations and no difference in trial time indicates that their average fixation duration on these AOIs was likely shorter during their worst performance than was the average fixation duration of OA with lower Aβ deposition. These findings are in agreement with previous research that observed those with dementia with an etiology of AD to exhibit shorter gaze durations than healthy OAs during a visuospatial working memory task, especially during the encoding portion of the task [21]. These results suggest that with increasing Aβ deposition in the current study, these OA may exhibit an increasing encoding impairment, leading to an inefficient search for a prespecified grocery item. More specifically, these results suggest that as Aβ deposition increases, OA may exhibit increasingly poor encoding due to impaired feature-binding [23], causing them to have difficulty with accurately perceiving and remembering the unique characteristics of each grocery item viewed over time. Indeed, previous research has provided evidence that those with MCI and those at risk of MCI exhibit impaired feature-binding, similar to that exhibited by those with dementia due to AD [56, 57]. Thus, it is likely that OA with greater Aβ deposition may also exhibit impaired feature-binding. Interestingly, years of formal education completed was a significant covariate of the percentage of time fixating on the correct shelf, with increasing years of education associated with increasing percentages of time fixating on the correct shelf. These results suggest that education may enable OA to compensate for impairments associated with increasing Aβ deposition and lengthen the time between pathological Aβ deposition and dementia diagnosis with confirmed AD, in agreement with previous research that determined education can play a protective role in the clinical trajectory that precedes a dementia diagnosis with AD etiology [10].
Additionally in the OA analysis, greater Aβ deposition was associated with evidence of increasingly poor working memory during the best and worst grocery shopping trials. Specifically, during the best and worst trials, greater Aβ deposition predicted a greater number of fixations on the grocery note, suggesting poor working memory and encoding of the words written on the notecard. This interpretation is supported by previous research that determined those with MCI at elevated risk of developing AD exhibited poor recognition memory performance during a visual comparison task, relative to healthy controls, and relatedly exhibited impaired immediate working memory for verbal information on a standardized neuropsychological assessment [58]. Despite an association with a likely working memory impairment, Aβ deposition did not impact the number of correct items or trial time, in contrast with previous research that determined those with MCI took a longer time to complete and made more mistakes during a virtual grocery shopping task than healthy controls [28]. This contrast in results suggests that cognitively normal OA with elevated Aβ deposition may be able to compensate for their impaired working memory. In agreement with this interpretation, previous research determined that individuals with early-onset dementia and probable AD exhibited a greater number of fixations on two target locations during a visuospatial and visuoconstructional functioning task and switched their focus from one target location to the other a greater number of times than did healthy OA controls or individuals with late-onset dementia and probable AD [59]. The differences between individuals with early- and late-onset dementia and probable AD occurred despite the fact that these two groups achieved similar scores on the task [59]. The authors of this study interpreted these results as evidence of those with early-onset dementia and probable AD having more significant working memory deficits than the healthy OAs or those with late-onset dementia and probable AD but that those with early-onset dementia and probable AD may have compensated for their impaired working memory by studying the figure in the task more often to achieve a similar score as those with late-onset dementia and probable AD [59]. Similarly, in the current study, Aβ deposition did not affect overall performance on the grocery shopping trials, as evidenced by no impact of Aβ deposition on the number of correct items selected in the four grocery shopping trials or the elapsed time to select a can in the best or worst performance, even though those with greater Aβ deposition had to fixate more often on the grocery notecard to achieve a performance similar to those with lower Aβ deposition. Thus, subtle working memory impairments and less efficient search strategies in cognitively normal OA with elevated Aβ deposition may be a precursor to more evident grocery shopping performance deficits observed in those who progress to MCI and eventually dementia with an etiology of AD.
This study has some limitations to acknowledge. First, we did not conduct a power calculation prior to data collection. The current study was added as a supplemental visit to the larger B-NET study only after incidence of elevated Aβ deposition was discovered within our participant cohort. Therefore, the decision to include the analyses in the current study was driven by the desire to thoroughly investigate the observed elevated risk of developing AD and was not guided by a formal power calculation. The study sample size was relatively small in comparison to the number of outcome measures included in the analyses. This relatively large number of outcome measures was necessary, however, to fully account for performance on the ecologically valid and challenging version of a grocery shopping task utilized in this study. Similarly, to control for Type I errors, we utilized a FDR correction for multiple hypothesis testing, using the Benjamini-Hochberg procedure, but still reported and interpreted the results that were no longer significant after the FDR correction; this approach was chosen due to the exploratory and hypothesis-generating aspects of the novel grocery shopping task, allowing us to generate new research questions more liberally within the context of this novel task. However, it is still crucial to recognize that the findings related to our specific hypotheses should be interpreted with caution and considered preliminary. Future research with a confirmatory approach and more rigorous error control measures is needed to extend and validate the conclusions drawn from this initial study. Additionally, the novel experimental design of tracking eye movements during an ecologically valid performance of a complex, everyday task and the unique study sample of OA at both elevated and low risk of developing AD, based on Aβ deposition, make this study an important initial exploration of how objective quantification of the performance of complex, everyday tasks could be used to assess Aβ deposition in an OA and its impact on the coordination of multiple cognitive domains. Future studies should include a larger sample size. Finally, the COVID-19 pandemic posed challenges for performing the PET scans and extra study visit on a set schedule. We did not expect this timing difference across participants to impact any of the results because all OA were determined to be without cognitive impairment at the start of the parent study, they completed the extra study visit within 24 months of their PET scan, and Aβ deposition occurs slowly, approximately at the rate of -0.02 to +0.07 PiB SUVR per year in OA who are Aβ- or Aβ+ but without cognitive impairment [60]. However, the timing difference was included as a covariate in the OA analysis in the second aim to account for any potential impact of the timing differences across participants. Notably, this timing difference covariate was not significant in any of the analyses.
Conclusions
This study aimed to determine if eye movements during grocery shopping performance could differentiate OAer, OAlr, and YA, as well as be predicted by Aβ deposition in OA. Both OA groups exhibited age-related deficits in search efficiency, relative to YA, but OAer may have failed to discern the shelf organization as well as OAlr did. Relatedly, increasing Aβ deposition in OA was predictive of less efficient search strategies and evidence of poor working memory. Thus, OA with elevated Aβ deposition may exhibit subtle working memory impairments and less efficient visual search strategies that can be observed during the performance of a complex and cognitively demanding everyday task in the preclinical phase of AD. Eye movements during grocery shopping performance should be further explored as a potential screening tool for elevated risk of developing AD, with a specific focus on identifying and prioritizing the most influential variables among those presented in our initial analysis.
AUTHOR CONTRIBUTIONS
Lisa A. Zukowski (Conceptualization; Funding acquisition; Investigation; Methodology; Project administration; Resources; Writing – original draft); Sarah A. Brinkerhoff (Formal analysis; Visualization; Writing – original draft); Ilana Levin (Data curation; Investigation; Writing – review & editing); Troy M. Herter (Methodology; Resources; Writing – review & editing); Lena Hetrick (Data curation; Investigation; Writing – review & editing); Samuel N. Lockhart (Investigation; Methodology; Resources; Writing – review & editing); Michael E. Miller (Data curation; Formal analysis; Writing – review & editing); Paul J. Laurienti (Conceptualization; Funding acquisition; Project administration; Writing – review & editing); Stephen B. Kritchevsky (Conceptualization; Funding acquisition; Project administration; Writing – review & editing); Christina E. Hugenschmidt (Conceptualization; Funding acquisition; Methodology; Writing – review & editing).
ACKNOWLEDGMENTS
The authors have no acknowledgments to report.
FUNDING
This project was supported by the National Institutes of Health, through an Administrative Supplement funded through B-NET (R01-AG052419), the Wake Forest University Claude D. Pepper Older Americans Independence Center (P30-AG21332). and the Wake Forest Clinical and Translational Science Institute (UL1-TR001420).
CONFLICT OF INTEREST
The authors have no conflict of interest to report.
DATA AVAILABILITY
The data supporting the findings of this study are available on request from the corresponding author.
SUPPLEMENTARY MATERIAL
[1] The supplementary material is available in the electronic version of this article: https://dx.doi.org/10.3233/JAD-231108.
REFERENCES
[1] | Dubbelman MA , Mimmack KJ , Sprague EH , Amariglio RE , Vannini P , Marshall GA , for the Alzheimer’s Disease Neuroimaging Initiative ((2023) ) Regional cerebral tau predicts decline in everyday functioning across the Alzheimer’s disease spectrum. Alzheimers Res Ther 15: , 120. |
[2] | Colucci L , Bosco M , Fasanaro AM , Gaeta GL , Ricci G , Amenta F ((2014) ) Alzheimer’s disease costs: What we know and what we should take into account. J Alzheimers Dis 42: , 1311–1324. |
[3] | Cotrell V , Hooker K ((2005) ) Possible selves of individuals with Alzheimer’s disease. Psychol Aging 20: , 285–294. |
[4] | Braak H , Thal DR , Ghebremedhin E , Del Tredici K ((2011) ) Stages of the pathologic process in Alzheimer disease: Age categories from 1 to 100 years. J Neuropathol Exp Neurol 70: , 960–969. |
[5] | Jack CR , Knopman DS , Jagust WJ , Petersen RC , Weiner MW , Aisen PS , Shaw LM , Vemuri P , Wiste HJ , Weigand SD , Lesnick TG , Pankratz VS , Donohue MC , Trojanowski JQ ((2013) ) Tracking pathophysiological processes in Alzheimer’s disease: An updated hypothetical model of dynamic biomarkers. Lancet Neurol 12: , 207–216. |
[6] | Cedazo-Minguez A , Winblad B ((2010) ) Biomarkers for Alzheimer’s disease and other forms of dementia: Clinical needs, limitations and future aspects. Exp Gerontol 45: , 5–14. |
[7] | Dubois B , Villain N , Frisoni GB , Rabinovici GD , Sabbagh M , Cappa S , Bejanin A , Bombois S , Epelbaum S , Teichmann M , Habert M-O , Nordberg A , Blennow K , Galasko D , Stern Y , Rowe CC , Salloway S , Schneider LS , Cummings JL , Feldman HH ((2021) ) Clinical diagnosis of Alzheimer’s disease: Recommendations of the International Working Group. Lancet Neurol 20: , 484–496. |
[8] | Porsteinsson AP , Isaacson RS , Knox S , Sabbagh MN , Rubino I ((2021) ) Diagnosis of early Alzheimer’s disease: Clinical practice in 2021. J Prev Alzheimers Dis 8: , 371–386. |
[9] | Villemagne VL , Burnham S , Bourgeat P , Brown B , Ellis KA , Salvado O , Szoeke C , Macaulay SL , Martins R , Maruff P , Ames D , Rowe CC , Masters CL , Australian Imaging Biomarkers and Lifestyle (AIBL) Research Group ((2013) ) Amyloid β deposition, neurodegeneration, and cognitive decline in sporadic Alzheimer’s disease: A prospective cohort study. Lancet Neurol 12: , 357–367. |
[10] | Amieva H , Mokri H , Le Goff M , Meillon C , Jacqmin-Gadda H , Foubert-Samier A , Orgogozo J-M , Stern Y , Dartigues J-F ((2014) ) Compensatory mechanisms in higher-educated subjects with Alzheimer’s disease: A study of 20 years of cognitive decline. Brain 137: , 1167–1175. |
[11] | Buracchio T , Dodge HH , Howieson D , Wasserman D , Kaye J ((2010) ) The trajectory of gait speed preceding mild cognitive impairment. Arch Neurol 67: , 980–986. |
[12] | Fitzpatrick AL , Buchanan CK , Nahin RL , Dekosky ST , Atkinson HH , Carlson MC , Williamson JD , Ginkgo Evaluation of Memory (GEM) Study Investigators ((2007) ) Associations of gait speed and other measures of physical function with cognition in a healthy cohort of elderly persons. J Gerontol A Biol Sci Med Sci 62: , 1244–1251. |
[13] | Nadkarni NK , Nunley KA , Aizenstein H , Harris TB , Yaffe K , Satterfield S , Newman AB , Rosano C , Health ABC Study ((2014) ) Association between cerebellar gray matter volumes, gait speed, and information-processing ability in older adults enrolled in the Health ABC study. J Gerontol A Biol Sci Med Sci 69: , 996–1003. |
[14] | Verghese J , Wang C , Lipton RB , Holtzer R ((2013) ) Motoric cognitive risk syndrome and the risk of dementia. J Gerontol A Biol Sci Med Sci 68: , 412–418. |
[15] | Verghese J , Annweiler C , Ayers E , Barzilai N , Beauchet O , Bennett DA , Bridenbaugh SA , Buchman AS , Callisaya ML , Camicioli R , Capistrant B , Chatterji S , De Cock A-M , Ferrucci L , Giladi N , Guralnik JM , Hausdorff JM , Holtzer R , Kim KW , Kowal P , Kressig RW , Lim J-Y , Lord S , Meguro K , Montero-Odasso M , Muir-Hunter SW , Noone ML , Rochester L , Srikanth V , Wang C ((2014) ) Motoric cognitive risk syndrome: Multicountry prevalence and dementia risk. Neurology 83: , 718–726. |
[16] | Watson NL , Rosano C , Boudreau RM , Simonsick EM , Ferrucci L , Sutton-Tyrrell K , Hardy SE , Atkinson HH , Yaffe K , Satterfield S , Harris TB , Newman AB , Health ABC Study ((2010) ) Executive function, memory, and gait speed decline in well-functioning older adults. J Gerontol A Biol Sci Med Sci 65: , 1093–1100. |
[17] | Graf C ((2008) ) How to Try This: The Lawton Instrumental Activities of Daily Living Scale. Am J Nurs 108: , 52–63. |
[18] | Meghanathan RN , Van Leeuwen C , Nikolaev AR ((2015) ) Fixation duration surpasses pupil size as a measure of memory load in free viewing. Front Hum Neurosci 8: , 1063. |
[19] | Laukka EJ , Macdonald SWS , Fratiglioni L , Bäckman L ((2012) ) Preclinical cognitive trajectories differ for Alzheimer’s disease and vascular dementia. J Int Neuropsychol Soc JINS 18: , 191–199. |
[20] | Fernández G , Parra MA ((2021) ) Oculomotor behaviors and integrative memory functions in the Alzheimer’s clinical syndrome. J Alzheimers Dis 82: , 1033–1044. |
[21] | Fernández G , Orozco D , Agamennoni O , Schumacher M , Sañudo S , Biondi J , Parra MA ((2018) ) Visual processing during short-term memory binding in mild Alzheimer’s disease. J Alzheimers Dis 63: , 185–194. |
[22] | Ritchie K , Carrière I , Howett D , Su L , Hornberger M , O’Brien JT , Ritchie CW , Chan D ((2018) ) Allocentric and egocentric spatial processing in middle-aged adults at high risk of late-onset Alzheimer’s disease: The PREVENT Dementia Study. J Alzheimers Dis 65: , 885–896. |
[23] | Parra MA , Sala SD , Abrahams S , Logie RH , Méndez LG , Lopera F ((2011) ) Specific deficit of colour-colour short-term memory binding in sporadic and familial Alzheimer’s disease. Neuropsychologia 49: , 1943–1952. |
[24] | Bharti AK , Yadav SK , Jaswal S ((2020) ) Feature binding of sequentially presented stimuli in visual working memory. Front Psychol 11: , 33. |
[25] | Howett D , Castegnaro A , Krzywicka K , Hagman J , Marchment D , Henson R , Rio M , King JA , Burgess N , Chan D ((2019) ) Differentiation of mild cognitive impairment using an entorhinal cortex-based test of virtual reality navigation. Brain 142: , 1751–1766. |
[26] | Neyland BR , Hugenschmidt CE , Lyday RG , Burdette JH , Baker LD , Rejeski WJ , Miller ME , Kritchevsky SB , Laurienti PJ ((2021) ) Effects of a motor imagery task on functional brain network community structure in older adults: Data from the Brain Networks and Mobility Function (B-NET) Study. Brain Sci 11: , 118. |
[27] | Porffy LA , Mehta MA , Patchitt J , Boussebaa C , Brett J , D’Oliveira T , Mouchlianitis E , Shergill SS ((2022) ) A novel virtual reality assessment of functional cognition: Validation study. J Med Internet Res 24: , e27641. |
[28] | Zygouris S , Giakoumis D , Votis K , Doumpoulakis S , Ntovas K , Segkouli S , Karagiannidis C , Tzovaras D , Tsolaki M ((2015) ) Can a virtual reality cognitive training application fulfill a dual role? Using the virtual supermarket cognitive training application as a screening tool for mild cognitive impairment. J Alzheimers Dis 44: , 1333–1347. |
[29] | Carlesimo GA , Fadda L , Lorusso S , Caltagirone C ((2009) ) Verbal and spatial memory spans in Alzheimer’s and multi-infarct dementia. Acta Neurol Scand 89: , 132–138. |
[30] | Schneider-Garces NJ , Gordon BA , Brumback-Peltz CR , Shin E , Lee Y , Sutton BP , Maclin EL , Gratton G , Fabiani M ((2010) ) Span, CRUNCH, and beyond: Working memory capacity and the aging brain. J Cogn Neurosci 22: , 655–669. |
[31] | Carvalho D , Bessa M , Oliveira L , Guedes C , Peres E , Magalhães L ((2012) ) New interaction paradigms to fight the digital divide: A pilot case study regarding multi-touch technology. Procedia Comput Sci 14: , 128–137. |
[32] | Palmer BW , Heaton RK , Paulsen JS , Kuck J , Braff D , Harris MJ , Zisook S , Jeste DV ((1997) ) Is it possible to be schizophrenic yet neuropsychologically normal? Neuropsychology 11: , 437–446. |
[33] | Aubin G , Béliveau M-F , Klinger E ((2018) ) An exploration of the ecological validity of the Virtual Action Planning-Supermarket (VAP-S) with people with schizophrenia. Neuropsychol Rehabil 28: , 689–708. |
[34] | Larøi F , Canlaire J , Mourad H , Van Der Linden M ((2010) ) Relations between a computerized shopping task and cognitive tests in a group of persons diagnosed with schizophrenia compared with healthy controls. J Int Neuropsychol Soc 16: , 180–189. |
[35] | Laurienti PJ , Miller ME , Lyday RG , Boyd MC , Tanase AD , Burdette JH , Hugenschmidt CE , Rejeski WJ , Simpson SL , Baker LD , Tomlinson CE , Kritchevsky SB ((2023) ) Associations of physical function and body mass index with functional brain networks in community-dwelling older adults. Neurobiol Aging 127: , 43–53. |
[36] | Klunk WE , Engler H , Nordberg A , Wang Y , Blomqvist G , Holt DP , Bergström M , Savitcheva I , Huang G-F , Estrada S , Ausén B , Debnath ML , Barletta J , Price JC , Sandell J , Lopresti BJ , Wall A , Koivisto P , Antoni G , Mathis CA , Långström B ((2004) ) Imaging brain amyloid in Alzheimer’s disease with Pittsburgh Compound-B: Imaging Amyloid in AD with PIB. Ann Neurol 55: , 306–319. |
[37] | Mormino EC , Brandel MG , Madison CM , Rabinovici GD , Marks S , Baker SL , Jagust WJ ((2012) ) Not quite PIB-positive, not quite PIB-negative: Slight PIB elevations in elderly normal control subjects are biologically relevant. NeuroImage 59: , 1152–1160. |
[38] | Maass A , Lockhart SN , Harrison TM , Bell RK , Mellinger T , Swinnerton K , Baker SL , Rabinovici GD , Jagust WJ ((2018) ) Entorhinal tau pathology, episodic memory decline, and neurodegeneration in aging. J Neurosci 38: , 530–543. |
[39] | Collij LE , Salvadó G , Shekari M , Lopes Alves I , Reimand J , Wink AM , Zwan M , Niñerola-Baizán A , Perissinotti A , Scheltens P , Ikonomovic MD , Smith APL , Farrar G , Molinuevo JL , Barkhof F , Buckley CJ , van Berckel BNM , Gispert JD , ALFA study, AMYPAD consortium ((2021) ) Visual assessment of [18F]flutemetamol PET images can detect early amyloid pathology and grade its extent. Eur J Nucl Med Mol Imaging 48: , 2169–2182. |
[40] | Wechsler D ((2008) ) Wechsler Adult Intelligence Scale, Pearson Assessment, San Antonio, TX. |
[41] | Reynolds CR ((2020) ) Comprehensive trail making test (CTMT), PRO-ED, Inc., Austin, TX. |
[42] | Simonsick EM , Newman AB , Nevitt MC , Kritchevsky SB , Ferrucci L , Guralnik JM , Harris T , for the Health ABC Study Group ((2001) ) Measuring higher level physical function in well-functioning older adults: Expanding familiar approaches in the Health ABC Study. J Gerontol A Biol Sci Med Sci 56: , M644–M649. |
[43] | Washburn RA , McAuley E , Katula J , Mihalko SL , Boileau RA ((1999) ) The physical activity scale for the elderly (PASE): Evidence for validity. J Clin Epidemiol 52: , 643–651. |
[44] | Powell LE , Myers AM ((1995) ) The Activities-specific Balance Confidence (ABC) Scale. J Gerontol A Biol Sci Med Sci 50A: , M28–M34. |
[45] | Rejeski WJ , Marsh AP , Anton S , Chen S-H , Church T , Gill TM , Guralnik JM , Glynn NW , King AC , Rushing J , Ip EH , for the LIFE Research Group ((2013) ) The MAT-sf: Clinical relevance and validity. J Gerontol A Biol Sci Med Sci 68: , 1567–1574. |
[46] | Salthouse TA , Ellis CL ((1980) ) Determinants of eye-fixation duration. Am J Psychol 93: , 207–234. |
[47] | Singh T , Perry CM , Herter TM ((2016) ) A geometric method for computing ocular kinematics and classifying gaze events using monocular remote eye tracking in a robotic environment. J Neuroengineering Rehabil 13: , 10. |
[48] | Wickham H , Averick M , Bryan J , Chang W , McGowan L , François R , Grolemund G , Hayes A , Henry L , Hester J , Kuhn M , Pedersen T , Miller E , Bache S , Müller K , Ooms J , Robinson D , Seidel D , Spinu V , Takahashi K , Vaughan D , Wilke C , Woo K , Yutani H ((2019) ) Welcome to the Tidyverse. J Open Source Softw 4: , 1686. |
[49] | Bates D , Maechler M , Bolker B , Walker S ((2015) ) Fitting Linear mixed-effects models using lme4. J Stat Softw 67: , 1–48. |
[50] | McPhee LC , Scialfa CT , Dennis WM , Ho G , Caird JK ((2004) ) Age differences in visual search for traffic signs during a simulated conversation. Hum Factors 46: , 674–685. |
[51] | Lavallière M , Tremblay M , Cantin V , Simoneau M , Teasdale N ((2006) ) Aging yields a smaller number of fixations and a reduced gaze amplitude when driving in a simulator. Adv Transp Stud, pp. 21–30. |
[52] | Maltz M , Shinar D ((1999) ) Eye movements of younger and older drivers. Hum Factors 41: , 15–25. |
[53] | Ball KK , Beard BL , Roenker DL , Miller RL , Griggs DS ((1988) ) Age and visual search: Expanding the useful field of view. J Opt Soc Am A 5: , 2210–2219. |
[54] | Davis R , Sikorskii A ((2020) ) Eye tracking analysis of visual cues during wayfinding in early stage Alzheimer’s disease. Dement Geriatr Cogn Disord 49: , 91–97. |
[55] | Mosimann UP , Felblinger J , Ballinari P , Hess CW , Müri RM ((2004) ) Visual exploration behaviour during clock reading in Alzheimer’s disease. Brain 127: , 431–438. |
[56] | Pereira MLGDF , Camargo MVZDA , Bellan AFR , Tahira AC , Dos Santos B , Dos Santos J , Machado-Lima A , Nunes FLS , Forlenza OV ((2020) ) Visual search efficiency in mild cognitive impairment and Alzheimer’s disease: An eye movement study. J Alzheimers Dis 75: , 261–275. |
[57] | Yeung L-K , Ryan JD , Cowell RA , Barense MD ((2013) ) Recognition memory impairments caused by false recognition of novel objects. J Exp Psychol Gen 142: , 1384–1397. |
[58] | Crutcher MD , Calhoun-Haney R , Manzanares CM , Lah JJ , Levey AI , Zola SM ((2009) ) Eye tracking during a visual paired comparison task as a predictor of early dementia. Am J Alzheimers Dis Dementiasr 24: , 258–266. |
[59] | Kim KW , Choi J , Chin J , Lee BH , Na DL ((2022) ) Eye-tracking metrics for figure-copying processes in early- vs. late-onset Alzheimer’s disease. Front Neurol 13: , 844341. |
[60] | Hanseeuw BJ , Betensky RA , Jacobs HIL , Schultz AP , Sepulcre J , Becker JA , Cosio DMO , Farrell M , Quiroz YT , Mormino EC , Buckley RF , Papp KV , Amariglio RA , Dewachter I , Ivanoiu A , Huijbers W , Hedden T , Marshall GA , Chhatwal JP , Rentz DM , Sperling RA , Johnson K ((2019) ) Association of amyloid and tau with cognition in preclinical Alzheimer disease: A longitudinal study. JAMA Neurol 76: , 915–924. |