Re-Addressing Dementia by Network Medicine and Mechanism-Based Molecular Endotypes
Abstract
Alzheimer’s disease (AD) and other forms of dementia are together a leading cause of disability and death in the aging global population, imposing a high personal, societal, and economic burden. They are also among the most prominent examples of failed drug developments. Indeed, after more than 40 AD trials of anti-amyloid interventions, reduction of amyloid-β (Aβ) has never translated into clinically relevant benefits, and in several cases yielded harm. The fundamental problem is the century-old, brain-centric phenotype-based definitions of diseases that ignore causal mechanisms and comorbidities. In this hypothesis article, we discuss how such current outdated nosology of dementia is a key roadblock to precision medicine and articulate how Network Medicine enables the substitution of clinicopathologic phenotypes with molecular endotypes and propose a new framework to achieve precision and curative medicine for patients with neurodegenerative disorders.
INTRODUCTION
Drug discovery is in a conceptual crisis. The landscape of chronic diseases has a glaring scarcity of curative, causal mechanism-based approaches. A clear illustration of this can be seen in Alzheimer’s disease (AD) and other forms of dementia such as vascular dementia, dementia with Lewy bodies (DLB), and Parkinson’s disease dementia (PDD), which despite countless hypotheses on possible pathomechanisms remain untreatable. One possible source of failure is the stubborn clinging to the one disease-one target-one drug dogma.
Virtually all existing drug development programs for dementia have been based on the “proteinopathy” concept, whereby proteins in an aggregated state (a cross-beta sheet configuration also known as amyloid, such as amyloid-β (Aβ) plaques in AD and Lewy bodies in DLB and PDD) shall cause the disease. As a consequence of this toxic gain-of-function concept of aggregated proteins causing neurodegenerative diseases, removing them from the brain should cure these diseases. The recent accelerated approval of the anti-amyloid monoclonal antibodies aducanumab and lecanemab by the US Food and Drug Administration became the culmination of this paradigm. The cumulative lessons of more than 40 anti-Aβ Alzheimer’s trials should have taught us otherwise: 15 monoclonal anti-amyloid antibodies significantly reduced amyloid and, surprisingly, significantly worsened patients’ outcomes compared to placebo. None of these trials was interpreted as a rejection of the hypothesis. Instead, the 16th and 17th anti-amyloid antibodies, lecanemab [1] and donanemab [2], which met the statistical threshold in the opposite direction, have been taken as a confirmation of the hypothesis [3], although this never translated into clinically relevant benefits [4]. Instead, reduction in soluble Aβ levels, as measured in cerebrospinal fluid, is harmful to humans, and removal of insoluble Aβ may lead to microhemorrhages, brain atrophy, and death [5, 6]. The toxic Aβ hypothesis has become virtually unfalsifiable [7].
Aβ, however, has important physiological roles, including neuroprotection. Thus, the loss of the soluble and therefore functional form of Aβ, i.e., a proteinopenia, may equally explain cognitive deficits and atrophy and possibly better than any particular plaque load, even among carriers of autosomal dominant AD-causing amyloid-beta precursor protein (APP), presenilin 1 (PSEN1), and presenilin 2 (PSEN2) mutations [8]. Many alternative causal hypotheses for dementia have been proposed; however, in the face of a “resilient” amyloid hypothesis, these have not received adequate support or buy-in to be tested [9–13]. Collective evidence should have sufficed to consider amyloid rather a downstream consequence in cellular pathophysiology, a sign of a range of biological stressors, not their cause.
Thus, the field of dementia—as those of many other chronic diseases—is in need of a paradigm shift to distance itself from using symptoms and pathology in organs as anchoring disease definitions. We call for a move from the current convergent clinico-pathologic towards a divergent, organ-agnostic, and mechanism-based disease nosology (Fig. 1). Even rare monogenic diseases cause symptoms in more than one organ, thus organ-based taxonomies make little sense and likely obstruct innovation.
Fig. 1
Shift from a brain-centric and neuropathology-based dementia nosology towards an organ-agnostic, mechanism-based nosology. Network Medicine accounts for causal heterogeneity and the identification of the molecular endotypes leading to the syndrome of dementia.
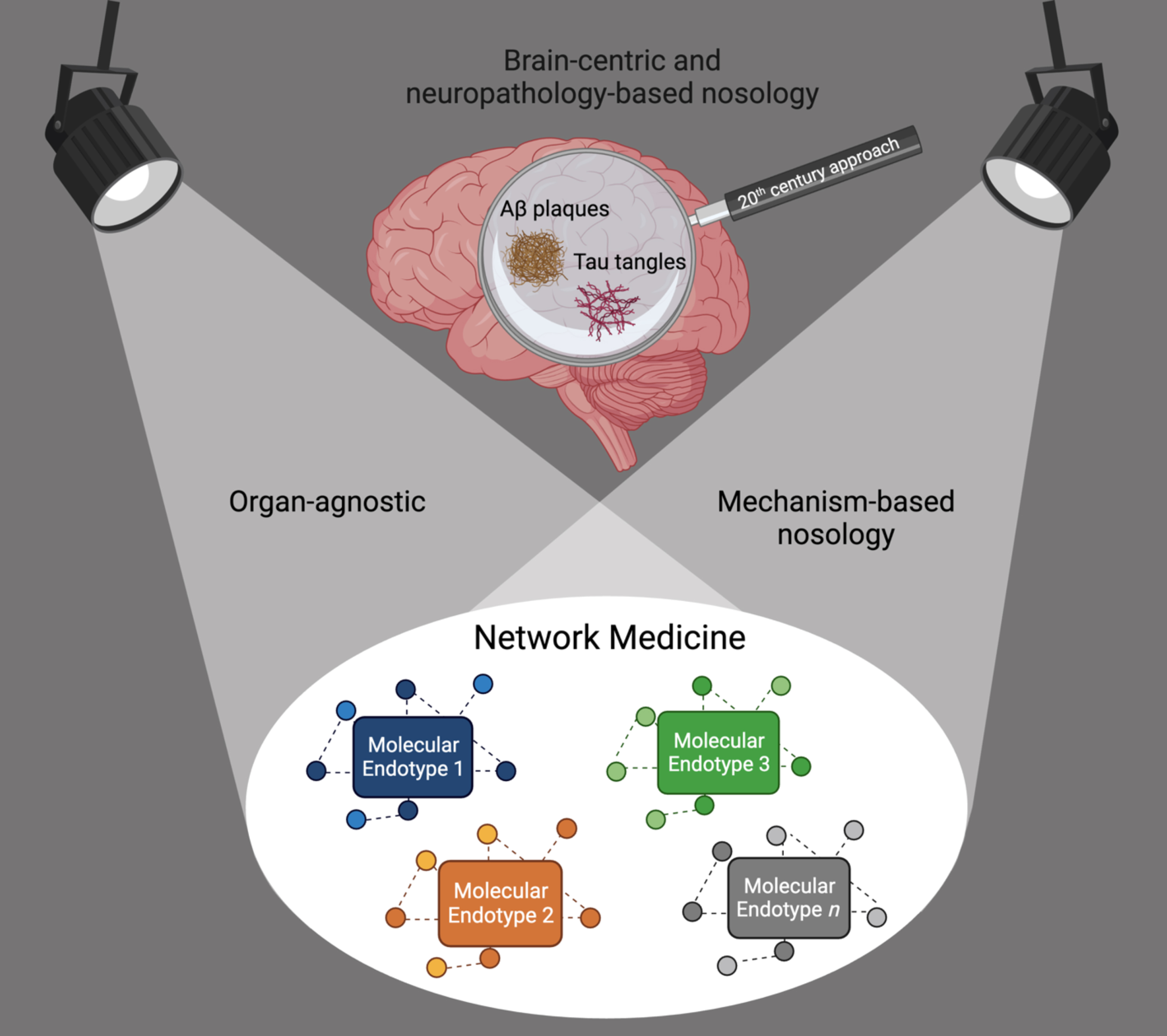
THE CURRENT OUTDATED FRAMEWORK TO DIAGNOSE AND TREAT DEMENTIA
The diagnosis of AD, DLB, PDD, PD, and other neurodegenerative disorders have been based on the combination of clinical descriptions and post-mortem microscopic observations, the limited technologies available in the 19th century. Even before Alois Alzheimer described the famous case of Auguste Deter in 1906, the prevailing approach was, and still is, to artificially separate pathological conditions within the same patient to be either in or outside the brain and define them as distinct diseases. Prolific work was carried on by Alois Alzheimer and contemporary psychiatrists in their search to define the links between biology and a plethora of mental disorders. The focus, however, has always been within the brain. This brain-centric approach has been maintained by the majority of clinicians and researchers to date, but now halts conceptual progress in understanding, preventing, and treating dementia.
A single pathology in the brain is the exception rather than the rule. Real-world aging individuals, with or without dementia, have mixed manifestations of neuropathological markers in their brains, such as AD markers, Lewy bodies, transactive response DNA binding protein 43 kDa (TDP-43) inclusions, and vascular pathologies, with almost 80% of these individuals presenting with at least two of such neuropathologies [14]. And in contrast with the model of brain-centricity, these “dementia specific” markers can be often found in organs outside the brain [15], as for example Aβ aggregates documented in skin, intestines [16], heart [17], and pancreas [18], with hyperphosphorylated tau also found in the last two.
Moreover, in clinical practice very rarely we find patients with dementia without any other concurrent diseases. Of all comorbidities, vascular disease and metabolic abnormalities stand out and are frequently observed preceding dementia [19–21]. More often than not, AD patients show morphological substrates of cerebrovascular disease with matching risk factors such as hypertension and diabetes, pointing to the existence of shared pathobiological mechanisms with well-defined genetic and molecular underpinnings [22–28].
A NEW FRAMEWORK FOR DEMENTIA BY NETWORK MEDICINE
The network of all human diseases linked through shared risk genes, the diseasome, was a landmark demonstration of the similarities and overlaps between dementias and other neurological phenotypes to non-neurological disorders [29] (Fig. 2). Since then, the mechanistic relationships among this group of heterogenous phenotypes have been extensively validated by multiscale disease networks based also on shared genes, protein-protein interactions, drugs, symptoms, and comorbidities [30–32]. These mechanistic relationships thus provide the leads to identifying causative molecular mechanisms underlying different subtypes of dementia.
Fig. 2
Schematic representation of a mechanism-based redefinition of dementia nosology for precision medicine. Dementia is an umbrella term, comorbid with several non-neurological disease phenotypes. Their associated shared risk genes point to mutual mechanistic endotypes. This then leads to molecular disease definitions allowing precision diagnosis and tailored therapeutic intervention. A) The Human Disease Network, the diseasome, adapted from [23] and first presented in 2007 by Goh et al. [17], was a landmark for the field of network medicine and disease research. It provided a novel perspective on the connections between various human diseases by their shared risk genes. B) A diseasome-derived cluster of heterogeneous phenotypes indicates shared mechanisms between dementias and other neurological and non-neurological disorders. C) A new framework to guide how we classify dementia by molecular endotypes, going from non-specific, homogenized umbrella terms to the identification of the underlying heterogeneous molecular mechanisms, followed by the development of mechanism-based diagnostic tools and precision and personalized treatment.
![Schematic representation of a mechanism-based redefinition of dementia nosology for precision medicine. Dementia is an umbrella term, comorbid with several non-neurological disease phenotypes. Their associated shared risk genes point to mutual mechanistic endotypes. This then leads to molecular disease definitions allowing precision diagnosis and tailored therapeutic intervention. A) The Human Disease Network, the diseasome, adapted from [23] and first presented in 2007 by Goh et al. [17], was a landmark for the field of network medicine and disease research. It provided a novel perspective on the connections between various human diseases by their shared risk genes. B) A diseasome-derived cluster of heterogeneous phenotypes indicates shared mechanisms between dementias and other neurological and non-neurological disorders. C) A new framework to guide how we classify dementia by molecular endotypes, going from non-specific, homogenized umbrella terms to the identification of the underlying heterogeneous molecular mechanisms, followed by the development of mechanism-based diagnostic tools and precision and personalized treatment.](https://ip.ios.semcs.net:443/media/jad/2023/96-1/jad-96-1-jad230694/jad-96-jad230694-g002.jpg)
Systems and network medicine approaches enable the substitution of brain-centric umbrella definitions of disease, such as AD, by shared mechanistic endotypes. It is therefore useful to define endotype and phenotype beforehand for clarity. An endotype is a molecular mechanism underlying one or more phenotypes, which are, in turn, the functional (pathological) consequences, signs, or symptoms that can be observed in an individual. Current criteria for classifying dementias are phenotype-based, not endotype-based. This means that they are based on medical consensus about which signs and symptoms (such as brain atrophy, hyperintensities, tremor, memory loss, hallucinations, type of proteinopathy, etc.), age of onset, speed of progression, severity, or other descriptive features best describe a condition. However, different mechanistic endotypes can cause similar phenotypes of dementia but also different phenotypes in organs other than the brain. For example, AD is not only highly related at the causal level (shared risk genes) to other neurodegenerative diseases with common clinical features such as leukodystrophy and amyotrophic lateral sclerosis, but also to otherwise entirely distinct diseases such as polycystic ovary syndrome, which cannot be understood based on an organ-based disease taxonomy [33, 34]. These, and many other cases, are unlikely to represent “comorbidities” but different phenotypic expressions of the same common underlying mechanism, or endotype, in those affected. Exploiting this concept for precision medicine requires however, both the identification of potential endotypes and the availability of drugs that target the underlying causal mechanism in the relevant individuals, not organ-based symptom reduction across a clinically-diagnosed population.
It is worth acknowledging that the past decade has seen a marked increase in endotyping efforts aimed at addressing a wide range of complex diseases and syndromes in many other areas of medicine. These include, but are not limited to, cancer [35], coronary artery disease [36], asthma [37, 38], retinopathies [39, 40], chronic inflammatory diseases [41], sepsis [42], and sepsis in the context of COVID-19 [43].
PRECISION ENDOTYPE-BASED DIAGNOSTICS
Endotype identification starts by the definition of disease modules. Currently, these can be best constructed from disease validated seed genes and their neighbors in the human protein-protein interaction network (the interactome). The resulting so-called protein-protein interaction modules, the disease modules, are the basis also for a mechanism-based redefinition of dementia and its endotypes. Though not all the interactions between human proteins are known and some of the known interactions are prone to false positives (i.e., experimental artifacts), the use of such disease modules on the interactome has provided valuable insights on the unifying biological processes and comorbidities across diseases and allowed the identification of candidate treatments targeting these modules [44, 45]. Recent advances in deep learning-based protein structure prediction [46] and large-scale sequencing and genome-wide association studies across individuals [47, 48] are expected to further improve the research gap in the characterization of protein interactions towards a more complete and refined disease module identification.
One example of such a disease module is related to reactive oxygen species (ROS) formation and cyclic guanosine monophosphate (cGMP) signaling (the ROCG disease module) [49]. A striking feature of this ROCG module is that it includes two of the most relevant AD risk genes, PSEN2 and apolipoprotein E (APOE), and these interact directly with endothelial nitric oxide synthase (NOS3), a master modulator of vascular function. Indeed, non-physiological ROS formation and dysfunction of cGMP signaling have deleterious effects on the vasculature and are key mechanisms underlying pathophysiological features common to different types of dementia such as chronic hypoperfusion, impaired neurovascular coupling, and blood-brain barrier integrity. In addition, mechanistic preclinical studies show that disturbances of the functions of the proteins within the ROCG module are upstream events causally affecting traditionally dementia-associated markers such as tau [50, 51] and Aβ [52, 53]. Thus, when considering the hypothesis that not only this but many other disease modules may lead to tau and Aβ alterations widely found in the aged population, one can understand why clustering individuals based on such markers is preventing the identification of the true causes of dementia at the individual level.
PRECISION NETWORK PHARMACOLOGY TARGETING MECHANISTIC ENDOTYPES IN DEMENTIA
Therapeutically, endotypes must not be reduced to the one-target-one-disease or “magic bullet” treatment approach. Disease modules are rather small localized multi-protein signaling networks, typically distinct from curated canonical signaling pathways. Currently known disease-gene associations that lay the foundation for describing disease modules typically reflect more common mechanisms across the general disease population. By incorporating the interactions of such common disease genes in the network together with omics data from patients, one can capture less frequently observed risk genes across individuals that are convergent at the pathway level and identify drug-target interactions for tailored therapy in patients [54–56].
In addition, a network needs network pharmacology, which is a different pharmacological approach from traditional polypharmacy and means targeting different proteins in the same disease module in a synergistic manner. Lack of a mechanism-based patient stratification and a single target approach, for example, may explain why the phosphodiesterase 5 (PDE5) inhibitor sildenafil, which potentially improves cGMP signaling within a defective ROCG module, did not decrease AD risk in general [57]. In addition, not all patients with AD dementia-like symptoms share the ROCG endotype. Therefore, this new mechanism-based diagnostic and therapeutic framework for dementia will be best tested in proof-of-concept adaptive clinical trials, where interventions align with the relevant mechanistic endotypes of those being treated [17].
In this context, numerous endotypes are yet to be defined and validated for future network pharmacology-based clinical trials. Potential new disease modules include other relevant dementia risk genes, and can be related for example to relevant loss of function of proteins such as AβPP, Aβ, other AβPP cleavage products (e.g., soluble AβPP alpha and AβPP-intracellular domain), and PSEN1 which have essential physiological roles in synaptic plasticity, hippocampal neurotransmitter release (e.g., soluble Aβ-mediated activation of α7-nicotinic acetylcholine receptors), as well as maintenance of metal ion homeostasis [58, 59]. An example of another potential endotype could be related to phosphodiesterase 4 (PDE4), with PDE4B [60] and PDE4D [61] gene variants reported to be associated with AD risk and disease progression. Interestingly, PDE4D is a direct neighbor of APP and bridging integrator 1 (BIN1), also an important AD risk gene, within the interactome, and its dysfunction is hypothesized to result in dysfunctional cAMP signaling affecting synaptic plasticity, cognition, regulation of inflammatory processes and tau pathology [62, 63]. The construction of these and further disease modules is the steppingstone towards a mechanism-based approach to dementia endotypes. Importantly, while in approximately 40% of the cases dementia can be potentially preventable by lifestyle interventions alone [64], network pharmacology stands to benefit from the increasing recognition of endotypes, disease modules, and their corresponding precision diagnostic tools to usher an era of precision medicine for patients with neurodegenerativedisorders.
CONCLUSION
The path to precision clinical practice at the dawn of network medicine is challenging but achievable and starts with fundamentally rethinking how we define diseases. Throughout the last decade, network medicine has consistently demonstrated the pathobiological similarities among certain comorbidities (or phenotypes) and that these relationships can be exploited to identify disease modules (endotypes) and mechanism-based therapeutic strategies to treat these conditions despite current knowledge gaps or incomplete data [49]. The realization of such a conceptual revolution, of course, requires a matching level of methodological and technological readiness, including the assembly of phenotype-agnostic study cohorts, such as the CCBP, to determine endotypes of relevance to individuals with different phenotypes [65].
Above all, we need to recognize that we are dealing with a new discovery pipeline, one that necessarily must begin with genetics-based disease module discovery, not direct drug discovery, and the identification and validation of functionally meaningful and actionable (meaning treatable) molecular disease mechanisms, i.e., endotypes. Network-based approaches [66–69] offer the tools to systematically characterize the phenotypic heterogeneity observed in individuals with dementia [70, 71]. Accordingly, accounting for an individual’s comorbidities and specific patterns of neuroimaging, genetic, and molecular variability will be critical towards endotype-driven clinical decision making [72, 73]. Open challenges include the selection of only relevant and causal disease genes and reliable protein-protein interaction databases to construct the dementia-relevant signaling modules. Clinical diagnoses such as AD, PD, and other umbrella classifications, have clearly outlived their reductive usefulness for precision medicine. Their replacement with network medicine-derived endotypes, precision diagnosis and therapeutics will lead to the end of ‘dementia’ as we know it.
ACKNOWLEDGMENTS
Figure 1 was created with BioRender (BioRender.com).
FUNDING
Mayra Pacheco Pachado, Ana I. Casas, Cristian Nogales, Emre Guney, and Harald H.H.W. Schmidt
The European Union’s Horizon 2020 research and innovation programme funded the REPO-TRIAL project grant agreement No 777111. This reflects only the author’s view, and the European Commission is not responsible for any use that may be made of the information it contains.
The European Union’s Horizon Europe research and innovation programme funded the REPO4EU project under grant agreement No 101057619. This reflects only the author’s view, and the European Commission is not responsible for any use that may be made of the information it contains.
Ana I. Casas
Förderprogramm der Corona-Stiftung im Stifterverband and Germany Federal Ministry of Education and Research 01EJ2205D.
Mahmoud H. Elbatreek
Science and Technology Development Fund, STDF, grant No. 46040 and Science and Technology Development Fund, STDF, grant No. 46049.
Alberto J. Espay
National Institute of Health grant R01AG059417, National Institute of Health grant U01 NS113851, National Institute of Health grant 1R01 NS125294-01, and Michael J Fox Foundation grant NCT04477785.
CONFLICT OF INTEREST
Prof. Espay has received grant support from the NIH and the Michael J Fox Foundation; personal compensation as a consultant/scientific advisory board member for Neuroderm, Amneal, Acadia, Avion Pharmaceuticals, Acorda, Kyowa Kirin, Sunovion, Supernus (formerly, USWorldMeds), and Herantis Pharma; personal honoraria for speakership for Avion, Amneal, and Supernus; and publishing royalties from Lippincott Williams & Wilkins, Cambridge University Press, and Springer. He cofounded REGAIN Therapeutics and is co-inventor of the patent “Compositions and methods for treatment and/or prophylaxis of proteinopathies.”
The other authors have no conflict of interest to report.
REFERENCES
[1] | van Dyck CH , Swanson CJ , Aisen P , Bateman RJ , Chen C , Gee M , Kanekiyo M , Li D , Reyderman L , Cohen S , Froelich L , Katayama S , Sabbagh M , Vellas B , Watson D , Dhadda S , Irizarry M , Kramer LD , Iwatsubo T ((2022) ) Lecanemab in early Alzheimer’s disease. N Engl J Med 388: , 9–21. |
[2] | Sims JR , Zimmer JA , Evans CD , Lu M , Ardayfio P , Sparks J , Wessels AM , Shcherbinin S , Wang H , Nery ESM , Collins EC , Solomon P , Salloway S , Hansson O , Ritchie C , Brooks DA , Mintun M , Skovronsky DM , TRAILBLAZER-ALZ 2 Investigators ((2023) ) Donanemab in early symptomatic Alzheimer disease: The TRAILBLAZER-ALZ 2 randomized clinical trial. JAMA 330: , 512–527. |
[3] | Reiman EM ((2023) ) Drug trial for Alzheimer’s disease is a game changer. Nature 615: , 42–43. |
[4] | Espay AJ , Sturchio A , Schneider LS , Ezzat K ((2021) ) Soluble amyloid-β consumption in Alzheimer’s disease. J Alzheimers Dis 82: , 1403–1415. |
[5] | Alves F , Kalinowski P , Ayton S ((2023) ) Accelerated brain volume loss caused by anti-β-amyloid drugs. Neurology 100: , e2114–e2124. |
[6] | Castellani RJ , Shanes ED , McCord M , Reish NJ , Flanagan ME , Mesulam M-M , Jamshidi P ((2023) ) Neuropathology of anti-amyloid-β immunotherapy: A case report. J Alzheimers Dis 93: , 803–813. |
[7] | Espay AJ , Herrup K , Daly T (2023) Finding the falsification threshold of the toxic proteinopathy hypothesis in neurodegeneration. In Handbook of Clinical Neurology, Espay AJ, ed. Elsevier, pp.143–154. |
[8] | Sturchio A , Dwivedi AK , Malm T , Wood MJA , Cilia R , Sharma JS , Hill EJ , Schneider LS , Graff-Radford NR , Mori H , Nübling G , El Andaloussi S , Svenningsson P , Ezzat K , Espay AJ , Dominantly Inherited Alzheimer Consortia (DIAN) ((2022) ) High soluble amyloid-β42 predicts normal cognition in amyloid-positive individuals with Alzheimer’s disease-causing mutations. J Alzheimers Dis 90: , 333–348. |
[9] | Behl C ((2017) ) Amyloid in Alzheimer’s disease: Guilty beyond reasonable doubt? Trends Pharmacol Sci 38: , 849–851. |
[10] | Karran E , De Strooper B ((2016) ) The amyloid cascade hypothesis: Are we poised for success or failure? J Neurochem 139: , 237–252. |
[11] | Itzhaki RF , Lathe R , Balin BJ , Ball MJ , Bearer EL , Braak H , Bullido MJ , Carter C , Clerici M , Cosby SL , Del Tredici K , Field H , Fulop T , Grassi C , Griffin WST , Haas J , Hudson AP , Kamer AR , Kell DB , Licastro F , Letenneur L , Lövheim H , Mancuso R , Miklossy J , Otth C , Palamara AT , Perry G , Preston C , Pretorius E , Strandberg T , Tabet N , Taylor-Robinson SD , Whittum-Hudson JA ((2016) ) Microbes and Alzheimer’s disease. J Alzheimers Dis 51: , 979–984. |
[12] | Harris SA , Harris EA ((2015) ) Herpes simplex virus type 1 and other pathogens are key causative factors in sporadic Alzheimer’s disease. J Alzheimers Dis 48: , 319–353. |
[13] | Soscia SJ , Kirby JE , Washicosky KJ , Tucker SM , Ingelsson M , Hyman B , Burton MA , Goldstein LE , Duong S , Tanzi RE , Moir RD ((2010) ) The Alzheimer’s disease-associated amyloid β-protein is an antimicrobial peptide. PLoS One 5: , e9505. |
[14] | Boyle PA , Yu L , Wilson RS , Leurgans SE , Schneider JA , Bennett DA ((2018) ) Person-specific contribution of neuropathologies to cognitive loss in old age. Ann Neurol 83: , 74–83. |
[15] | Wang J , Gu BJ , Masters CL , Wang Y-J ((2017) ) A systemic view of Alzheimer disease - insights from amyloid-β metabolism beyond the brain. Nat Rev Neurol 13: , 703. |
[16] | Joachim CL , Mori H , Selkoe DJ ((1989) ) Amyloid β-protein deposition in tissues other than brain in Alzheimer’s disease. Nature 341: , 226–230. |
[17] | Luciani M , Montalbano M , Troncone L , Bacchin C , Uchida K , Daniele G , Jacobs WB , Butler HM , Kiel J , Berto S , Gensemer C , Moore K , Morningstar J , Diteepeng T , Albayram O , Abisambra JF , Norris RA , Di Salvo TG , Prosser B , Kayed R , Del Monte F ((2023) ) Big tau aggregation disrupts microtubule tyrosination and causes myocardial diastolic dysfunction: From discovery to therapy. Eur Heart J 44: , 1560–1570. |
[18] | Miklossy J , Qing H , Radenovic A , Kis A , Vileno B , Làszló F , Miller L , Martins RN , Waeber G , Mooser V , Bosman F , Khalili K , Darbinian N , McGeer PL ((2010) ) Beta amyloid and hyperphosphorylated tau deposits in the pancreas in type 2 diabetes. Neurobiol Aging 31: , 1503–15. |
[19] | Wolters FJ , Zonneveld HI , Hofman A , van der Lugt A , Koudstaal PJ , Vernooij MW , Ikram MA ((2017) ) Cerebral perfusion and the risk of dementia: A population-based study. Circulation 136: , 719–728. |
[20] | Ruitenberg A , den Heijer T , Bakker SLM , van Swieten JC , Koudstaal PJ , Hofman A , Breteler MMB ((2005) ) Cerebral hypoperfusion and clinical onset of dementia: The Rotterdam study. Ann Neurol 57: , 789–794. |
[21] | Amidei CB , Fayosse A , Dumurgier J , Machado-Fragua MD , Tabak AG , van Sloten T , Kivimäki M , Dugravot A , Sabia S , Singh-Manoux A ((2021) ) Association between age at diabetes onset and subsequent risk of dementia. JAMA 325: , 1640–1649. |
[22] | Iturria-Medina Y , Sotero RC , Toussaint PJ , Mateos-Pérez JM , Evans AC ((2016) ) Early role of vascular dysregulation on late-onset Alzheimer’s disease based on multifactorial data-driven analysis. Nat Commun 7: , 11934. |
[23] | Walker KA , Sharrett AR , Wu A , Schneider ALC , Albert M , Lutsey PL , Bandeen-Roche K , Coresh J , Gross AL , Windham BG , Knopman DS , Power MC , Rawlings AM , Mosley TH , Gottesman RF ((2019) ) Association of midlife to late-life blood pressure patterns with incident dementia. JAMA 322: , 535–545. |
[24] | Iadecola C ((2010) ) The overlap between neurodegenerative and vascular factors in the pathogenesis of dementia. Acta Neuropathol 120: , 287. |
[25] | Bangen KJ , Nation DA , Delano-Wood L , Weissberger GH , Hansen LA , Galasko DR , Salmon DP , Bondi MW ((2015) ) Aggregate effects of vascular risk factors on cerebrovascular changes in autopsy-confirmed Alzheimer’s disease. Alzheimers Dement 11: , 394. |
[26] | Arvanitakis Z , Capuano AW , Leurgans SE , Bennett DA , Schneider JA ((2016) ) Relation of cerebral vessel disease to Alzheimer’s disease dementia and cognitive function in elderly people: A cross-sectional study. Lancet Neurol 15: , 934–943. |
[27] | Zhu Z , Lin Y , Li X , Driver JA , Liang L ((2019) ) Shared genetic architecture between metabolic traits and Alzheimer’s disease: A large-scale genome-wide cross-trait analysis. Hum Genet 138: , 271–285. |
[28] | European Alzheimer’s & Dementia Biobank Mendelian Randomization (EADB-MR) Collaboration, Luo J , Thomassen JQ , Bellenguez C , Grenier-Boley B , de Rojas I , Castillo A , Parveen K , KüçKükali F , Nicolas A , Peters O , Schneider A , Dichgans M , Rujescu D , Scherbaum N , Jürgen D , Riedel-Heller S , Hausner L , Porcel LM , Düzel E , Grimmer T , Wiltfang J , Heilmann-Heimbach S , Moebus S , Tegos T , Scarmeas N , Clarimon J , Moreno F , Pérez-Tur J , Bullido MJ , Pastor P , Sánchez-Valle R , Álvarez V , Boada M , García-González P , Puerta R , Mir P , Real LM , Piñol-Ripoll G , García-Alberca JM , Royo JL , Rodriguez-Rodriguez E , Soininen H , Kuulasmaa T , de Mendonça A , Mehrabian S , Hort J , Vyhnalek M , van der Lee S , Graff C , Papenberg G , Giedraitis V , Boland A , Bacq-Daian D , Deleuze J-F , Nicolas G , Dufouil C , Pasquier F , Hanon O , Debette S , Grünblatt E , Popp J , Benussi L , Galimberti D , Arosio B , Mecocci P , Solfrizzi V , Parnetti L , Squassina A , Tremolizzo L , Borroni B , Nacmias B , Sorbi S , Caffarra P , Seripa D , Rainero I , Daniele A , Masullo C , Spalletta G , Williams J , Amouyel P , Jessen F , Kehoe P , Tsolaki M , Rossi G , Sánchez-Juan P , Sleegers K , Ingelsson M , Andreassen OA , Hiltunen M , Van Duijn C , Sims R , van der Flier W , Ruiz A , Ramirez A , Lambert J-C , Frikke-Schmidt R ((2023) ) Genetic associations between modifiable risk factors and Alzheimer disease. JAMA Netw Open 6: , e2313734. |
[29] | Goh K-I , Cusick ME , Valle D , Childs B , Vidal M , Barabási A-L ((2007) ) The human disease network. Proc Natl Acad Sci U S A 104: , 8685–8690. |
[30] | Langhauser F , Casas AI , Dao V-T-V , Guney E , Menche J , Geuss E , Kleikers PWM , López MG , Barabási A-L , Kleinschnitz C , Schmidt HHHW ((2018) ) A diseasome cluster-based drug repurposing of soluble guanylate cyclase activators from smooth muscle relaxation to direct neuroprotection. NPJ Syst Biol Appl 4: , 8. |
[31] | Santiago JA , Potashkin JA ((2013) ) Shared dysregulated pathways lead to Parkinson’s disease and diabetes. Trends Mol Med 19: , 176–186. |
[32] | Aguirre-Plans J , Piñero J , Menche J , Sanz F , Furlong LI , Schmidt HHHW , Oliva B , Guney E ((2018) ) Proximal pathway enrichment analysis for targeting comorbid diseases via network endopharmacology. Pharmaceuticals (Basel) 11: , 61. |
[33] | Maiorino E , Baek SH , Guo F , Zhou X , Kothari PH , Silverman EK , Barabási A-L , Weiss ST , Raby BA , Sharma A ((2020) ) Discovering the genes mediating the interactions between chronic respiratory diseases in the human interactome. Nat Commun 11: , 811. |
[34] | Meier A , Leahy L , Pontius A , Zappia S , Brennan M , Papapetropoulos S , Wszolek Z ((2023) ) Adult-onset leukoencephalopathy with axonal spheroids and pigmented glia (ALSP) is commonly misdiagnosed as frontotemporal dementia (FTD), multiple sclerosis (MS), Alzheimer’s disease (AD), or other adult-onset leukodystrophies (P12-4.003). Neurology 100: , 3824. |
[35] | Sicklick JK , Kato S , Okamura R , Schwaederle M , Hahn ME , Williams CB , De P , Krie A , Piccioni DE , Miller VA , Ross JS , Benson A , Webster J , Stephens PJ , Lee JJ , Fanta PT , Lippman SM , Leyland-Jones B , Kurzrock R ((2019) ) Molecular profiling of cancer patients enables personalized combination therapy: The I-PREDICT study. Nat Med 25: , 744–750. |
[36] | Wang R-S , Loscalzo J ((2018) ) Network-based disease module discovery by a novel seed connector algorithm with pathobiological implications. J Mol Biol 430: , 2939. |
[37] | Sharma A , Menche J , Huang CC , Ort T , Zhou X , Kitsak M , Sahni N , Thibault D , Voung L , Guo F , Ghiassian SD , Gulbahce N , Baribaud F , Tocker J , Dobrin R , Barnathan E , Liu H , Panettieri RA , Tantisira KG , Qiu W , Raby BA , Silverman EK , Vidal M , Weiss ST , Barabási AL ((2015) ) A disease module in the interactome explains disease heterogeneity, drug response and captures novel pathways and genes in asthma. Hum Mol Genet 24: , 3005–3020. |
[38] | Anderson GP ((2008) ) Endotyping asthma: New insights into key pathogenic mechanisms in a complex, heterogeneous disease. Lancet 372: , 1107–1119. |
[39] | Kiel C , Lastrucci C , Luthert PJ , Serrano L ((2017) ) Simple and complex retinal dystrophies are associated with profoundly different disease networks. Sci Rep 7: , 41835. |
[40] | Pool FM , Kiel C , Serrano L , Luthert PJ ((2020) ) Repository of proposed pathways and protein–protein interaction networks in age-related macular degeneration. NPJ Aging Mech Dis 6: , 1–11. |
[41] | Schultze JL , Rosenstiel P ((2018) ) Systems medicine in chronic inflammatory diseases. Immunity 48: , 608–613. |
[42] | Baghela A , Pena OM , Lee AH , Baquir B , Falsafi R , An A , Farmer SW , Hurlburt A , Mondragon-Cardona A , Rivera JD , Baker A , Trahtemberg U , Shojaei M , Jimenez-Canizales CE , Dos Santos CC , Tang B , Bouma HR , Cohen Freue GV , Hancock REW ((2022) ) Predicting sepsis severity at first clinical presentation: The role of endotypes and mechanistic signatures. EBioMedicine 75: , 103776. |
[43] | Baghela A , An A , Zhang P , Acton E , Gauthier J , Brunet-Ratnasingham E , Blimkie T , Freue GC , Kaufmann D , Lee AHY , Levesque RC , Hancock REW ((2023) ) Predicting severity in COVID-19 disease using sepsis blood gene expression signatures. Sci Rep 13: , 1247. |
[44] | Menche J , Sharma A , Kitsak M , Ghiassian SD , Vidal M , Loscalzo J , Barabási A-L ((2015) ) Uncovering disease-disease relationships through the incomplete interactome. Science 347: , 1257601. |
[45] | Guney E , Menche J , Vidal M , Barábasi A-L ((2016) ) Network-based in silico drug efficacy screening. Nat Commun 7: , 10331. |
[46] | Varadi M , Anyango S , Deshpande M , Nair S , Natassia C , Yordanova G , Yuan D , Stroe O , Wood G , Laydon A , Žídek A , Green T , Tunyasuvunakool K , Petersen S , Jumper J , Clancy E , Green R , Vora A , Lutfi M , Figurnov M , Cowie A , Hobbs N , Kohli P , Kleywegt G , Birney E , Hassabis D , Velankar S ((2021) ) AlphaFold Protein Structure Database: Massively expanding the structural coverage of protein-sequence space with high-accuracy models. Nucleic Acids Res 50: , D439–D444. |
[47] | Genomics England, 100,000 Genomes Project https://www.genomicsengland.co.uk/initiatives/100000-genomes-project. |
[48] | Tcheandjieu C , Zhu X , Hilliard AT , Clarke SL , Napolioni V , Ma S , Lee KM , Fang H , Chen F , Lu Y , Tsao NL , Raghavan S , Koyama S , Gorman BR , Vujkovic M , Klarin D , Levin MG , Sinnott-Armstrong N , Wojcik GL , Plomondon ME , Maddox TM , Waldo SW , Bick AG , Pyarajan S , Huang J , Song R , Ho Y-L , Buyske S , Kooperberg C , Haessler J , Loos RJF , Do R , Verbanck M , Chaudhary K , North KE , Avery CL , Graff M , Haiman CA , Le Marchand L , Wilkens LR , Bis JC , Leonard H , Shen B , Lange LA , Giri A , Dikilitas O , Kullo IJ , Stanaway IB , Jarvik GP , Gordon AS , Hebbring S , Namjou B , Kaufman KM , Ito K , Ishigaki K , Kamatani Y , Verma SS , Ritchie MD , Kember RL , Baras A , Lotta LA , Kathiresan S , Hauser ER , Miller DR , Lee JS , Saleheen D , Reaven PD , Cho K , Gaziano JM , Natarajan P , Huffman JE , Voight BF , Rader DJ , Chang K-M , Lynch JA , Damrauer SM , Wilson PWF , Tang H , Sun YV , Tsao PS , O’Donnell CJ , Assimes TL ((2022) ) Large-scale genome-wide association study of coronary artery disease in genetically diverse populations. Nat Med 28: , 1679–1692. |
[49] | Nogales C , Mamdouh ZM , List M , Kiel C , Casas AI , Schmidt HHHW ((2022) ) Network pharmacology: Curing causal mechanisms instead of treating symptoms. Trends Pharmacol Sci 43: , 136–150. |
[50] | Seo D-O , O’Donnell D , Jain N , Ulrich JD , Herz J , Li Y , Lemieux M , Cheng J , Hu H , Serrano JR , Bao X , Franke E , Karlsson M , Meier M , Deng S , Desai C , Dodiya H , Lelwala-Guruge J , Handley SA , Kipnis J , Sisodia SS , Gordon JI , Holtzman DM ((2023) ) ApoE isoform- and microbiota-dependent progression of neurodegeneration in a mouse model of tauopathy. Science 379: , eadd1236. |
[51] | Faraco G , Hochrainer K , Segarra SG , Schaeffer S , Santisteban MM , Menon A , Jiang H , Holtzman DM , Anrather J , Iadecola C ((2019) ) Dietary salt promotes cognitive impairment through tau phosphorylation. Nature 574: , 686–690. |
[52] | Ahmed S , Jing Y , Mockett BG , Zhang H , Abraham WC , Liu P ((2022) ) Partial endothelial nitric oxide synthase deficiency exacerbates cognitive deficit and amyloid pathology in the APPswe/PS1ΔE9 mouse model of Alzheimer’s disease. Int J Mol Sci 23: , 7316. |
[53] | Austin SA , Katusic ZS ((2020) ) Partial loss of endothelial nitric oxide leads to increased cerebrovascular beta amyloid. J Cereb Blood Flow Metab 40: , 392–403. |
[54] | Ozturk K , Dow M , Carlin DE , Bejar R , Carter H ((2018) ) The emerging potential for network analysis to inform precision cancer medicine. J Mol Biol 430: , 2875–2899. |
[55] | Schulte-Sasse R , Budach S , Hnisz D , Marsico A ((2021) ) Integration of multiomics data with graph convolutional networks to identify new cancer genes and their associated molecular mechanisms. Nat Mach Intell 3: , 513–526. |
[56] | Menche J , Guney E , Sharma A , Branigan PJ , Loza MJ , Baribaud F , Dobrin R , Barabási AL ((2017) ) Integrating personalized gene expression profiles into predictive disease-associated gene pools. NPJ Syst Biol Appl 3: , 10. |
[57] | Desai RJ , Mahesri M , Lee SB , Varma VR , Loeffler T , Schilcher I , Gerhard T , Segal JB , Ritchey ME , Horton DB , Kim SC , Schneeweiss S , Thambisetty M ((2022) ) No association between initiation of phosphodiesterase-5 inhibitors and risk of incident Alzheimer’s disease and related dementia: Results from the Drug Repurposing for Effective Alzheimer’s Medicines study. Brain Commun 4: , fcac247. |
[58] | Kepp KP ((2016) ) Alzheimer’s disease due to loss of function: A new synthesis of the available data. Prog Neurobiol 143: , 36–60. |
[59] | Letsinger AC , Gu Z , Yakel JL ((2022) ) α7 nicotinic acetylcholine receptors in the hippocampal circuit: Taming complexity. Trends Neurosci 45: , 145–157. |
[60] | Adewuyi EO , O’Brien EK , Nyholt DR , Porter T , Laws SM ((2022) ) A large-scale genome-wide cross-trait analysis reveals shared genetic architecture between Alzheimer’s disease and gastrointestinal tract disorders. Commun Biol 5: , 691. |
[61] | Sherva R , Gross A , Mukherjee S , Koesterer R , Amouyel P , Bellenguez C , Dufouil C , Bennett DA , Chibnik L , Cruchaga C , del-Aguila J , Farrer LA , Mayeux R , Munsie L , Winslow A , Newhouse S , Saykin AJ , Kauwe JSK , Alzheimer’s Disease Genetics Consortium, Crane PK , Green RC ((2020) ) Genome-wide association study of rate of cognitive decline in Alzheimer’s Disease patients identifies novel genes and pathways. Alzheimers Dement 16: , 1134. |
[62] | Schreiber R , Hollands R , Blokland A ((2020) ) A mechanistic rationale for PDE-4 inhibitors to treat residual cognitive deficits in acquired brain injury. Curr Neuropharmacol 18: , 188–201. |
[63] | Ricciarelli R , Fedele E ((2018) ) cAMP, cGMP and amyloid β: Three ideal partners for memory formation. Trends Neurosci 41: , 255–266. |
[64] | Livingston G , Huntley J , Sommerlad A , Ames D , Ballard C , Banerjee S , Brayne C , Burns A , Cohen-Mansfield J , Cooper C , Costafreda SG , Dias A , Fox N , Gitlin LN , Howard R , Kales HC , Kivimäki M , Larson EB , Ogunniyi A , Orgeta V , Ritchie K , Rockwood K , Sampson EL , Samus Q , Schneider LS , Selbæk G , Teri L , Mukadam N ((2020) ) Dementia prevention, intervention, and care: 2020 report of the Lancet Commission. Lancet 396: , 413–446. |
[65] | Sturchio A , Marsili L , Vizcarra JA , Dwivedi AK , Kauffman MA , Duker AP , Lu P , Pauciulo MW , Wissel BD , Hill EJ , Stecher B , Keeling EG , Vagal AS , Wang L , Haslam DB , Robson MJ , Tanner CM , Hagey DW , El Andaloussi S , Ezzat K , Fleming RMT , Lu LJ , Little MA , Espay AJ ((2020) ) Phenotype-agnostic molecular subtyping of neurodegenerative disorders: The Cincinnati Cohort Biomarker Program (CCBP). Front Aging Neurosci 12: , 553635. |
[66] | Žitnik M , Janjić V , Larminie C , Zupan B , Pržulj N ((2013) ) Discovering disease-disease associations by fusing systems-level molecular data. Sci Rep 3: , 3202. |
[67] | Halu A , De Domenico M , Arenas A , Sharma A ((2019) ) The multiplex network of human diseases. NPJ Syst Biol Appl 5: , 15. |
[68] | Li MM , Huang K , Zitnik M ((2022) ) Graph representation learning in biomedicine and healthcare. Nat Biomed Eng 6: , 1353–1369. |
[69] | Zhou X , Lei L , Liu J , Halu A , Zhang Y , Li B , Guo Z , Liu G , Sun C , Loscalzo J , Sharma A , Wang Z ((2018) ) A systems approach to refine disease taxonomy by integrating phenotypic and molecular networks. eBioMedicine 31: , 79–91. |
[70] | Echávarri C , Burgmans S , Caballero MC , García-Bragado F , Verhey FRJ , Uylings HBM ((2012) ) Co-occurrence of different pathologies in dementia: Implications for dementia diagnosis. J Alzheimers Dis 30: , 909–917. |
[71] | Rabinovici GD , Carrillo MC , Forman M , DeSanti S , Miller DS , Kozauer N , Petersen RC , Randolph C , Knopman DS , Smith EE , Isaac M , Mattsson N , Bain LJ , Hendrix JA , Sims JR ((2016) ) Multiple comorbid neuropathologies in the setting of Alzheimer’s disease neuropathology and implications for drug development. Alzheimers Dement (N Y) 3: , 83–91. |
[72] | Fischer DL , Seeley WW ((2023) ) A precision medicine approach to dementia care: Syndrome, etiology, and copathology. Pract Neurol 2023: , 17–22. |
[73] | Liu M-N , Lau C-I , Lin C-P ((2019) ) Precision medicine for frontotemporal dementia. Front Psychiatry 10: , 75. |