Computational Evaluation of Azadirachta indica-Derived Bioactive Compounds as Potential Inhibitors of NLRP3 in the Treatment of Alzheimer’s Disease
Abstract
Background:
The development of therapeutic agents against Alzheimer’s disease (AD) has stalled recently. Drug candidates targeting amyloid-β (Aβ) deposition have often failed clinical trials at different stages, prompting the search for novel targets for AD therapy. The NLRP3 inflammasome is an integral part of innate immunity, contributing to neuroinflammation and AD pathophysiology. Thus, it has become a promising new target for AD therapy.
Objective:
The study sought to investigate the potential of bioactive compounds derived from Azadirachta-indica to inhibit the NLRP3 protein implicated in the pathophysiology of AD.
Methods:
Structural bioinformatics via molecular docking and density functional theory (DFT) analysis was utilized for the identification of novel NLRP3 inhibitors from A. indica bioactive compounds. The compounds were further subjected to pharmacokinetic and drug-likeness analysis. Results obtained from the compounds were compared against that of oridonin, a known NLRP3 inhibitor.
Results:
The studied compounds optimally saturated the binding site of the NLRP3 NACHT domain, forming principal interactions with the different amino acids at its binding site. The studied compounds also demonstrated better bioactivity and chemical reactivity as ascertained by DFT analysis and all the compounds except 7-desacetyl-7-benzoylazadiradione, which had two violations, conformed to Lipinski’s rule of five.
Conclusion:
In silico studies show that A. indica derived compounds have better inhibitory potential against NLRP3 and better pharmacokinetic profiles when compared with the reference ligand (oridonin). These compounds are thus proposed as novel NLRP3 inhibitors for the treatment of AD. Further wet-lab studies are needed to confirm the potency of the studied compounds.
INTRODUCTION
Alzheimer’s disease (AD) is a progressive neurodegenerative disease characterized by memory loss and cognitive decline which worsens over time. AD impacts behavior, communication, visuospatial orientation, motor systems, and other cognitive functions [1]. AD is the primary cause of dementia in persons above 60 years and one of the major causes of disability in seniors [2]. AD dementia poses significant challenges for patients, caregivers, and healthcare systems providers, with an estimated $321 billion projected spent on AD care in 2022 in the United States alone [3]. Currently, no treatment options reverse dementia progression, and the associated mortality is staggering, with AD causing more deaths than prostate and breast cancers combined.
Various hypotheses regarding the mechanism behind AD etiology and progression have been put forward. The two main neuropathological indicators of AD are the build-up of plaque-like extracellular amyloid-β (Aβ) and the generation of intracellular neurofibrillary tangles [4]. Cholinergic system dysfunction [5], neuroinflammation induced by astrocytes and microglia [6], NMDA dysregulation and dysfunction in calcium ion homeostasis [7], cerebrovascular dysregulation [8], and many other hypotheses have also been put forward. Despite extensive research into this area, treatment options are minimal. Current options, including the NMDA receptor antagonist, memantine, and acetylcholinesterase inhibitors, donepezil, rivastigmine, and galantamine, fail to reverse symptoms or slow disease progression. While these medications improve patients’ quality of life, their effects are not continuous and eventually fail to benefit patients [9]. The US Food and Drug Administration (FDA) recently approved the novel anti-amyloid monoclonal antibody, aducanumab, for AD treatment [10]. Aducanumab was shown to reduce brain Aβ plaques during clinical trials. However, its clinical efficacy is meager [11] and currently holds no cost-effectiveness for patients most especially in developing nations like Nigeria [12].
Over the past 20 years, many anti-AD drugs, mainly targeted at limiting Aβ plaque formation and aggregation, have failed clinical trials at various stages and for different reasons [13]. It is well known that AD is not a single-pathophysiology disease, as numerous etiologies are interlinked in a loop-like manner [14]. Recently, a better understanding of AD pathogenesis has led to the discovery of newer targets and exploration of these targets for developing curative AD therapies. One of such newer targets is the NLRP3 inflammasome [15].
Inflammasomes are known to induce inflammation in immune cells. The nucleotide-binding domain (Fig. 1) leucine-rich repeat and pyrin domain-containing receptor protein 3 (NLRP3) is a tripartite protein that belongs to the NALP family known for its role in apoptosis and inflammation. NLRP3 is structurally characterized by the presence of an amino-terminal pyrin domain, a central nucleotide-binding and oligomerization domain (NOD; also known as the NACHT domain), and a C-terminal leucine-rich repeat domain [16]. NLRP3 is found in the cytosol of granulocytes, monocytes, dendritic cells, T and B cells, epithelial cells and osteoblasts [17, 18] and predominantly expressed in macrophages, and encoded by the NLRP3 gene located on the long arm of chromosome 1 [15]. Studies have shown that inflammation caused by viral or bacterial infections contributes to pro-inflammatory activation of the central nervous system (CNS) resident immune cells, including astrocytes and microglia, resulting in neuronal death. Since the uncovering of NLRP3 as a major inflammasome associated with numerous age-related inflammation such as gout, multiple sclerosis, diabetes, ulcerative colitis, and AD, NLRP3 has received intense consideration as a therapeutic target via its effects on activated astrocytes and microglia found to be enriched in genes associated with AD [19].
Fig. 1
Binding pocket of the NLRP3 NACHT domain generated from its co-crystalized inhibitor.
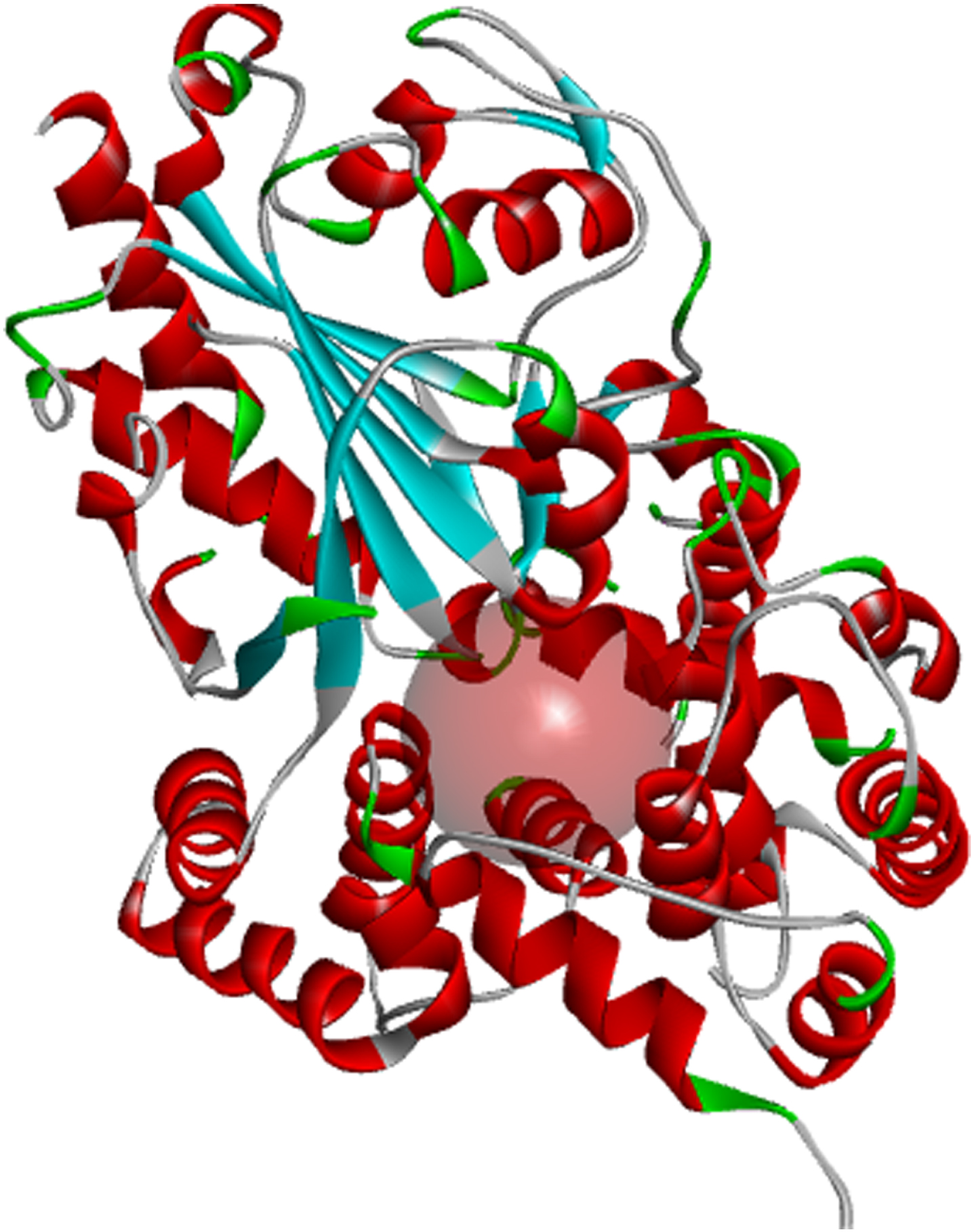
Upon activation via Aβ deposition, NLRP3 regulates the initialization of caspase-1, thereby stimulating the development and release of the active cytokines [20]. These cytokines (interleukin-1 beta precursor protein (pro-IL-1b) and pro-IL-18) enhance Aβ aggregation by reducing Aβ phagocytosis, leading to increased tau phosphorylation. The formation of tau monomers and oligomers and Aβ aggregation, contributes to other pathological changes in AD [15]. The link between the deposition of amyloid and the formation of tangles is largely involved in AD pathogenesis and is targeted in drug development. Inhibiting NLRP3 activity reduces levels of initialized interleukin1β (IL-1β) and IL-18, which inhibits both amyloid deposition and neurofibrillary tangle formation [21].
Several small molecules show efficacy in inhibiting NLRP3 activity. Oridonin is an electron-loving natural diterpenoid derivative present in Rabdosia rubescens. In East Asian traditional medicine, the herb Rabdosia rubescens, also known as Donglingcao, is used in the treatment of inflammation and cancer [22]. Oridonin inhibits NLRP3 by forming a covalent bond with Cys279 in the NLRP3 NACHT domain [23]. The bonding of oridonin to Cys279 blocks the chemical interaction between NLRP3 and NEK7, which is responsible for NLRP3 conformational change and activation [24]. This mechanism underlies oridonin’s anti-inflammatory activity, leading to its development as a potential therapeutic agent against several NLRP3-driven inflammatory diseases. Several NLRP3 inhibitory small molecules are currently in preclinical or clinical trials, and the prospects are exciting [23].
Plant sources have always been considered in the search for novel AD therapies [25]. Between 1999 and 2013, 31 (28%) of the 112 medications approved by the FDA were either natural compounds or their derivatives [26]. Several studies have described the potential of plant-derived bioactive compounds in AD treatment via several mechanisms, including acetylcholinesterase and butyrylcholinesterase inhibition [27], inhibition of Aβ accumulation via BACE-1 inhibition [28], monoamine oxidase-B inhibition [29], and inhibition of tau aggregation [30]. Oridonin is a plant-derived bioactive, and its inhibitory activity on the NLRP3 inflammasome highlights the potential of plant-based compounds as therapeutic agents for AD via NLRP3 receptor targeting.
Azadirachta indica (Neem) is an evergreen tree that belongs to the family Meliaceae and is indigenous to the Indian subcontinent, South East Asia, and parts of Africa. Due to its diverse and structurally complex phytochemicals, neem is extensively used in traditional medical practices, particularly Indian traditional medicine as a therapeutic agent against various conditions including skin infections, chicken pox, rheumatism, cancers, respiratory and digestive problems [31]. A. indica has been validated in many disease models and has been reported to have antioxidant, anti-inflammatory, anti-cancer, anti-diabetic, neuroprotective, and cardioprotective properties [32]. Previous research has described the potency of A. indica extract in resolving experimental models of AD [33], although the exact mechanisms are unknown. However, a study confirmed the inhibitory activity of neem-derived bioactive compounds against tau phosphorylation in vitro [34].
Herein, we investigated the therapeutic potential of A. indica derived compounds, including 7-deacetyl-7-benzoylazadiradione, 7-deacetyl-7-benzoylgedunin, 17-hydroxyazadiradione, nimbiol, nimbolide, chlorogenic acids, and quercetin [31] as NLRP3 inhibitors with reference to the natural ligand oridonin with known NLRP3 inhibitory property using computer aided approach. We utilized structural bioinformatics via molecular docking, advanced theoretical chemistry via density functional theory analysis, and pharmacokinetic and drug-likeness study. From the results, new molecules were suggested as therapeutic agents against AD via NLRP3 inhibition.
MATERIALS AND METHODS
Protein preparation
The 3-dimensional (3D) structure of the NLRP3 NACHT domain in complex with a potent inhibitor was obtained from the protein data bank (PDB) (http://rcsb.org) with PDB ID: 7ALV [35]. Discovery Studio Visualizer was used in preparing the retrieved protein for docking. Protein preparation involved removing the complexed inhibitor and water molecules from the protein while hydrogen bonds and missing amino acids were added. Using the Auto Dock Vina Wizard integrated in the PyRx Virtual Screening Tool, the prepared protein was then converted to Protein Data Bank, Partial Charge & Atom Type (PDBQT) format.
Ligand preparation
Medicinal bioactive compounds of A. indica leaves were identified from published literature [31]. The 3-dimensional structures of the bioactive compounds, including 7-deacetyl-7-benzoylazadiradione, 7-dea-cetyl-7-benzoylgedunin, nimbiol, 17-hydroxyazadi-radione, quercetin, nimbolide, and chlorogenic acids were retrieved from the PubChem library (https://pubchem.ncbi.nlm.nih.gov/). The 3-dimensional structure of Oridonin, a compound with known NLRP3 inhibitory activity, was also retrieved from the PubChem library. Using the Open babel tool integrated in the PyRx Virtual Screening Tool, the ligands including the standard were converted to AutoDock ligands (PDBQT) after energy minimization using the universal force field (UFF).
Receptor-grid generation and molecular docking
The coordinates and size of the NLRP3 NACHT active site was generated following the selection of various amino acid at the active site through the use of the receptor grid generation module on PyRx software. The x, y, and z grid sizes were 14.09, 33.96, and 123.68, respectively. The prepared ligands were then docked into binding pocket of NLRP3 using the Vina Wizard tool embedded on PyRx with exhaustiveness set to 8.
Density functional theory analysis
Density functional theory (DFT), an advance theoretical chemistry approach was employed to probe the physicochemical properties of A. indica bioactive compounds and to distinguish bioactive compounds with outstanding biological activities. The most stable conformer was employed for the DFT quantum calculations after a conformer distribution examination was carried out on each of the bioactive compounds using B3LYP functional methods [36], with the 6–31G* basis set [37] implemented in Spartan 14 computational software on a HP EliteBook 840 computer of processor intel® core(TM) i5-53000 CPU @ 2.30 GHz, 512 GB SSD, 8.00 GB ram specification. Several calculations are described below, including highest occupied molecular orbital energy (EHOMO), lowest unoccupied molecular orbital energy (ELUMO), ionization energy (I), electron affinity (A), chemical hardness (η), chemical softness (δ), chemical potential (μ), electronegativity (χ), enthalpy (AU), electron energy, dipole moment (D), and Gibb’s free energy.
Energy bandgap (Eg) was computed from differences between ELUMO and EHOMO as shown in Equation (1)
(1)
(2)
(3)
Parr and Pearson [39] calculations were used to obtain the electronegativity (χ) and chemical hardness (η) of the compounds.
(4)
(5)
Also, chemical softness (δ) is described as inverse of chemical hardness,
(6)
Pharmacokinetic/ADMET screening
Pharmacokinetic screening of the docked bioactive compounds was analyzed using admetSAR server (https://lmmd.ecust.edu.cn/) [40]. Compounds were assessed based on pharmacokinetic properties and were also screened for drug likeness, Lipinski’s rule of five using the SwissADME server (https://www.swissadme.ch) [41].
RESULTS
Fifteen (15) bioactive derivatives of A. indica were identified from literature search. These include 7-desacetyl-7-benzoylazadiradione, 7-deacetyl-7-benzoylgedunin, 17-hydroxyazadiradione, Quercetin, Nimbiol, Nimbolide, Chlorogenic acid, Curcumin, 6-Deacetylnimbinene, Nimbandiol, Flufenamic acid, Pterostilbene, Resveratrol, Ascorbic acid, and Sulforaphane. The binding pocket of the NACHT domain was generated using its co-ligand inhibitor (Fig. 1). The amino acids with which the co-ligand inhibitor formed principal interactions were utilized in grid generation. These residues include Ala227, Ala228, Arg351, Pro352, Met408, Ile411, Thr239, Phe575, Arg578, Leu628, Thr632, and Met661 (Fig. 2). The generated grid box had the size; X = 14.0878; Y = 33.9602; and Z = 123.6774.
Fig. 2
2D interaction between co-ligand inhibitor and amino acid residues in the NLRP3 binding pocket. Residues utilized in grid generation.
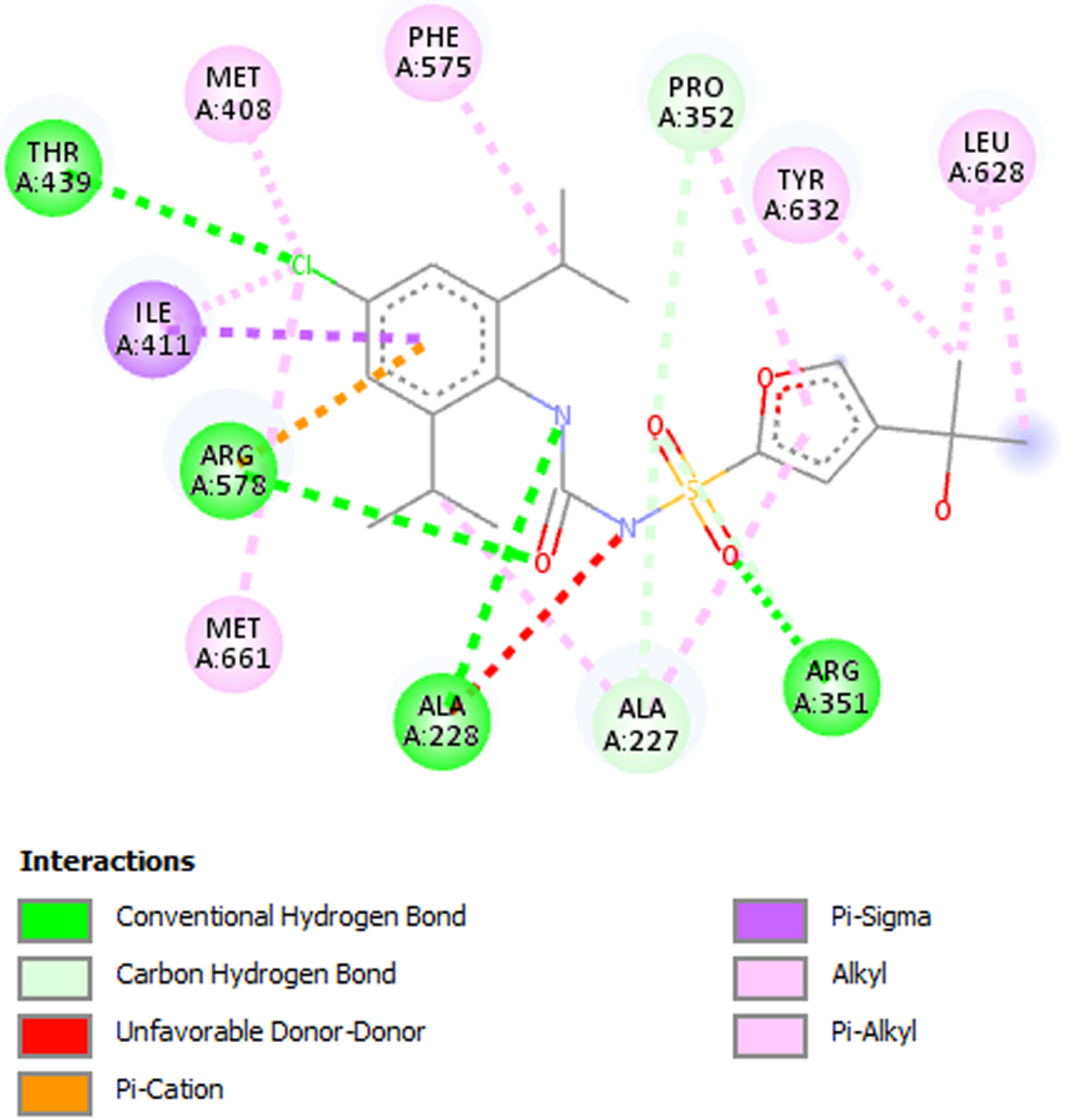
The test compounds, oridonin, and the isolated co-ligand inhibitor were docked against the prepared NLRP3 protein, optimally saturating the binding pocket (Figs. 3 and 4) and forming principal interactions with the Tyr632, Glu629, Gln624, Ala228, Ala227, Arg351, and Arg578 residues (Fig. 5).
Fig. 3
Test compounds, oridonin (orange), and co-ligand inhibitor (brown) optimally saturated the NACHT binding pocket.
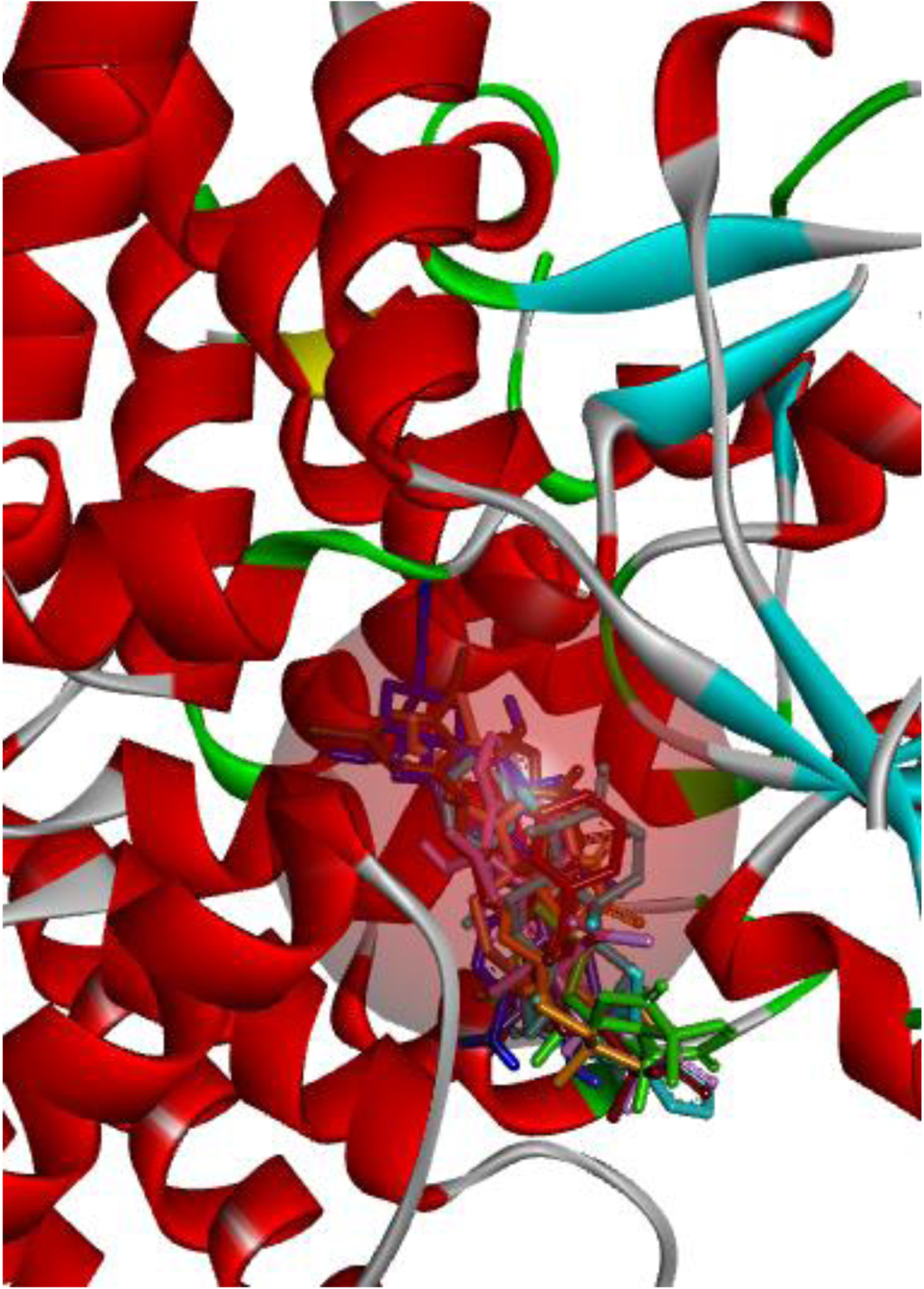
Fig. 4
The figure (a-h) represents the 3D-molecular interaction of the docked compounds against NLRP3. a) 7-desacetyl-7-benzoylazadiradione; b) 7-deacetyl-7-benzoylgedunin; c) 17-hydroxyazadiradione; d) Quercetin; e) Nimbiol; f) Nimbolide; g) Chlorogenic acid; h) Oridonin.
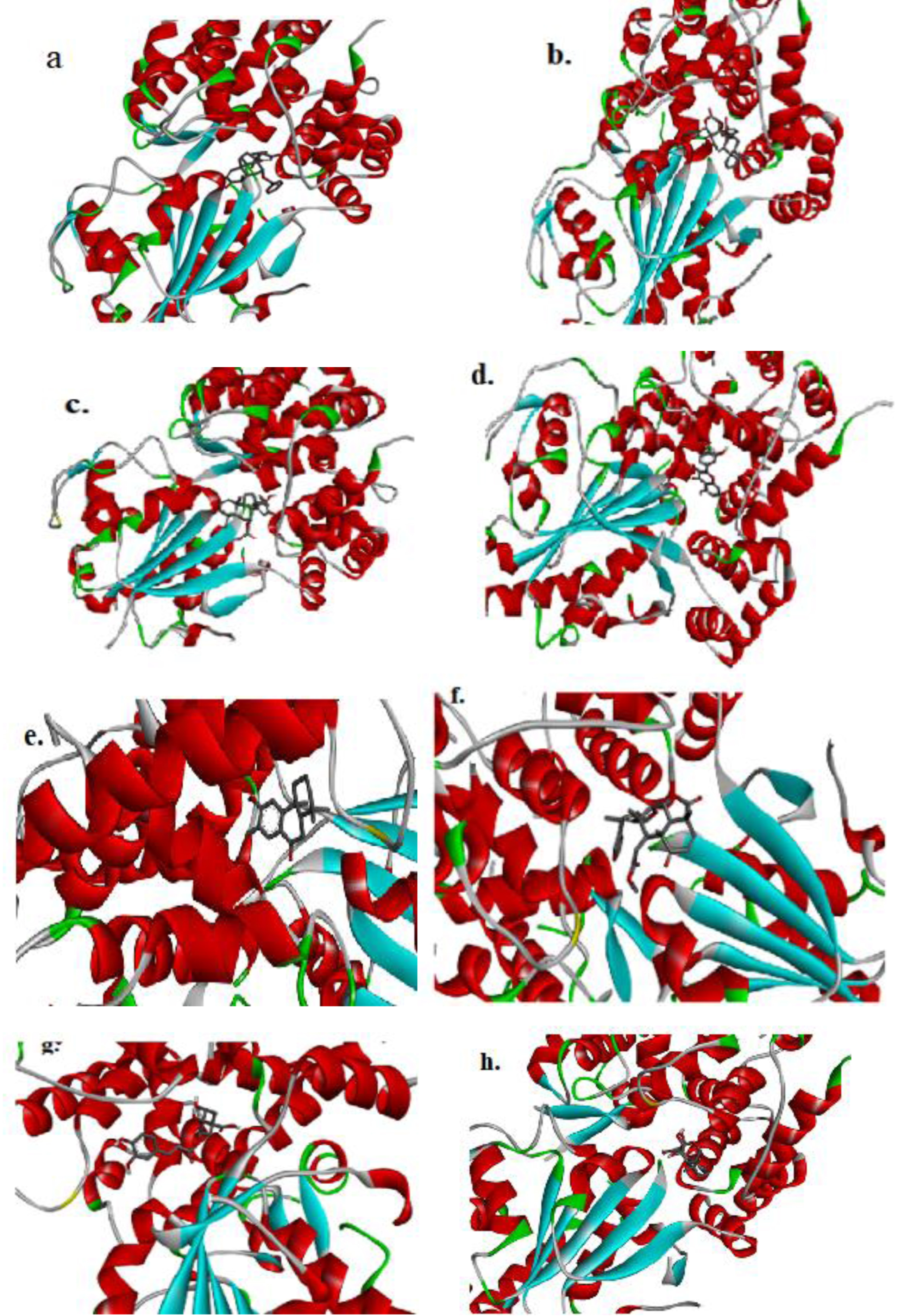
Fig. 5
The figure (a-h) represents the 2D-molecular complex of the docked compounds against NLRP3 after visualization using Discovery Studio. a) 7-desacetyl-7-benzoylazadiradione; b) 7-deacetyl-7-benzoylgedunin; c) 17-hydroxyazadiradione; d) Quercetin; e) Nimbiol; f) Nimbolide; g) Chlorogenic acid; h) Oridonin
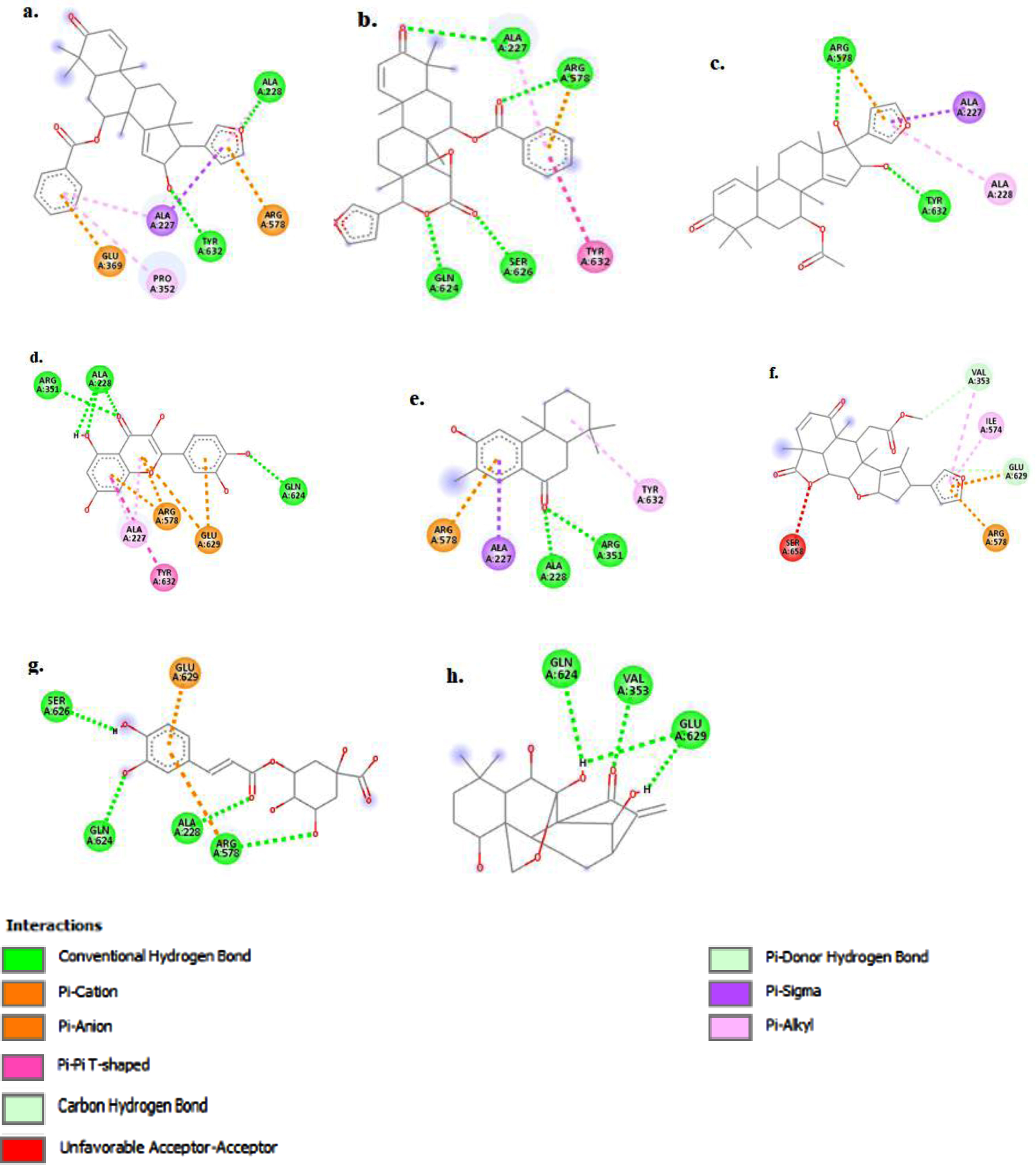
Following initial receptor-ligand docking, seven of the screened compounds demonstrated better binding affinity than Oridonin used as standard. Only these compounds are elaborated on and subjected to DFT analysis and pharmacokinetic screening.
Molecular interactions
The top-scoring compound, 7-desacetyl-7-benzoylazadiradione, with a docking score of –10.6 kcal/mol (Table 1), formed primary interactions with Ala227, Ala228, Pro352, Glu369, Arg578, and Tyr632 (Fig. 5a). 7-desacetyl-7-benzoylazadiradione formed two hydrogen bond interactions with Ala228 and Tyr632 yielding strong interactions with these sites. The amino acids Ala228 and Tyr632 are essential components of the NLRP active site, with the co-crystallized inhibitor binding to these amino acids to accomplish inhibition (Fig. 2). Strong interactions between the test ligands and these amino acid residues are thus, expected to produce inhibition. The benzene ring of 7-desacetyl-7-benzoylazadiradione stacked against Pro352 and Ala227 residues to form non-covalent pi-alkyl bonds and against Glu369, forming another non-covalent pi-anion bond (Fig. 5b). The second-highest-ranking compound, 7-deacetyl-7-benzoylgedunin, predominantly formed conventional hydrogen bond interactions with the Gln624, Ser626, Ala227, and Arg578 residues, while its benzene ring formed non-covalent pi-pi interaction with the Tyr632 residue at the NACHT domain.
Table 1
Molecular docking score of Azadirachta indica compounds and FDA-approved drug
Compound | Docking Score |
Kcal/mol | |
7-desacetyl-7-benzoylazadiradione | –10.6 |
7-deacetyl-7-benzoylgedunin | –10.4 |
Co-ligand Inhibitor | –9.2 |
17-hydroxyazadiradione | –8.8 |
Quercetin | –8.6 |
Nimbiol | –8.5 |
Nimbolide | –8.5 |
Chlorogenic acid | –8.3 |
**Oridonin | –8.2 |
The bioactive compound 17-hydroxyazadiradione formed two conventional hydrogen bond interactions with Tyr632 and Arg578 (Fig. 5c). Meanwhile, its furan moiety forms non-covalent Pi-alkyl, Pi-cation, and Pi-Sigma interactions with the Ala228, Arg578, and Ala227 residues respectively of the NLRP3 NACHT domain. Quercetin forms hydrogen bond interactions with Ala 228, Arg 351, and Gln 624, non-covalent Pi-Alkyl interactions are observed between its chromene moiety and the Arg 578 and Ala 227 residues respectively, while Pi-Anion interaction is formed between the pyran and phenyl components of quercetin and the Glu 629 amino acid residue (Fig. 5d).
Nimbiol forms two hydrogen bonds with the Ala 228 and Arg 351 residues and non-covalent Pi-Cation, Pi-Sigma and Pi-Alkyl bonds with Arg 578, Ala 227 and Tyr 632 residues, respectively (Fig. 5e). Interestingly, Nimbolide forms two covalent carbon-hydrogen interactions with Val 353 and Glu 369 and an unfavorable acceptor-acceptor pair with Ser 658 (Fig. 5f). Chlorogenic acid forms four hydrogen bonds with Ser 626, Gln 624, Ala 228, and Arg 578, indicating a strong binding interaction with the binding pocket of NLRP3 (Fig. 5 g).
Oridonin demonstrated principal interactions with the Val353, Gln624, and Glu 629 residues, forming conventional hydrogen bonds with all three residues (Fig. 5h). Key conformational differences were observed between the co-ligand inhibitor complexed with NLRP3, and the isolated and re-docked co-ligand inhibitor. As such, the redocked inhibitor formed covalent hydrogen bonds with the Arg351, Arg578, and Glu629 residues, carbon-hydrogen bond with the Pro352 residue, and alkyl bond with the Met408 residue. Non-covalent interactions, including Pi-Alkyl, Pi-Cation, Pi-Sulfur, and Pi-Sigma bonds were also observed between the inhibitor and NACHT domain residues, including Ala227, Val353, Ile411, Met661, and Pro352. An unfavorable bump (donor-donor interaction) was also observed with the Ala228 residue. Similar interaction also existed between the inhibitor and NLRP3 protein in the natural state.
DFT analysis
Initial DFT analyses revealed that 7-deacetyl-7-benzoylazadiradione and 7-deacetyl-7-benzoyl-gedunin both have molecular weights that are higher than 500 AMU whereas the values for the other test compounds fell below 500 AMU in each case. Further, Quercetin gave the lowest dipole moment (0.22) while the electronic energy (AU) of all the compounds ranged from 850.832 to 1805.682 AU (Table 2).
Table 2
Molecular weight, electronic energy, enthalpy, dipole moment values were obtained via DFT at B3LYP/6–31G* level
Compound | Molecular Weight (AMU) | Electronic Energy (AU) | Enthalpy (AU) | Dipole Moment (D) |
7-deacetyl-7-benzoylgedunin | 544.644 | –1805.682 | –1805.699 | 3.85 |
7-desacetyl-7-benzoylazadiradione | 512.646 | –1655.265 | –1655.278 | 3.38 |
17-hydroxyazadiradione | 466.574 | –1538.733 | –1538.747 | 3.27 |
Quercetin | 302.238 | –1104.176 | –1104.209 | 0.22 |
Nimbiol | 272.388 | –850.832 | –850.843 | 2.81 |
Nimbolide | 466.530 | –1573.432 | –1573.449 | 5.35 |
Chlorogenic acid | 354.311 | –1297.544 | –1297.575 | 1.90 |
**Oridonin | 364.438 | –1230.255 | –1230.270 | 4.84 |
The DFT analyses at BLYP/6-31*G level showed that 7-deacetyl-7-benzoylgedunin had the highest HOMO (highest occupied molecular orbital energy) value followed by that of 17-hydroxyazadiradione (Table 3). The LUMO (lowest unoccupied molecular orbital energy) values of the test compounds ranged from –1.06 eV to –1.84 eV, while the chemical hardness was between 1.82 eV and 2.52 eV. Overall, Nimbiol gave the highest binding energy gap among all the test compounds (–5.04 eV) among all the compounds which indicates that it binds more efficiently to the target site when compared with Oridonin that has a binding energy gap of 4.81 eV (Table 3). The optimized structures, the HOMO and LUMO structures of the test compounds were presented in Table 4.
Table 3
Chemical parameters obtained by via DFT at the B3LYP/6-31*G Level
Compound | HOMO | LUMO | Eg | I | A | η | δ | μ | χ |
(eV) | (eV) | (eV) | (eV) | (eV) | (eV) | (ev-1) | (eV) | (eV) | |
7-deacetyl-7-benzoylgedunin | –6.40 | –1.58 | –4.82 | 6.40 | 1.58 | 2.41 | 0.41 | –3.99 | 3.99 |
7-desacetyl-7-benzoylazadiradione | –6.02 | –1.58 | –4.44 | 6.02 | 1.58 | 2.22 | 0.45 | –3.80 | 3.80 |
17-hydroxyazadir adione | –6.25 | –1.57 | –4.68 | 6.25 | 1.57 | 2.34 | 0.43 | –3.91 | 3.91 |
Quercetin | –5.48 | –1.84 | –3.64 | 5.48 | 1.84 | 1.82 | 0.55 | –3.66 | 3.66 |
Nimbiol | –6.10 | –1.06 | –5.04 | 6.10 | 1.06 | 2.52 | 0.40 | –3.58 | 3.58 |
Nimbolide | –6.05 | –1.84 | –4.21 | 6.05 | 1.84 | 2.10 | 0.48 | –3.95 | 3.95 |
Chlorogenic acid | –6.06 | –1.78 | –4.28 | 6.06 | 1.78 | 2.14 | 0.47 | –3.92 | 3.92 |
**Oridonin | –6.29 | –1.48 | –4.81 | 6.29 | 1.48 | 2.405 | 0.41 | –3.89 | 3.89 |
Table 4
The compound, optimized structure, HUMO and LUMO
Compound | Optimized Structure | HUMO | LUMO |
7-deacetyl-7- benzoylgedunin | ![]() | ![]() | ![]() |
7-desacetyl-7- benzoylazadiradione | ![]() | ![]() | ![]() |
17 hydroxyazadiradione | ![]() | ![]() | ![]() |
Quercetin | ![]() | ![]() | ![]() |
Nimbolide | ![]() | ![]() | ![]() |
Nimbiol | ![]() | ![]() | ![]() |
Chlorogenic acid | ![]() | ![]() | ![]() |
**Oridonin | ![]() | ![]() | ![]() |
Pharmacokinetic analysis
A comparison of the pharmacokinetic properties of the test and control compounds is given in Table 4. Besides quercetin, none of the compounds was found to be mutagenic or carcinogenic. 7-desacetyl- 7 benzoylazadiradione and 7-deacetyl-7- benzoylgedunin had similar acute toxicity score as the FDA approved drug oridonin, while 17- hydroxyaza diradione had the lowest acute toxicity score. Besides quercetin and chlorogenic acid, all the compounds from neem as evaluated in the current study were positive for crossing the blood-brain barrier (BBB). However, the control drug, oridonin, does not cross the BBB. Further, oridonin had lower plasma protein binding score when compared to all the test compounds besides chorogenic acid (Table 5).
Table 5
Pharmacokinetic prediction
7-desacetyl- 7 benzoylaza diradione | 7-deacetyl-7-benzoylgedunin | 17-hydroxyaza diradione | Quercetin | Nimbiol | Nimbolid e | Chlorogenic acid | Oridonin | |
Ames Mutagenesis | – | – | – | + | – | – | – | – |
Acute Oral Toxicity (c) | III | III | I | II | III | III | III | III |
Blood-Brain Barrier | + | + | + | – | + | + | – | – |
Biodegradation | – | – | – | – | – | – | – | – |
Caco-2 | – | – | – | – | + | – | – | – |
Carcinogenicity (binary) | – | – | – | – | – | – | – | – |
CYP1A2 Inhibition | – | – | + | + | + | – | – | – |
CYP2C19 Inhibition | – | – | – | – | – | – | – | – |
CYP2C9 Inhibition | – | – | – | – | – | – | – | – |
CYP2C9 Substrate | – | – | – | – | – | – | + | – |
CYP2D6 Inhibition | – | – | – | – | – | – | – | – |
CYP2D6 Substrate | – | – | – | – | – | – | – | – |
CYP3A4 Inhibition | + | + | – | + | – | + | – | – |
CYP3A4 Substrate | + | + | + | + | + | + | + | + |
CYP inhibitory promiscuity | – | – | – | + | – | + | – | – |
Hepatotoxicity | – | – | – | + | – | + | – | – |
Human Ether-a-go- go-Related Gene Inhibition | + | + | + | – | – | + | – | – |
Human Intestinal Absorption | + | + | + | + | + | + | + | + |
Human oral bioavailability | – | – | – | – | – | – | – | – |
Acute Oral Toxicity | 2.4061 | 2.4193 | 1.7991 | 2.5264 | 3.0243 | 2.7556 | 1.8352 | 3.6448 |
P-glycoprotein inhibitor | + | + | + | – | – | + | – | – |
P-glycoprotein substrate | – | + | – | – | – | + | – | – |
Plasma protein binding | 1.1310 | 1.0222 | 0.8995 | 1.1638 | 0.7934 | 0.9812 | 0.6339 | 0.7676 |
Subcellular localization | Mitochondria | Mitochondria | Mitochondria | Mitochondria | Mitochondria | Mitochondria | Mitochondria | Mitochondria |
UGT Catalyzed | – | – | + | + | + | – | – | + |
Water Solubility | –4.8633 | –4.7909 | –4.5866 | –2.9993 | –4.9274 | –4.4551 | –2.4571 | –3.5310 |
Following evaluation for drug-likeness, it was observed that apart from 7-desacetyl- 7- benzoylazadiradione which had two violations, all the other test compounds had either one or zero violation of the Lipinski’s rule of five (Table 6).
Table 6
Drug-likeness predictions
Compound | Chemical Structure | Chemical Formula | Mol Wt (g/Mol) | Num of HB Acceptor | Num of HB Donor | ILOGP | Lipinski’s rule of five violations |
7-desacetyl- 7-benzoylazadiradione | ![]() | C33H36O5 | 512.64 | 5 | 0 | 3.39 | No; 2 violations: MW >500, MLOGP >4.15 |
7-deacetyl- 7-Benzoylgedunin | ![]() | C33H36O7 | 544.63 | 7 | 0 | 3.88 | Yes; 1 violation: MW >500 |
17-hydroxyaza diradione | ![]() | C28H34O6 | 466.54 | 6 | 1 | 3.02 | Yes; 0 violation |
Quercetin | ![]() | C15H10O7 | 302.24 | 7 | 5 | 1.63 | Yes; 0 violation |
Nimbiol | ![]() | C18H24O2 | 272.38 | 2 | 1 | 2.86 | Yes; 0 violation |
Nimbolide | ![]() | C27H30O7 | 466.52 | 7 | 0 | 3.51 | Yes; 0 violation |
Chlorogeni c acid | ![]() | C16H18O9 | 354.31 | 9 | 6 | 0.96 | Yes; 1 violation: NhorOH >5 |
**Oridonin | ![]() | C20H28O6 | 364.63 | 6 | 4 | 1.98 | Yes; 0 violation |
DISCUSSION
The NLRP3 protein is triggered in response to inflammatory states and is involved in the progression of AD. Inhibition of NLRP3 activity via ligands, such as oridonin, that bind to the NLRP3 NACHT domain has demonstrated potentials in AD therapy [23, 42]. Various studies have pointed out the potential of A. indica (Neem plant) as a therapy for neurological ailments, including AD [33, 43]; however, they fail to demonstrate the mechanisms by which A. indica act in AD therapy. This study evaluates the inhibitory potential of A. indica-derived bioactive substances on NLRP3 activity via computational methods.
In drug development using computational tools, the molecular docking procedure helps to predict the structure of a protein (receptor)-ligand (drug) complex by sampling different conformers of the ligand at the target site of the protein and then ranking the different conformations [44]. The binding affinity (kcal/mol) expresses how tightly the ligand binds to the protein scaffold, with more negative binding energies representing more stable receptor-ligand interactions (Table 1).
Following molecular docking of the test and control compounds against NLRP3, the bioactive compounds from A. indica demonstrated favorable binding activity at the NLRP3 binding site (Fig. 6). As seen in Table 1, 7-desacetyl-7-benzoylazadiradione had the highest binding affinity (–10.6 kcal/mol) and chlorogenic acid had a lowest binding affinity (–8.3 kcal/mol), with all A. indica-derived compounds, and the co-ligand inhibitor having higher binding affinities than oridonin (–8.2 kcal/mmol).
Fig. 6
The graph shows the molecular docking score of Azadirachta indica compounds and the reference ligand against NLRP3 target protein
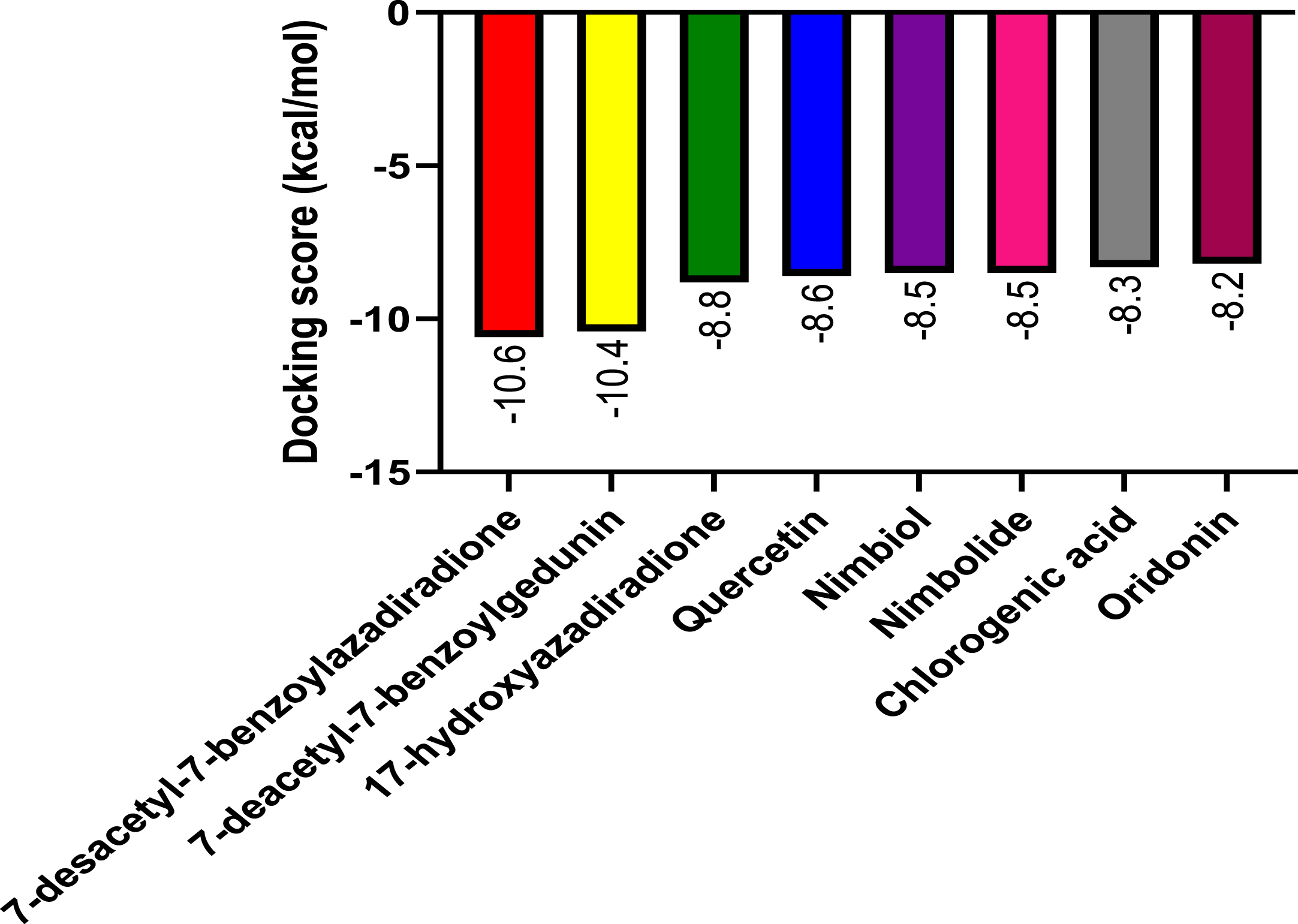
Previous computational studies performed on the NLRP3 protein confirmed the stability of the NACHT active site, with major interactions formed between inhibitory ligands and the Ala227, Ala228, Pro352, Ile411, Phe575, and Arg578 residues in the most stable conformations [45]. Besides Phe575, all of these residues were essential in interactions between the A. indica-derived ligands and the NLRP3 protein.
Ligand-protein binding affinity describes how tightly a drug candidate can bind to its target protein, and is theoretically a primary measure for drug candidate selection [46]. Based on this criterion, it is expected that 7-desacetyl-7-benzoylazadiradione will have greater inhibitory effect on the NLRP3 protein compared with oridonin and other A. indica-derived compounds. However, it has been established that greater binding affinity does not always indicate better potential as a drug candidate as compounds may have unique in vitro characteristics and in vivo clinical efficacy [47]. The docking and binding affinities reported herein do serve as good predictors for further wet-lab experiments, including enzymatic assays.
Density functional theory analysis
Frontier molecular orbitals
The frontier molecular orbital (FMO) theory can be described as a model that is utilized to describe reactivity of chemical compounds. The highest occupied and lowest vacant molecular orbitals (HOMO and LUMO) is a key feature of the frontier electron theory. The HOMO (highest occupied molecular orbital) represents the state at which molecules have the highest ability to donate an electron. In contrast, the LUMO (lowest unoccupied molecular orbital) represents the state at which molecules have the highest ability to accept an electron. Conversely, molecules with higher HOMO values and lower LUMO values have lower stability and higher reactivity, and those with lower HOMO and higher LUMO show more stability and less reactivity [48].
Table 3 shows the HOMO values for the studied bioactive compounds. Arranged in increasing order, 7-deacetyl-7-benzoylgedunin < 17-hydroxyazadira-dione < Nimbiol < Chlorogenic acid < Nimbolide < 7-desacetyl-7-benzoylazadiradione < Quercetin. With a HOMO value of –5.48 eV, quercetin demonstrates the lowest stability and better tendency to donate electrons to the target site than other studied compounds. Table 3 also shows the values for the LUMO of the studied bioactive compounds, which arranged in decreasing order gives Nimbiol > 17-hydro-xyazadiradione>7-desacetyl-7-benzoylazadiradione>7-desacetyl-7-benzoylazadiradione >Chlorogenic acid > Nimbolide>Quercetin. Quercetin and nimbolide have the lowest LUMO values (–1.84 eV), indicating their high reactivity and higher potential to accept electrons from the target receptor than the other studied compounds. Table 4 summarizes the obtained values of HOMO and LUMO for the studied compounds.
The band energy gap predicts the chemical reactivity and stability of chemical compounds. The band energy gap is obtained by calculating the differences between the HUMO and LUMO values. Compounds with large band gaps will be less reactive, hard, and more stable, while compounds with small band gaps will be more reactive, soft, and less stable. The band energy gap of the chemical compounds is in the order: Quercetin < Nimbolide < Chlorogenic acid < 7-desacetyl-7-benzoylazadiradione < 17-hyd-roxyazadiradione < 7-deacetyl-7-benzoylgedunin < Nimbiol. From Table 3, Quercetin pointed out to have the lowest energy band gap (–3.64 eV). This indicates that it is more reactive toward the target site than all other studied bioactive compounds.
Global reactivity descriptors
To understand the chemical reactivity and stability of bioactive chemical compounds towards the target protein, the global reactivity descriptors (GRD) are calculated from the HUMO and LUMO values. The calculated parameters are: I, ionization energy; A, electron affinity; η, chemical hardness; δ, chemical softness; μ, chemical potential; X, electronegativity. The amount of energy required to remove an electron from a molecule is known as ionization energy (I). Low ionization energy suggests that the chemical compound is very reactive, while high ionization energy indicates that the chemical compound is chemically inert and have good stability. The term “energy” refers to the atoms and molecules’ high reactivity [49]. The ionization energies from the table indicate that Quercetin has the lowest ionization energy (5.48 eV) among all the compounds. Compared to other ligands, Quercetin requires a low energy for the removal of outermost electron to the target site.
Electron affinity (A) is the energy generated or released when an electron is added to a neutral molecule. Molecules with high electron affinity are susceptible to accepting electrons easily as compared to molecules with low electron affinity. Table 3 indicates that Quercetin and Nimbolide have the highest values (1.84 eV). The concepts of hardness (δ) and softness (S) clarify the behavior of chemical compounds [49]. Chemical hardness and softness are crucial qualities for determining the stability and reactivity of molecules. It is self-evident that chemical hardness is fundamentally a measure of resistance [50]. The band gap energy of a hard molecule is large, whereas the band gap energy of a soft molecule is small. The soft molecule will be more polarizable than the hard molecule [44]. From Table 3, Nimbiol has the highest value (2.52 eV) while Quercetin has the lowest value (1.82 eV) of chemical hardness. This indicates that Quercetin is the most polarizable compound as compared to other compounds, while Nimbiol is the least polarizable. Regarding chemical softness, Quercetin shows the highest value of 0.55 eV-1, while 7-deacetyl-7-benzoylgedunin has the lowest value of 0.41 eV-1. This indicates that Quercetin is the softest molecule.
Electronegativity is a chemical property that describes an atom’s or functional group’s capacity to attract electrons to itself. Table 3 shows Nimbolide has the highest electronegativity value (3.95 eV), which indicates higher, stronger electrophilic properties in Nimbolide than other bioactive compounds.
Pharmacokinetic properties
ADMET is an abbreviation for Absorption, Distribution, Metabolism, Excretion, and Toxicity. The ADMET properties of a compound helps to predict the pharmacokinetic properties of bioactive molecules [51]. The ADMET properties are shown in Table 5.
Human intestinal absorption (HIA) and the p-glycoprotein (P-gp) are the two pharmacological variables used to determine the absorption of the bioactive compounds. HIA is an essential ADMET property as it plays a vital role in the transportation of drugs to their target. Table 5 shows that all the natural ligands including the standard has a positive value for the HIA. This indicates that the ligands can be absorbed rapidly into the body for systemic circulation. P-gp is known for the ejection of drug molecules from the cell which on the long run reduces the bioavailability of drugs [52]. 17-hydroxyaza diradione and Nimbolide were found to inhibit P-gp. Inhibition of P-gp increases drugs bioavailability and as such, these ligands will be present at the target site at optimum concentrations to exhibit therapeutic effects.
The distribution properties of the ligands were measured based on their permeation through the BBB and action as a substrate of P-gp. The BBB is made up of semi-permeable endothelial cells that is selective on drug molecules that passes through it. Permeation through the BBB by drug molecules gives these molecules access to the extracellular fluid of the CNS where neurons are located [53]. From Table 5, All compounds except Quercetin have a positive value for BBB permeability, indicating that most of the bioactive molecules from A. indica will permeate the BBB and exhibit therapeutic action on the CNS. In contrast, the standard compound, Oridonin, has a negative value for the BBB permeability, suggesting a lack of effect on the CNS. In Addition, all the ligands except Nimbolide are non-P-gp substrate. Therefore, the distribution of Nimbolid e may be restricted by the efflux activity of P-gp.
Drug metabolism is a critical property, as almost 50% of drug excretion is attributable to metabolism by Cytochrome P450 enzymes [54]. The therapeutic effects of an administered drug are greatly dependent on metabolism [55]. About 90% of all drug molecules are metabolized by CYP1A2, CYP2C9, CYP2C19, CYP2D6, and CYP3A4/5, which are the most immensely studied CYP450 enzymes [56, 57]. Table 5 shows that CYP1A2 enzyme is inhibited by 17-hydroxyazadiradione, Quercetin while Quercetin and Nimbolide inhibit CYP3A4. However, the standard oridonin does not inhibit any of these enzymes. Due to this, the bioactive molecules obtained from A. indica may influence a drug-drug interaction when co-administrated with another drug and of which might be minimal.
A number of drug candidates often fail before getting to clinical trials due to toxicity [58]. The toxicity of the bioactive molecules obtained from A. indica was determined by the examination of their carcinogenicity, hepatotoxicity, human ether-a-go-go inhibition, and AMES mutagenesis. All the compounds except Nimbiol were shown to exhibit human ether-a-go-go-inhibition. The repolarization of the cardiac potential is largely mediated by the human ether-a-go-go related gene (hERG) and its inhibition by a chemical substance will lead to the prolongation of the QT interval leading to cardiac arrhythmias. Conversely, none of the compounds were shown to be carcinogenic while only Quercetin exhibits Ames Mutagenicity. Quercetin and Nimbolide were also shown to be hepatotoxic.
Drug likeness (rule of five)
In order to reduce the risk of going outside the drug-likeness space during drug development, the Lipinski’s rule of five is used to guide the drug design efforts which eventually reduce the risk of attrition. The rule of five states that an orally active drug should not flout more than one of the following parameters: 1) not more than 5 hydrogen-bond donors (HBD ≤5), 2) not more than 10 hydrogen bond acceptors (HBA ≤10), 3) Octanol-water partition coefficient not more than 5 (ilog P≤5), 4) molecular mass not greater than 500 Da (MW ≤500 KDa), and 5) topological surface area less than 120 (TPSA <120). With the exception of 7-desacetyl-7-benzoylazadiradione, the bioactive compounds from A. indica conformed to Lipinski’s rule of 5 as seen in Table 6. 7-desacetyl-7-benzoylazadiradione had two violations: molecular weight of 512.65 KDa (>500 KDa) and ilogP of 4.27 (>4.15). Compounds that conform to the rule of 5 generally have better oral bioavailability and permeability through physiologic barriers and are better candidates for drug development. However, this does not mean that compounds that violate Lipinski’s rule do not show potential. Such compounds typically have higher lipophilicity and lower water solubility and require non-conventional delivery systems to ensure bioavailability [59]. Combining the data from the docking results which show that A. indica-derived bioactives have a high binding affinity for the NLRP3 inflammasome, and the pharmacokinetic profile data which show that these compounds have favorable oral bioavailability and toxicity profiles leads us to suggest that Neem-derived bioactive compounds are potential therapeutic agents against AD.
Conclusion
This study sought to identify potential inhibitors of NLRP3 as a therapy for AD by molecular docking of bioactive compounds obtained from A. indica at the NLRP3 NACHT domain. After rigorous molecular docking and density functional theory analysis, the bioactive compounds from A. indica exhibited more stable interaction, had higher binding energies and greater chemical reactivity when compared to the reference compound, Oridonin. The pharmacokinetic evaluations predicted good oral bioavailability and safety of A. indica as a novel therapeutic candidate for AD. Overall, the development of bioactive compounds from A. indica is a promising direction in the treatment of AD. Nevertheless, in vitro and in vivo studies are required to corroborate A. indica compounds in NLRP3-targeted drug development.
ACKNOWLEDGMENTS
The authors sincerely appreciate the guidance, support, and supervision received from Abdulwasiu Ibrahim at each stage of this research work. Also, our sincere appreciation goes to the management and directors of the Institute of Bioinformatics and Molecular Therapeutics, Oshogbo, Osun State, Nigeria for the hands-on training that birth this research work.
FUNDING
The authors have no funding to report.
CONFLICT OF INTEREST
The authors have no conflict of interest to report.
DATA AVAILABILITY
The data supporting the findings of this study are available within this article.
REFERENCES
[1] | DeTure MA , Dickson DW ((2019) ) The neuropathological diagnosis of Alzheimer’s disease. Mol Neurodegener 14: , 32. |
[2] | Prince M , Ali G-C , Guerchet M , Prina AM , Albanese E , Wu Y-T ((2016) ) Recent global trends in the prevalence and incidence of dementia, and survival with dementia. Alzheimers Res Ther 8: , 23. |
[3] | Alzheimer’s Association ((2022) ) 2022 Alzheimer’s disease facts and figures. Alzheimers Dement 18: , 700–789. |
[4] | Vaz M , Silvestre S ((2020) ) Alzheimer’s disease: Recent treatment strategies. Eur J Pharmacol 887: , 173554. |
[5] | Mufson EJ , Counts SE , Perez SE , Ginsberg SD ((2008) ) Cholinergic system during the progression of Alzheimer’s disease: Therapeutic implications. Expert Rev Neurother 8: , 1703–1718. |
[6] | Park J-S , Kam T-I , Lee S , Park H , Oh Y , Kwon S-H , Song J-J , Kim D , Kim H , Jhaldiyal A , Na DH , Lee KC , Park EJ , Pomper MG , Pletnikova O , Troncoso JC , Ko HS , Dawson VL , Dawson TM , Lee S ((2021) ) Blocking microglial activation of reactive astrocytes is neuroprotective in models of Alzheimer’s disease. Acta Neuropathol Commun 9: , 78. |
[7] | Liu J , Chang L , Song Y , Li H , Wu Y ((2019) ) The role of NMDA receptors in Alzheimer’s disease. Front Neurosci 13: , 43. |
[8] | Kisler K , Nelson AR , Montagne A , Zlokovic BV ((2017) ) Cerebral blood flow regulation and neurovascular dysfunction in Alzheimer disease. Nat Rev Neurosci 18: , 419–434. |
[9] | Liu P-P , Xie Y , Meng X-Y , Kang J-S ((2019) ) History and progress of hypotheses and clinical trials for Alzheimer’s disease. Signal Transduct Target Ther 4: , 1–22. |
[10] | FDA, FDA Grants Accelerated Approval for Alzheimer’s Drug, https://www.fda.gov/news-events/press-announcements/fda-grants-accelerated-approval-alzheimers-drug. |
[11] | Knopman DS , Perlmutter JS ((2021) ) Prescribing Aducanumab in the face of meager efficacy and real risks. Neurology 97: , 545–547. |
[12] | Ross EL , Weinberg MS , Arnold SE ((2022) ) Cost-effectiveness of Aducanumab and Donanemab for early Alzheimer disease in the US. JAMA Neurol 79: , 478–487. |
[13] | Mehta D , Jackson R , Paul G , Shi J , Sabbagh M ((2017) ) Why do trials for Alzheimer’s disease drugs keep failing? A discontinued drug perspective for 2010–2015. Expert Opin Investig Drugs 26: , 735–739. |
[14] | Husna Ibrahim N , Yahaya MF , Mohamed W , Teoh SL , Hui CK , Kumar J ((2020) ) Pharmacotherapy of Alzheimer’s disease: Seeking clarity in a time of uncertainty. Front Pharmacol 11: , 261. |
[15] | Zhang Y , Dong Z , Song W ((2020) ) NLRP3 inflammasome as a novel therapeutic target for Alzheimer’s disease. Signal Transduct Target Ther 5: , 37. |
[16] | Schroder K , Tschopp J ((2010) ) The inflammasomes. Cell 140: , 821–832. |
[17] | McCall SH , Sahraei M , Young AB , Worley CS , Duncan JA , Ting JP-Y , Marriott I ((2007) ) Osteoblasts express NLRP3, a nucleotide-binding domain and leucine-rich repeat region containing receptor implicated in bacterially induced cell death. J Bone Miner Res 23: , 30–40. |
[18] | Kummer JA , Broekhuizen R , Everett H , Agostini L , Kuijk L , Martinon F , Bruggen van R , Tschopp J ((2007) ) Inflammasome components NALP 1 and 3 show distinct but separate expression profiles in human tissues suggesting a site-specific role in the inflammatory response. J Histochem Cytochem 55: , 443–452. |
[19] | Bai H , Zhang Q ((2021) ) Activation of NLRP3 inflammasome and onset of Alzheimer’s disease. Front Immunol 12: , 701282. |
[20] | Hamarsheh S , Zeiser R ((2020) ) NLRP3 inflammasome activation in cancer: A double-edged sword. Front Immunol 11: , 1444. |
[21] | Liang T , Zhang Y , Wu S , Chen Q , Wang L ((2022) ) The role of NLRP3 inflammasome in Alzheimer’s disease and potential therapeutic targets. Front Pharmacol 13: , 845185. |
[22] | Tan W , Lu J , Huang M , Li Y , Chen M , Wu G , Gong J , Zhong Z , Xu Z , Dang Y , Guo J , Chen X , Wang Y ((2011) ) Anti-cancer natural products isolated from Chinese medicinal herbs. Chin Med 6: , 27. |
[23] | El-Sharkawy LY , Brough D , Freeman S ((2020) ) Inhibiting the NLRP3 inflammasome. Molecules 25: , 5533. |
[24] | He H , Jiang H , Chen Y , Ye J , Wang A , Wang C , Liu Q , Liang G , Deng X , Jiang W , Zhou R ((2018) ) Oridonin is a covalent NLRP3 inhibitor with strong anti-inflammasome activity. Nat Commun 9: , 2550. |
[25] | Wojtunik-Kulesza K , Oniszczuk T , Mołdoch J , Kowalska I , Szponar J , Oniszczuk A ((2022) ) Selected natural products in neuroprotective strategies for Alzheimer’s disease—a non-systematic review. Int J Mol Sci 23: , 1212. |
[26] | Eder J , Sedrani R , Wiesmann C ((2014) ) The discovery of first-in-class drugs: Origins and evolution. Nat Rev Drug Discov 13: , 577–587. |
[27] | Chen X , Drew J , Berney W , Lei W ((2021) ) Neuroprotective natural products for Alzheimer’s disease. Cells 10: , 1309. |
[28] | Omar SH , Scott CJ , Hamlin AS , Obied HK ((2018) ) Biophenols: Enzymes (β-secretase, cholinesterases, histone deacetylase and tyrosinase) inhibitors from olive (Olea europaea L.). Fitoterapia 128: , 118–129. |
[29] | Zhang C , Yang K , Yu S , Su J , Yuan S , Han J , Chen Y , Gu J , Zhou T , Bai R , Xie Y ((2019) ) Design, synthesis and biological evaluation of hydroxypyridinone-coumarin hybrids as multimodal monoamine oxidase B inhibitors and iron chelates against Alzheimer’s disease. Eur J Med Chem 180: , 367–382. |
[30] | Karakani AM , Riazi G , Mahmood Ghaffari S , Ahmadian S , Mokhtari F , Jalili Firuzi M , Zahra Bathaie S ((2015) ) Inhibitory effect of corcin on aggregation of 1N/4R human tau protein in vitro. Iran J Basic Med Sci 18: , 485–492. |
[31] | Saleem S , Muhammad G , Hussain MA , Bukhari SNA ((2018) ) A comprehensive review of phytochemical profile, bioactives for pharmaceuticals, and pharmacological attributes of Azadirachta indica. Phytother Res PTR 32: , 1241–1272. |
[32] | Sandhir R , Khurana M , Singhal NK ((2021) ) Potential benefits of phytochemicals from Azadirachta indica against neurological disorders. Neurochem Int 146: , 105023. |
[33] | Raghavendra M , Maiti R , Kumar S , Acharya S ((2013) ) Role of aqueous extract of Azadirachta indica leaves in an experimental model of Alzheimer’s disease in rats. Int J Appl Basic Med Res 3: , 37–47. |
[34] | Gorantla NV , Das R , Mulani FA , Thulasiram HV , Chinnathambi S ((2019) ) Neem derivatives inhibits tau aggregation. J Alzheimers Dis Rep 3: , 169–178. |
[35] | Dekker C , Mattes H , Wright M , Boettcher A , Hinniger A , Hughes N , Kapps-Fouthier S , Eder J , Erbel P , Stiefl N , Mackay A , Farady CJ ((2021) ) Crystal structure of NLRP3 NACHT domain with an inhibitor defines mechanism of inflammasome inhibition. J Mol Biol 433: , 167309. |
[36] | Becke AD ((1993) ) Density-functional thermochemistry. III. The role of exact exchange. J Chem Phys 98: , 5648–5652. |
[37] | Jensen F ((2001) ) Polarization consistent basis sets: Principles. J Chem Phys 115: , 9113–9125. |
[38] | Koopmans T ((1934) ) Über die zuordnung von wellenfunktionen und eigenwerten zu den einzelnen elektronen eines atoms. Physica 1: , 104–113. |
[39] | Parr RG , Pearson RG ((1983) ) Absolute hardness: Companion parameter to absolute electronegativity. J Am Chem Soc 105: , 7512–7516. |
[40] | Cheng F , Li W , Zhou Y , Shen J , Wu Z , Liu G , Lee PW , Tang Y ((2012) ) admetSAR: A comprehensive source and free tool for assessment of chemical ADMET properties. J Chem Inf Model 52: , 3099–3105. |
[41] | Daina A , Michielin O , Zoete V ((2017) ) SwissADME: A free web tool to evaluate pharmacokinetics, drug-likeness and medicinal chemistry friendliness of small molecules. Sci Rep 7: , 42717. |
[42] | Coll RC , Schroder K , Pelegrín P ((2022) ) NLRP3 and pyroptosis blockers for treating inflammatory diseases. Trends Pharmacol Sci 43: , 653–668. |
[43] | Alzohairy MA ((2016) ) Therapeutics role of Azadirachta indica (Neem) and their active constituents in diseases prevention and treatment. Evid Based Complement Alternat Med 2016: , 1–11. |
[44] | Balogun TA , Ipinloju N , Abdullateef OT , Moses SI , Omoboyowa DA , James AC , Saibu OA , Akinyemi WF , Oni EA ((2021) ) Computational evaluation of bioactive compounds from Colocasia affinis Schott as a novel EGFR inhibitor for cancer treatment. Cancer Inform 20: , 117693512110492. |
[45] | Santos Nascimento IJD , Aquino de TM , Silva-Júnior da EF (2022) Molecular docking and dynamics simulations studies of a dataset of NLRP3 inflammasome inhibitors. Recent Adv Inflamm Allergy Drug Discov. |
[46] | Tang Z , Roberts CC , Chang CA ((2017) ) Understanding ligand-receptor non-covalent binding kinetics using molecular modeling. Front Biosci Landmark Ed 22: , 960–981. |
[47] | Pan AC , Borhani DW , Dror RO , Shaw DE ((2013) ) Molecular determinants of drug–receptor binding kinetics. Drug Discov Today 18: , 667–673. |
[48] | Talmaciu MM , Bodoki E , Oprean R ((2016) ) Global chemical reactivity parameters for several chiral beta-blockers from the density functional theory viewpoint. Clujul Med 89: , 513–518. |
[49] | Bendjeddou A , Abbaz T , Maache S , Rehamnia R , Gouasmia A , Villemin D ((2016) ) Quantum chemical descriptors of some P-aminophenyl tetrathiafulvalenes through density functional theory (DFT). Rasayan J Chem 9: , 18–26. |
[50] | Babu NS , Onoka I ((2016) ) Computational and chemometrics study of molecular descriptors for butene derivates by density functional theory (DFT). J Chem Pharm Res 8: , 1107–1117. |
[51] | Srivastava V , Yadav A , Sarkar P ((2022) ) Molecular docking and ADMET study of bioactive compounds of Glycyrrhiza glabra against main protease of SARS-CoV2. Mater Today Proc 49: , 2999–3007. |
[52] | Esposito C , Wang S , Lange UEW , Oellien F , Riniker S ((2020) ) Combining machine learning and molecular dynamics to predict P-glycoprotein substrates. J Chem Inf Model 60: , 4730–4749. |
[53] | Nguyen TT , Nguyen TTD , Nguyen TKO , Vo TK , Vo VG ((2021) ) Advances in developing therapeutic strategies for Alzheimer’s disease. Biomed Pharmacother 139: , 111623. |
[54] | Song Y , Li C , Liu G , Liu R , Chen Y , Li W , Cao Z , Zhao B , Lu C , Liu Y ((2021) ) Drug-metabolizing cytochrome P450 enzymes have multifarious influences on treatment outcomes. Clin Pharmacokinet 60: , 585–601. |
[55] | Kazmi SR , Jun R , Yu M-S , Jung C , Na D ((2019) ) In silico approaches and tools for the prediction of drug metabolism and fate: A review. Comput Biol Med 106: , 54–64. |
[56] | Zhang Z , Tang W ((2018) ) Drug metabolism in drug discovery and development. Acta Pharm Sin B 8: , 721–732. |
[57] | Fatunde OA , Brown S-A ((2020) ) The role of CYP450 drug metabolism in precision cardio-oncology. Int J Mol Sci 21: , E604. |
[58] | Jia C-Y , Li J-Y , Hao G-F , Yang G-F ((2020) ) A drug-likeness toolbox facilitates ADMET study in drug discovery. Drug Discov Today 25: , 248–258. |
[59] | Doak BC , Over B , Giordanetto F , Kihlberg J ((2014) ) Oral druggable space beyond the rule of 5: Insights from drugs and clinical candidates. Chem Biol 21: , 1115–1142. |