Impact of Sociodemographic Features and Lifestyle on Cognitive Performance of Peruvian Adults
Abstract
Background:
Cognitive impairment and dementia may result from a combination of modifiable and nonmodifiable risk and protective factors, such as the environment, educational attainment, time devoted to cognitively stimulating activities, and physical activity.
Objective:
This study aimed to investigate the mediating role of sociodemographic characteristics and lifestyle factors in the years of education and cognitive performance in Peruvian adults.
Methods:
This cross-sectional study included 1,478 subjects assessed by Addenbrooke’s Cognitive Examination Revised (ACE-R). Using mediation models, we evaluated the mediation role of parents’ educational level, reading time (RT), and physical activity time (PAT) in the years of education (IYE) and cognitive performance.
Results:
People who reported having lived in an urban area during their childhood are estimated to have, on average, 2.085 years more formal education than those who lived in rural areas. In addition, 49% of cognitive performance scores are explained by the mediation effect of reading and physical activity time in the IYE. This implies that higher levels of education, mediated by RT and PAT per week, are 1.596 units associated with higher scores on the ACE-R.
Conclusion:
Despite the fact that nonmodifiable factors (i.e., childhood residence area, parents’ educational level) seem to exert an effect on older adults’ cognition, their influence is mediated by other factors that are indeed modifiable (i.e., reading time, physical activity engagement). In this sense, lifestyle changes could help prevent or decrease the risk of cognitive impairment and reduce the disease’s impact on vulnerable environments in Latin American and Caribbean countries.
INTRODUCTION
In recent years, differences in dementia rates across diverse populations have drawn the attention of scientists around the globe [1–3]. Cognitive impairment results from a combination of modifiable and nonmodifiable risk and protective factors. Some of the most widely studied protective modifiable factors include educational attainment, time devoted to cognitively stimulating activities (e.g., reading), and physical activity [4–7]. In fact, a recent publication shows that up to 56% of the dementia burden in Latin American and Caribbean countries (LACs) could be attributable to a wide range of modifiable risk factors [8]. On the other hand, it has been well established that higher socioeconomic status is associated with a healthier lifestyle [9] through a combination of increased purchasing power and understanding of the implications of unhealthy behaviors. In this context, the study of the impact of socioeconomic inequalities and other social determinants of health on brain health and cognitive function across the lifespan has drawn increasing attention [10, 11]. This approach takes particular importance in LAC, characterized by poor access to health care, lower education rates, heterogeneity, diversity, unstable economy, and political instability [12], which affect the establishment and implementation of prevention and promotion policies.
The elevated level of inequity in LAC countries might contribute to the diverse estimations of dementia prevalence across countries. Along this line, it is crucial to consider additional risk factors associated with cognitive decline that are particularly common within these regions. For example, access to education in LAC is still concentrated in the wealthier social strata of societies, and the most impoverished strata have fewer opportunities to complete secondary or superior education [13]. Access to education influences cognitive abilities, especially in early life, and protects against the development of dementia [14, 15]. This factor represents the 7.1% risk factor prevalence in early life to develop dementia [8].
Even if recent economic analyses reflect a better current situation for certain LAC countries, moderate poverty increased from 21% to 27%, and extreme poverty rose from 7% to 11% by 2015 [16], affecting primarily rural and suburban areas in the access to education or the possibility to educate the children owing to the lack of financial resources. In this sense, estimating the prevalence of risk factors should consider the lifetime of elderly subjects in LAC.
Beyond sociodemographic factor data, other risk factors, such as diabetes [17, 18], obesity [19], physical inactivity [20, 21], and hypertension [22], could contribute to increasing the risk of cognitive decline and dementia [23], suggesting that lifestyle changes could be critical. Poor access to health care, treatment, cultural influences on nutrition, and physical activity aggravates the picture, and all these factors influence the development and increase of dementia in LAC. The lifestyle changes could be critical to reducing this situation, although the evidence remains mixed and inconclusive about the amount of dementia that can be prevented with lifestyle changes [24].
In the specific case of Peru, inequality has been associated with several factors, including migration, poverty, and centralism (i.e., centralization of health and economic resources in large cities such as Lima, with the concurrent decrease in health or educational services in the periphery of the city and rural areas) [25, 26]. These factors impact the social determinants of health across the territory and act in combination with a lack of effective public health policies and programs [25, 27]. In this sense, the absence of specific policies, the difficulty of health services delivery to specific targets, such as the elderly population, and the deficient design of health care programs [28] are likely to negatively impact the brain health of the Peruvian population. Therefore, assessing these features could be the first step in the prevention of cognitive decline and its associated reduction in the quality of life of Peruvian older adults.
In Peru, living in a rural or urban environment could determine access to a healthier lifestyle, negatively affecting cognitive performance during aging. Some studies suggest large inequalities between rural and urban areas in low-income countries in contextual, cognitive, nutritional, and academic opportunities [29–31]. Some reports suggest that socioeconomic inequalities are related to dementia risk differences and modifiable health conditions and lifestyle factors [10]. Nonetheless, the specific social determinants that influence cognitive performance are not well understood, especially in LAC countries. Considering all of the evidence presented above, the present study aimed to investigate sociodemographic characteristics and lifestyle factors specifically influencing cognitive function in Peruvian older adults, applying mediation modeling to assess their contribution.
MATERIALS AND METHODS
Participants
The baseline sample consisted of 1,689 subjects of both sexes who were recruited from 14 public health centers in Arequipa, Perú, as part of the following Survey in Health Services of Primary Attention. Participants were volunteering county dwellers, members of the aging center, who could participate in approximately 60 min of neuropsychological evaluation under appropriate conditions. The aging center is a governmental program associated with public institutions to promote the physical, mental, and social well-being of older adults across the country. General inclusion criteria were as follows: age between 50 and 90 years, a short form Geriatric Depression Scale score ≤5, capable of speaking in Spanish, with no verbal comprehension or expression problems, and no history of psychiatric or neurological disorders. The institutional review boards of aging centers approved the study protocol, and the procedure was performed according to the guidelines of the Helsinki Declaration. The participants gave written informed consent to participate in the study.
For the present analysis, we excluded subjects with a Geriatric Depression Scale score >5 because of the relationship between depression and worse cognitive performance [32] (n = 120), those living in institutions (n = 30), and those with missing cognitive test scores (n = 61).
The sample for this study, therefore, included 1,478 participants (64.7% female). The results of the descriptive analysis of participants’ performance are displayed in Table 1.
Table 1
Sociodemographic characteristics of the sample
All | Rural | Urban | p corrected | ||
(n = 1478) | (n = 800) | (n = 678) | |||
Age (y) | 67.30 ± 9.98 | 67.88 ± 9.32 | 66.49 ± 10.66 | 0.001a | |
Sex (%) | Female | 64.7 | 65.4 | 63.9 | 0.59b |
Male | 35.3 | 34.6 | 36.1 | ||
Individual’s educational level (y) | 6.69 ± 5.06 | 5.11 ± 4.47 | 8.55 ± 5.08 | <0.001a | |
Parent’s educational level (%) | Illiterate | 40.6 | 50.4 | 29.0 | < 0.001b |
Fewer than 7 y | 37.6 | 35.8 | 39.9 | ||
Between 7 and 11 y | 17.4 | 11.8 | 24.0 | ||
More than 12 y | 4.3 | 2.0 | 7.1 | ||
Medical history (%) | Yes | 49.4 | 53.6 | 44.4 | <0.001b |
No | 50.6 | 46.4 | 55.6 | ||
Reading time per week (h) | 1.95 ± 3.96 | 1.40 ± 3.10 | 2.59 ± 4.70 | <0.001a | |
Physical activity time per week (h) | 0.67 ± 1.71 | 0.55 ± 1.39 | 0.80 ± 2.03 | 0.102a | |
Current smoking status (%) | Do not consume | 93.9 | 95.3 | 92.3 | 0.069b |
Very rarely | 0 | 0 | 0 | ||
Rarely | 5 | 4 | 6.2 | ||
Occasionally | 1.1 | 0.8 | 1.5 | ||
Frequently | 0 | 0 | 0 | ||
Alcohol consumption (%) | Do not consume | 0 | 0 | 0 | 0.069b |
Very rarely | 81 | 82.8 | 78.9 | ||
Rarely | 15 | 12.9 | 17.6 | ||
Occasionally | 3.7 | 4 | 3.4 | ||
Frequently | 0.3 | 0.4 | 0.1 | ||
ACE-R score | 63.77 ± 19.09 | 59.66 ± 18.61 | 68.63 ± 18.53 | <0.001a |
Table 1 provides the mean values ± standard deviations (in the case of continuous variables) or percentages (in the case of categorical and ordinal variables) for relevant sample characteristics. The results are provided for the whole sample and stratified by childhood residence area (rural or urban). Group comparisons were performed for all variables of interest (rural versus urban), where (a) the Mann-Whitney test was applied in the case of continuous variables and (b) the chi-squared test was calculated for categorical and ordinal variables. p values were corrected using the false discovery rate (FDR) to account for multiple testing. Significant p values are shown and marked in bold.
Measures
Sociodemographic features, lifestyle factors, and clinical history were analyzed through a detailed survey administered via a 20-min face-to-face interview in which data about demographics, lifestyle, and comorbidities were collected. This survey was composed of questions about sociocultural variables such as gender, age, education, socioeconomic status, and lifestyle factors. These measures were categorized as binary, tertial, or quartile variables based on their frequency distributions. Education was reported by the number of Years of Education (YE). Marital status was classified as single, cohabitant, married, widowed, or divorced. The parent’s educational level was treated as a dichotomous variable (yes-no) and then displayed a list divided into no schooling, between one and six YE, between seven and 11 YE, and more than 11 YE. Medical history was answered with yes (one or more chronic medical conditions) or no (absence of chronic medical condition), and then multiple options were provided to define the disease, including cardiovascular, metabolic endocrine, psychiatric, oncological disease, and others. The childhood residence area (CRA) was evaluated with an open question about where the participants lived before they were six years old. Then, we classified the location between urban and rural areas.
Regarding lifestyle factors, reading time was evaluated considering the number of hours per week in the last month. The information about physical activity per week was determined by the reported frequency of hours per week of physical activity, including walking at a moderate pace, dancing, floor or stretching exercises, jogging, gym, and other relative activities. Current and ex-smokers were identified using two questions: “Do you smoke?” and “How often do you consume?” Then, self-reported information on the frequency of smoke consumption was used to classify participants into four groups according to the Likert scale: not consume (never consume), very rarely (once a month or less), rarely (2-3 times a month), occasionally (1-2 times per week), and frequently (1-2 times a day). The same questions were used with alcohol and drug consumption.
We included these factors (educational level, childhood residence area, individual years of education, parents’ education level, reading time per week, physical activity time per week) based on previous reports that explain how they impact cognition [14, 15, 33, 34].
To evaluate cognitive performance, participants were screened using Addenbrooke’s Cognitive Examination - Revised (ACE-R). This test was applied to explore their cognitive functioning in different domains, such as verbal memory, attention and executive functions, language, and praxis. The ACE-R is a screening test that assesses six cognitive areas (orientation, attention, memory, language, verbal fluency, and visual construction functions). The version of the test used here is a linguistic cross-cultural adaptation of Torralva et al. [35]. The maximum score is 100 points, and the most commonly used cutoff point is 88, with lower scores indicating the presence of cognitive impairment. The internal consistency analysis for the ACE-R in this study showed a Cronbach’s alpha coefficient of 0.904 (elements = 24), which is considered acceptable.
Statistical analysis
To ensure the quality and reliability of the data, we conducted several preliminary analyses prior to the formal analyses. First, we use descriptive statistics to assess the frequencies, percentages, central tendency, and dispersion measures. Nonparametric contrast tests (χ2, U Mann-Whitney) were used considering the distribution of the data (checked using Kolmogorov-Smirnov test) and homogeneity of variances (Levene test). Second, Spearman’s correlation test examined how cognitive performance was associated with age, educational level, reading time, and physical activity time. Then, extension of simple linear regression was used to investigate the overall associations between each factor and cognitive function, adjusting for the covariables (age, sex, and chronic conditions).
Mediation analysis investigated the mechanisms underlying observed relationships between sociodemographic factors, lifestyle, and cognitive performance and examined additional hypothesized variables on the causal pathway. Therefore, the final model was constructed considering two steps: Step 1. To evaluate the mediation role of parents’ educational level (PEL) between the individual’s years of education (IYE) and childhood residence area (rural versus urban; CRA), we used a simple mediation analysis, where CRA is the independent variable and IYE the dependent variable. The model construction is presented in Table 3. Step 2: Regarding the lifestyle factors, we evaluated the influence of reading time per week, physical activity time per week, current smoking status, and alcohol consumption as mediators between educational level and cognitive performance (see Table 4). The mediation models in both cases were constructed as a function of the variance explained in every step for the independent variable (i.e., in step 1: individual level of education, and in step 2: cognitive performance), as illustrated in Fig. 1, exploring covariables such as age, sex, and chronic illness and the overall indirect effect of cognitive scores using Process Macro. Process Macro is a bootstrapping statistical computed tool written by Andrew Hayes as an extension for SPSS and computes X’s direct, indirect, and total effects on Y and unstandardized and standardized regression coefficients, standard errors, t, p values, and R2. Adjusted R-squared indicated the proportion of variance explained by the independent variables [36]. Additionally, previous regressions and a final mediation model were run to confirm predictors with 95% bias-corrected bootstrapped confidence intervals with 10000 repetitions (to correct for nonnormally distributed data). The SPSS V. 22.0 statistical package was used for this analysis.
Table 3
Model Construction: Educational level and childhood residence area
Model 1 | Model 2 | Model 3 | ||||
Antecedent | Coeff. | p | Coeff. | p | Coeff. | p |
X_CRA | 2.13 | <0.001 | 2.08 | <0.001 | 2.08 | <0.001 |
M1_PEL | 2.97 | <0.001 | 2.50 | <0.001 | 2.50 | <0.001 |
C1 (age) | – | – | –0.1518 | <0.001 | –0.15 | <0.001 |
C2 (sex) | 1.7666 | <0.001 | 1.76 | <0.001 | ||
C3 (chronic illness) | –0.0552 | 0.78 | ||||
Constant | 3.16 | <0.001 | 13.26 | 0.00 | 13.15 | <0.001 |
R2 | 0.352 | <0.001 | 0.459 | 0.000 | 0.459 | <0.001 |
Table 3 provides coefficients (Coeff.) and p values for the individual’s educational level assessed during the model construction process, including variables such as childhood residence area (X_CRA), parents’ education level (M1_PEL), and covariables such as age, sex, and chronic illness. The final model was constructed considering the influence of the covariables on the result. Model 1: unadjusted; Model 2: adjusted for age, sex, and chronic illness, F (5,1472) = 249.7334. Model 3: Adjusted for age and sex, F (4, 1473) = 312.3429. The use of chronic illness as a covariable did not show any significant result in the final model.
Table 4
Model Construction: Lifestyle factors, educational level and cognitive performance
Model 1 | Model 2 | Model 3 | Model 4 | |||||
Antecedent | Coeff. | p | Coeff. | p | Coeff. | p | Coeff. | p |
X_IEL | 2.06 | <0.001 | 1.60 | <0.001 | 1.6 | <0.001 | 1.62 | <0.001 |
M1_RT | 0.73 | <0.001 | 0.79 | <0.001 | 0.8 | <0.001 | 0.8 | <0.001 |
M2_PAT | 0.48 | 0.04 | 0.52 | 0.02 | 0.53 | 0.02 | 0.54 | 0.02 |
M3_CSS | –1.36 | 0.31 | –0.47 | 0.71 | – | – | – | – |
M4_AC | 2.42 | <0.001 | 0.69 | 0.35 | – | – | – | – |
C1 (Age) | – | – | –0.58 | <0.001 | –0.58 | <0.001 | –0.57 | <0.001 |
C2 (Sex) | – | – | 0.86 | 0.28 | 0.98 | 0.20 | – | – |
C3 (Chronic illness) | – | – | –0.28 | 0.70 | – | – | – | – |
Constant | 47.76 | <0.001 | 89.97 | <0.001 | 89.93 | <0.001 | 89.7 | <0.001 |
R2 | 0.4163 | <0.001 | 0.4889 | <0.001 | 0.4885 | <0.001 | 0.4879 | <0.001 |
Table 4 provides the coefficient (Coeff.) and p values for cognitive performance tested during the model construction process, including variables such as individual educational level (in years; X_ IEL), reading time per week (M1_RT), physical activity time per week (M2_PAT), current smoking status (M3_CSS), and alcohol consumption (M4_AC), and covariables such as age, sex, and chronic illness. Model 1: unadjusted including all variables, F (5,1472) = 209.9829, Model 2: Includes all variables, adjusted for age, sex, and chronic illness, F (8,1469) = 175.6224; Model 3: Including IEL, RT and ST, adjusted for age and sex, F (5,1472) = 281.1679; and Model 4: Including IEL, RT and ST, adjusted for age, F (4,1473) = 350.9081. The use of chronic illness as a covariable did not show any significant result in the final model.
Fig. 1
Mediation analyses. Represents the two final models combined, where there is a significant effect from childhood residence area on the individual’s educational level, which is mediated by the parent’s educational level. Moreover, there is a significant effect of the individual’s educational level on the individual’s cognitive performance, which is mediated by time spent both reading and practicing physical activity. Finally, reading time also predicts physical activity time. In both models, age, sex, and chronic illness were used as covariates. Coefficient values (coeff.) are included in the figure, and significant relationships are marked with an asterisk (*).
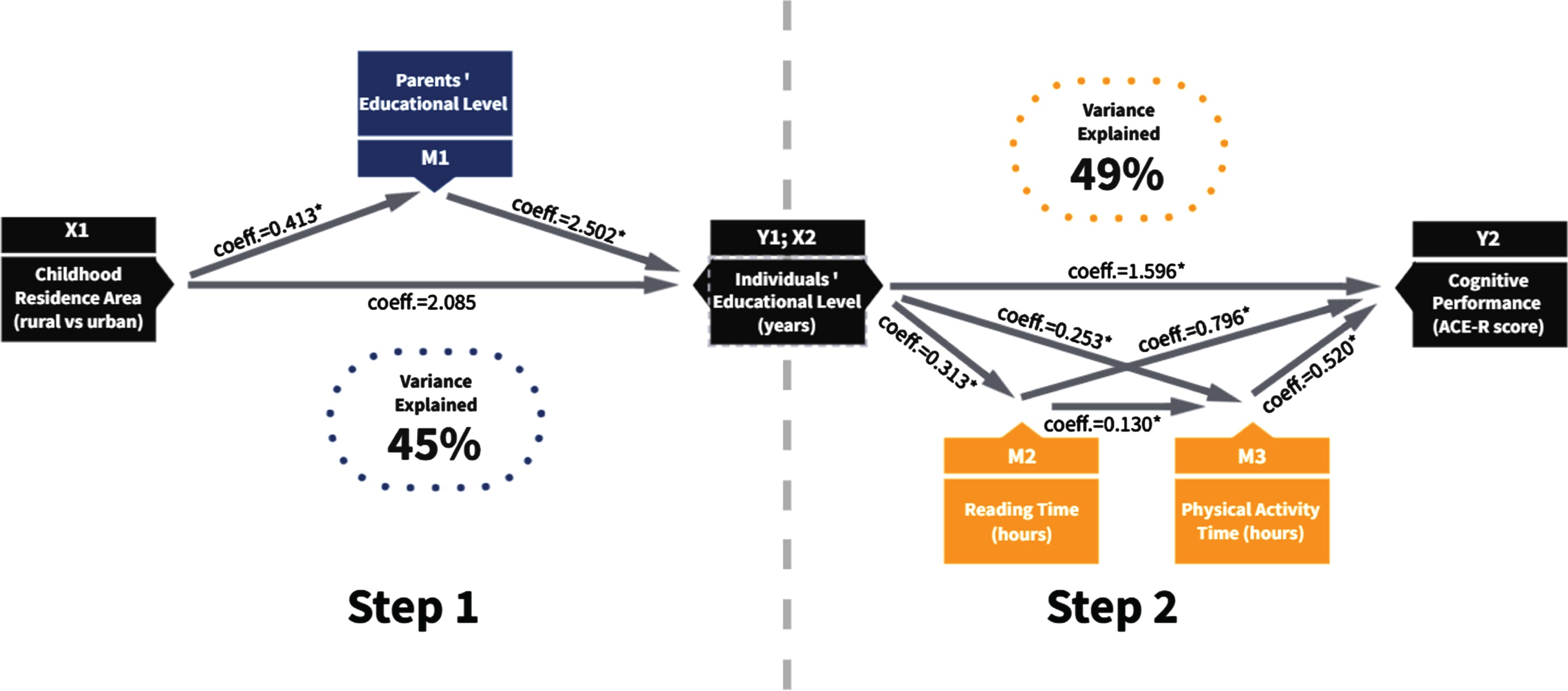
RESULTS
Among the sample, the mean age was 67.30 years old (±9.98), and 64.7% of the participants were females. The largest group (43.1%) had between one and six years of education; no schooling represented 15.7% of the sample. Approximately 50% of the participants’ parents (fathers and mothers) had attained fewer than seven years of formal education (43.2% and 52.7%, respectively). Regarding lifestyle factors, the mean Reading Time (RT) per week was 1.95 h (±3.96), and the Physical Activity Time (PAT) per week was 0.67 h (±1.71). Finally, the mean ACE-R score was 63.77 (±19.09), and we found significant differences in this score between rural and urban areas (59.66 ± 18.61; 68.63 ± 18.53). See Table 1.
The association between sociodemographic variables and lifestyle factors is reported in Table 2; we can see that all variables are related to cognitive performance. Older age is associated with lower scores on the ACE-R (Coeff. –0.506; p < 0.01). Fewer years of education are related to lower performance in ACE-R (Coeff. 0.641; p < 0.01), reading time (Coeff. 0.458; p < 0.01), and physical activity (Coeff. 0.191; p < 0.01); also, physical activity per week (Coeff. 0.191; p < 0.01) is also associated with cognitive performance (Coeff. 0.213; p < 0.01).
Table 2
Associations between sociodemographic characteristics and lifestyle factors
1 | 2 | 3 | 4 | 5 | 6 | |
1. Age | 1 | |||||
2. IYE | –0.402 ** | 1 | ||||
3. PEL | –0.224 ** | 0.541 ** | 1 | |||
4. RT | –0.157 ** | 0.458 ** | 0.277 ** | 1 | ||
5. PAT | –0.046 | 0.191 ** | 0.126 ** | 0.358 ** | 1 | |
6. ACE-R | –0.506 ** | 0.641 ** | 0.366 ** | 0.504 ** | 0.213 ** | 1 |
Spearman’s correlation test was used to define the associations between age, individual years of education (IYE), parents’ education level (PEL), reading time per week (RT), physical activity time per week (PAT), and ACE-R scores. **p < 0.001.
The final model was constructed in two steps. Step 1: To evaluate the mediation role of parents’ educational level (PEL) between years of education (IEL) and childhood residence area (rural versus urban; CRA), we used a simple mediation analysis, where CRA is the independent variable and ILE the dependent variable. The unadjusted model explained 35% of the variance; including age and sex as covariables increased that percentage up to 45% of variance explained in the full model, as shown in Fig. 1 Step 1. Chronic illness did not report meaningful results related to IELs.
In this sense, people who reported having lived in an urban area during their childhood are estimated to have more than 2.085 years of formal education, on average, compared to those who lived in rural areas. The direct effect is c = 3.117 (CI: 2.681–3.554), and the indirect effect ab = 1.032 (CI: 0.799–1.273); two effects are significant. The direct effect is c = 3.117 (CI: 2.681–3.554), and the indirect effect ab = 1.032 (CI: 0.799–1.273); two effects are significant.
Regarding lifestyle factors, we evaluated the impact of mediators such as reading time per week, physical activity time per week, current smoking status, and alcohol consumption on the cognitive performance of the participants. Table 4 provides the coefficient (coeff.) and p values for the cognitive performance (dependent variable) of four models considering covariables such as age, sex, and chronic illness. We identified an effect of chronic illness on physical activity time per week (Coeff. –0.357; p < 0.01); we did not find any significant association with cognitive performance (Coeff. –0.257; p = 0.73). The final model includes RT and ST as mediators between years of education (independent variable) and cognitive performance (dependent variable). This model explains 49% of the variance and considers covariables such as age, sex, and chronic illness (Fig. 1 Step 2). This means that higher levels of education, mediated by RT and ST per week, are 1.596 units associated with higher scores on the ACE-R. The direct effect (c = 1.596; CI: 1.430–1.763) and the indirect effect (ab = 0.02; CI: 0.005–0.037) are significant.
DISCUSSION
The evidence regarding which factors contribute to the promotion of healthy aging and prevent cognitive decline at an advanced age remains mixed and inconclusive, especially when we refer to the lifestyle changes that can successfully prevent dementia through aging. Among the list of potentially modifiable lifestyle factors associated with reduced risk levels, we find years of education, cognitive activity, and physical activity [20, 21]. According to the Lancet Commission Report, modifiable risk factors, including low education and physical inactivity among several others, could account for as much as 56% of the dementia burden in LAC [8]. Nevertheless, most of the studies looking at the protective effect of lifestyle factors are based in certain world regions (e.g., Europe and North America). As a result, the effect of these variables on late-life cognition in diverse populations remains to be elucidated. Given that both genetic makeup and engagement in diverse risk and protective activities vary across diverse populations [9, 37–39], it is crucial to understand to what extent the results found in Western societies can be replicated in other regions. Moreover, the risk of pathological aging results from the complex interaction between genetics and environmental exposures experienced throughout life [40, 41], and thus focusing on a single aspect of lifestyle may be insufficient to reduce the risk of cognitive decline. Therefore, the present study is focused on selected risk and protective factors of cognitive decline grouped under two categories: sociodemographic and lifestyle factors. Our objective was to investigate the potential mediating effect of these factors on the cognitive function of Peruvian older adults who had been raised in either rural or urban environments.
The impact of sociodemographic factors has been studied extensively in the literature. The place where people live defines many aspects of their health and well-being, especially during the early years’ development. These conditions, influenced also by economic, social, political, and environmental forces, are called social determinants [27, 42] and impact the populations across the LAC [12, 43, 44], where the inequities are visible even within the same country. The residence area during childhood is strongly associated with intrauterine growth and development, mediated by nutrition, living conditions, access to education, and early stimulation at home [29, 42]. However, these adverse conditions are potentially modified by parenting support, education, and the inclusion of lifestyle protective factors [10, 42].
Our results confirm the effect of sociodemographic factors on years of formal education, which in later life predicts cognitive performance. Importantly, our study also provides a deeper analysis of the complex relationships that emerge among these key variables. First, nonmodifiable sociodemographic factors (i.e., childhood residence area) predict the participant’s education level, an effect mediated by the parent’s formal education level. In this sense, people who reported having lived in a rural area during their childhood and who had less educated parents are estimated to have on average 2 points lower formal education than those who lived in urban areas. Second, the effect of years of formal education on late-life cognitive performance appears to be mediated by modifiable factors (i.e., reading time per week and physical activity time per week), explaining 49% of the variance in ACE-R scores. These protective factors could exert their effects by means of increasing cognitive reserve, which has been described as the ability of the brain to preserve its normal functioning despite the presence of underlying structural damage [6, 42], and represent behaviors related to cognitive enrichment, which may stimulate neurotrophic factors in the brain [45]. Nevertheless, we should bear in mind that the individual’s educational level might have also affected the participant’s ability to comprehend and navigate the cognitive test, which could have influenced our overall results [46].
Interestingly, even though there has been straightforward evidence that alcohol consumption [47, 48] and smoking [2, 49, 50] are independent risk factors for dementia, these two factors were not significantly associated with cognitive performance in our study. The probable reason is the low consumption levels in the sample, whose percentage is lower than that reported in 2016 on consumption in the last 30 days (17.4% versus 20.7%) of older adults consuming alcohol. Something to consider here is the sociocultural validation and the roots of alcoholic beverages in the Andean region and rural areas. It is possible that its consumption is not considered a health problem and is underestimated [48].
Finally, projections suggest that by 2050, the prevalence of dementia in Latin America would quadruple [3], while in Europe and the US, it is expected to double [51, 52]. Furthermore, the economic burden attributable to dementia in low- and middle-income countries has also been estimated to increase between 47–71% over the next 15 years [12, 44, 53, 54]. In this context, our results might aid in the design of science-based strategies to partially prevent or alleviate the impact of such burdensome predictions. Nevertheless, better estimations are needed considering the limited reliable epidemiologic data, socioeconomic and sociocultural differences, reduced access to mental health facilities, and the absence of specific policies and protocols for the early diagnosis and treatment of dementia [12].
This study is not exempt from limitations. For example, although participants underwent extensive cognitive screening, the present study lacks the utilization of proper diagnostic tools that would have enabled us to identify cases of mild cognitive impairment or dementia. Along this line, lifestyle characterization relied solely on self-reported measurements. Moreover, participants were recruited from urban settings, and information regarding childhood residence areas after the age of 6 was not available. This means that the exact timing when rural children moved to the city was not collected, which could have been used in secondary analyses. Furthermore, future studies could aim to include the analysis of social determinants at different ages as well as their influence on cognitive performance at different life stages (i.e., before the age of 10 and between 35 and 45 years old). Nevertheless, the utilization of mediation analyses contributed to deepen our understanding of the relationships among the variables included in the model. Beyond the statistical approach, this study presents several strengths, for example, the ample sample size and the thorough characterization of an understudied population in the wider literature.
Finally, our results suggest that there is room for the implementation of evidence-based public policies that could restrict the detrimental effects of nonmodifiable factors, reducing cognitive decline and dementia risk in the Peruvian population. Along this line, the political support for the elderly and dementia prevention in Peru is visible in two laws focusing on the improvement of the quality of life of the elderly (Law N° 30490) and on the prevention and treatment of Alzheimer’s disease and other dementias (Law N° 30795). Despite these initiatives, their full implementation is far from complete, especially considering the absence of specific mental health facilities or governmental budgets for dementia [55].
Conclusions
Altogether, this study fosters our understanding of the interaction between modifiable and nonmodifiable sociodemographic and lifestyle factors on cognitive performance in late life. Here, we show that although nonmodifiable factors (i.e., childhood residence area, parents’ educational level) have an impact on older adults’ cognition, their influence is mediated by other factors that are indeed modifiable (i.e., reading time, physical activity engagement).
For instance, there is no effective pharmacological treatment currently available to prevent or cure dementia and cognitive decline. It is appropriate that more initiatives encouraging a healthy lifestyle and promoting brain health activities (for example, through exercise, diet, brain enrichment activities, social interaction) and other factors be promoted by the government and other organizations. In addition, more studies are needed to identify lifestyle protective factors that reverse the influence of nonmodifiable factors that impact cognition.
ACKNOWLEDGMENTS
Dr. Chino acknowledges the financial support of the National Council of Science, Technology and Technological Innovation (CONCYTEC, Perú) through the National Fund for Scientific and Technological Development (FONDECYT, Perú). Additionally, Dr. Zegarra-Valdivia acknowledges the support of the Global Brain Health Institute (GBHI) at the University of California, San Francisco, and Universidad Señor de Sipán, Chiclayo - Perú.
Authors’ disclosures available online (https://www.j-alz.com/manuscript-disclosures/22-0428r3).
SUPPLEMENTARY MATERIAL
[1] The supplementary material is available in the electronic version of this article: https://dx.doi.org/10.3233/JAD-220428.
REFERENCES
[1] | Prince M , Bryce R , Albanese E , Wimo A , Ribeiro W , Ferri CP ((2013) ) The global prevalence of dementia: A systematic review and metaanalysis. Alzheimers Dement 9: , 63–75. |
[2] | GBD 2016 Dementia Collaborators ((2016) ) Global, regional, and national burden of Alzheimer’s disease and other dementias, 1990-2016: A systematic analysis for the Global Burden of Disease Study 2016. Lancet Neurol 18: , 88–106. |
[3] | Custodio N , Wheelock A , Thumala D , Slachevsky A ((2017) ) Dementia in Latin America: Epidemiological evidence and implications for public policy. Front Aging Neurosci 9: , 221. |
[4] | Clare L , Wu Y-T , Teale JC , MacLeaod C , Matthews F , Brayne C , Woods B , CFAS –Wales study team ((2017) ) Potentially modifiable lifestyle factors, cognitive reserve, and cognitive function in later life: A cross-sectional study. PLoS Med 14: , e1002259. |
[5] | Foubert-Samier A , Catheline G , Amieva H , Dilharreguy B , Helmer C , Allard M , Dartigues JF ((2012) ) Education, occupation, leisure activities, and brain reserve: A population-based study. Neurobiol Aging 33: , 423.e15–25. |
[6] | Stern Y ((2009) ) Cognitive reserve. Neuropsychologia 47: , 2015–2028. |
[7] | Stern Y , MacKay-Brandt A , Lee S , McKinley P , McIntyre K , Razlighi Q , Agarunov E , Bartels M , Sloan RP ((2019) ) Effect of aerobic exercise on cognition in younger adults: A randomized clinical trial. Neurology 92: , e905–e916. |
[8] | Livingston G , Huntley J , Sommerlad A , Ames D , Ballard C , Banerjee S , Brayne C , Burns A , Cohen-Mansfield J , Cooper C , Costafreda SG , Dias A , Fox N , Gitlin LN , Howard R , Kales HC , Kivimäki M , Larson EB , Ogunniyi A , Orgeta V , Ritchie K , Rockwood K , Sampson EL , Samus Q , Schneider LS , Selbæk G , Teri L , Mukadam N ((2020) ) Dementiaprevention, intervention, and care: 2020 report of the LancetCommission. Lancet 396: , 413–446. |
[9] | World Health Organization (2018) Global action plan on physical activity 2018–2030: More active people for a healthier world. https://apps.who.int/iris/handle/10665/272722, Accessed on April 1, 2022. |
[10] | Deckers K , Cadar D , van Boxtel MPJ , Verhey FRJ , Steptoe A , Köhler S ((2019) ) Modifiable risk factors explain socioeconomic inequalities in dementia risk: Evidence from a population-based prospective cohort study. J Alzheimers Dis 71: , 549–557. |
[11] | Yaffe K , Falvey C , Harris TB , Newman A , Satterfield S , Koster A , Ayonayon H , Simonsick E Health ABC Study; ((2013) ) Effect of socioeconomic disparities on incidence of dementia among biracial older adults: Prospective study. BMJ 347: , f7051. |
[12] | Parra MA , Baez S , Sedeño L , Gonzalez Campo C , Santamaría-García H , Aprahamian I , Bertolucci PH , Bustin J , Camargos Bicalho MA , Cano-Gutierrez C , Caramelli P , Chaves MLF , Cogram P , Beber BC , Court FA , de Souza LC , Custodio N , Damian A , dela Cruz M , Diehl Rodriguez R , Brucki SMD , Fajersztajn L , Farías GA , De Felice FG , Ferrari R , de Oliveira FF , Ferreira ST , Ferretti C , Figueredo Balthazar ML , Ferreira Frota NA , Fuentes P , García AM , Garcia PJ , de Gobbi Porto FH , Duque Peñailillo L , Engler HW , Maier I , Mata IF , Gonzalez-Billault C , Lopez OL , Morelli L , Nitrini R , Quiroz YT , Guerrero Barragan A , Huepe D , Pio FJ , Suemoto CK , Kochhann R , Kochen S , Kumfor F , Lanata S , Miller B , Mansur LL , Hosogi ML , Lillo P , Llibre Guerra J , Lira D , Lopera F , Comas A , Avila-Funes JA , Sosa AL , Ramos C , Resende EPF , Snyder HM , Tarnanas I , Yokoyama J , Llibre J , Cardona JF , Possin K , Kosik KS , Montesinos R , Moguilner S , Solis PCL , Ferretti-Rebustini REL , Ramirez JM , Matallana D , Mbakile-Mahlanza L , Marques Ton AM , Tavares RM , Miotto EC , Muniz-Terrera G , Muñoz-Nevárez LA , Orozco D , Okada deOliveira M , Piguet O , Pintado Caipa M , Piña Escudero SD , Schilling LP , Rodrigues Palmeira AL , Yassuda MS , Santacruz-Escudero JM , Serafim RB , Smid J , Slachevsky A , Serrano C , Soto-Añari M , Takada LT , Grinberg LT , Teixeira AL , Barbosa MT , Trépel D , Ibanez A ((2021) ) Dementia in Latin America: Paving the way toward aregional action plan. Alzheimers Dement 17: , 295–313. |
[13] | UNESCO-IESALC (2020) Towards universal access to higher education: International trends, https://unesdoc.unesco.org/ark:/48223/pf0000375686/PDF/375686spa.pdf.multi, Last updated March 15, 2022, Accessed on May 21, 2022. |
[14] | Chen Y , Lv C , Li X , Zhang J , Chen K , Liu Z , Li H , Fan J , Qin T , Luo L , Zhang Z ((2019) ) The positive impacts of early-life education on cognition, leisure activity, and brain structure in healthy aging. Aging 11: , 4923–4942. |
[15] | Lövdén M , Fratiglioni L , Glymour MM , Lindenberger U , Tucker-Drob EM ((2020) ) Education and cognitive functioning across thelife span. Psychol Sci Public Interest 21: , 6–41. |
[16] | Helwege A , Birch MBL ((2007) ) Declining poverty in Latin America? A critical analysis of new estimates by international institutions, Global Development and Environment Institute, Tufts University, Working Paper, 07–02. |
[17] | Athanasaki A , Melanis K , Tsantzali I , Stefanou MI , Ntymenou S , Paraskevas SG , Kalamatianos T , Boutati E , Lambadiari V , Voumvourakis KI , Stranjalis G , Giannopoulos S , Tsivgoulis G , Paraskevas GP ((2022) ) Type 2 diabetes mellitus as a risk factor for Alzheimer’s disease: Review and meta-analysis. Biomedicines 10: , 778. |
[18] | Ferguson SA , Panos JJ , Sloper D , Varma V , Sarkar S ((2021) ) Alzheimer’s disease: A step closer to understanding type 3 diabetes in African Americans. Metab Brain Dis 36: , 1803–1816. |
[19] | Dye L , Boyle NB , Champ C , Lawton C ((2017) ) The relationship between obesity and cognitive health and decline. Proc Nutr Soc 76: , 443–454. |
[20] | Scarmeas N , Stern Y ((2003) ) Cognitive reserve and lifestyle. J Clin Exp Neuropsychol 25: , 625–633. |
[21] | Song S , Stern Y , Gu Y ((2022) ) Modifiable lifestyle factors and cognitive reserve: A systematic review of current evidence. Ageing Res Rev 74: , 101551. |
[22] | Reitz C , Tang MX , Manly J , Mayeux R , Luchsinger JA ((2007) ) Hypertension and the risk of mild cognitive impairment. Arch Neurol 64: , 1734–1740. |
[23] | Avilés-Santa ML , Monroig-Rivera A , Soto-Soto A , Lindberg NM ((2020) ) Current state of diabetes mellitus prevalence, awareness,treatment, and control in Latin America: Challenges and innovativesolutions to improve health outcomes across the continent. CurrDiab Rep 20: , 62. |
[24] | Montero-Odasso M , Ismail Z , Livingston G ((2020) ) One third of dementia cases can be prevented within the next 25 years by tackling risk factors. The case “for” and “against”. Alzheimers Res Ther 12: , 81. |
[25] | Carrillo-Larco RM , Guzman-Vilca WC , Leon-Velarde F , Bernabe-Ortiz A , Jimenez MM , Penny ME , Gianella C , Leguía M , Tsukayama P , Hartinger SM , Lescano AG , Cuba-Fuentes MS , Cutipé Y , Diez-Canseco F , Mendoza W , Ugarte-Gil C , Valdivia-Gago A , Zavaleta-Cortijo C , Miranda JJ ((2022) ) Peru – Progress inhealth and sciences in 200 years of independence. Lancet RegHealth Am 7: , 100148. |
[26] | Rougeaux E , Miranda JJ , Fewtrell M , Wells JCK ((2022) ) Maternal internal migration and child growth and nutritional health in Peru: An analysis of the demographic and health surveys from 1991 to 2017. BMC Public Health 22: , 37. |
[27] | Allen J , Balfour R , Bell R , Marmot M ((2014) ) Social determinants of mental health. Int Rev Psychiatry 26: , 392–407. |
[28] | Yamada G , Castro JF ((2012) ) Poverty, inequality, and social policies in Peru: As poor as it gets, http://repositorio.up.edu.pe/handle/11354/316 Last updated June 07, 2012, Accessed on May 21, 2022. |
[29] | Fagbamigbe AF , Kandala NB , Uthman AO ((2020) ) Demystifying the factors associated with rural– urban gaps in severe acute malnutrition among under-five children in low- and middle-income countries: A decomposition analysis. Sci Rep 10: , 11172. |
[30] | Tine M ((2016) ) Growing up in rural vs. urban poverty: Contextual, academic, and cognitive differences. In Poverty, Inequality and Policy, StaicuG, ed. IntechOpen, pp. 1–22. |
[31] | Goodburn C ((2014) ) Rural– urban migration and gender disparities in child healthcare in China and India. Dev Change 45: , 631–655. |
[32] | Ganguli M ((2009) ) Depression, cognitive impairment and dementia: Why should clinicians care about the web of causation? Indian J Psychiatry 51: , S29–S34. |
[33] | Mandolesi L , Polverino A , Montuori S , Foti F , Ferraioli G , Sorrentino P , Sorrentino G ((2022) ) Effects of physical exercise on cognitive functioning and wellbeing: Biological and psychological benefits. Front Psychol 9: , 509. |
[34] | Peterson RL , Gilsanz P , George KM , Ackley S , Glymour MM , Mungas DM , Whitmer RA ((2020) ) Differences in association of leisure time activities and cognition in a racially/ethnically diverse cohort of older adults: Findings from the KHANDLE study. Alzheimers Dement 6: , e12047. |
[35] | Torralva T , Roca M , Gleichgerrcht E , Bonifacio A , Raimondi C , Manes F ((2011) ) Validación de la versión en español del Addenbrooke’s Cognitive Examination-Revisado (ACE-R). Neurología 26: , 351–356. |
[36] | Hayes A ((2022) ) Introduction to Mediation, Moderation, and Conditional Process Analysis: A Regression-Based Approach Third edition, The Guilford Press New York. |
[37] | Dhana K , Aggarwal NT , Beck T , Desai P , Liu X , Barnes LL , Evans DA , Rajan KB ((2021) ) Healthy lifestyle and cognitive decline in people living with stroke in the community. Alzheimers Dement 17: , e054337. |
[38] | O’Bryant SE , Johnson L , Balldin V , Edwards M , Barber R , Williams B , Devous M , Cushings B , Knebl J , Hall J ((2013) ) Characterization of Mexican Americans with mild cognitive impairment and Alzheimer’s disease. J Alzheimers Dis 33: , 373–379. |
[39] | Raj T , Chibnik LB , McCabe C , Wong A , Replogle JM , Yu L , Gao S , Unverzagt FW , Stranger B , Murrell J , Barnes L , Hendrie HC , Foroud T , Krichevsky A , Bennett DA , Hall KS , Evans DA , De Jager PL ((2016) ) Genetic architecture of age-related cognitive decline in African Americans. Neurol Genet 3: , e125. |
[40] | Dunn AR , O’Connell KMS , Kaczorowski CC ((2019) ) Gene-by-environment interactions in Alzheimer’s disease and Parkinson’s disease. Neurosci Biobehav Rev 103: , 73–80. |
[41] | Finch CE , Kulminski AM ((2019) ) The Alzheimer’s disease exposome. Alzheimers Dement 15: , 1123–1132. |
[42] | Borenstein A , Mortimer J ((2016) ) Alzheimer’s Disease: Life Course Perspectives on Risk Reduction. Academic Press, Sydney. |
[43] | Nitrini R , Bottino CM , Albala C , Custodio Capuñay NS , Ketzoian C , Llibre Rodriguez JJ , Maestre GE , Ramos-Cerqueira AT , Caramelli P ((2009) ) Prevalence of dementia in Latin America: A collaborative study of population-based cohorts. Int Psychogeriatr 21: , 622–630. |
[44] | Parra MA ((2014) ) Overcoming barriers in cognitive assessment ofAlzheimer’s disease. Dement Neuropsychol 8: , 95–98. |
[45] | Fernandez AM , Santi A , Torres I ((2018) ) Insulin peptides as mediators of the impact of lifestyle in Alzheimer’s disease. Brain Plast 4: , 3–15. |
[46] | de Azeredo Passos VM , Giatti L , Bensenor I , Tiemeier H , Arfan M , Carvalho de Figueiredo R , Chor D , Schmidt MI , Barreto SM ((2015) ) Education plays a greater role than age in cognitive test performance among participants of the Brazilian Longitudinal Study of Adult Health (ELSA-Brasil). BMC Neurol 15: , 191. |
[47] | Sudhinaraset M , Wigglesworth C , Takeuchi DT ((2016) ) Social and cultural contexts of alcohol use. Alcohol Res Curr Rev 38: , 35–45. |
[48] | Baumgart M , Snyder HM , Carrillo MC , Fazio S , Kim H , Johns H ((2015) ) Summary of the evidence on modifiable risk factors for cognitive decline and dementia: A population-based perspective. Alzheimers Dement 11: , 718–726. |
[49] | Rusanen M , Kivipelto M , Quesenberry CP Jr , Zhou J , Whitmer RA ((2011) ) Heavy smoking in midlife and long-term risk of Alzheimer disease and vascular dementia. Arch Intern Med 171: , 333–339. |
[50] | Chen JH , Lin KP , Chen YC ((2009) ) Risk factors for dementia. J Formos Med Assoc 108: , 754–764. |
[51] | Alzheimer Europe, Prevalence of dementia in Europe, https://www.alzheimer-europe.org/dementia/prevalence-dementia-europe Last updated May 20, 2022, Accessed on May 21, 2022. |
[52] | Alzheimer Association (2022) 2022 Alzheimer’s disease facts and figures. https://www.alz.org/media/documents/alzheimers-facts-and-figures.pdf, Last updated March 15, 2022, Accessed on May 21, 2022. |
[53] | Reyes-Marin KE , Zegarra-Valdivia JA , Nuñez A ((2021) ) Seizure susceptibility in Alzheimer’s disease. Med Res Arch 9: , 5. |
[54] | Kenne A , Thebaut C , Achille D , Preux PM , Guerchet M ((2021) ) Costs of dementia in low-and-middle income countries: A systematic review. 7th International Conference on Neurology and Epidemiology (ICNE) Virtual Conference 55: , 59. |
[55] | Custodio N ((2016) ) Vivir con demencia en Perú: ¿El sistema desalud está enfrentando la sobrecarga? Rev Neuropsiquiatr 79: , 1. |