A Comparison of Traditional and Serious Game-Based Digital Markers of Cognition in Older Adults with Mild Cognitive Impairment and Healthy Controls
Abstract
Background:
Electroencephalography (EEG) has been used to assess brain activity while users are playing an immersive serious game.
Objective:
To assess differences in brain activation as measured with a non-intrusive wearable EEG device, differences in game performance and correlations between EEG power, game performance and global cognition, between cognitively impaired and non-impaired older adults, during the administration of a novel self-administered serious game-based test, the Virtual Supermarket Test (VST).
Methods:
43 older adults with subjective cognitive decline (SCD) and 33 older adults with mild cognitive impairment (MCI) were recruited from day centers for cognitive disorders. Global cognition was assessed with the Montreal Cognitive Assessment (MoCA). Brain activity was measured with a non-intrusive wearable EEG device in a resting state condition and while they were administered the VST.
Results:
During resting state condition, the MCI group showed increased alpha, beta, delta, and theta band power compared to the SCD group. During the administration of the VST, the MCI group showed increased beta and theta band power compared to the SCD group. Regarding game performance, alpha, beta, delta, and theta rhythms were positively correlated with average duration, while delta rhythm was positively correlated with mean errors. MoCA correlated with alpha, beta, delta, and theta rhythms and with average game duration and mean game errors indicating that elevated EEG rhythms in MCI may be associated with an overall cognitive decline.
Conclusion:
VST performance can be used as a digital biomarker. Cheap commercially available wearable EEG devices can be used for obtaining brain activity biomarkers.
INTRODUCTION
Dementia is a neurodegenerative disease characterized by cognitive decline, personality changes, behavioral disorders, and problems in everyday functioning. It currently affects about 50 million people globally and there are 10 million new cases per year according to World Health Organization (WHO) estimations [1]. Detection of dementia in its earliest stages is instrumental for providing effective interventions and reducing its economical and societal impact. Mild cognitive impairment (MCI) is a diagnostic entity that describes the state between normal aging and dementia [2]. MCI patients manage to maintain their everyday functionality and retain their autonomy thus not necessitating any caregiving [3–5]. At the same time, they can present with deficits in any cognitive domain including memory, attention, executive function, language, and visuospatial abilities [5]. Lately there has been increased interest in the use of virtual reality (VR) and serious game applications for measuring cognition and detecting cognitive impairment [6–8]. Such applications allow for a high degree of ecological validity while remaining pleasant and well accepted by older adults [9–11]. At the same time, they have exhibited strong potential for differentiating between healthy and impaired older adults [12, 13] and their use as digital biomarkers has also been proposed [14].
Serious games in virtual environments
VR technology enables users to enter a computer-generated immersive environment and interact with it in real-time, thus allowing for a high degree of ecological validity. VR applications can be tailored to suit specific therapeutic or research needs [7, 9]. VR applications have been used successfully for the assessment of various cognitive functions such as episodic memory, executive functions, and navigation [6–8, 15]. VR technology has been proposed as an ecologically valid way of assessing cognitive functioning and detecting possible impairment; however, it has been absent from most commercially available computerized cognitive tests/batteries [7]. The main advantage of VR applications when combined with serious games is that they can collect real-time data from immersed users while those users are simultaneously assessed with neuroimaging techniques such as functional magnetic resonance imaging and electroencephalography (EEG) [16].
Electroencephalography
EEG rhythmic activity is usually described in specific frequency bands: 0.1–3 Hz (delta), 4–7 Hz (theta), 8–12 Hz (alpha), 12–30 Hz (beta), and 30–60 Hz (gamma). Numerous studies over the years have accumulated a wealth of evidence indicating that changes in the amplitude of specific brain oscillations are associated with different mental states and cognitive processes [17].
EEG rhythmic activity also changes as a function of age and of neurological diseases such as Alzheimer’s disease (AD) [18–21]. Studies employing analyses of resting-state EEG, task-related and event-related potentials (ERPs) have focused on MCI which is often considered as a prodromal stage of AD. The aim of these studies was to discriminate MCI patients from healthy older adults and to find possible markers which could predict the conversion of MCI to AD. Results of these studies have often been inconsistent. Resting state EEG rhythms of MCI patients have been found to differ from the EEG rhythms of healthy older adults. Alpha is the most investigated cortical rhythm in AD and MCI. MCI patients with higher alpha amplitude showed greater global cortical atrophy [22]. On the contrary, Babiloni has consistently reported in his studies that, in MCI subjects, resting state alpha sources were lower in amplitude than controls [23–25]. Another study reported increased alpha and beta band power in addition to increased theta power and a negative correlation with Mini-Mental State Examination (MMSE) scores [26]. Similar results in regards to beta and theta bands have been reported by Fauzan and Amral [27]. Studies have also shown an increase in theta power in MCI patients compared to healthy controls [27], which correlated with hippocampal volume loss [28]. Alpha, theta and delta power and coherence have been found to be higher in amplitude in MCI to AD converters compared to stable subjects [29].
Relative to resting state EEG (eyes-open or eyes-closed) which records brain spontaneous activity, task-elicited EEG can provide more information regarding the functional activity of neuronal circuits involved in the specific cognitive task being studied. Additionally, the high temporal resolution of EEG offers a real-time measure of task performance and the association of EEG oscillations with various cognitive processes in patients with cognitive deficits. MCI patients displayed smaller decrease of lower alpha power during a picture memory task relative to controls [30]. Increased gamma band activity has been reported in MCI and AD groups compared to a control group during a cognitive task [31].
In conclusion, MCI patients present different EEG profiles compared to healthy older adults, and it is suggested that these differences may be associated with structural differences (i.e., brain atrophy), cognitive deficits, and an increased risk of conversion to dementia. Compared to other imaging techniques, the combined use of EEG and serious games or simple virtual reality applications is affordable, non-invasive, and allows for higher temporal resolution. Studies employing continuous EEG recording usually compare EEG activity during a VR task to a baseline/resting state before or after the task. There are also studies comparing ERP averages with task performance [32, 33]. For example, one study combined EEG with a Virtual Museum task (VAP-M) and was able to differentiate MCI patients from healthy controls [34].
Study aims and hypotheses
This study is part of a larger study that aims to assess the ability of a new computerized testing paradigm, based on a self-administered serious game-based test, to detect MCI in a sample of older adults with subjective cognitive decline (SCD) [35]. It is tied to a wider effort to assess biomarkers and diagnostic instruments in a sample that is representative of people that present for examination in memory clinics, namely older adults with SCD. Older adults with SCD may have already declined from their own baseline of cognitive performance and on average they score lower than healthy older adults without subjective memory complaints in neuropsychological testing while not reaching the threshold for a diagnosis of MCI [36, 37]. Furthermore, they are at risk of progressing to MCI and dementia, and they also display a higher prevalence of positive biomarkers for amyloidosis and neurodegeneration compared to older adults without SCD [37, 38]. Therefore, it is imperative to assess whether existing and novel instruments can meaningfully differentiate between SCD and MCI. To our knowledge, this is the first study where a wearable EEG device is used during the administration of a serious game application modelled on a virtual representation of a common everyday activity in a sample of older adults with SCD.
The main aim of this study is to examine differences in brain activation between MCI patients and healthy older adults with SCD while they are engaged in the Virtual Supermarket Test (VST) serious game application. It is hypothesized that, compared to healthy older adults with SCD, MCI patients will have higher overall brain activation, as depicted by EEG rhythms, in order to compensate for their decline in cognitive functioning [26, 31, 39–42]. Furthermore, we aim to investigate the relationship between VST performance, EEG rhythm activity, and general cognitive status (as measured by the Montreal Cognitive Assessment, MoCA). It is hypothesized that VST performance metrics will correlate with brain activation while users are engaged in the VST task. Furthermore, it is hypothesized that MoCA scores will correlate with both VST performance and EEG rhythms.
MATERIALS AND METHODS
Virtual reality serious game-based cognitive screening test
The VST application has been developed by the Centre for Research and Technology Hellas/ Information Technologies Institute (CERTH/ITI) in collaboration with the Greek Association of Alzheimer’s Disease and Related Disorders (GAADRD). It is based on a simple virtual reality task with a low degree of immersion, designed according to the state of the art in the field of VR applications for cognitive assessment. It runs on a tablet device with Android® operating system, whereas PC and web-based versions also exist. The application has been described in detail in previous studies [6, 8, 35, 43]. The VST leverages the positive attitude of older adults toward self-administered serious games to increase their willingness to be screened for cognitive decline [44, 45]. It is an ecologically valid test, based on a common everyday activity, which comprises a fully self-administered serious game routine with embedded instructions and training sessions. The user has to navigate through a virtual supermarket using specific waypoints, buy the products indicated in a shopping list, and pay the correct amount at the cashier desk. Figure 1 provides screenshots of the VST virtual environment.
Fig. 1
The Virtual Supermarket Test (VST) digital environment (tablet view).
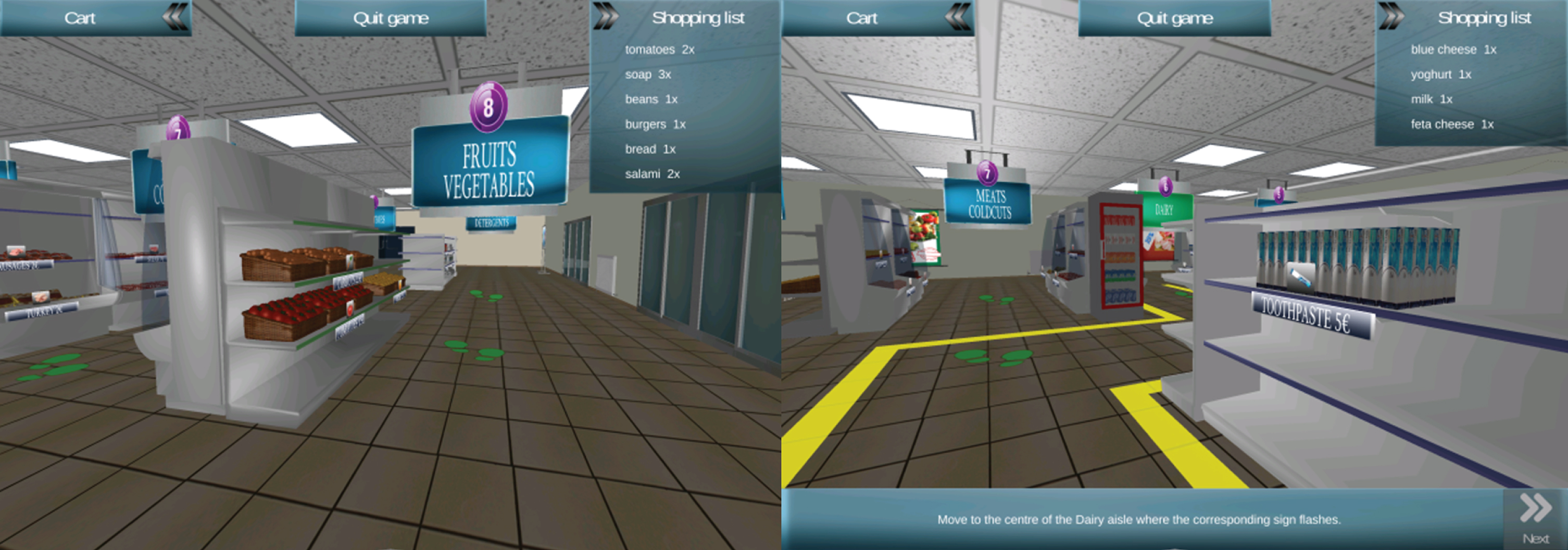
The current VST version features an interactive training session followed by a test session that is repeated three times. It includes new metrics and a refined protocol which allow for more detailed assessment, including trajectory analysis and assessment of practice effects. Furthermore, traditional metrics such as mistakes made while completing the exercise and time needed to complete each test trial are also recorded. A CE class 1 medical device approval for the VST is pending. Studies have shown that the VST outperforms gold standard pencil and paper cognitive screening tests, such as the MoCA, in differentiating between MCI patients and healthy controls [6, 8, 35, 43]. The VST application is aimed at activating a multitude of cognitive functions namely visual and verbal memory, executive function, attention and spatial navigation with the emphasis placed on executive function and navigation. The existence of a strong executive and visuospatial component in the VST has been demonstrated in previous studies through correlations of VST performance with performance on established pencil and paper tests assessing these domains [6, 8]. The need of simultaneous activation of different cognitive functions makes the VST challenging enough to correspond to the ability level of the target population (healthy older adults with SCD and MCI patients) while reducing ceiling effects.
EEG equipment and data acquisition
EEG data was obtained with a custom-made system working in parallel to the VST application. The data collection unit was purely unintrusive using a wireless EEG device equipped with a dry sensor (MindWave Mobile EEG Headset, NeuroSky Inc., USA) and was working in parallel to the user’s game experience. The synchronization between the user’s performance in the gaming environment and the EEG recording (data collection system) was achieved by a software component which was developed in-house by the authors using the Application Programming Interface (API) of the EEG portable device manufacturer (NeuroSky Inc., USA) and an adapted version of the VST game environment. Those adaptations were required in order to achieve integration of the two systems in a single and robust construct and also to achieve synchronization during data recordings so as to allow automatic annotation on the EEG recordings. In other words, the VST could add annotations for user’s interactivity tasks (e.g., tapping or swiping on the screen of the tablet where the VST app was running, etc.) on the raw EEG data recording at real-time. Finally, the architecture of the overall EEG system used to sense and store the variations in EEG oscillations arising from the cognitive load caused by the VST can be seen in Fig. 2.
Fig. 2
The overall architecture of the EEG data collection and game synchronization system.
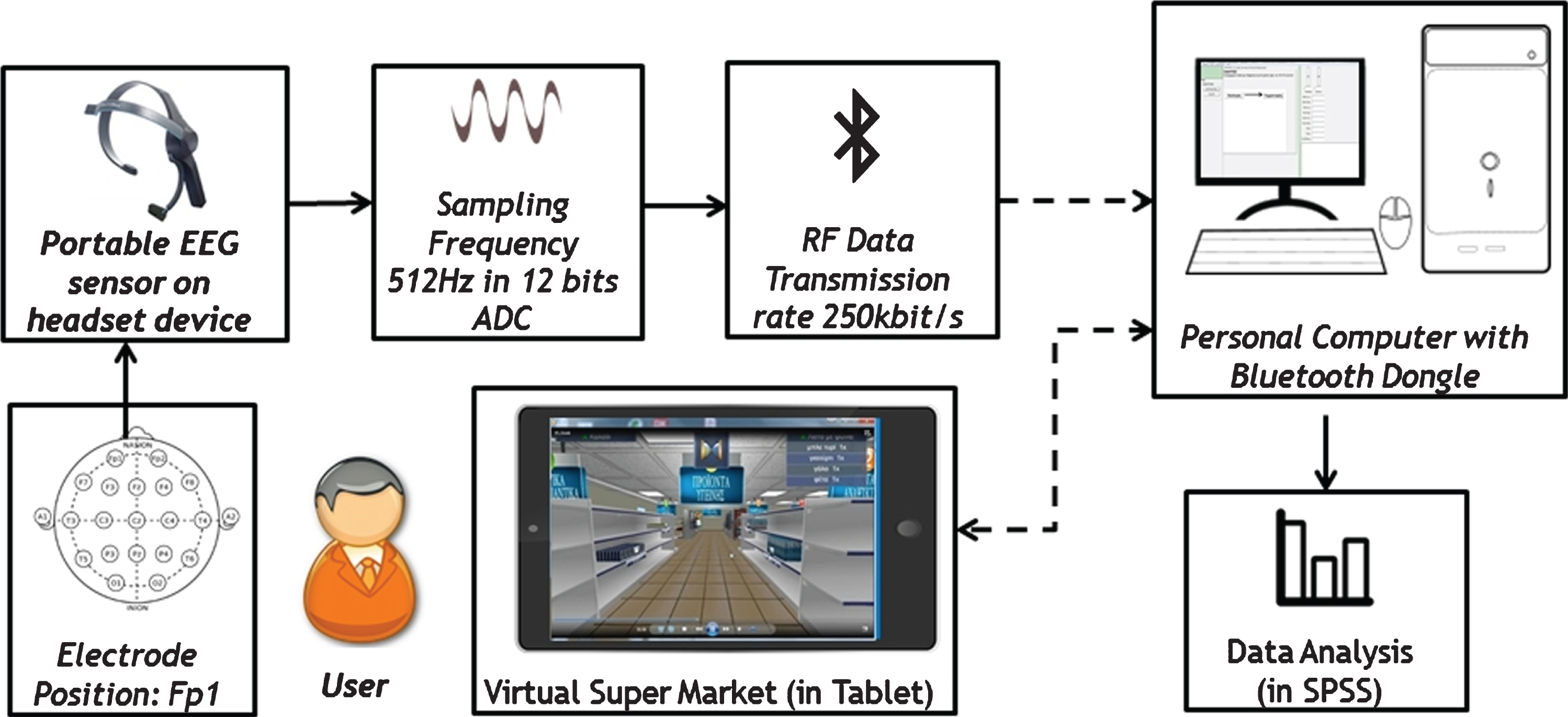
The part of the system which carries the physical sensors is the wearable dry biosensor device shown in the left part of the diagram. This device is capable of sensing and coding EEG signals in the reference bands, numerically expressed in the 100% scale of reference signal strength. A complete dataset consists of an array of eight (8) integer numbers in the range of 0–100, one for each EEG band (alpha low, alpha high, beta low, beta high, gamma low, gamma high, delta, and theta). Each signal strength was sensed from the left side of the forehead at Fp1 position according to the International 10–20 System of electrode placement recommended by the International Federation of Societies for Electroencephalography and Clinical Neurophysiology (https://www.ifcn.info), while a reference electrode was clipped to the left earlobe. The dry sensor device was selected because it is unintrusive, easy to use, portable, wireless, it can operate for long periods of time without the need to refresh lubricant on the contact point, and also because it allows the user to freely move the head during gameplay.
The sensing software was created by the study team and was capable of capturing the data from the device at a sampling frequency of 512 Hz in 12 bits ADC, but the data storage frequency was adapted according to each EEG band to avoid repetitive data (e.g., alpha band required less sampling frequency than 512 Hz). The wireless connection between the portable EEG sensor and the data collection software was made in RF 250 Kbit per second and a Bluetooth Dongle unit was used on the PC that was used for EEG data acquisition and storage to enable the data flow.
The VST game was installed in a tablet PC from which logfiles from the experiments were being sent to the PC that was used for EEG data acquisition and storage. At the same time (i.e., synchronized), the EEG sensing system used a simple application messaging protocol to send data to a local database on that PC (the VST was sending predefined text labels to the sensing software which were made visible in the log recordings). Each user recording was 30 to 45 min long and stored in computer files (logs) according to an Extensible Markup Language (XML) encoding schema. Those log files were later on statistically processed using the SPSS (version 24.0) statistical software package [46].
Participants
Participants were recruited from the sample of a study for the validation of a new computerized screening test for the detection of MCI [35]. The study sample included healthy older adults with SCD and older adults with amnestic MCI. Exclusion criteria were: diagnosis of dementia or another major neurological or psychiatric disorder, illiteracy, health issues such as motor and vision difficulties that could interfere with the use of the VST, treatment with cholinesterase inhibitors or other drugs that can affect cognitive performance, alcoholism, or drug abuse. Recruitment took place between April 2018 and April 2019 at the day centers of the GAADRD in Thessaloniki, Greece. MCI patients were paired randomly with older adults of similar age and education level, without MCI symptoms but with subjective memory complaints, in order to ensure a balanced sample. All participants were informed about the purpose of the study before providing their consent. Diagnosis was conferred by a neurologist after a full neurological, neuropsychological, and laboratory assessment. MCI diagnosis was based on Petersen criteria [2–4]. Participants with no objective impairment in neuropsychological testing were allocated to the SCD group.
Neuropsychological assessment
Participants were administered the MoCA, a brief, widely-used screening test for cognitive decline found to be more sensitive than the MMSE in detecting MCI [47, 48]. The Greek version of the MoCA was used in this study as a measure of global cognition [49].
As part of the diagnostic procedure for assigning participants to one of the two study groups (SCD and MCI), all participants were examined with a neuropsychological test battery including the following cognitive tests: MMSE [50], Rey Auditory Verbal Learning Test [51], a Greek version of the “F-A-S” verbal fluency test [52], Rey-Osterrieth Complex Figure Test [53], Rivermead Behavioural Memory Test [54], Test of Everyday Attention [55] subscales of Map Search, Visual Elevator, and Telephone Search, and Trail Making Test part B [56]. The battery also included the following functional scales: Functional Rating Scale for Symptoms of Dementia [57], Functional Cognitive Assessment Scale [58], and Clinical Dementia Rating (CDR) [59]. Furthermore, the battery included the following measures of anxiety and depression: Short Anxiety Screening Test [60] and the short form of the Geriatric Depression Scale [61].
Study protocol
After recording basic demographic information, participants were examined with the MoCA. EEG recordings were obtained in the morning with the participants sitting in a comfortable chair in a quiet room with the experimenter sitting beside them throughout the entire session. The tablet was placed approximately 30 cm on the desk in front of them. After explaining the procedure, the experimenter fitted the headset to the participant’s head, with the active electrode placed at FP1 of the international 10–20 system. All participants were exposed to five experimental conditions in the following order: 2 min resting state, VST interactive instructions, VST 1st trial, VST 2nd trial, VST 3rd trial (please see Fig. 1 for the experimental protocol). During the resting state, participants were asked to sit quietly with their eyes open for 2 min. Immediately after the resting state, the VST started with the instructions which were followed by the three test trials. All test trials featured the exact same task; essentially the participants had to repeat the same task three times. The overall procedure lasted approximately 30 min. The study was conducted in accordance with the Declaration of Helsinki ethical guidelines and was approved by the Scientific and Ethical Committee of the GAADRD.
2.6Statistical analysis
As per the study protocol, the performance of the study participants was systematically collected in a local database. Core game performance metrics include: 1) average duration for the three VST test trials, 2) mean number of errors conducted during the three VST test trials. Group differences in age and education were assessed by the Mann–Whitney U test while group differences in gender were assessed by the Chi-square test (Fisher’s exact). Average values for all three VST test trials were calculated for EEG bands, for all participants. These served as a measure of average brain activation (per band) throughout the three trials of the VST exercise in order to allow for comparison with global cognition metrics and VST overall performance metrics. Correlations between average EEG band values, the MoCA test score and VST core game performance metrics were calculated using Pearson correlation coefficient. Statistical analysis was conducted with the SPSS (version 24.0) statistical software package [46]. All EEG data was expressed as mean strength values of frequency bands, and neuropsychological test results as means±standard deviation (SD). Comparisons between user groups were performed using non-parametric tests. Results of these tests with a p value of less than 0.05 were considered as statistically significant. Pearson’s correlation analysis was used to determine the relationship between EEG and neuropsychological tests and between EEG and game scores.
RESULTS
Demographic characteristics of participants
The sample included 43 healthy older adults with SCD and 33 MCI patients. 18 participants were male and 58 were female. Mean age was 66.09 years (SD = 4.73) ranging from 54 to 75 years. Subjects had a mean of 13.05 (SD = 2.96) years of formal education ranging from 6 to 21 years of formal education. No statistically significant differences were observed between the SCD and MCI groups in age and education. Demographic characteristics of participants are presented in Table 1.
Table 1
Demographics characteristics of MCI patients and SCD
MCI (n = 33) | SCD (n = 43) | p | |
M (SD) | M (SD) | ||
Age (y) | 66.79 (5.01) | 65.56 (4.43) | 0.264 |
Education (y) | 12.39 (2.69) | 13.56 (3.09) | 0.089 |
Gender (M/F) | 9/24 | 9/34 | 0.591 |
MoCA | 25.41 (2.92) | 28.79 (1.08) | 0.000** |
MMSE | 28.06 (1.69) | 28.79 (1.08) | 0.025* |
M, mean; SD, standard deviation; MoCA, Montreal Cognitive Assessment; MMSE, Mini-Mental State Examination. Age and education were tested by Mann–Whitney U test, gender by Chi-square test (Fisher’s exact). *p < 0.05, **p < 0.001.
3.2VST performance per user group
MCI patients needed more time to complete the VST test trials (M = 215844.525 ms, SD =17916.684) in comparison to controls (M = 152279.441 ms, SD = 9542.890). The same pattern of differences was also evident when comparing time needed to complete the interactive instructions part of the VST. MCI patients needed 538381.225 ms to complete instructions (SD = 177135.610), compared to controls who completed instructions in 428671.333 ms (SD = 100372.768). Using the Mann-Whitney test, it was shown that group differences in time needed to complete the instructions (U = 0.399, p = 0.005) and the three test trials (U = 0.421, p = 0.002) were both statistically significant at p < 0.05. The mean number of errors in all 3 trials was 0.7 (SD = 1.1) in MCI whereas in SCD was 0.3 (SD = 0.5). Using the Mann-Whitney test, group differences in the mean number of errors in the three test trials were not found to be statistically significant (U = 0.597, p = 0.154).
EEG results
Group differences are presented in Fig. 3. The mean power of each frequency band for each group during resting state, VST instructions, and VST trials is presented in Table 2.
Fig. 3
Differences between user groups (MCI versus SCD) in EEG power during resting state condition, instruction and 3 trials. *p < 0.05, **p < 0.01.
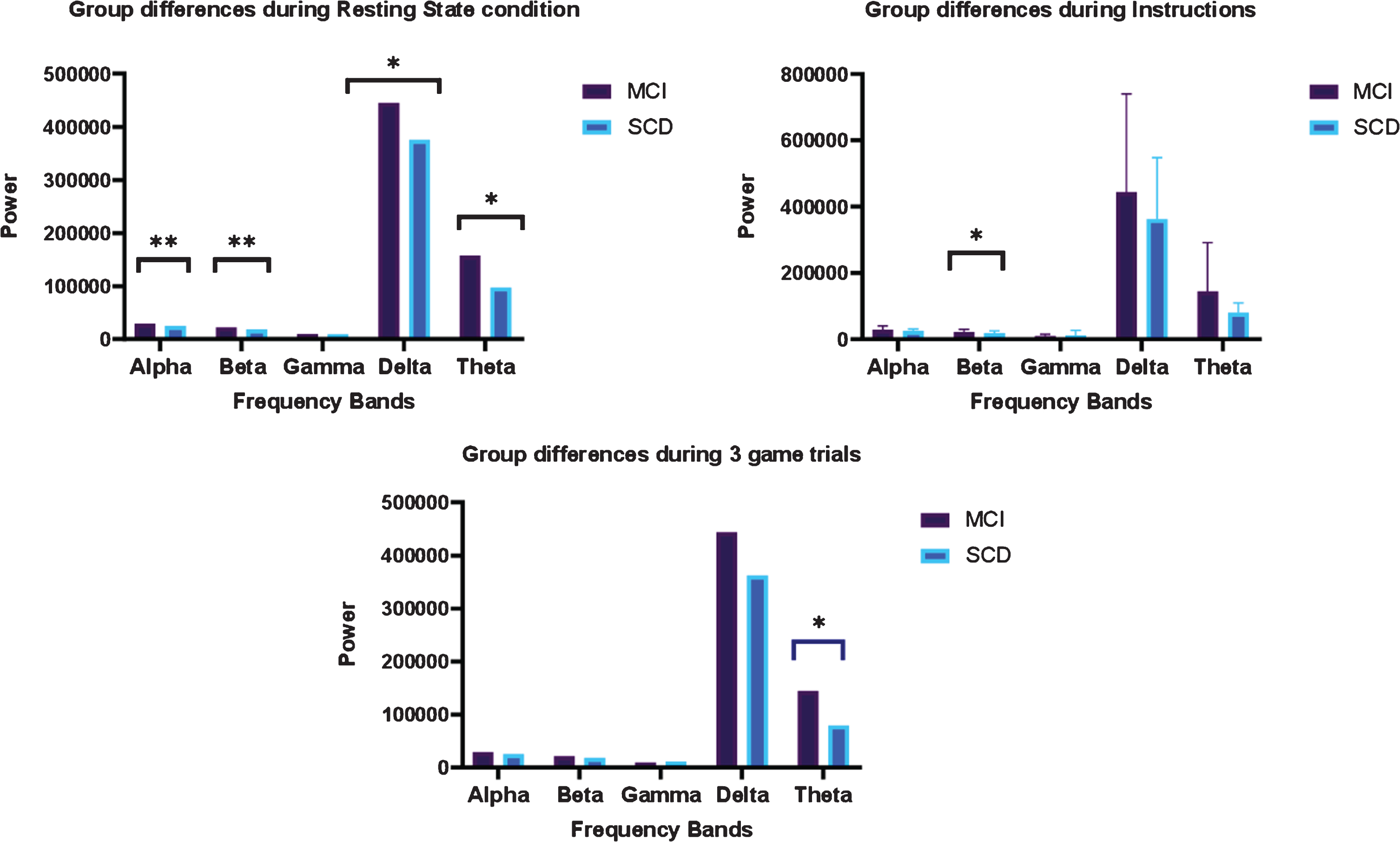
Table 2
Mean EEG frequency band strength
EEG frequency | MCI | SCD |
Resting State | ||
Alpha** | 22841.14 (SD = 9226.24) | 18389.09 (SD = 10420.13) |
Beta** | 18766.99 (SD = 9264.52) | 13655.78 (SD = 6043.81) |
Gamma | 7395.63 (SD = 3890.81) | 9128.54 (SD = 17127.57) |
Delta* | 321625.52 (SD = 233208.6) | 226263.76 (SD = 178521.89) |
Theta* | 87707.55 (SD = 66053.99) | 61088.38 (SD = 27199.06) |
Instructions | ||
Alpha | 27097.55 (SD = 13273.86) | 23312.23 (SD = 8837.58) |
Beta* | 20869.61 (SD = 11676.05) | 17079.91 (SD = 8052.66) |
Gamma | 8927.11 (SD = 5244.65) | 9006.06 (SD = 6113.83) |
Delta | 458691.16 (SD = 321263.01) | 351031.18 (SD = 205289.9) |
Theta | 179578.99 (SD = 129943.27) | 152082.97 (SD = 81218.24) |
Game Trials | ||
Alpha | 28924.75 (SD = 11095.65) | 25146.01 (SD = 5864.55) |
Beta | 21551.79 (SD = 7942.19) | 18314.62 (SD = 6415.07) |
Gamma | 9541.1 (SD = 5409.58) | 10930.74 (SD = 15831.19) |
Delta | 443569.48 (SD = 296656.56) | 362153.78 (SD = 185422.1) |
Theta* | 144494.91 (SD = 146916.58) | 79578.58 (SD = 29854.99) |
M, mean; SD, standard deviation *Mann-Whitney’s U test (p < 0.05); **Mann-Whitney’s U test (p < 0.001).
Group differences during resting state
During resting state, there were statistically significant group differences in alpha (U = 0.445, p = 0.009), beta (U = 0.426, p = 0.005), delta (U = 0.503, p = 0.048), and theta band (U = 0.495, p = 0.039). The mean power of alpha, beta, delta, and theta bands was significantly higher in MCI compared to SCD individuals.
Group differences during instructions
During instructions, there was a statistically significant difference (U = 0.497, p = 0.041) between MCI and SCD in beta rhythm. MCI showed increased beta power (M = 20869.61, SD = 11676.05) compared to SCD individuals (M = 17079.91, SD = 8052.66).
3.3.3Group differences during game trials (average of three game trials)
During test trials, there was a statistically significant difference between MCI and SCD in theta power (U = 0.411, p = 0.029) again with the first showing higher (M = 144494.91, SD = 146916.58) and the latter showing lower power (M = 79578.58, SD = 29854.99).
Correlations between global cognition and VST performance with EEG average values
Correlations between global cognition (as reflected by MoCA scores), VST performance and EEG average values are presented in Table 3. Alpha, beta, delta, and theta rhythms correlated with average game duration. Delta rhythm also positively correlated with mean errors. The magnitude of alpha, beta, delta, and theta activity was positively correlated with average game duration, i.e., the higher the EEG power in these frequency bands, the longer the duration. MoCA correlated with alpha, beta, delta, and theta rhythms. It also correlated with average game duration and mean number of errors. The magnitude of all correlations of MoCA with VST and EEG variables was negative, i.e., the higher the MoCA score the lower the EEG power, total average game duration and mean errors.
Table 3
Correlation coefficients between EEG frequency bands, MoCA score and VST game performance
MOCA | Game duration in 3 trials | Average errors 3 trials | Alpha | Beta | Gamma | Delta | Theta | ||
MOCA | Pearson Correlation | 1 | –0.487** | –0.456** | –0.478** | –0.247* | –0.097 | –0.286* | –0.338** |
Sig. (2-tailed) | 0.000 | 0.000 | 0.000 | 0.041 | 0.450 | 0.017 | 0.005 | ||
N | 75 | 75 | 75 | 68 | 69 | 63 | 69 | 69 | |
Game duration in 3 trials | Pearson Correlation | –0.487** | 1 | 0.575** | 0.488** | 0.406** | 0.104 | 0.516** | 0.276* |
Sig. (2-tailed) | 0.000 | 0.000 | 0.000 | 0.000 | 0.415 | 0.000 | 0.021 | ||
N | 75 | 76 | 76 | 69 | 70 | 64 | 70 | 70 | |
Average errors in 3 trials | Pearson Correlation | –0.456** | 0.575** | 1 | 0.220 | 0.197 | 0.107 | 0.386** | 0.130 |
Sig. (2-tailed) | 0.000 | 0.000 | 0.069 | 0.103 | 0.402 | 0.001 | 0.285 | ||
N | 75 | 76 | 76 | 69 | 70 | 64 | 70 | 70 |
MoCA, Montreal Cognitive Assessment; **Correlation is significant at the p < 0.01 level (2-tailed); *Correlation is significant at the p < 0.05 level (2-tailed).
DISCUSSION
The present study provides preliminary data on EEG power amplitude in MCI patients and healthy older adults with SCD, during the administration of the VST, by employing a new method of single-channel EEG measurement. Each of the two distinct VST conditions (interactive instructions VS test trials) placed different processing demands on participants and, as it was hypothesized, we found both EEG and game performance differences between our two study groups as well as correlations between EEG amplitude, game performance, and global cognitive performance (as assessed by the MoCA). In terms of VST performance, although the mean number of errors was not significantly different between groups, the time needed to complete the VST test trials (and also the time needed to complete the VST interactive instructions) was higher in the MCI compared to the SCD group. This finding is in line with previous studies using the VST [6, 8, 35, 43]. Furthermore, Weniger et al. [62] have also found that a greater percentage of MCI patients exceeded the time limit of a virtual park and a virtual maze task compared to controls, tasks that are similar to our virtual supermarket.
Regarding EEG recordings, our hypothesis has been confirmed. We hypothesized that MCI patients will have higher overall brain activation as measured by EEG. Indeed, during resting state condition, the MCI group showed increased alpha, beta, delta, and theta power compared to the control group. These results confirm previous studies demonstrating higher EEG power in the alpha, beta, and theta bands in MCI patients [27], which correlated negatively with global cognitive performance, i.e., MMSE scores [26]. This means that when EEG power increases, the MMSE scores decrease which could be a sign of cognitive decline. Moreover, studies have shown that MCI patients displaying higher alpha rhythm power also display greater global cortical atrophy suggesting a possible link between alpha rhythm and neurodegeneration [22].
While the majority of EEG studies (for all EEG rhythms) were focused on resting state EEG, only a few studies were focused on task-related EEG power changes. Our findings during instructions condition showed increased beta power in the MCI group compared to the control group. During instructions, which are a learning phase, participants are required to sustain their attention as well as understand and encode the game instructions in their working memory. Literature concerning beta oscillations during working memory tasks is not conclusive and often presents contradicting results. Jiang [26] investigated EEG power and coherence both at resting state and during a digit-span/ mental calculation test (assessing working memory) and found that all values of the EEG power in alpha, beta, and theta bands of MCI patients were higher than those of normal controls. On the contrary, a recent study showed significantly lower event-related synchronization (ERS)—band power increase—in the alpha and beta frequency band in MCI patients compared to healthy control participants during working memory maintenance [63]. A possible explanation for these contradictory results may be that the two studies used different working memory tasks and different methodology (ERPs versus mean absolute power). The increased alertness together with the motor demands of a novel stimulus, the game instructions, could be the cause of the generation of increased beta activity in MCI patients. The role of theta oscillations is clearer. During the game trials we found significant group differences in theta frequency band, again with MCI patients showing increased theta power compared to controls. Theta activity has been linked to episodic memory demands [30]. Increased theta power has been associated with cognitive decline [64] and with a slowing of processing speed in older adults [65]. Furthermore, a negative correlation has been observed between hippocampal volume and theta power in MCI and mild AD indicating a possible link between theta rhythm and hippocampal atrophy, but the exact underlying mechanism remains unclear [28]. Thus, our results agree with previous studies and the increased theta could be associated not directly with the task demands but with a more general cognitive impairment related to MCI.
In the present study, significant positive correlations were found for all participants between the power of alpha, beta, delta, and theta rhythms and average game duration meaning the higher the EEG power in these frequency bands, the longer the duration. Delta rhythm was also positively correlated with mean number of errors. Furthermore, alpha, beta, delta, and theta power was negatively correlated with MoCA scores which means the higher the power of these bands the lower the MoCA scores. To summarize, we have demonstrated that MCI patients displayed increased EEG power in alpha, beta, and theta bands while playing a serious game compared to healthy older adults with SCD, and this increase correlated with lower game performance and with lower MoCA scores. As highlighted by other studies, this “hypersynchronization” may be a compensatory mechanism or could be caused by a possible loss of inhibitory neurons in MCI patients [22, 66]. A compensatory mechanism is the capacity of the brain to recruit additional neuronal circuits to maintain the task performance as a compensation for neuronal degeneration [67, 68]. Given that EEG rhythms reflect underlying brain network activity, alterations in EEG rhythms in MCI patients could be an early sign of the disease involving structural and functional networks. In addition, as the EEG recording was performed with a low-profile device using a dry sensor, we could probably reach the conclusion that expensive EEG equipment may not be required for lengthy game sessions.
Strengths of this study include a robust diagnostic procedure for assigning participants into groups and the use of a well validated serious game application (i.e., the VST). Furthermore, the study sample is representative of the people who reach out to brain health services and present to memory clinics for assessment.
Limitations of this study include the inclusion of significantly more female participants. Furthermore, the study sample was recruited from a day center for people with cognitive disorders. Thus all participants, even those who did not present with cognitive impairment, had concerns about their cognitive functioning. Therefore, they may differ from a sample of healthy older adults recruited from the wider community in the sense that they are expected to have a particular interest in brain health and thus be more engaged during the administration of the VST. Additionally, the one-sensor EEG system used in the study cannot measure specific brain areas but measures the average activity of the whole brain with a stronger contribution of the frontal lobes.
Future directions comprise studies with more participants including healthy older adults with and without SCD. We expect that EEG differences between healthy older adults, with and without SCD, and MCI patients would provide a clearer picture of how brain activation differs in healthy aging and in MCI. Moreover, a similar series of trials in the near future to study correlations with more electrode positions could allow for more detailed measurement of brain activity differences between groups.
CONCLUSION
This study detected differences in brain activity, as measured by a low-cost wearable EEG dry sensor, during the administration of the Virtual Supermarket Test. The combination of a low-cost and non-invasive method of measuring EEG power and a self-administered serious game-based cognitive screening test allows for a screening and assessment paradigm that is more accessible and enjoyable for older adults. Study results support the utility of VST performance as a digital biomarker and also support the use of cheap commercially available wearable EEG devices for obtaining brain activity biomarkers in environments where expensive specialized equipment may not be available. Taking into account the fact that differentiating MCI from SCD is more challenging than detecting MCI in a sample of older adults without SCD, we believe that the combination of in-game performance parameters (i.e., measurable gameplay metrics) with a cheap, non-intrusive, and easy to use system for measuring EEG fluctuations can lead to better and more timely detection of cognitive decline.
ACKNOWLEDGMENTS
Stelios Zygouris is an Atlantic Fellow for Equity in Brain Health at the Global Brain Health Institute (GBHI) and is supported with funding from GBHI and the Alzheimer’s Association (GBHI_ALZ-18-541600). Stelios Zygouris also received a scholarship by the Robert Bosch Foundation Stuttgart within the Graduate Program People with Dementia in General Hospitals, located at the Network Aging Research (NAR), Heidelberg University, Germany. The authors would like to express their gratitude to all the study participants who gave generously of their time.
Authors’ disclosures available online (https://www.j-alz.com/manuscript-disclosures/20-1300r1).
REFERENCES
[1] | World Health Organization (2017) Global action plan on the public health response to dementia 2017-2025. |
[2] | Petersen RC , Doody R , Kurz A , Mohs RC , Morris JC , Rabins PV , Ritchie K , Rossor M , Thal L , Winblad B ((2001) ) Current concepts in mild cognitive impairment. Arch Neurol 58: , 1985–1992. |
[3] | Petersen RC ((2004) ) Mild cognitive impairment as a diagnostic entity. J Intern Med 256: , 183–194. |
[4] | Petersen RC , Roberts RO , Knopman DS , Boeve BF , Geda YE , Ivnik RJ , Smith GE , Jack CR Jr. . ((2009) ) Mild cognitive impairment: Ten years later. Arch Neurol 66: , 1447–1455. |
[5] | Albert MS , DeKosky ST , Dickson D , Dubois B , Feldman HH , Fox NC , Gamst A , Holtzman DM , Jagust WJ , Petersen RC , Snyder PJ , Carrillo MC , Thies B , Phelps CH ((2011) ) The diagnosis of mild cognitive impairment due to Alzheimer’s disease: Recommendations from the National Institute on Aging-Alzheimer’s Association workgroups on diagnostic guidelines for Alzheimer’s disease. Alzheimers Dement 7: , 270–279. |
[6] | Zygouris S , Giakoumis D , Votis K , Doumpoulakis S , Konstantinos N , Segkouli S , Karagiannidis C , Tzovaras D , Tsolaki M ((2015) ) Can a virtual reality cognitive training application fulfill a dual role? Using the Virtual Super Market cognitive training application as a screening tool for mild cognitive impairment. J Alzheimers Dis 44: , 1333–1347. |
[7] | Zygouris S , Tsolaki M (2015) New technologies and neuropsychological evaluation of older adults: Issues and challenges. In Handbook of Research on Innovations in the Diagnosis and Treatment of Dementia, Bamidis PD, Tarnanas I, Hadjileontiadis L, Tsolaki M, eds. IGI Global, USA, pp. 1-17. |
[8] | Zygouris S , Ntovas K , Giakoumis D , Votis K , Doumpoulakis S , Segkouli S , Karagiannidis C , Tzovaras D , Tsolaki M ((2017) ) A preliminary study on the feasibility of using a virtual reality cognitive training application for remote detection of mild cognitive impairment. J Alzheimers Dis 56: , 619–627. |
[9] | Tarnanas I , Schlee W , Tsolaki M , Müri R , Mosimann U , Nef T ((2013) ) Ecological validity of virtual reality daily living activities screening for early dementia: Longitudinal study. JMIR Serious Games 1: , e1. |
[10] | Torres A (2008) Cognitive effects of video games on older people. Proceedings. ICDVRAT, pp. 191-198. |
[11] | Lee B , Chen Y , Hewitt L ((2011) ) Age differences in constraints encountered by seniors in their use of computers and the internet. Comput Human Behav 27: , 1231–1237. |
[12] | Mohammadi A , Kargar M , Hesami E ((2018) ) Using virtual reality to distinguish subjects with multiple- but not single-domain amnestic mild cognitive impairment from normal elderly subjects. Psychogeriatrics 18: , 132–142. |
[13] | Atkins AS , Khan A , Ulshen D , Vaughan A , Balentin D , Dickerson H , Liharska LE , Plassman B , Welsh-Bohmer K , Keefe RSE ((2018) ) Assessment of instrumental activities of daily living in older adults with subjective cognitive decline using the Virtual Reality Functional Capacity Assessment Tool (VRFCAT). J Prev Alzheimers Dis 5: , 216–234. |
[14] | Gold M , Amatniek J , Carrillo MC , Cedarbaum JM , Hendrix JA , Miller BB , Robillard JM , Rice JJ , Soares H , Tome MB , Tarnanas I , Vargas G , Bain LJ , Czaja SJ ((2018) ) Digital technologies as biomarkers, clinical outcomes assessment, and recruitment tools in Alzheimer’s disease clinical trials. Alzheimers Dement (N Y) 4: , 234–242. |
[15] | Parsons TD , Rizzo AA , Brennan J , Bittman M , Zelinski E (2008) Assessment of executive functioning using virtual reality: Virtual environment grocery store. Proceedings. The 6th International Conference of the International Society for Gerontechnology. |
[16] | Ninaus M , Kober S , Witte M , Koschutnig K , Stangl M , Neuper C , Wood G ((2013) ) Neural substrates of cognitive control under the belief of getting neurofeedback training. Front Hum Neurosci 7: , 914. |
[17] | Schomer DL , Lopes da Silva FH ((2017) ) Niedermeyer’s Electroencephalography: Basic Principles, Clinical Applications, and Related Fields. Oxford University Press. |
[18] | Lizio R , Vecchio F , Frisoni GB , Ferri R , Rodriguez G , Babiloni C ((2011) ) Electroencephalographic rhythms in Alzheimer’s disease. Int J Alzheimers Dis 2011: , 927573. |
[19] | Hsu CF , Chao H-H , Yang AC , Yeh C-W , Hsu L , Chi S ((2020) ) Discrimination of severity of Alzheimer’s disease with multiscale entropy analysis of EEG dynamics. Appl Sci 10: , 1244. |
[20] | Tzimourta KD , Afrantou T , Ioannidis P , Karatzikou M , Tzallas AT , Giannakeas N , Astrakas LG , Angelidis P , Glavas E , Grigoriadis N , Tsalikakis DG , Tsipouras MG ((2019) ) Analysis of electroencephalographic signals complexity regarding Alzheimer’s disease. Comput Electr Eng 76: , 198–212. |
[21] | Oltu B , Akşahin MF , Kibaroğlu S ((2021) ) A novel electroencephalography based approach for Alzheimer’s disease and mild cognitive impairment detection. Biomed Signal Process Control 63: , 102223. |
[22] | Moretti DV ((2015) ) Theta and alpha EEG frequency interplay in subjects with mild cognitive impairment: Evidence from EEG, MRI, and SPECT brain modifications. Front Aging Neurosci 7: , 31–31. |
[23] | Babiloni C , Binetti G , Cassetta E , Forno GD , Percio CD , Ferreri F , Ferri R , Frisoni G , Hirata K , Lanuzza B , Miniussi C , Moretti DV , Nobili F , Rodriguez G , Romani GL , Salinari S , Rossini PM ((2006) ) Sources of cortical rhythms change as a function of cognitive impairment in pathological aging: A multicenter study. Clin Neurophysiol 117: , 252–268. |
[24] | Babiloni C , Frisoni G , Vecchio F , Lizio R , Pievani M , Geroldi C , Fracassi C , Vernieri F , Ursini F , Rodriguez G , Nobili F , Salinari S , Dijkman SV , Ferri R , Rossini PM ((2009) ) Global functional coupling of resting EEG rhythms is abnormal in mild cognitive impairment and Alzheimer’s disease. J Psychophysiol 23: , 224–234. |
[25] | Babiloni C , Visser PJ , Frisoni G , De Deyn PP , Bresciani L , Jelic V , Nagels G , Rodriguez G , Rossini PM , Vecchio F , Colombo D , Verhey F , Wahlund L-O , Nobili F ((2010) ) Cortical sources of resting EEG rhythms in mild cognitive impairment and subjective memory complaint. Neurobiol Aging 31: , 1787–1798. |
[26] | Jiang ZY ((2005) ) Study on EEG power and coherence in patients with mild cognitive impairment during working memory task. J Zhejiang Univ Sci B 6: , 1213–1219. |
[27] | Fauzan N , Amran NH ((2015) ) Brain dynamics of mild cognitive impairment (MCI) from EEG features. Procedia Soc Behav Sci 165: , 284–290. |
[28] | Grunwald M , Busse F , Hensel A , Kruggel F , Riedel-Heller S , Wolf H , Arendt T , Gertz HJ ((2001) ) Correlation between cortical theta activity and hippocampal volumes in health, mild cognitive impairment, and mild dementia. J Clin Neurophysiol 18: , 178–184. |
[29] | Rossini PM , Del Percio C , Pasqualetti P , Cassetta E , Binetti G , Dal Forno G , Ferreri F , Frisoni G , Chiovenda P , Miniussi C , Parisi L , Tombini M , Vecchio F , Babiloni C ((2006) ) Conversion from mild cognitive impairment to Alzheimer’s disease is predicted by sources and coherence of brain electroencephalography rhythms. Neuroscience 143: , 793–803. |
[30] | van der Hiele K , Vein AA , Reijntjes RH , Westendorp RG , Bollen EL , van Buchem MA , van Dijk JG , Middelkoop HA ((2007) ) EEG correlates in the spectrum of cognitive decline. Clin Neurophysiol 118: , 1931–1939. |
[31] | Das S , Puthankattil SD ((2020) ) Complex network analysis of MCI-AD EEG signals under cognitive and resting state. Brain Res 1735: , 146743. |
[32] | Ninaus M , Kober SE , Friedrich EVC , Dunwell I , Freitas Sd , Arnab S , Ott M , Kravcik M , Lim T , Louchart S , Bellotti F , Hannemann A , Thin AG , Berta R , Wood G , Neuper C ((2014) ) Neurophysiological methods for monitoring brain activity in serious games and virtual environments: A review. Int J Technol Enhanc Learn 6: , 78–103. |
[33] | Wen D , Lan X , Zhou Y , Li G , Hsu S-H , Jung T-P ((2018) ) The study of evaluation and rehabilitation of patients with different cognitive impairment phases based on virtual reality and EEG. Front Aging Neurosci 10: , 88. |
[34] | Tarnanas I , Laskaris N , Tsolaki M , Muri R , Nef T , Mosimann UP ((2015) ) On the comparison of a novel serious game and electroencephalography biomarkers for early dementia screening. Adv Exp Med Biol 821: , 63–77. |
[35] | Zygouris S , Iliadou P , Lazarou E , Giakoumis D , Votis K , Alexiadis A , Triantafyllidis A , Segkouli S , Tzovaras D , Tsiatsos T , Papagianopoulos S , Tsolaki M ((2020) ) Detection of mild cognitive impairment in an at-risk group of older adults: Can a novel self-administered serious game-based screening test improve diagnostic accuracy? J Alzheimers Dis 78: , 405–412. |
[36] | Molinuevo JL , Rabin LA , Amariglio R , Buckley R , Dubois B , Ellis KA , Ewers M , Hampel H , Klöppel S , Rami L , Reisberg B , Saykin AJ , Sikkes S , Smart CM , Snitz BE , Sperling R , van der Flier WM , Wagner M , Jessen F ((2017) ) Implementation of subjective cognitive decline criteria in research studies. Alzheimers Dement 13: , 296–311. |
[37] | Koppara A , Wagner M , Lange C , Ernst A , Wiese B , König H-H , Brettschneider C , Riedel-Heller S , Luppa M , Weyerer S , Werle J , Bickel H , Mösch E , Pentzek M , Fuchs A , Wolfsgruber S , Beauducel A , Scherer M , Maier W , Jessen F ((2015) ) Cognitive performance before and after the onset of subjective cognitive decline in old age. Alzheimers Dement (Amst) 1: , 194–205. |
[38] | Studart AN , Nitrini R ((2016) ) Subjective cognitive decline: The first clinical manifestation of Alzheimer’s disease? Dement Neuropsychol 10: , 170–177. |
[39] | Dauwels J , Vialatte F , Cichocki A ((2010) ) Diagnosis of Alzheimer’s disease from EEG signals: Where are we standing? Curr Alzheimer Res 7: , 487–505. |
[40] | Fraga FJ , Ferreira LA , Falk TH , Johns E , Phillips ND (2017) Event-related synchronisation responses to N-back memory tasks discriminate between healthy ageing, mild cognitive impairment, and mild Alzheimer’s disease. Proceedings. 2017 IEEE International Conference on Acoustics, Speech and Signal Processing (ICASSP), pp. 964–968. |
[41] | Han Y , Wang K , Jia J , Wu W ((2017) ) Changes of EEG spectra and functional connectivity during an object-location memory task in Alzheimer’s disease. Front Behav Neurosci 11: , 107. |
[42] | Iliadou P , Kladi A , Frantzidis CA , Gilou S , Tepelena I , Gialaouzidis M , Papaliagkas V , Nigdelis V , Nday CM , Kiosseoglou G , Papantoniou G , Bamidis PD , Tsolaki M , Moraitou D ((2019) ) The pattern of mu rhythm modulation during emotional destination memory: Comparison between mild cognitive impairment patients and healthy controls. J Alzheimers Dis 71: , 1201–1215. |
[43] | Eraslan Boz H , Limoncu H , Zygouris S , Tsolaki M , Giakoumis D , Votis K , Tzovaras D , Öztürk V , Yener GG ((2020) ) A new tool to assess amnestic mild cognitive impairment in Turkish older adults: Virtual supermarket (VSM). Neuropsychol Dev Cogn B Aging Neuropsychol Cogn 27: , 639–653. |
[44] | Tsolaki M , Zygouris S , Lazarou I , Kompatsiaris I , Chatzileontiadis L , Votis C , Tzovaras D , Karakostas A , Karagkiozi C , Dimitriou T , Tsiatsios T , Dimitriadis S , Tarnanas I , Dranidis D , Bamidis P ((2015) ) Our experience with informative and communication technologies (ICT) in dementia. Hell J Nucl Med 18: , 131–139. |
[45] | Wandke H , Sengpiel M , Sönksen M ((2012) ) Myths about older people’s use of information and communication technology. Gerontology 58: , 564–570. |
[46] | IBM Corp. (2016) IBM SPSS Statistics for Windows (V 24.0) |
[47] | Ciesielska N , Sokołowski R , Mazur E , Podhorecka M , Polak-Szabela A , Kędziora-Kornatowska K ((2016) ) Is the Montreal Cognitive Assessment (MoCA) test better suited than the Mini-Mental State Examination (MMSE) in mild cognitive impairment (MCI) detection among people aged over 60? Meta-analysis. Psychiatr Pol 50: , 1039–1052. |
[48] | Pinto TCC , Machado L , Bulgacov TM , Rodrigues-Júnior AL , Costa MLG , Ximenes RCC , Sougey EB ((2019) ) Is the Montreal Cognitive Assessment (MoCA) screening superior to the Mini-Mental State Examination (MMSE) in the detection of mild cognitive impairment (MCI) and Alzheimer’s disease (AD) in the elderly? Int Psychogeriatr 31: , 491–504. |
[49] | Poptsi E , Moraitou D , Eleftheriou M , Kounti-Zafeiropoulou F , Papasozomenou C , Agogiatou C , Bakoglidou E , Batsila G , Liapi D , Markou N , Nikolaidou E , Ouzouni F , Soumpourou A , Vasiloglou M , Tsolaki M ((2019) ) Normative data for the Montreal Cognitive Assessment in Greek older adults with subjective cognitive decline, mild cognitive impairment and dementia. J Geriatr Psychiatry Neurol 32: , 265–274. |
[50] | Fountoulakis KN , Tsolaki M , Chantzi H , Kazis A ((2000) ) Mini Mental State Examination (MMSE): A validation study in Greece. Am J Alzheimers Dis Other Demen 15: , 342–345. |
[51] | Messinis L , Tsakona I , Malefaki S , Papathanasopoulos P ((2007) ) Normative data and discriminant validity of Rey’s Verbal Learning Test for the Greek adult population. Arch Clin Neuropsychol 22: , 739–752. |
[52] | Kosmidis MH , Vlahou CH , Panagiotaki P , Kiosseoglou G ((2004) ) The verbal fluency task in the Greek population: Normative data, and clustering and switching strategies. J Int Neuropsychol Soc 10: , 164–172. |
[53] | Duley JF , Wilkins JW , Hamby SL , Hopkins DG , Burwell RD , Barry NS ((1993) ) Explicit scoring criteria for the Rey-Osterrieth and Taylor complex figures. Clin Neuropsychol 7: , 29–38. |
[54] | Efklides A , Yiultsi E , Kangellidou T , Kounti F , Dina F , Tsolaki M ((2002) ) Wechsler Memory Scale, Rivermead Behavioral Memory Test, and Everyday Memory Questionnaire in healthy adults and Alzheimer’s patients. Eur J Psychol Assess 18: , 63–77. |
[55] | Robertson IH , Ward T , Ridgeway V , Nimmo-Smith I ((1996) ) The structure of normal human attention: The Test of Everyday Attention. J Int Neuropsychol Soc 2: , 525–534. |
[56] | Zalonis I , Kararizou E , Triantafyllou NI , Kapaki E , Papageorgiou S , Sgouropoulos P , Vassilopoulos D ((2008) ) A normative study of the Trail Making Test A and B in Greek adults. Clin Neuropsychol 22: , 842–850. |
[57] | Hutton TJ (1990) Alzheimer’s disease. In Conn’s Current Therapy, Rakel RE, ed. WB Saunders, Philadelphia, pp. 780. |
[58] | Kounti F , Tsolaki M , Kiosseoglou G ((2006) ) Functional cognitive assessment scale (FUCAS): A new scale to assess executive cognitive function in daily life activities in patients with dementia and mild cognitive impairment. Hum Psychopharmacol Clin Exp 21: , 305–311. |
[59] | Morris JC ((1997) ) Clinical dementia rating: A reliable and valid diagnostic and staging measure for dementia of the Alzheimer type. Int Psychogeriatr 9: (Suppl 1), 173–176; discussion 177-178. |
[60] | Grammatikopoulos IA , Sinoff G , Alegakis A , Kounalakis D , Antonopoulou M , Lionis C ((2010) ) The Short Anxiety Screening Test in Greek: Translation and validation. Ann Gen Psychiatr 9: , 1. |
[61] | Fountoulakis KN , Tsolaki M , Iacovides A , Yesavage J , O’Hara R , Kazis A , Ierodiakonou C ((1999) ) The validation of the short form of the Geriatric Depression Scale (GDS) in Greece. Aging Clin Exp Res 11: , 367–372. |
[62] | Weniger G , Ruhleder M , Lange C , Wolf S , Irle E ((2011) ) Egocentric and allocentric memory as assessed by virtual reality in individuals with amnestic mild cognitive impairment. Neuropsychologia 49: , 518–527. |
[63] | Fodor Z , Sirály E , Horváth A , Salacz P , Hidasi Z , Csibri É , Szabó Á , Csukly G ((2018) ) Decreased event-related beta synchronization during memory maintenance marks early cognitive decline in mild cognitive impairment. J Alzheimers Dis 63: , 489–502. |
[64] | Trammell JP , MacRae PG , Davis G , Bergstedt D , Anderson AE ((2017) ) The relationship of cognitive performance and the theta-alpha power ratio is age-dependent: An EEG study of short term memory and reasoning during task and resting-state in healthy young and old adults. Front Aging Neurosci 9: , 364–364. |
[65] | Stomrud E , Hansson O , Minthon L , Blennow K , Rosén I , Londos E ((2010) ) Slowing of EEG correlates with CSF biomarkers and reduced cognitive speed in elderly with normal cognition over 4 years. Neurobiol Aging 31: , 215–223. |
[66] | López ME , Aurtenetxe S , Pereda E , Cuesta P , Castellanos NP , Bruña R , Niso G , Maestú F , Bajo R ((2014) ) Cognitive reserve is associated with the functional organization of the brain in healthy aging: A MEG study. Front Aging Neurosci 6: , 125–125. |
[67] | Clément F , Belleville S ((2010) ) Compensation and disease severity on the memory-related activations in mild cognitive impairment. Biol Psychiatry 68: , 894–902. |
[68] | Stern Y , Barnes CA , Grady C , Jones RN , Raz N ((2019) ) Brain reserve, cognitive reserve, compensation, and maintenance: Operationalization, validity, and mechanisms of cognitive resilience. Neurobiol Aging 83: , 124–129. |