The Characteristics of Social Network Structure in Later Life in Relation to Incidence of Mild Cognitive Impairment and Conversion to Probable Dementia
Abstract
Background:
Larger, more active social networks are estimated to be associated with lower risks of cognitive decline. However, roles of various social relationships in a broad social network in protecting against cognitive decline remain to be elucidated.
Objective:
We aimed to investigate how social roles within a social network and number of social network members are associated with cognitive decline.
Methods:
Six waves of National Health and Aging Trends Study (2011-2016, NHATS) were utilized to examine the development of mild cognitive impairment (MCI) and probable dementia determined using validated criteria. Multivariable-adjusted multi-state survival models were used to model incidences and transitions, jointly with misclassification errors.
Results:
A total of 6,078 eligible NHATS participants were included (average age: 77.49±7.79 years; female: 58.42%; non-Hispanic white: 68.99%). Multivariable-adjusted analyses revealed that having more social network members was associated with lower hazards of conversion from MCI to probable dementia (adjusted Hazard Ratio; aHR = 0.82; 95%confidence intervals; 95%CI = [0.67–0.99]), meanwhile having at least one college-educated family member within a social network was associated with lower incidence of probable dementia (aHR = 0.37 [0.26–0.51]). Having at least one friend within a social network was associated with a lower hazard of incidence of probable dementia (aHR = 0.48 [0.33–0.71]), but a higher risk of mortality in the MCI group (aHR = 2.58 [1.47–4.51]).
Conclusion:
Having more social network members, having at least one friend, and having at least one college-educated family member within a social network, were associated with lower risks of incidence of dementia or conversion from MCI to dementia.
INTRODUCTION
Alzheimer’s disease, the most common cause of old-age dementias, is the 6th leading cause of death in the United States (U.S.) and a substantial public hea-lth concern [1]. As the U.S. population ages, dementia in old age brings increasingly significant economic, psychological, and physical burdens [2]. Given that no effective diagnostic or therapeutic approaches for dementia are available to date, while some risk factors for cognitive impairment can be reversible when identified early [3], investigating modifiable risk factors associated with cognitive decline is critical to efforts to reduce the impact of the disease [4].
Social networks, defined as the collection of soc-ially meaningful ties that an individual subjectively recognizes and maintains [5], is one such modifiable factor in the cognitive aging process that has received an increasing amount of attention [6–8]. Decades of research have consistently revealed that the soc-ial network play a significant role in the progression of dementia in late life [9–11]. Some researchers have theorized that benefits of having larger, denser, or more active social networks result from reduced physiological stress [12], reduced cardiovascular risks [13], increased knowledge leading to healthier practices, increased sense of responsibility for others [14], or increased awareness of opportunities for better access to health care [15]. As an umbrella concept, social network definitions also can incorporate the provision of social support as a critical process in providing downstream health benefits [16].
Previous research has suggested that differences in role specificity within a social network can lead to differences in cognitive decline by providing div-erse cognitive interactions and resources [17, 18]. Relationships with family members and friends can provide instrumental (i.e., tangible aid and service) and emotional support (i.e., expressions of empathy, love, trust and caring) for health-related needs [19], while friendships are often assumed of higher quality and can exert greater emotional influence than family relationships [20]. Some studies even suggest that friendship is one of the most important factors impacting cognitive health [21]. Nonetheless, social networks exhibit many constants at midlife, notably in their size (i.e., number of social network members) and their hierarchical structuring but begin to change as individuals age. Indeed, creating and maintaining friendships can be costly—both in the amount of time that must be invested and the cognitive tasks that underlie social relationships [22]. Making and retaining social relationships as individuals age, however, can become difficult—an effect that may be compounded by onset of mild cognitive impairment (MCI) and dementia [23]. Yet, friendships may also be accompanied by engagement of social and cognitive skills and activities that promote both physical and mental wellbeing, and may in turn, reduce cognitive effects of aging [24].
Most studies have centered on social network size, without specific considerations about the characteristics of social network structures, including components of role specificity (i.e., spouses, relat-ives, friends, neighbors, and professionals) and characteristics (i.e., age, sex, and education) of members within a person’s social network [25]. The mechanism by which social network structure facilitates reductions in cognitive decline is less clear, such as whether the benefits come from diverse social relationships, a certain relationship role, or merely a larger network size [25]. Additionally, most studies estimated associations between social network and incidences of MCI and dementia, but few investigated the effects of social networks on the series of cognitive decline simultaneously, such as how social networks might affect incidence of MCI, conversion from MCI to probable dementia, and reversion from MCI to being cognitively unimpaired. Yet, the benefits to a complex social network likely operate differently at different points in time, potentially delaying the onset of MCI and/or dementia in old age and potentially reducing the risk of mortality for individuals at all points in life, as knowledgeable friends/family members seek out diagnosis and work to improve health as people age. Furthermore, it remains unclear including how social network composition and role specificity affect the holistic dementing processes stage by stage, as cognitive declines are sometimes nonlinear processes characterized by tremendous variation.
In this study, we aimed to investigate the impact of social network structure on the incidences of MCI and dementia, as well as on cognitive conversions from MCI to dementia, in the general U.S. population. While previous research commonly used traditional survival analyses to estimate effects of risk factors on a single cognitive outcome of interest, such as incidence of dementia, individuals can experience transitions between multiple cognitive stages that are often ignored by traditional modeling efforts. Recent advances have developed and applied a novel validated method to describe a series of change processes in cognitive function, which is the multi-state survival model (MSM) [26–28]. This method can be applied in MSMs among a large sample population to estimate the effect of social networks on the process of cognitive decline in one cohesive model, including the possibility of reversion, rather than separate estimations for different cognitive status in different models, as was done in previous studies. Consistent with prior analyses, we hypothesized that having a larger social network would be associated with reduced incidence of MCI and dementia, and lower rates of conversion from MCI to dementia. To extend this into social characteristics, we hypothesized that the characteristics of the social network structure, including having more friends and college-educated social network members, will further operate to reduce the risk of incidence of MCI and then conversion from MCI to dementia.
METHODS
Data
Data came from National Health and Aging Trends Study (NHATS), a national study of persons aged 65 and over, sampled from the Medicare enrollment file (Grant number NIA U01AG032947; available at http://www.nhats.org) and conducted by the Johns Hopkins Bloomberg School of Public Health. The NHATS study collects information of individuals living in communities and residential care settings, not from nursing homes. It allows a proxy to provide inf-ormation for a respondent with severe cognitive, speech, or hearing problems. We included the initial sample recruited in 2011 and followed annually until the wave in 2016. Although a replenishment of the sample to maintain representation for the older Medicare population was conducted in 2015 [29], our ana-lytic sample only targeted at the first cohort enrolled in 2011, because we could not reliably measure inc-idence and conversion to dementia in a sample with only two waves. We excluded respondents who had only one wave of cognitive status, because they lac-ked the key outcomes (transitions in cognitive status).
Measures
Cognitive status was characterized in NHATS into three groups, cognitively unimpaired, MCI, and probable dementia, with reasonably good sensitivity and specificity [30, 31]. This protocol used data from three types of information: 1) self-reported or proxy-reported physician diagnosis of dementia including Alzheimer’s disease; 2) a score indicating probable dementia on the eight-item Dementia Screening Interview (AD8) reported by an informant of participants [32]; and 3) a series of cognitive tests for memory (immediate and delayed 10-word recall test), orientation (date, month, year, and day of the week; naming the President and Vice President test), and executive function (clock drawing test). Probable dementia was identified by at least one of the following: 1) reported dementia diagnosis; or 2) AD8 scores > = 2; or 3) a score of ≤1.5 standard deviations below the mean in at least two of three (orientation, memory, and executive functioning) cognitive functioning domains tested. MCI was defined by a score of ≤1.5 standard deviations below the mean on at least one of the three cognitive tests (orientation, memory, and executive functioning), which is the frequently adapted “typical criteria” [33]. Respondents were classified into cognitively unimpaired if conditions of MCI and probable dementia were not satisfied.
Social network size and structure were measured by the most well-known and widely used “name generator” approach [5, 34, 35], wherein responde-nts were asked to list up to five individuals with whom they could discuss important matters. Each respondent completed a detailed survey discussing relationship type and information of his/her social network members. An individual’s social network size is defined as a continuous variable denoting the total number of one’s social network members. Frie-nds were subjectively recognized by the respondents themselves. Dichotomous variables were created separately for having at least one family member, having at least one friend, and having at least one college-educated family member, within the individual’s social network. We used baseline social network variables in data analysis.
Confounders included participant’s age in years at baseline, sex (male or female), educational level (less than high school graduate, high school graduate and equivalent, college graduate and beyond), and race/ethnicity (Non-Hispanic white, Non-Hispanic black, Hispanic, other minorities). According to previous research, advanced age and sex are the two strongest risk factors for cognitive decline. Race and ethnicity are also found to be related to risks of cognitive impairment and progression to dementia [36]. Educational level plays an important role in both cognitive assessment and decline processes.
Statistical analyses
MSM can be appropriately used to explore ind-ividual-level longitudinal data based on the Markov disease process, where an individual can enter or exit one of several defined distinct states to transit at any given time during the observation period. Just as a survival model is understood via the hazard function, MSM can be understood via transition intensity functions. In our MSMs, a state represented the cognitive status of an individual in interview. We used a four-state (i.e., cognitively unimpaired, MCI, probable dementia, and death) MSM to assess transitions between these cognitive states. Transitions from one state to another reflected changes in the individual’s cognitive condition. In our MSMs, cognitive states of respondents can transit between four consecutive states in cognitive decline (successively progressing from cognitively unimpaired to, MCI to, probable dementia to death), revert from only MCI to cognitively unimpaired, develop probable dementia directly from cognitively unimpaired, and transit to death from any state (Fig. 1) [27, 37]. Time-to-event analyses require dates of each interview, as well as dates of death. As such, all the follow-up dates (month/year) were reported and each time interval between the first survey and a follow-up interview was measured in months. Missing values of survey month among individuals with a follow-up year were replaced by the modal month in that wave. Death dates were reported by informants, and missing values of the month of death were estimated to be six months after the last follow-up.
Fig. 1
Four-state survival model for cognition. We built a multi-state survival model (MSM) for cognitive functioning of the respondents, including cognitively unimpaired state, mild cognitive impairment (MCI) state, probable dementia state and death state. The arrows indicate the feasible instantaneous state-to-state transitions. In our model, respondents can transit between consecutive states in cognitive decline (i.e., successively progressing from cognitively unimpaired to, MCI to, probable dementia to death), revert from MCI to cognitively unimpaired, develop probable dementia from cognitively unimpaired, and transit to death from any state.
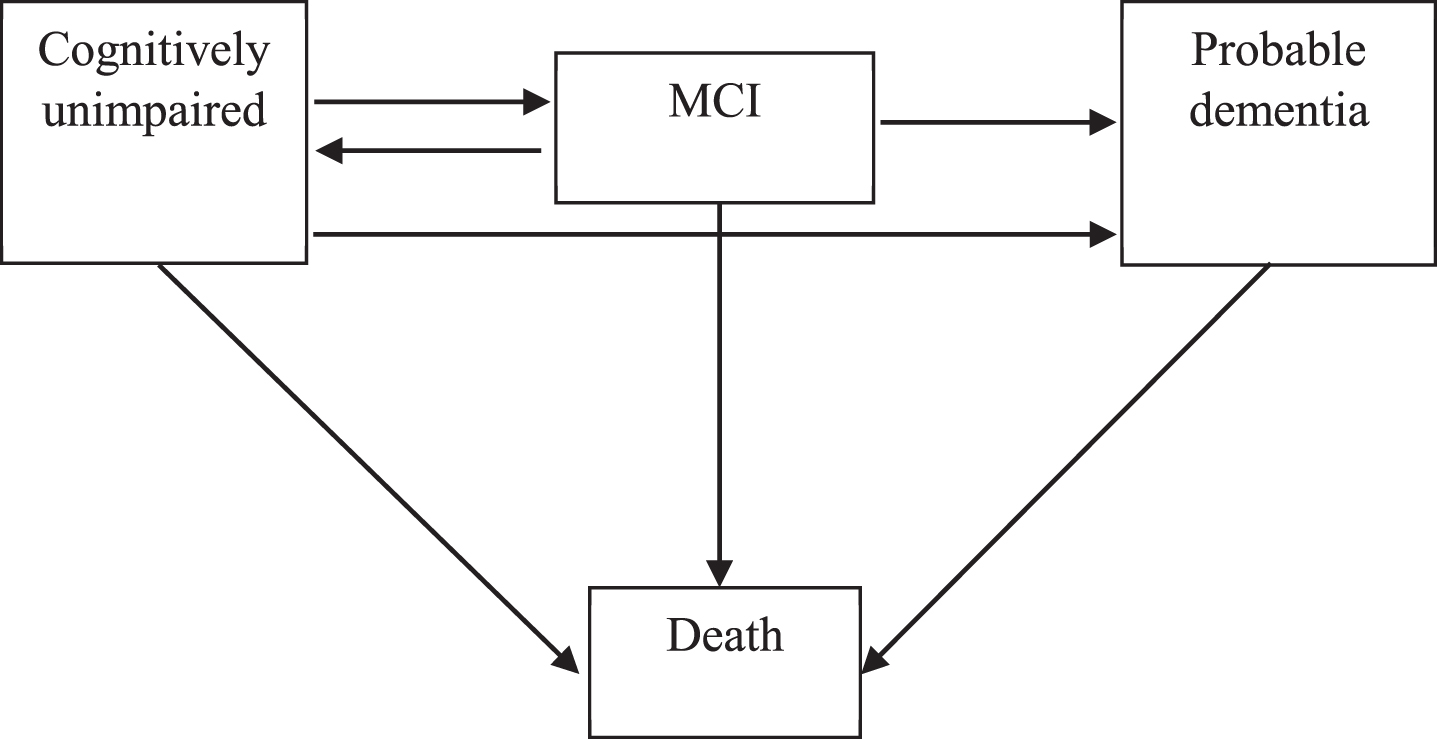
The test scores and diagnosis information of res-pondents can be seen as assessments of their latent cognitive statuses. There is a possibility that test scores and diagnosis information of a few individu-als were affected by measurement errors and other types of errors, causing misclassifications of the actual state. For example, respondents with MCI may reasonably be misclassified as dementia, or as cognitively unimpaired. Notably, reversion from dementia to MCI or to cognitively unimpaired have been found uncommon in old age, which should be treated as misclassifications. The simple MSM model was governed by a transition intensity matrix for the observation process of the underlying states; to account for misclassification, we further fit the simple MSM with a misclassification matrix as an extension, indicating the possibility of error. Covariates measured at baseline included age in years, sex, education, and race/ethnicity.
Statistical analyses were performed using a MSM package in R Studio [38]. All statistical tests used two-tailed alpha = 0.05 to determine statistical significance coefficients, and 95%confidence intervals (95%CI) were also used.
RESULTS
Baseline characteristics of respondents are shown in Table 1. A total of 6,078 respondents were in-cluded. The respondents had an average age of 77.49±7.79, annually followed on average of 4.72±1.61 years. The majority were high school or equivalent graduates (51.27%) and Non-Hispanic whites (68.99%). At baseline, their average social network size was 1.93±1.29. Most respondents had at least one family member in their social network (81.66%), but only a few had at least one friend in their social network (17.50%). Nearly one third of the respondents had at least one college-educated family member within a social network (30.07%). In Supplementary Table 1, we presented baseline characteristics of the respondents accounting for NHATS survey design of weights, strata, and clusters [29]. The unadjusted conversion rates were reported in the Supplementary Table 2.
Table 1
Baseline demographic characteristics of eligible respondents
Characteristic | N (%) |
Female sex | 3,551 (58.42) |
Education | |
Less than high school | 1,585 (26.32) |
High school graduate or equivalent | 3,088 (51.27) |
College graduate or beyond | 1,350 (22.41) |
Race/ethnicity | |
Non-Hispanic white | 4,193 (68.99) |
Non-Hispanic black | 1,316 (21.65) |
Hispanic | 353 (5.81) |
Other minorities | 216 (3.55) |
Social network structure | |
Having at least one family member within a social network | 4,942 (81.66) |
Having at least one friend within a social network | 1,059 (17.50) |
Having at least one college-educated family member within a social network | 1,820 (30.07) |
Total | 6,078 |
Mean (SD), N | |
Age at baseline | 77.49 (7.79), 6,078 |
Follow-up time (y) | 4.72 (1.61), 6,078 |
Social network size | 1.93 (1.29), 5,709 |
Number of family members within a social network | 1.51 (1.21), 6,052 |
Number of friends within a social network | 0.25 (0.61), 6,052 |
Analytic sample includes NHATS respondents who had at least two waves of cognitive status during the follow-up period of 2011-2016. SD, standard deviation.
As shown in Fig. 2, we ran three MSMs accounting for age, sex, education, and race/ethnicity, to separately explore the effects of social network size, having a friend in the social network, and having a college-educated family member within a social network on cognitive decline. We found that having a larger social network was significantly associated with decreased the risk of conversion from MCI to probable dementia (adjusted Hazard Ratio; aHR = 0.82; 95%CI [0.67–0.99]). Having at least one friend within a social network was associated with a 52%lower hazard of incidence of dementia (aHR = 0.48 [0.33–0.71]), but an increased mortality risk in the MCI group (aHR = 2.58 [1.47–4.51]). Having at least one college-educated family member within a social network was associated with reduced incidence of probable dementia (aHR = 0.37 [0.26–0.51]). We did not find statistically significant associations between social network structure and incidence of MCI.
Fig. 2
Four-state survival models for adjusted Hazard Ratios of social network structure on cognitive transitions. Adjusted hazard ratios of social network size (see Supplementary Table 3 for full results). B) Adjusted hazard ratios of having at least one friend within a social network (see Supplementary Table 4 for full results). C) Adjusted hazard ratios of having at least one college-educated family member within a social network (see Supplementary Table 5 for full results). Numbers expressed as pooled adjusted Hazard Ratios with [95%Confidence Interval]. Full results emerging from multivariable adjusted models are presented in Supplementary Tables 3-5.
![Four-state survival models for adjusted Hazard Ratios of social network structure on cognitive transitions. Adjusted hazard ratios of social network size (see Supplementary Table 3 for full results). B) Adjusted hazard ratios of having at least one friend within a social network (see Supplementary Table 4 for full results). C) Adjusted hazard ratios of having at least one college-educated family member within a social network (see Supplementary Table 5 for full results). Numbers expressed as pooled adjusted Hazard Ratios with [95%Confidence Interval]. Full results emerging from multivariable adjusted models are presented in Supplementary Tables 3-5.](https://ip.ios.semcs.net:443/media/jad/2021/81-2/jad-81-2-jad201196/jad-81-jad201196-g002.jpg)
Particularly, among respondents who developed probable dementia from cognitively unimpaired and those who experienced transition from MCI to death, we explored their demographic characteristics by various social network structure. As is shown in Table 2, among respondents experienced transition from MCI to death, no significant differences in sex, education, and race/ethnicity were observed between respondents who had at least one friend within a social network and who did not. Among respondents who developed probable dementia from cognitively unimpaired, respondents having at least one friend within a social network were more likely to be fem-ales (p < 0.001), meanwhile those who had at least one college-educated family member within a social network were more likely to be college graduates themselves (p < 0.001) and Non-Hispanic whites (p = 0.006).
Table 2
Descriptive characteristics of respondents classified by cognitive transition
Cognitively unimpaired to probable dementia | Mild cognitive impairment to death | Cognitively unimpaired to probable dementia | |||||||||||||
Characteristics | Have a friend1 | No friend1 | P | Have a friend2 | No friend2 | p | Have a college-educated family member1 | Have no college-educated family member1 | p | ||||||
n | % | n | % | n | % | n | % | n | % | n | % | ||||
Female | 49 | 81.67 | 269 | 59.65 | <0.0013 | 15 | 75.00 | 37 | 50.68 | 0.0523 | 101 | 66.01 | 217 | 60.61 | 0.2493 |
Education | |||||||||||||||
Less than high school | 20 | 33.90 | 169 | 37.64 | 0.6963 | 6 | 30.00 | 30 | 41.10 | 0.3123 | 32 | 21.19 | 157 | 43.98 | <0.0013 |
High school graduate or equivalent | 30 | 50.85 | 202 | 44.99 | 10 | 50.00 | 37 | 50.68 | 71 | 47.02 | 161 | 45.10 | |||
College graduate or beyond | 9 | 15.25 | 78 | 17.37 | 4 | 20.00 | 6 | 8.22 | 48 | 31.79 | 39 | 10.92 | |||
Race/ethnicity | |||||||||||||||
Non-Hispanic white | 36 | 60.00 | 279 | 61.86 | 0.3234 | 12 | 60.00 | 45 | 61.64 | 0.9154 | 112 | 73.20 | 203 | 56.70 | 0.0064 |
Non-Hispanic black | 20 | 33.33 | 109 | 24.17 | 6 | 30.00 | 23 | 31.51 | 28 | 18.30 | 101 | 28.21 | |||
Hispanic | 3 | 5.00 | 46 | 10.20 | 1 | 5.00 | 3 | 4.11 | 10 | 6.54 | 39 | 10.89 | |||
Other minorities | 1 | 1.67 | 17 | 3.77 | 1 | 5.00 | 2 | 2.74 | 3 | 1.96 | 15 | 4.19 | |||
Total | 60 | 451 | 20 | 73 | 153 | 358 |
1Among individuals experienced incidence of probable dementia from cognitively unimpaired. 2Among individuals experienced transition from mild cognitive impairment to death. 3Tested by Chi-square test. 4Tested by Fisher’s exact test.
In Table 3, we described the characteristics of soc-ial network members linked to our respondents. The majority of the social network members were females (63.96%). They were mainly children (45.02%) and spouse/partners (21.59%) of the participants.
Table 3
Characteristics of social network members linked to NHATS respondents
Social network members of all the respondents | Social network members of respondents who experienced incidence of dementia from cognitively unimpaired | Social network members of respondents experienced mild cognitive impairment to death | ||||
Characteristics | N | (%) | N | (%) | N | (%) |
Female sex | 7063 | 63.96 | 553 | 62.2 | 114 | 69.51 |
Relationship type | ||||||
Spouse/partner | 2384 | 21.59 | 139 | 15.64 | 26 | 15.85 |
Children | 4972 | 45.02 | 477 | 53.66 | 76 | 46.34 |
Sibling | 1254 | 11.36 | 74 | 8.32 | 9 | 5.49 |
Parent | 36 | 0.33 | 3 | 0.34 | 2 | 1.22 |
Grandchildren | 156 | 1.41 | 21 | 2.36 | 1 | 0.61 |
Niece/nephew/cousin | 282 | 2.55 | 41 | 4.61 | 10 | 6.1 |
Aunt/uncle | 23 | 0.21 | ||||
Other relative | 40 | 0.36 | 6 | 0.67 | 1 | 0.61 |
Friend | 1506 | 13.64 | 81 | 9.11 | 26 | 15.85 |
Other non-relative | 390 | 3.53 | 47 | 5.29 | 13 | 7.93 |
Total | 11043 | 100 | 889 | 100 | 164 | 100 |
This table describes characteristics of social members linked to NHATS respondents.
The covariate effects of age, sex, education, and race/ethnicity were also estimated in our adjusted MSMs and presented in Supplementary Tables 3–5. Consistent across all the MSMs, baseline age was significantly associated with increased possibilities of MCI and probable dementia, an increased risk of death in the probable dementia group, as well as an increased possibility of reversion from MCI to cognitively unimpaired. Compared to females, males with MCI suffered from the greater mortality risk. Older adults with higher education levels were at decreased risks of developing MCI and probable dementia from cognitively unimpaired. Compared to Non-Hispanic whites, Non-Hispanic blacks and Hispanics both suffered increased risks of incidence of MCI and probable dementia.
DISCUSSION
The social network is a central part of everyday life and a critical component to healthy aging of older adults. Controlling for baseline age, sex, education, and race/ethnicity, our results suggested that social network structure in later life was effectual in reducing the risk of cognitive decline. Here, we found three aspects of social networks that are protective against worse cognitive decline, including: 1) having more social network members, 2) having at least one friend within a social network, and 3) having at least one college-educated family member within a social network. These findings supported multiple potential pathways, including social network size and specific type and characteristic of social network members, that link social networks to the incidence and transition of MCI and dementia. In particular, we add to the previous literature on the benefits of social network structure by defining the role of network diversity against abnormal aging processes.
We found important protective effects of having more social network members, consistent with previous studies. Indeed, since Berkman and Syme’s seminal paper revealed the strong associations between social networks and health outcomes [39], a number of studies have confirmed that individuals who have fewer social members tend to have worse cognitive function and more severe cognitive decline [40, 41]. Crooks and colleagues demonstrated that women with one more social network member have a decreased adjusted hazard ratio of dementia of 0.74, compared to those who had one less [41]. Our results support this work in suggesting that even one more social network member specifically provides an 18%reduction of risk of dementia.
Our findings also provide evidence for the beneficial effects of having friends within one’s social network, showing that the resilient protective effects brought by role specificity of social networks play a significant role in compensating for cognitive damages. Research posits that social network structure affects cognitive decline by shaping the flow of resources related to access to opportunities and constraints on behavior [42, 43]. As health declines, older adults tend to seek immediate and tangible support from family members [44], while their friends can bring opportunities for diverse social engagements and social resources that help to promote emotional health, further help to maintain good cognitive function [12].
The diversity of the social roles of social network members can better capture the provision of different and complementary resources for various needs in specific situations [25]. One interesting study classified social networks into four types (diverse, friend-focused, family-focused, and restricted) by a K-means clustering method [45] and then reported a vicious cycle emerged when worse cognitive fun-ctioning degraded relationship-diverse and friend-focused networks to become more restricted, which in turn might cause a greater reduction of health benefits from social networks [45]. As people age, they gradually lose, forget, or withdraw from their original social network connections, and they also lose the ability to form new relationships [46]. Consequently, the presence of family members within a social net-work increases while other roles decrease. Yet, cognitive decline brought by the loss of friends and neighbors is ultimately irreplaceable by an increase in the presence of family members in the social network [47]. It further indicates a worrying crisis characterized by the accelerated decline of cognitive function, as people age and lose friends. It is important to address not only social network size, but also the structure of social networks, which is commonly underscored.
Apart from the significant effects of role specificity within a social network, our results further highlight the impact of education levels of social network members on the cognitive decline of the network owner. Independent from one’s own education level, an individual having at least one college-educated family member within a social network had a lower risk of dementia incidence (aHR = 0.37 [0.26–0.51]). We propose that the benefits of having college-educated family members come from the provision of social networks with embedded social resources brought by college-educated family members within social networks, which can help stimulate the brain and retards cognitive decline [48]. Increased health knowledge and access to health information can help reduce the effects of stress on the central nervous systems, which otherwise would alter immune response, impair cardiovascular function and memory process. At the same time, increased knowledge and a better awareness of access to health care brought by communications with college-educated people can lead to healthier behaviors. In the classical cognitive reserve theory, education is said to help maintain cognitive function in late life by buffering against the effects of clinical neuropathology expression [49]. Expanding on the theory of cognitive reserve, our study uniquely noted the importance of benefits provided by having college-educated social network members. This is a novel finding, and future research could further investigate this phenomenon and mechanisms behind.
Our analysis of the characteristics of our respondents further demonstrated that, compared to people who do not have any family member within a social network, those who have are more likely to be col-lege-educated himself/herself and a non-Hispanic white. This reveals a potential family clustering of cumulative effects of education on cognitive decline, considering that college-educated older citizens tend to have college-educated family members within their social networks, and vice versa. Independent of one’s own education level, an individual can be affected by his/her family members’ education levels. The val-ues, norms, and attitudes prevalent in social networks have been shown to influence family members in ways that can promote or damage health [50]; for example, unhealthy habits can be accepted or encou-raged easily within the family environment. Mean-while, consistent with previous evidence of racial/ethnic disparities, minority groups of non-Hispanic black and Hispanic have an increased cognitive dec-line risk compared to non-Hispanic whites. Racial/ethnic groups are at obvious disadvantages in education, further raising a concern of the hidden risks and disparities among less educated minority groups, which calls for special further attention. In sum, socioeconomic status (SES) and cultural conditions shape the nature and characteristics of an individual’s social network, which in turn impacts one’s cognitive health by providing different interaction and exchanges.
Given the significant protective role and modifiable nature of social networks, interventions that leverage social networks to decrease the risk of cognitive decline are supported [10]. Our research recommends the provision of sophisticated network models, not only to correspond to the popular “aging in place” model [51], which assumes that living in a residence of an individual’s preference can help better maintain a familiar and well-rounded life, but also to tap into the many more dimensions of social and community ties, especially with friends and higher educated people, in addition to the original social ties [52]. Those social ties can offer opportunities for social engagement and help reinforce social ties within family, community, and even beyond, which in turn provide a sense of value, belonging and attachment and facilitate better access to social resources through the flow of social networks.
Strengths and limitations
Commonly used approaches to analyze the effects of social networks on cognitive decline in previous studies include the Cox proportional hazards regression and multilevel modeling, but these approaches are increasingly recognized as being impeded by multiple barriers, which fail to provide insights into the processes that occur after the first event of interest [53–55]. Thus, our study is novel in using MSMs and a national sample to explore the effects of social network structure on the processes of cognitive decline. Especially, our MSMs used a non-homogeneous Markov process to simulate the entire dementing processes accounting for potential misclassifications.
Nevertheless, despite the key strengths above, there are some limitations in our research worth considering when examining the conclusions of this study.
First, the NHATS study design included oversamples of older age groups and black individuals race on the Medicare enrollment file. However, the MSM package we used does not yet allow researchers to account for the survey design [38], thus we reported unweighted analyses, thereby diminishing generalizability in particular by over-weighting populations, such as the oversampled minorities.
Second, NHATS participants were classified based on cut offs of cognitive tests with some complementary information, rather than clinical diagnosis, which may cause misclassifications. However, though impe-rfect, NHATS protocol can still be seen as a validated screening approach with reasonably good sensitivity and specificity in population study [30, 31]. Also, the standardized informant reporting metrics were used to help indicate dementia diagnoses. Furthermore, our MSMs accounting for misclassifications also helped improve the estimating accuracy.
Third, we allowed some direct progressions from cognitively unimpaired to probable dementia in MSMs, ignoring their transitional MCI states. This relationship, although successfully modeled here, indicated rapid decline in the dementing processes among some individuals. These individuals experienced more rapid cognitive decline than the general population. We anticipate that these individuals are more likely to have dementia from cerebrovascular disease, which has been previously shown to cause rapid declines in cognition [56]. Future studies on cognitive transitions could follow up and observe more frequently to explore.
Fourth, potential confounding effects coming from SES and mental and physical function are also important to consider and account for in future research. Social networks are often confounded with marital status, which is a social network in itself, but also with higher SES and its relevant influence on social contextual factors including community social capital. Higher SES is known to increase access to beneficial social networks—indeed, prominent sociomedical theories, including Fundamental Cause Theory [57, 58], argue that higher SES is characterized by the presence of so-called beneficial social networks. Household income and the life-longest occupation of the respondents could help explain our finding regarding the presence of college-educated social network members. However, due to limitations that regularly occur when MSMs incorporate larger numbers of covariates, these models did not converge, and we therefore suggest that future research should focus explicitly on understanding the causal relationship between SES and social networks when examining outcomes relating to the onset of later-life health performances including MCI and dementia. Concurrently, mental and physical health could also confound the relationships between various social network characteristics and cognitive outcomes. We have separately examined adding physical performance tests, and chronic diseases and conditions into multivariable-adjusted MSMs models, which routinely failed to converge. We also attempted to incorporate the depression status of the respondents into our models but concerned about the validity of the brief screening instrument, which consisted of merely two questions, led us to avoid incorporating depression into our final models. In sum, although we have explored the following confounding variables, including marital status, household income, life-longest occupation, physical performance, and chronic diseases and conditions, we cannot include them at this time.
Fifth, although education levels of all types of social network member and frequency of social interactions can provide supplementary information on the effects of social ties and social interactions, we failed to assess such effects due to lack of data. Future research that focuses on both social network structure and the frequency of social interactions can contribute to a better understanding of the effects of social networks on cognitive decline.
Finally, considering our relatively short follow-up period for both observation of incidence of MCI and conversion to dementia, there is an increased concern regarding the potential for reverse causation resulting from the potential for characteristics of social networks to be influenced by the MCI process at baseline. Moreover, our research is limited in representing the analyses of only one study. Thus, further replication of these results with longer observation times is left open for future studies.
Conclusions
Our study jointly estimated correlates of social network structure on the processes of cognitive decline and dementia in a large national longitudinal cohort. Our results suggest that having more members, having at least one friend, and having at least one college-educated family member within a social network are associated with decreased risks of cognitive decline and dementia. In particular, our results further demonstrated the protective effects of having college-educated social network members against incidence of dementia. We also proposed the cumulative effects of education from family clustering on cognitive decline. Considering the effects of education, race/ethnicity, and social network structure, it is imperative to pay special attention to monitoring the rising inequality of dementia incidence in less educated families. Our findings contribute to a better understanding of the risk factors implicated in the process of developing MCI and dementia, to help pave the way for novel networks-based intervention strategies.
ACKNOWLEDGMENTS
We thank Dr. Erica Diminich, Dr. Yael Deri, Dr. Douglas Hanes, and Dr. Minos Kritikos for useful discussions, and thank Amber Luo for helpful proofreading and editing. We thank the National Institute on Aging (NIH/NIA R01 AG058595) for support.
Funding for this analysis was provided by the National Institute on Aging (NIH/NIA R01 AG058595). The National Health and Aging Trends Study (NHATS) is sponsored by the National Institute on Aging (grant number NIA U01AG032947) through a cooperative agreement with the Johns Hopkins Bloomberg School of Public Health.
Authors’ disclosures available online (https://www.j-alz.com/manuscript-disclosures/20-1196r2).
SUPPLEMENTARY MATERIAL
[1] The supplementary material is available in the electronic version of this article: https://dx.doi.org/10.3233/JAD-201196.
REFERENCES
[1] | Alzheimer’s Association ((2019) ) 2019 Alzheimer’s disease facts and figures. Alzheimers Dement 15: , 321–387. |
[2] | Conde-Sala JL , Turró-Garriga O , Calvo-Perxas L , Vilalta-Franch J , Lopez-Pousa S , Garre-Olmo J ((2014) ) Three-year trajectories of caregiver burden in Alzheimer’s disease. J Alzheimers Dis 42: , 623–633. |
[3] | Perry W , Lacritz L , Roebuck-Spencer T , Silver C , Denney RL , Meyers J , McConnel CE , Pliskin N , Adler D , Alban C ((2018) ) Population health solutions for assessing cognitive impairment in geriatric patients. Innov Aging 2: , igy025. |
[4] | Baumgart M , Snyder HM , Carrillo MC , Fazio S , Kim H , Johns H ((2015) ) Summary of the evidence on modifiable risk factors for cognitive decline and dementia: A population-based perspective. Alzheimers Dement 11: , 718–726. |
[5] | Bidart C , Charbonneau J ((2011) ) How to generate personal networks: Issues and tools for a sociological perspective. Field Methods 23: , 266–286. |
[6] | Sarkisian CA , Liu H , Gutierrez PR , Seeley DG , Cummings SR , Mangione CM ((2000) ) Modifiable risk factors predict functional decline among older women: A prospectively validated clinical prediction tool. The Study of Osteoporotic Fractures Research Group. J Am Geriatr Soc 48: , 170–178. |
[7] | Clare L , Wu Y-T , Teale JC , MacLeod C , Matthews F , Brayne C , Woods B , Team C-WS ((2017) ) Potentially modifiable lifestyle factors, cognitive reserve, and cognitive function in later life: A cross-sectional study. PLoS Med 14: , e1002259. |
[8] | Stoykova R , Matharan F , Dartigues JF , Amieva H ((2011) ) Impact of social network on cognitive performances and age-related cognitive decline across a 20-year follow-up. Int Psychogeriatr 23: , 1405–1412. |
[9] | Beland F , Zunzunegui MV , Alvarado B , Otero A , Del Ser T ((2005) ) Trajectories of cognitive decline and social relations. J Gerontol B Psychol Sci Soc Sci 60: , 320–330. |
[10] | Penninkilampi R , Casey AN , Singh MF , Brodaty H ((2018) ) The association between social engagement, loneliness, and risk of dementia: A systematic review and meta-analysis. J Alzheimers Dis 66: , 1619–1633. |
[11] | Cohen S , Wills TA ((1985) ) Stress, social support, and the buffering hypothesis. Psychol Bull 98: , 310–357. |
[12] | Seeman TE , Berkman LF , Blazer D , Rowe JW ((1994) ) Social ties and support and neuroendocrine function: The MacArthur studies of successful aging. Ann Behav Med 16: , 95–106. |
[13] | Marmot MG , Bosma H , Hemingway H , Brunner E , Stansfeld S ((1997) ) Contribution of job control and other risk factors to social variations in coronary heart disease incidence. Lancet 350: , 235–239. |
[14] | Pillai JA , Verghese J ((2009) ) Social networks and their role in preventing dementia. Indian J Psychiatry 51: , S22–S28. |
[15] | Griffiths F , Cave J , Boardman F , Ren J , Pawlikowska T , Ball R , Clarke A , Cohen A ((2012) ) Social networks–The future for health care delivery. Soc Sci Med 75: , 2233–2241. |
[16] | Glanz K , Rimer BK , Viswanath K ((2008) ) Health behavior and health education: Theory, research, and practice, John Wiley & Sons. |
[17] | Sörman DE , Rönnlund M , Sundström A , Adolfsson R , Nilsson L-G ((2015) ) Social relationships and risk of dementia: A population-based study. Int Psychogeriatr 27: , 1391–1399. |
[18] | Lara E , Martín-María N , De la Torre-Luque A , Koyanagi A , Vancampfort D , Izquierdo A , Miret M ((2019) ) Does loneliness contribute to mild cognitive impairment and dementia? A systematic review and meta-analysis of longitudinal studies. Ageing Res Rev 52: , 7–16. |
[19] | Windsor TD , Gerstorf D , Pearson E , Ryan LH , Anstey KJ ((2014) ) Positive and negative social exchanges and cognitive aging in young-old adults: Differential associations across family, friend, and spouse domains. Psychol Aging 29: , 28–43. |
[20] | Nguyen AW , Chatters LM , Taylor RJ , Mouzon DM ((2016) ) Social support from family and friends and subjective well-being of older African Americans. J Happiness Stud 17: , 959–979. |
[21] | Dunbar RI ((2018) ) The anatomy of friendship. Trends Cogn Sci 22: , 32–51. |
[22] | Ma HK ((2017) ) The development of altruism with special reference to human relationships: A 10-stage theory. Front Public Health 5: , 271. |
[23] | Lang FR ((2001) ) Regulation of social relationships in later adulthood. J Gerontol B Psychol Sci Soc Sci 56: , 321–326. |
[24] | Demir M , Jaafar J , Bilyk N , Mohd Ariff MR ((2012) ) Social skills, friendship and happiness: A cross-cultural investigation. J Soc Psychol 152: , 379–385. |
[25] | Ali T , Nilsson CJ , Weuve J , Rajan KB , de Leon CFM ((2018) ) Effects of social network diversity on mortality, cognition and physical function in the elderly: A longitudinal analysis of the Chicago Health and Aging Project (CHAP). J Epidemiol Community Health 72: , 990–996. |
[26] | Commenges D ((1999) ) Multi-state models in epidemiology. Lifetime Data Anal 5: , 315–327. |
[27] | Robitaille A , van den Hout A , Machado RJ , Bennett DA , Čukić I , Deary IJ , Hofer SM , Hoogendijk EO , Huisman M , Johansson B ((2018) ) Transitions across cognitive states and death among older adults in relation to education: A multistate survival model using data from six longitudinal studies. Alzheimers Dement 14: , 462–472. |
[28] | Marioni RE , van den Hout A , Valenzuela MJ , Brayne C , Matthews FE ((2012) ) Active cognitive lifestyle associates with cognitive recovery and a reduced risk of cognitive decline. J Alzheimers Dis 28: , 223–230. |
[29] | Montaquila J , Freedman VA , Edwards B , Kasper JD (2012) National Health and Aging Trends Study Round 1 Sample Design and Selection.NHATS TechnicalPaper 1. Baltimore: Johns Hopkins University School of Public Health. |
[30] | Morris JC ((2012) ) Revised criteria for mild cognitive impairment may compromise the diagnosis of Alzheimer disease dementia. Arch Neurol 69: , 700–708. |
[31] | Kasper JD , Freedman VA , Spillman BC (2013) Classification of persons by dementia status in the National Health and Aging Trends Study. NHATS Technical Paper 5. Baltimore: Johns Hopkins University School of Public Health. |
[32] | Hendry K , Green C , McShane R , Noel-Storr AH , Stott DJ , Anwer S , Sutton AJ , Burton JK , Quinn TJ ((2019) ) AD-8 for detection of dementia across a variety of healthcare settings. Cochrane Database Syst Rev 3: , CD011121. |
[33] | Petersen RC , Morris JC ((2005) ) Mild cognitive impairment as a clinical entity and treatment target. Arch Neurol 62: , 1160–1163. |
[34] | Laumann EO ((1966) ) Prestige and association in an urban community: An analysis of an urban stratification system, Bobbs-Merrill Company. |
[35] | Marin A , Hampton KN ((2007) ) Simplifying the personal network name generator: Alternatives to traditional multiple and single name generators. Field Methods 19: , 163–193. |
[36] | Mehta KM , Yeo GW ((2017) ) Systematic review of dementia prevalence and incidence in United States race/ethnic populations. Alzheimers Dement 13: , 72–83. |
[37] | Kryscio R , Schmitt F , Salazar J , Mendiondo M , Markesbery W ((2006) ) Risk factors for transitions from normal to mild cognitive impairment and dementia. Neurology 66: , 828–832. |
[38] | Jackson CH ((2011) ) Multi-state models for panel data: The msm package for R. J Stat Softw 38: , 1–29. |
[39] | Berkman LF , Syme SL ((1979) ) Social networks, host resistance, and mortality: A nine-year follow-up study of Alameda County residents. Am J Epidemiol 109: , 186–204. |
[40] | Bennett DA , Schneider JA , Tang Y , Arnold SE , Wilson RS ((2006) ) The effect of social networks on the relation between Alzheimer’s disease pathology and level of cognitive function in old people: A longitudinal cohort study. Lancet Neurol 5: , 406–412. |
[41] | Crooks VC , Lubben J , Petitti DB , Little D , Chiu V ((2008) ) Social network, cognitive function, and dementia incidence among elderly women. Am J Public Health 98: , 1221–1227. |
[42] | Berkman LF ((2001) ) Social integration, social networks, and health. In International Encyclopedia of the Social & ehavioral Sciences, Smelser NJ, Baltes PB, eds. Pergamon, pp. 14327–14332. |
[43] | Berkman LF , Glass T , Brissette I , Seeman TE ((2000) ) From social integration to health: Durkheim in the new millennium. Soc Sci Med 51: , 843–857. |
[44] | Becofsky KM , Shook RP , Sui X , Wilcox S , Lavie CJ , Blair SN ((2015) ) Influence of the source of social support and size of social network on all-cause mortality. Mayo Clin Proc 90: , 895–902. |
[45] | Li T , Zhang Y ((2015) ) Social network types and the health of older adults: Exploring reciprocal associations. Soc Sci Med 130: , 59–68. |
[46] | Thomas PA ((2011) ) Trajectories of social engagement and limitations in late life. J Health Soc Behav 52: , 430–443. |
[47] | Aartsen MJ , Van Tilburg T , Smits CH , Knipscheer KC ((2004) ) A longitudinal study of the impact of physical and cognitive decline on the personal network in old age. J Soc Pers Relat 21: , 249–266. |
[48] | Seeman TE , Lusignolo TM , Albert M , Berkman L ((2001) ) Social relationships, social support, and patterns of cognitive aging in healthy, high-functioning older adults: MacArthur studies of successful aging. Health Psychol 20: , 243–255. |
[49] | Stern Y ((2013) ) Cognitive reserve: Theory and applications, Psychology Press. |
[50] | WHO (2015) World report on ageing and health, World Health Organization. |
[51] | Wiles JL , Leibing A , Guberman N , Reeve J , Allen RE ((2012) ) The meaning of “aging in place” to older people. Gerontologist 52: , 357–366. |
[52] | Vanleerberghe P , De Witte N , Claes C , Schalock RL , Verté D ((2017) ) The quality of life of older people aging in place: A literature review. Qual Life Res 26: , 2899–2907. |
[53] | Hinterberger M , Fischer P , Zehetmayer S ((2016) ) Incidence of dementia over three decades in the Framingham Heart Study. N Engl J Med 375: , 93. |
[54] | Derby CA , Katz MJ , Lipton RB , Hall CB ((2017) ) Trends in dementia incidence in a birth cohort analysis of the Einstein Aging Study. JAMA Neurol 74: , 1345–1351. |
[55] | Clouston SA , Smith DM , Mukherjee S , Zhang Y , Hou W , Link BG , Richards M ((2019) ) Education and cognitive decline: An integrative analysis of global longitudinal studies of cognitive aging. J Gerontol B Psychol Sci Soc Sci 75: , e151–e160. |
[56] | Clouston SA , Zhang Y , Smith DM ((2019) ) Pattern recognition to identify stroke in the cognitive profile: Secondary analyses of a prospective cohort study. Cerebrovasc Dis 9: , 114–122. |
[57] | Link BG , Phelan J ((1995) ) Social conditions as fundamental causes of disease. J Health Soc Behav Spec No: , 80–94. |
[58] | Clouston SA , Link BG ((2021) ) A retrospective on fundamental cause theory: State of the literature, and goals for the future. Annu Rev Sociol 47: , https://doi.org/10.1146/annurev-soc-090320-094912. |