Do Cardiovascular Risk Factors and Cardiovascular Disease Explain Sex Differences in Cognitive Functioning in Old Age?
Abstract
Background:
Sex differences in cognitive functioning in old age are known to exist yet are still poorly understood.
Objective:
This study examines to what extent differences in cardiovascular risk factors and cardiovascular disease between men and women explain sex differences in cognitive functioning.
Methods:
Data from 2,724 older adults from the Longitudinal Aging Study Amsterdam were used. Information processing speed and episodic memory, measured three times during six years of follow-up, served as outcomes. The mediating role of cardiovascular risk factors and cardiovascular disease was examined in single and multiple mediator models. Determinant-mediator effects were estimated using linear or logistic regression, and determinant-outcome and mediator-outcome effects were estimated using linear mixed models. Indirect effects were estimated using the product-of-coefficients estimator.
Results:
Women scored 1.58 points higher on information processing speed and 1.53 points higher on episodic memory. Several cardiovascular risk factors had small mediating effects. The sex difference in information processing speed was mediated by smoking, depressive symptoms, obesity, and systolic blood pressure. The sex difference in episodic memory was mediated by smoking, physical activity, and depressive symptoms. Effects of smoking, LDL cholesterol, and diabetes mellitus on information processing speed differed between men and women.
Conclusion:
Differences in cardiovascular risk factors between women and men partially explained why women had better cognitive functioning. A healthy cardiovascular lifestyle seems beneficial for cognition and sex-specific strategies may be important to preserve cognitive functioning at older age.
INTRODUCTION
Cognitive functioning differs between older men and women. On average, men have better visuo-spatial abilities and women have a better episodic memory (e.g., [1–3]). Sex differences in other domains of cognitive functioning have also been reported, but these results are inconsistent [1].
Several mechanisms suggested to partially explain sex differences in cognitive functioning have been examined, such as differences in education, income, and occupational complexity [4]; differences in brain structure and function [5, 6]; and differences in hormones and their influence on the brain [7]. However, none of the proposed mechanisms sufficiently explained sex differences in cognitive functioning and other mechanisms may also play an important role.
One other potentially important mechanism in-volves cardiovascular risk factors (CVRFs) and cardiovascular disease (CVD) [8]. First, the prevalence of CVRFs and CVD differs between older men and women. For example, the prevalence of smoking and diabetes is higher in men and women have lower levels of physical activity [9–12]. In addition, the prevalence of obstructive coronary artery disease is higher in men and the prevalence of non-obstructive coronary artery disease is higher in women, which is likely due to sex-specific pathophysiological mechanisms [13, 14]. Second, patients with CVD are known to have an increased risk of cognitive impairment [15]. This could be due to (sub)clinical strokes, cerebral small vessel disease, or brain hypoperfusion, or due to overlapping risk factors between CVD and cognitive impairment since CVRFs, such as diabetes mellitus, hypertension, and depression, have also been associated with changes in brain structure and cognitive impairment [8, 16–18]. Third, the strength of the association between CVRFs and CVD and cognitive functioning may differ between men and women [8, 9, 19]. For example, research suggests that the effect of diabetes mellitus on cognitive functioning is stronger in women and that myocardial infarction is associated with impaired cognitive functioning in men only, possibly due to differences in sex hormones between men and women. However, few studies have empirically investigated whether differences in CVRFs and CVD between older men and women contribute to sex differences in cognitive functioning at older age.
Therefore, using 6-year longitudinal data from a large population-based cohort of older adults in the Netherlands, we assessed to what extent the effect of sex on cognitive functioning is mediated by CVRFs and CVD. Following guidelines from the causal mediation framework, we examined whether exposure-mediator interaction was present [20, 21]. Our findings can provide insight into how preventive and curative efforts may be tailored to men and women specifically [8, 22].
MATERIALS AND METHODS
Participants
Data for this study were derived from the Longitudinal Aging Study Amsterdam (LASA), an ongoing nationally-representative prospective cohort study on physical, emotional, cognitive, and social functioning of Dutch adults originally aged between 55 and 85 years [23, 24]. LASA was initiated by the Dutch Ministry of Health, Welfare and Sports in 1991. The sampling frame consisted of the municipal registries of three culturally distinct geographical areas. N = 3,107 respondents were included at baseline in 1992-1993. New measurements were conducted about every three years. In 2002-2003 and 2012-2013, a second and third cohort of N = 1,002 and N = 1,023 respectively, aged between 55 and 65 years, were included from the same sampling frame as in 1992-1993. All participants signed informed consent. LASA was approved by the Medical Ethics Committee of the VU University Medical Center.
In the current study, we pooled data from three subsequent measurements from each cohort (first cohort: 1995-1996, 1998-1999, and 2001-2002 (we could not use the 1992-1993 measurement because blood pressure measurements were not available); second cohort: 2002-2003, 2005-2006, and 2008-2009; third cohort: 2012-2013, 2015-2016, and 2018-2019). The total sample size was 4,570. Respondents with at most one measurement of cognitive functioning (N = 1,795) were excluded from the analyses, as were respondents with missing data on any of the mediator variables except cholesterol levels (N = 50) or confounding variables (N = 1). Missing data were mainly due to drop out (N = 733, 39.8%), not willing to participate in the medical interview, which contains most of the variables used in this study (N = 632, 34.3%), or a combination of these (N = 266, 14.4%). The remainder of missing data was due to incidental missingness (N = 218, 11.8%). This resulted in a final sample of 2,724 respondents.
Cognitive functioning
We used two measures of cognitive functioning: information processing speed and episodic memory. We chose these measures based upon availability and because processing speed has been proven to be more sensitive to age-related changes than, for example, global measures of cognition such as the Mini-Mental State Examination (MMSE), and memory problems are one of the most common complaints at older age that negatively affect daily functioning [25, 26]. Information processing speed was assessed with an adjusted version of the Coding Task, which is a letter substitution task [27]. Two rows of characters were shown and each character in the upper row belonged to a character in the bottom row. Subsequently, two additional rows were shown: one with characters and one without characters. The respondent had to complete as many character combinations as possible in three trials of one minute. While in the original Coding Task respondents were asked to write down the corresponding character, LASA respondents were asked to name them because a pilot study showed that some older adults had difficulty in writing the letters and it was sometimes difficult to read the handwriting of the respondents [28]. The mean score of the three trials was used in the analyses (range 1–51.3). Higher scores indicate faster processing speed.
Episodic memory was assessed with an adapted version of the Dutch Auditory Verbal Learning Test [29]. This test consists of three trials and a delayed recall. In the three trials, a list of 15 words was read out loud by the interviewer and the respondent directly recalls as many words as possible. After a distraction period of 20 min, the respondent was asked to name the words he or she remembered from the three trials (‘delayed recall’). The number of correctly remembered words from the delayed recall was used as a measure for episodic memory (range 0–15). Higher scores indicate better episodic memory. Information processing speed and episodic memory were measured at every measurement.
MMSE scores at the first measurement were not used as an outcome measure, but as a descriptive variable to give an impression of global cognitive functioning of the sample [30]. The MMSE ranges from 0–30 and higher scores indicate better cognitive functioning. A score < 24 is an indicator of cognitive impairment.
Sex
Sex, referring to biological sex, was coded as male (0) or female (1).
Mediator variables
We included smoking, physical activity, depressive symptoms, obesity, waist-hip ratio (WHR), systolic blood pressure, diastolic blood pressure, total cholesterol, high-density lipoprotein (HDL) cholesterol, low-density lipoprotein (LDL) cholesterol, diabetes mellitus, and a history of CVD as mediator variables.
Self-reported smoking was categorized as currently smoking or stopped smoking < 10 years ago (1) versus never smoking or stopped ≥10 years ago (0), because current smoking and < 10 years of cessation have been associated with impaired cognitive functioning [31]. Physical activity was mea-sured with the LASA Physical Activity Questionnaire, which measures the frequency and duration of activities, including walking outdoors, biking, gardening, light household activities, heavy household activities, and two of the respondent’s most frequently performed sports activities [32]. Intensity-weighted total physical activity scores in metabolic equivalent of task hours/week were calculated, with higher scores indicating more physical activity [33]. Depressive symptoms were measured with the Center for Epidemiologic Studies Depression Scale (range 0–60, higher scores indicate more depressive symptoms) [34]. Depressive symptoms were included because they have consistently been associated with an increased risk of CVD and the acknowledgement of depression as a potentially modifiable CVRF is growing [35]. Furthermore, depressive symptoms are associated with impaired cognitive functioning [36] and the prevalence differs between men and women [35]. Obesity (no/yes) was defined as a body mass index above 30 kg/m2 and was calculated from measured height and weight, or self-reported height and weight when measurement was not possible. Waist and hip circumference were measured during the interview to calculate the WHR (waist circumference / hip circumference). Systolic and diastolic blood pressure were measured electronically in mmHg (model HEM-706; OMRON) at the upper left arm with participants in a seated position after 5 min of rest. The number of retests differed between measurement moments (range 0–4). When retests were done, there was significant time between the measurements. The mean scores of the blood pressure measurements were used. Total, HDL, and LDL cholesterol levels were obtained from morning blood samples in mmol/L and were determined in one laboratory. Total cholesterol levels were determined in the first and second cohort, HDL and LDL cholesterol levels were determined in the first cohort only. History of diabetes mellitus (no/yes) was determined with a disease ascertainment algorithm combining data on self-report, general practitioner’s diagnoses and medication [37]. History of CVD (no/yes) was determined by combining the outputs of disease-specific ascertainment algorithms for coronary artery disease, cerebrovascular accident, peripheral artery disease, and heart failure [38].
All mediators were included as measured at the first measurement (i.e., 1995-1996, 2002-2003, or 2012-2013). When data on that measurement were missing, data from the other two measurements were used to impute the missing data, as we assumed the sex differences in risk factor status to be relatively stable over a six-year follow-up.
Confounding variables
Age at the first measurement, cohort (first, second, or third), education, use of cholesterol lowering medication, and use of blood pressure lowering medication were included as putative confounding variables. Education was based on the highest degree earned, converted into the nominal number of years of education needed for that degree (range 5–18 years). Medication use was based on the containers of all medication used in the two weeks before the interview, as shown by the respondent to the interviewer. It was categorized according to the ATC Classification System, with ATC codes starting with C10 indicating use of cholesterol lowering medication (no/yes) and ATC codes starting with C02, C03, C07, C08, and C09 indicating use of blood pressure lowering medication (no/yes).
Statistical analysis
Data were analyzed in STATA, version 14 [39]. Sex differences in socio-demographic characteristics, mediator variables, MMSE scores and both measures of cognitive functioning at the first measurement were assessed using independent samples t-tests (for normally distributed variables or log transformed variables) or Chi squared tests (categorical variables).
Subsequently, the following analysis procedure was executed for the two cognitive outcomes separately. First, sex differences in the level of cognitive functioning across all measurements (c-path in Fig. 1) were estimated with linear mixed models. Second, single mediator models (separate models with one mediator at a time) were estimated. The effects of sex on the mediator variables (a-path in Fig. 1) were estimated with linear regression for continuous mediator variables and logistic regression for dichotomous mediator variables. To ensure that the scale of the mediator variable was the same across the a- and b-paths, we converted a-paths estimated with logistic regression from the log-odds scale to the risk-difference scale using the following formula [40, 41]:
Fig. 1
Single mediator model.
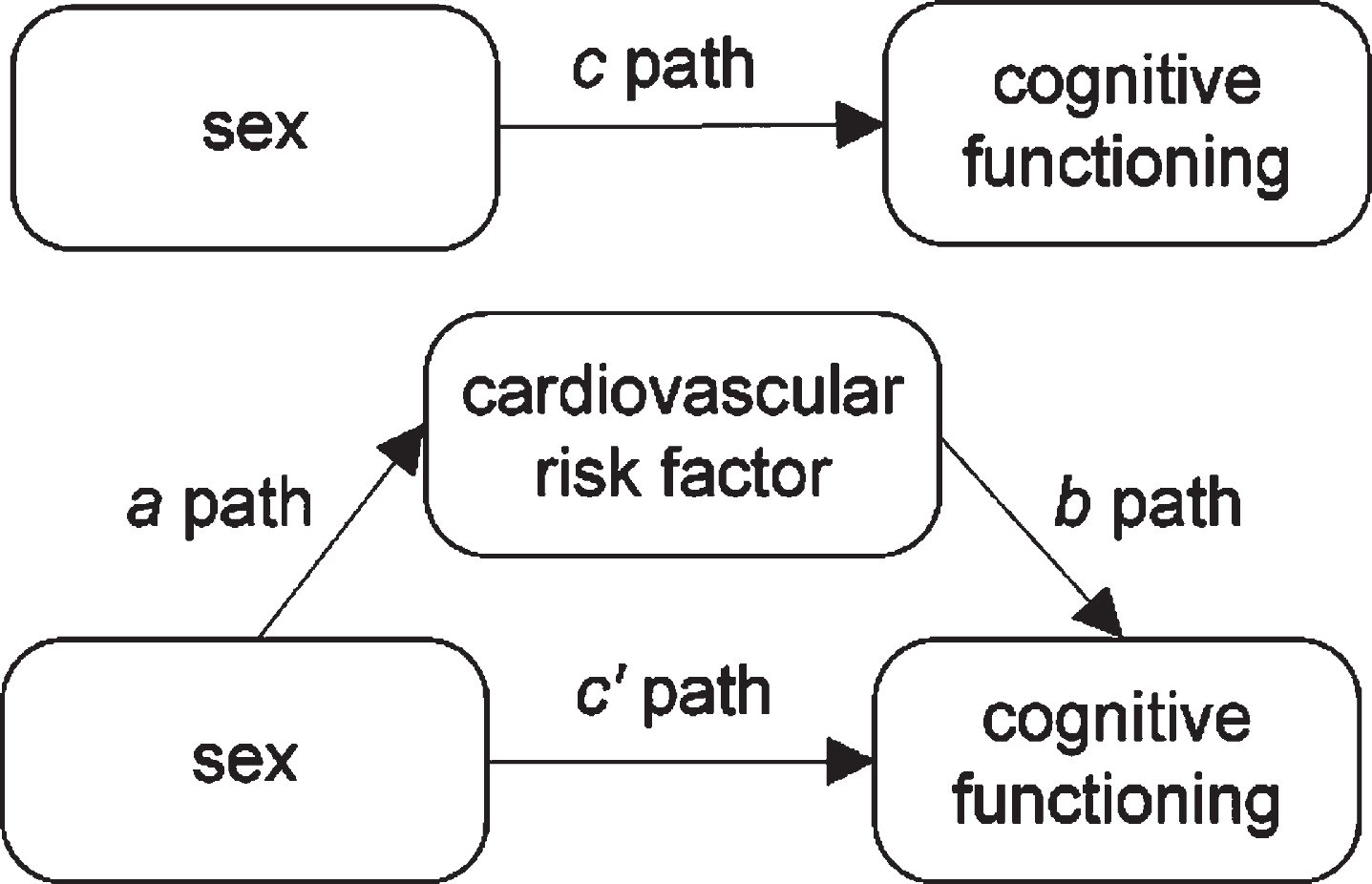
Betaa is the B of the a-path as calculated with logistic regression analysis, Im is the intercept in the logistic regression model, a pathriskdifference is the a-path on the risk difference scale.
The effects of the mediator variables on cognitive functioning (b-path in Fig. 1) and the direct effect of sex on cognitive functioning after accounting for the mediator (c’-path in Fig. 1) were estimated with linear mixed models. Third, indirect effect estimates were calculated by multiplying the a-path and b-path. The 95% percentile confidence intervals for the indirect effect estimates were calculated based on 1000 bootstrap draws.
Fourth, parallel multiple mediator models were estimated, in which all mediators are simultaneously assessed in one model (Fig. 3). Consequently, the indirect effect estimate for a mediator is adjusted for the other mediators in the model. We excluded CVD because of a causal association with CVRFs [42]. In case of multicollinearity (systolic and diastolic blood pressure and WHR and obesity), we excluded the mediator with the smallest indirect effect estimate in the single mediator models, which were diastolic blood pressure and WHR. We excluded cholesterol levels from the multiple mediator models because the large proportion of missing data (35–53%) would result in a large decrease in sample size and power.
Sex-by-time interactions and mediator-by-time interactions were included in all models to determine whether sex differences in cognitive functioning and whether the effect estimates of mediators on cognitive functioning remained stable over time, respectively. Following guidelines from the causal mediation framework, we additionally tested exposure-medi-ator interaction in all models to determine if the effect estimates for mediator variables on cognition differed between men and women [21]. In case of a significant exposure-mediator interaction, group-mean centering was used to calculate the effects for both sexes separately [20]. All models were adjusted for cohort, age, and education. Models that included cholesterol levels were additionally adjusted for the use of cholesterol lowering medication and models that included blood pressure were additionally adjusted for the use of blood pressure lowering medication. All continuous confounders were grand-mean centered.
Given the lower power for detecting interaction terms, these were considered statistically significant at p < 0.10, other effect estimates were considered statistically significant at p < 0.05 [43]. Although our analyses comprised many tests, we did not adjust for multiple comparisons as this may decrease type-1-error rates, but simultaneously increases type-2-error rates [44].
Planned sensitivity analyses
Two sensitivity analyses were done. First, as cholesterol was excluded from the multiple mediator models because of a large amount of missing data, we included LDL cholesterol as a sensitivity analysis and compared the results to those of the models without LDL cholesterol. Second, as studies showed that age moderates the effects of CVRFs on cognitive functioning, we stratified for age 55–75 and 75 and over and compared the indirect effect estimates [45, 46].
RESULTS
Attrition and sample description
The proportion of men and women did not differ between included and excluded respondents. Excluded respondents scored lower on information processing speed, episodic memory and MMSE than included respondents. Excluded respondents also had an unhealthier cardiovascular risk profile than included respondents.
The final study sample consisted of 2,724 respondents, of whom 47.2% were men. On average, respondents were 65.8 years old (±8.2, range 55–88) and had 10.2 years (±3.5) of education. The median MMSE score was 28 (IQR 2.0, range 13–30) and 6.3% of the respondents had a score < 24. Age and MMSE scores did not differ between men and women.
Sex differences in cognitive functioning
Women had higher information processing speed and episodic memory scores than men (Fig. 2). At baseline, adjusted for cohort, age, and education, women scored on average 1.58 points (95% C.I.: 1.07–2.09) higher on information processing speed than men (total effect of sex on information processing speed, Cohen’s D = 0.22) and this sex difference increased with 0.07 points (95% C.I.: 0.02–0.12) per year. At baseline, women scored on average 1.53 points (95% C.I.: 1.36–1.71) higher on episodic memory (total effect of sex on episodic memory, Cohen’s D = 0.53), which remained stable over time.
Fig. 2
Sex differences in cognitive functioning over time. The sex difference in information processing speed was 1.58 (95% C.I.: 1.07–2.09, p < 0.01), the sex difference in episodic memory was 1.53 (95% C.I.: 1.36–1.71, p < 0.01). For models with blood pressure, total cholesterol, HDL cholesterol or LDL cholesterol, total effects of sex on information processing speed were 1.57 (95% C.I.: 1.06–2.07, p < 0.01), 1.32 (95% C.I.: 0.67–1.97, p < 0.01), 0.67 (95% C.I.: –0.19–1.53, p = 0.13) and 0.66 (95% C.I.: –0.19–1.53, p = 0.13), respectively, and the total effects of sex on episodic memory were 1.53 (95% C.I.: 1.35–1.70, p < 0.01), 1.45 (95% C.I.: 1.22–1.67, p < 0.01), 1.55 (95% C.I.: 1.24–1.85, p < 0.01), and 1.55 (95% C.I.: 1.24–1.85, p < 0.01), respectively.
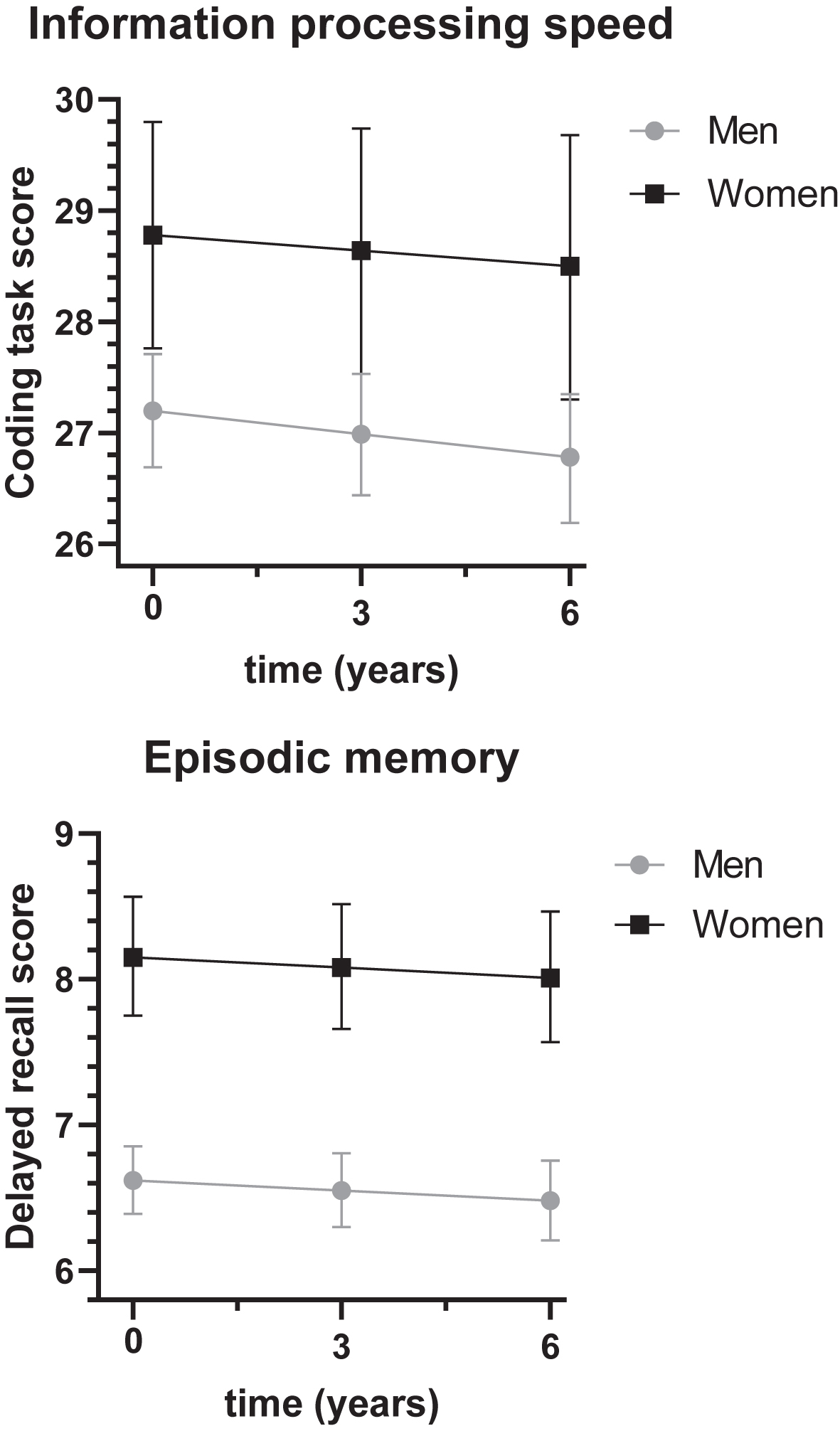
Sex differences in CVRFs and CVD
Men and women had different cardiovascular risk profiles (Table 1 and a-paths in Tables 2 and 3). Women attained less years of education, had a higher prevalence of obesity, a higher total and LDL cholesterol, and more depressive symptoms than men. Men had higher systolic and diastolic blood pressure, a lower HDL cholesterol, a higher WHR, and a higher prevalence of smoking and CVD than women. In addition, men were less physically active, which we found was mainly due to men spending less time on household tasks [47]. Age and the prevalence of diabetes mellitus did not differ significantly between sexes.
Table 1
Characteristics of respondents at the first measurement
Men | Women | |||||
N | N = 1,287 | N = 1,437 | p | |||
(47.2%) | (52.8%) | |||||
Age mean±SD | 2724 | 65.7 | 8.2 | 65.9 | 8.3 | 0.53 |
Years of education mean±SD | 2724 | 10.9 | 3.6 | 9.6 | 3.4 | < 0.01 |
Smoking % n | 2724 | 37.9 | 488 | 24.9 | 358 | < 0.01 |
Physical activity (ln MET h/week) mean±SD | 2724 | 3.7 | 0.9 | 4.1 | 0.7 | < 0.01 |
Depressive symptoms median±IQR | 2724 | 4.0 | 7.0 | 7.0 | 10.0 | < 0.011 |
Obesity % n | 2724 | 17.2 | 222 | 26.4 | 380 | < 0.01 |
Waist-hip ratio mean±SD | 2724 | 1.0 | 0.1 | 0.9 | 0.1 | < 0.01 |
Systolic blood pressure (mmHg) mean±SD | 2724 | 146.5 | 22.0 | 142.9 | 24.5 | < 0.01 |
Diastolic blood pressure (mmHg) mean±SD | 2724 | 84.9 | 11.6 | 82.7 | 11.5 | < 0.01 |
Total cholesterol (mmol/l) mean±SD | 1626 | 5.5 | 0.9 | 6.0 | 1.0 | < 0.01 |
HDL cholesterol (mmol/l) mean±SD | 954 | 1.2 | 0.4 | 1.4 | 0.4 | < 0.01 |
LDL cholesterol (mmol/l) mean±SD | 948 | 3.5 | 0.9 | 3.8 | 1.0 | < 0.01 |
Diabetes mellitus % n | 2724 | 7.9 | 102 | 7.2 | 104 | 0.27 |
History of CVD % n | 2724 | 29.1 | 374 | 19.9 | 286 | < 0.01 |
MMSE median±IQR | 2709 | 28.0 | 2.0 | 28.0 | 2.0 | 0.081 |
Information processing speed mean±SD | 2512 | 27.1 | 6.6 | 28.1 | 7.3 | < 0.01 |
Episodic memory mean±SD | 2501 | 5.8 | 2.7 | 7.2 | 2.8 | < 0.01 |
In, natural logarithm; HDL, high-density lipoprotein; LDL, low-density lipoprotein; CVD, cardiovascular disease; MMSE, Mini-Mental State Examination. 1Independent-Samples Mann-Whitney U Test.
Table 2
Single mediator models for information processing speed
Mediator | Direct effect | a-path | b-path | Indirect effect | |||||||
B | (SE) | p | B | (SE) | p | B | (SE) | p | B | (95% CI) | |
Smoking | 1.49 | (0.26) | < 0.01 | –0.15 | (0.02) | < 0.01 | –0.61 | (0.23) | < 0.01 | 0.09 | (0.02 –0.18) |
Physical activity | 1.52 | (0.27) | < 0.01 | 0.39 | (0.03) | < 0.01 | 0.16 | (0.16) | 0.33 | 0.06 | (–0.08 –0.18) |
Depressive symptoms | 1.87 | (0.26) | < 0.01 | 2.60 | (0.29) | < 0.01 | –0.11 | (0.01) | < 0.01 | –0.29 | (–0.39 ––0.20) |
Obesity | 1.64 | (0.26) | < 0.01 | 0.07 | (0.02) | < 0.01 | –0.83 | (0.26) | < 0.01 | –0.06 | (–0.10 ––0.02) |
Waist-hip ratio | 1.10 | (0.30) | < 0.01 | –0.10 | (0.00) | < 0.01 | –4.77 | (1.45) | < 0.01 | 0.48 | (0.19 –0.82) |
Systolic blood pressure | 1.64 | (0.26) | < 0.01 | –4.31 | (0.86) | < 0.01 | 0.02 | (0.01) | < 0.01 | –0.08 | (–0.15 ––0.03) |
Diastolic blood pressure | 1.60 | (0.26) | < 0.01 | –2.17 | (0.45) | < 0.01 | 0.01 | (0.01) | 0.17 | –0.03 | (–0.08 –0.01) |
Total cholesterol | 1.10 | (0.34) | < 0.01 | 0.52 | (0.05) | < 0.01 | 0.42 | (0.14) | < 0.01 | 0.22 | (0.06 –0.38) |
HDL cholesterol | 0.41 | (0.45) | 0.36 | 0.23 | (0.03) | < 0.01 | 1.14 | (0.44) | 0.01 | 0.26 | (0.05 –0.50) |
LDL cholesterol | 0.27 | (0.06) | < 0.01 | ||||||||
Men | 0.58 | (0.45) | 0.19 | –0.31 | (0.33) | 0.35 | –0.08 | (–0.26 –0.09) | |||
Women | –0.78 | (0.45) | 0.08 | 0.33 | (0.29) | 0.26 | 0.09 | (–0.08 –0.24) | |||
Diabetes mellitus | –0.01 | (0.01) | 0.22 | ||||||||
Men | 1.60 | (0.25) | < 0.01 | 0.12 | (0.63) | 0.85 | –0.00 | (–0.02 –0.01) | |||
Women | 1.61 | (0.25) | < 0.01 | –1.47 | (0.62) | 0.02 | 0.01 | (–0.01 –0.05) | |||
History of CVD | 1.53 | (0.26) | < 0.01 | –0.11 | (0.02) | < 0.01 | –0.31 | (0.31) | 0.31 | 0.04 | (–0.03 –0.11) |
Total effect of sex: 1.58 (95% C.I. 1.07–2.09). The a-paths represent the difference between men and women, with men as the reference category. The single mediator models also include a sex-by-time interaction and mediator-by-time interactions for physical activity, systolic blood pressure, diabetes mellitus, LDL cholesterol, and history of CVD.
Table 3
Single mediator models for episodic memory
Mediator | Direct effect | a-path | b-path | Indirect effect | ||||
B (SE) | p | B (SE) | p | B (SE) | p | B | (95% CI) | |
Smoking | 1.51 (0.09) | < 0.01 | –0.15 (0.02) | < 0.01 | –0.18 (0.10) | 0.06 | 0.03 | (0.00 – 0.06) |
Physical activity | 1.46 (0.09) | < 0.01 | 0.39 (0.03) | < 0.01 | 0.19 (0.05) | < 0.01 | 0.07 | (0.03 – 0.11) |
Depressive symptoms | 1.58 (0.09) | < 0.01 | 2.60 (0.29) | < 0.01 | –0.02 (0.01) | < 0.01 | –0.05 | (–0.08 – –0.02) |
Obesity | 1.55 (0.09) | < 0.01 | 0.07 (0.02) | < 0.01 | –0.28 (0.11) | 0.01 | –0.02 | (–0.04 – –0.00) |
Waist-hip ratio | 1.34 (0.11) | < 0.01 | –0.10 (0.00) | < 0.01 | –0.43 (0.86) | 0.61 | 0.04 | (–0.12 – 0.21) |
Systolic blood pressure | 1.52 (0.09) | < 0.01 | –4.31 (0.86) | < 0.01 | 0.00 (0.00) | 0.19 | –0.02 | (–0.04 – 0.01) |
Diastolic blood pressure | 1.53 (0.09) | < 0.01 | –2.17 (0.45) | < 0.01 | 0.00 (0.00) | 0.81 | –0.00 | (–0.02 – 0.02) |
Total cholesterol | 1.44 (0.12) | < 0.01 | 0.52 (0.05) | < 0.01 | 0.01 (0.06) | 0.84 | 0.01 | (–0.06 – 0.07) |
HDL cholesterol | 1.48 (0.16) | < 0.01 | 0.23 (0.03) | < 0.01 | 0.28 (0.19) | 0.13 | 0.07 | (–0.03 – 0.16) |
LDL cholesterol | 1.55 (0.16) | < 0.01 | 0.27 (0.06) | < 0.01 | –0.02 (0.08) | 0.81 | –0.01 | (–0.06 – 0.04) |
Diabetes mellitus | 1.53 (0.09) | < 0.01 | –0.01 (0.01) | 0.22 | –0.52 (0.17) | < 0.01 | 0.00 | (–0.00 – 0.02) |
History of CVD | 1.50 (0.09) | < 0.01 | –0.11 (0.02) | < 0.01 | –0.03 (0.17) | 0.85 | 0.00 | (–0.03 – 0.04) |
Total effect of sex: 1.53 (1.36–1.71). The a-paths represent the difference between men and women, with men as the reference category. The single mediator models for waist-hip ratio, systolic blood pressure, and a history of CVD also include mediator-by-time interactions.
Cardiovascular health and cognitive functioning
Smoking, more depressive symptoms, obesity, and a higher WHR were associated with lower information processing speed (b-paths in Table 2). A higher systolic blood pressure and a higher total and HDL cholesterol were associated with higher information processing speed. Diabetes mellitus was associated with lower information processing speed in women but had no effect in men. Other cardiovascular risk factors or a history of CVD were not statistically significantly associated with information processing speed.
More depressive symptoms, obesity, and diabetes mellitus were associated with lower episodic memory scores (b-paths in Table 3). More physical activity was associated with higher episodic memory scores. Other CVRFs and a history of CVD were not statistically significantly associated with episodic memory.
Mediators of the sex difference in information processing speed
The sex difference in information processing speed was partially mediated by smoking, depressive symptoms, obesity, WHR, systolic blood pressure, total cholesterol, and HDL cholesterol (Table 2).
As the indirect effect estimates for smoking, WHR, total cholesterol, and HDL cholesterol were positive, these risk factors partially explained why women on average scored higher on information processing speed than men. For example, because women smoked less often (a-path: –0.15) and smoking was associated with a lower information processing speed (b-path: –0.61), women scored on average 0.09 (95% C.I.: 0.02–0.18) points higher on information processing speed than men. This means that 0.09 points of the 1.58-points difference in information processing speed between men and women were attributable to the fact that women smoked less often. In a similar manner, 0.48, 0.22, and 0.26 points of the 1.58-points sex difference in information processing speed were attributable to a lower WHR, a higher total cholesterol, and a higher HDL cholesterol, respectively, in women.
We found significant exposure-by-mediator interactions for LDL cholesterol and diabetes mellitus; however, the indirect effect estimates were statistically significant in neither men nor women.
As the indirect effect estimates for depressive symptoms, obesity, and systolic blood pressure on information processing speed were negative, these were suppressors of the sex difference in information processing speed. For example, as women on average had more depressive symptoms (a-path: 2.60) and more depressive symptoms were associated with lower information processing speed (b-path: –0.11), women on average scored 0.29 (95% C.I.: –0.39- –0.20) points lower on information processing speed than men. The 1.58-points difference in information processing speed between men and women would have been 0.29 points larger if the average depression score of women was equal to the score of men. In a similar manner, the 1.58-points sex difference in information processing speed would have been 0.06 and 0.08 points larger if the prevalence of obesity and the average systolic blood pressure, respectively, in women were equal to those in men.
In the multiple mediator model in which mediators were mutually adjusted, depressive symptoms, obesity and systolic blood pressure remained significant suppressors of the sex difference in information processing speed (Fig. 3A). The indirect effect estimates of these mediators were comparable to the effect estimates in the single mediator models. In addition, we found a significant exposure-by-mediator interaction for smoking. Smoking explained 0.14 points of the sex difference in information processing speed, because men smoked more often than women and smoking was associated with lower information processing speed in men only. After adjustment for the mediators, women scored 1.74 (95% C.I.: 1.13–2.35) points higher on information processing speed than men.
Fig. 3
Multiple mediator models. Arrows pointing from sex towards the mediator represent a-paths, arrows pointing from the mediator towards cognitive functioning represent b-paths. The indirect effect estimates (95% C.I.) are placed above or below the mediator. Mediators in bold indicate statistically significant indirect effect estimates. c = total effect, c’=direct effect. A) model for information processing speed, also includes a sex-by-time interaction (0.05 (0.03), p = 0.05) and mediator-by-time interactions for systolic blood pressure and physical activity. B) model for episodic memory, also includes a mediator-by-time interaction for systolic blood pressure.
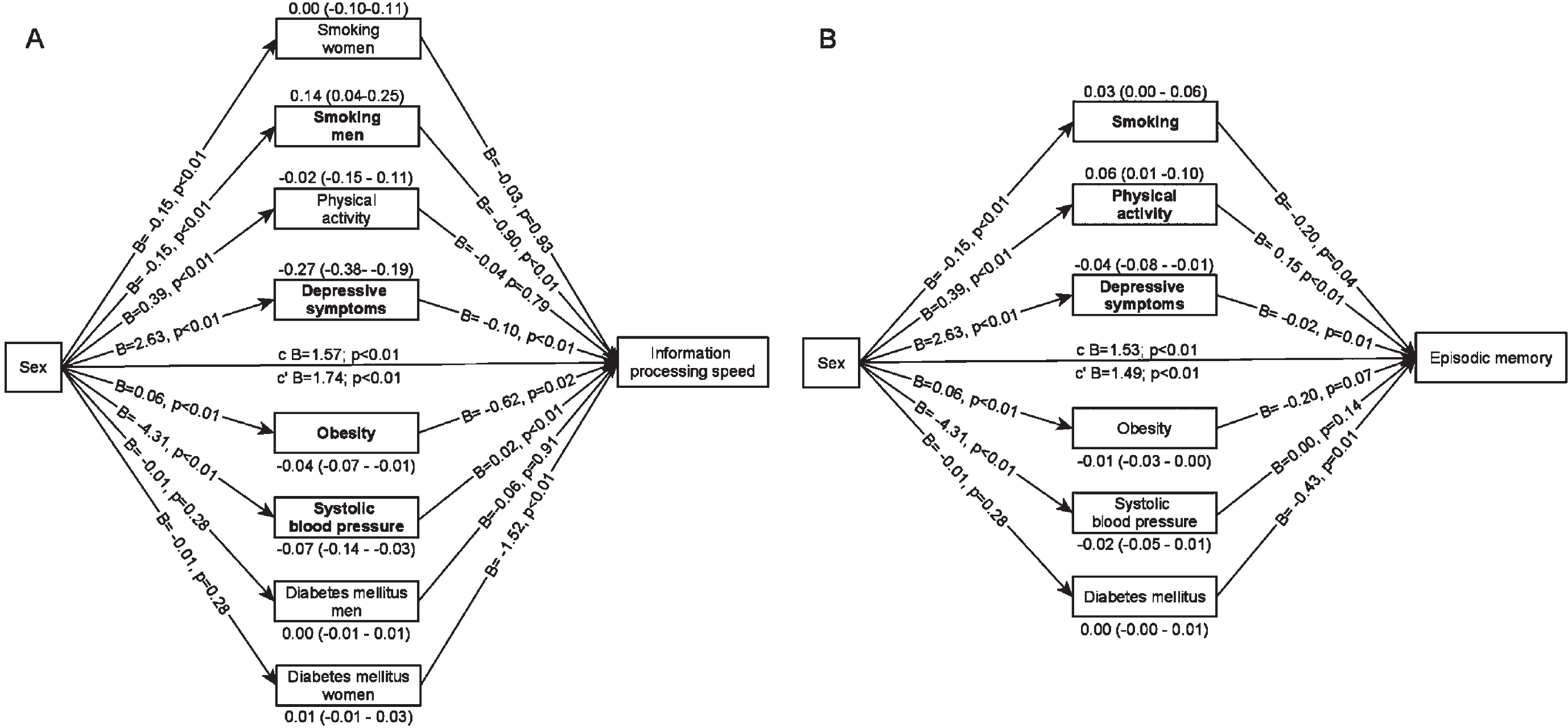
Mediators of the sex difference in episodic memory
Compared to information processing speed, we found a lower number of mediators of the sex difference in episodic memory.
In the single mediator models, less smoking and more physical activity partially explained why wo-men on average had a better episodic memory than men. So, on average 0.03 and 0.07 points of the 1.53-points sex difference in episodic memory were attributable to less smoking and more physical activity, respectively, in women. More depressive symptoms and obesity were suppressors of the sex difference in episodic memory: the 1.53-points sex difference in episodic memory would have been 0.05 and 0.02 points larger if the average depression score and prevalence of obesity in women were equal to those observed in men (Table 3).
In the multiple mediator model (Fig. 3B), less smoking and more physical activity explained why women scored higher on episodic memory and depressive symptoms were a suppressor of the sex difference. The indirect effect estimates were comparable to the indirect effect estimates in the single mediator models. After adjustment for the mediators, women scored 1.49 (95% C.I.: 1.30–1.68) points higher on episodic memory than men.
Sensitivity analyses
The multiple mediator models including LDL cho-lesterol were estimated in a sample of N = 948 (the LDL-subsample, 34.8% of the total study sample). After adjustment for the effects of other CVRFs, LDL cholesterol was no statistically significant mediator of sex differences in information processing speed nor episodic memory (Supplementary Tables 1 and 2). The CVRFs mediated more of the sex difference in information processing speed in the LDL-subsample than in the full sample because the sex difference in information processing speed was much smaller in the LDL-subsample, yet only the indirect effect estimate for depressive symptoms was statistically significant (Supplementary Table 1). For episodic memory, the magnitude of the total effect and indirect effect estimates in the LDL-subsample were comparable to those in the full sample. Only the indirect effect estimate for physical activity was statistically significant (Supplementary Table 2).
The association between CVRFs and cognitive functioning in older adults was moderated by age in previous studies and we also found differences between younger-olds (55–75 years) and older-olds (75 years and over) (Supplementary Tables 3 and 4). Information processing speed and episodic memory scores were higher in women in both age groups, but sex differences were larger in the younger-olds. Sex differences in cardiovascular risk profiles (a-paths) and effect estimates for CVRFs on cognitive functioning (b-paths) differed between age groups. The sex difference in information processing speed in young-olds was suppressed by depressive symptoms and smoking among women. In older-olds, none of the CVRFs were significant mediators. The sex difference in episodic memory in younger-olds was not mediated by CVRFs. In older-olds, the sex difference in episodic memory was partially mediated by physical activity.
DISCUSSION
The aim of this paper was to investigate if CVRFs and CVD mediate sex differences in cognitive functioning in Dutch older adults. Results showed that women had better information processing speed and episodic memory than men and that the sex difference in information processing speed further increased during the follow-up. Smoking, depressive symptoms, obesity, systolic blood pressure, WHR, total cholesterol, and HDL cholesterol explained the sex difference in information processing speed. Smoking, depressive symptoms, obesity, and physical activity explained the sex difference in episodic memory. In addition, we found an exposure-mediator interaction for smoking, LDL cholesterol, and diabetes mellitus on information processing speed, indicating that the effects of these mediators on information processing speed differed between men and women. Sex differences in cognitive functioning were thus partially mediated by CVRFs. However, the indirect effect estimates were small, suggesting that other mechanisms likely play a role as well.
Comparison to previous findings
To our knowledge, this is the first study assessing the mediating role of CVRFs and CVD in sex differences in cognitive functioning. Consistent with previous findings, sex differences in cognitive functioning were small to moderate [48–51]. In line with a previous study, the sex difference in information processing speed increased during follow-up, because men declined more rapidly than women, and the sex difference in episodic memory remained stable over time [2]. Due to a similar prevalence or mean value of CVRFs and CVD between men and women (small a-path), a small effect of CVRFs and CVD on cognitive functioning (small b-path) or both, the majority of the indirect effect estimates were small in comparison with the total effects of sex on information processing speed and episodic memory. Nevertheless, our study has provided evidence that CVRFs and CVD constitute one mechanism that, among other mechanisms, contributes to our understanding of sex differences in cognitive functioning.
Although female-specific risk factors such as early menopause and hormone therapy were not included in this study because these factors cannot be compared to men, female-specific risk factors have a negative influence on cognitive functioning and may have pulled down the mean scores of women [52]. Consequently, sex differences in cognitive functioning may have been even larger.
Mechanisms relating CVRFs to cognitive functioning
Interestingly, CVD did not come forward as a mediator in our analyses. Both the sex difference in the prevalence of CVD and the effect of CVD on cognitive functioning may have been too small. This suggests that the mechanisms explaining sex differences in cognitive functioning only to a limited extent operate through CVD and that CVRFs likely affect the brain more directly. Smoking, physical inactivity, obesity, depressive symptoms, and systolic blood pressure were statistically significant in at least one of the multiple mediator models. All have been associated with structural and functional brain changes, including grey matter atrophy, small vessel disease, reduced white matter integrity, decreased blood flow, and reduced functional activity, that have a negative influence on cognitive functioning [17, 53–57]. Several mechanisms have been hypothesized that could cause these structural and functional brain changes. First, an imbalance in cytokines and neurotransmitters has been observed in depressed or obese persons, impairing cellular signaling and dysregulating neuronal networks in the brain [17, 52, 55]. Second, brain-derived neurotrophic factor levels, important for maintaining the structural integrity of the brain, increase in response to physical activity and are low in depressed or obese persons [17, 54, 55, 57]. Third, compounds of cigarette smoke could have cytotoxic effects on cerebral tissue, resulting in cell injury and neuronal cell loss [53]. Finally, cerebral hypoperfusion can cause hypoxia, which interferes with normal brain function [58]. Cerebral hypoperfusion can stem from cytotoxic effects of compounds of cigarette smoke on blood vessels, but also from hypotension [53]. In younger adults, lower blood pressure has been associated with better cognitive functioning, but due to age-related impairment of the blood pressure regulation mechanisms, higher blood pressure may be beneficial for cognitive functioning in older adults [59, 60].
Therefore, some of these mechanisms could be related to CVD, but not all. In line with this, we found that indirect effect estimates for CVRFs were hardly attenuated in multiple mediator models. Moreover, in a post-hoc analysis (data not shown) we found that they were not further attenuated by CVD, suggesting that there was limited overlap between the mediating effects of CVRFs.
Finally, CVRFs seemed to explain a larger part of the sex difference in information processing speed than of episodic memory, suggesting that CVRFs have a larger influence on information processing speed [61, 62]. An explanation may be that impaired memory function likely results from damage to a specific brain area, the hippocampus, while impaired information processing speed likely results from damage to the more widespread white matter tracts covering various brain areas, and CVRFs appear to affect the brain more generally than a single area [63].
Strengths and limitations
A strength of this study is the availability of two measures of cognitive functioning, providing the opportunity to study and compare the effects on two domains of cognitive functioning. Furthermore, a wide variety of mediators were included, covering various aspects of cardiovascular health. Another strength is the use of a large observational population-based cohort of older adults with an almost equal number of men and women, which allowed us to estimate multiple mediator models, providing indirect effect estimates adjusted for the influence of the other hypothesized mediators [64].
A limitation of the current study is that follow-up data was not available for all respondents on all mediators, which is the reason we limited the follow-up duration to approximately six years. We assumed that changes in most CVRFs and CVD over a six-year period would be small on average. A study showed that, in general, the differences in average changes in CVRFs between men and women were small, which implies limited bias in the a-paths [65]. Evidence on how changes in CVRFs and CVD affect cognitive functioning is lacking, making it hard to determine how our assumption has biased the b-paths. Another source of bias is attrition, which was mainly because of missing data on follow-up. As sex differences in both measures of cognitive functioning were larger in included respondents than in excluded respondents, true sex differences may have been smaller. The proportion of men and women was similar in included and excluded respondents, suggesting limited bias in the a-paths. The b-paths may be underestimated because the excluded respondents on average had an unhealthier cardiovascular risk profile and worse cognitive functioning than included respondents. Another limitation is that we only took current blood pressure and cholesterol levels into account, as data on levels earlier in life that could also have affected cognitive functioning were not available. Also, we did not adjust for medication that can impair cognitive functioning, such as benzodiazepines and anticholinergics, however, these effects are likely to be small [66]. Finally, other studies are needed to verify whether the variety of associations that we tested are replicated in different samples.
Future perspectives on cardiovascular risk management to preserve cognitive functioning
Women had better cognitive functioning, which was partially explained by the fact that women, in general, had a healthier cardiovascular risk profile than men. As the CVRFs under study are modifiable, cardiovascular risk management may help to preserve cognitive functioning. Our study provides some additional insights.
First, not all CVRFs had a negative effect on cognitive functioning. Higher total cholesterol levels and higher blood pressure were associated with better cognitive functioning. For this reason, some aspects of cardiovascular risk management, but not all, may have a beneficial effect on cognitive functioning of older adults.
Second, sex- and age-specific strategies may be of importance to preserve cognitive functioning. For example, our results for information processing speed suggest that an intervention on smoking may be effective in men only and that an intervention on diabetes mellitus may be effective in women only.
Finally, the effects of lifestyle interventions and antihypertensive withdrawal on cognitive functioning were limited in clinical trials, yet some CVRFs were statistically significantly associated with worse cognitive functioning in our study [67–69]. This could mean that the effects we found are too small to be clinically relevant, but also that the effects of CVRFs on cognitive functioning are (partially) irreversible. In addition, our results suggest that interventions on CVRFs may be less beneficial for memory function than for processing speed. Both the reversibility as well as the clinical relevance of effects of CVRFs on different cognitive functions need to be explored in future studies.
CONCLUSION
The current study investigated the mediating role of CVRFs and CVD in sex differences in cognitive functioning. Differences in the prevalence of CVRFs partially explained why women had better cognitive functioning than men. In addition, the effects of smoking, LDL cholesterol, and diabetes mellitus on information processing speed differed between women and men. A healthy cardiovascular lifestyle seems beneficial for cognitive functioning and sex-specific strategies may be important to preserve cognitive functioning at older age.
ACKNOWLEDGMENTS
The Longitudinal Aging Study Amsterdam is supported by a grant from the Netherlands Ministry of Health Welfare and Sports, Directorate of Long-Term Care. The authors of this paper did not receive any specific grant from funding agencies in the public, commercial, or not-for-profit sectors.
Authors’ disclosures available online (https://www.j-alz.com/manuscript-disclosures/20-1173r2).
SUPPLEMENTARY MATERIAL
[1] The supplementary material is available in the electronic version of this article: https://dx.doi.org/10.3233/JAD-201173.
REFERENCES
[1] | Ferreira L , Ferreira Santos-Galduroz R , Ferri CP , Fernandes Galduroz JC ((2014) ) Rate of cognitive decline in relation to sex after 60 years-of-age: A systematic review. Geriatr Gerontol Int 14: , 23–31. |
[2] | McCarrey AC , An Y , Kitner-Triolo MH , Ferrucci L , Resnick SM ((2016) ) Sex differences in cognitive trajectories in clinically normal older adults. Psychol Aging 31: , 166–175. |
[3] | Munro CA , Winicki JM , Schretlen DJ , Gower EW , Turano KA , Munoz B , Keay L , Bandeen-Roche K , West SK ((2012) ) Sex differences in cognition in healthy elderly individuals. Neuropsychol Dev Cogn B Aging Neuropsychol Cogn 19: , 759–768. |
[4] | Andrew MK , Tierney MC ((2018) ) The puzzle of sex, gender and Alzheimer’s disease: Why are women more often affected than men? Womens Health 14: , 8. |
[5] | Hill AC , Laird AR , Robinson JL ((2014) ) Gender differences in working memory networks: A BrainMap meta-analysis. Biol Psychol 102: , 18–29. |
[6] | Sundermann EE , Maki PM , Rubin LH , Lipton RB , Landau S , Biegon A , Alzheimer’s Disease Neuroimaging Initiative ((2016) ) Female advantage in verbal memory: Evidence of sex-specific cognitive reserve. Neurology 87: , 1916–1924. |
[7] | Gurvich C , Hoy K , Thomas N , Kulkarni J ((2018) ) Sex differences and the influence of sex hormones on cognition through adulthood and the aging process. Brain Sci 8: , 163. |
[8] | Volgman AS , Bairey Merz CN , Aggarwal NT , Bittner V , Bunch TJ , Gorelick PB , Maki P , Patel HN , Poppas A , Ruskin J , Russo AM , Waldstein SR , Wenger NK , Yaffe K , Pepine CJ ((2019) ) Sex differences in cardiovascular disease and cognitive impairment: Another health disparity for women?e. J Am Heart Assoc 8: , 013154. |
[9] | Appelman Y , van Rijn BB , Ten Haaf ME , Boersma E , Peters SA ((2015) ) Sex differences in cardiovascular risk factors and disease prevention. Atherosclerosis 241: , 211–218. |
[10] | Humphries KH , Izadnegahdar M , Sedlak T , Saw J , Johnston N , Schenck-Gustafsson K , Shah RU , Regitz-Zagrosek V , Grewal J , Vaccarino V , Wei J , Bairey Merz CN ((2017) ) Sex differences in cardiovascular disease - Impact on care and outcomes. Front Neuroendocrinol 46: , 46–70. |
[11] | Huxley RR , Woodward M ((2011) ) Cigarette smoking as a risk factor for coronary heart disease in women compared with men: A systematic review and meta-analysis of prospective cohort studies. Lancet 378: , 1297–1305. |
[12] | Sun F , Norman IJ , While AE ((2013) ) Physical activity in older people: A systematic review.. BMC Public Health 13: , 449. |
[13] | Pepine CJ , Ferdinand KC , Shaw LJ , Light-McGroary KA , Shah RU , Gulati M , Duvernoy C , Walsh MN , Bairey Merz CN , ACC CVD in Women Committee ((2015) ) Emergence of nonobstructive coronary artery disease: A woman’s problem and need for change in definition on angiography. J Am Coll Cardiol 66: , 1918–1933. |
[14] | Virani SS , Alonso A , Benjamin EJ , Bittencourt MS , Callaway CW , Carson AP , Chamberlain AM , Chang AR , Cheng S , Delling FN , Djousse L , Elkind MSV , Ferguson JF , Fornage M , Khan SS , Kissela BM , Knutson KL , Kwan TW , Lackland DT , Lewis TT , Lichtman JH , Longenecker CT , Loop MS , Lutsey PL , Martin SS , Matsushita K , Moran AE , Mussolino ME , Perak AM , Rosamond WD , Roth GA , Sampson UKA , Satou GM , Schroeder EB , Shah SH , Shay CM , Spartano NL , Stokes A , Tirschwell DL , VanWagner LB , Tsao CW ; American Heart Association Council on Epidemiology and Prevention Statistics Committee and Stroke Statistics Subcommittee ((2020) ) Heart disease and stroke statistics-2020 update: A report from the American Heart Association. Circulation 141: , e139–e596. |
[15] | Johansen MC , Langton-Frost N , Gottesman RF ((2020) ) The role of cardiovascular disease in cognitive impairment. Curr Geriatr Rep 9: , 1–9. |
[16] | Leritz EC , McGlinchey RE , Kellison I , Rudolph JL , Milberg WP ((2011) ) Cardiovascular disease risk factors and cognition in the elderly. Curr Cardiovasc Risk Rep 5: , 407–412. |
[17] | Richardson L , Adams S ((2018) ) Cognitive deficits in patients with depression. J Nurse Pract 14: , P437–443.e3. |
[18] | Lourenco J , Serrano A , Santos-Silva A , Gomes M , Afonso C , Freitas P , Paul C , Costa E ((2018) ) Cardiovascular risk factors are correlated with low cognitive function among older adults across Europe based on The SHARE Database. Aging Dis 9: , 90–101. |
[19] | Gannon OJ , Robison LS , Custozzo AJ , Zuloaga KL ((2019) ) Sex differences in risk factors for vascular contributions to cognitive impairment & dementia.. Neurochem Int 127: , 38–55. |
[20] | MacKinnon DP , Valente MJ , Gonzalez O ((2020) ) The correspondence between causal and traditional mediation analysis: The link is the mediator by treatment interaction. Prev Sci 21: , 147–157. |
[21] | VanderWeele T , Vansteelandt S ((2009) ) Conceptual issues concerning mediation, interventions and composition. Stat Interface 2: , 457–468. |
[22] | Schievink SHJ , van Boxtel MPJ , Deckers K , van Oostenbrugge RJ , Verhey FRJ , Kohler S ((2017) ) Cognitive changes in prevalent and incident cardiovascular disease: A 12-year follow-up in the Maastricht Aging Study (MAAS). Eur Heart J, doi: 10.1093/eurheartj/ehx365 |
[23] | Hoogendijk EO , Deeg DJH , de Breij S , Klokgieters SS , Kok AAL , Stringa N , Timmermans EJ , van Schoor NM , van Zutphen EM , van der Horst M , Poppelaars J , Malhoe P , Huisman M ((2020) ) The Longitudinal Aging Study Amsterdam: Cohort update 2019 and additional data collections. Eur J Epidemiol 35: , 61–74. |
[24] | Huisman M , Poppelaars J , van der Horst M , Beekman AT , Brug J , van Tilburg TG , Deeg DJ ((2011) ) Cohort profile: The Longitudinal Aging Study Amsterdam. Int J Epidemiol 40: , 868–876. |
[25] | Salthouse TA ((1996) ) The processing-speed theory of adult age differences in cognition. Psychol Rev 103: , 403–428. |
[26] | Blazer DG , Yaffe K , Liverman CT ((2015) ) Cognitive Aging: Progress in Understanding and Opportunities for Action. Institute of Medicine of the National Academies, Washington, D.C. |
[27] | Savage RD ((1984) ) Alphabet Coding Task 15. Unpublished manuscript. Murdoch University, Western Australia. |
[28] | Deeg DJH , Westendorp-de Serriere M ((1994) ) Autonomy and Well-being in the AgingPopulation I: Report fromthe Longitudinal Aging Study, Amsterdam 1992–1993, VU University Press, Amsterdam. |
[29] | Rey A ((1964) ) L’examen Clinique en Psychologie, Presse universitaire de France, Paris. |
[30] | Folstein MF , Folstein SE , McHugh PR ((1975) ) “Mini-mental state”. A practical method for grading the cognitive state of patients for the clinician. J Psychiatr Res 12: , 189–198. |
[31] | Sabia S , Elbaz A , Dugravot A , Head J , Shipley M , Hagger-Johnson G , Kivimaki M , Singh-Manoux A ((2012) ) Impact of smoking on cognitive decline in early old age: The Whitehall II cohort study. Arch Gen Psychiatry 69: , 627–635. |
[32] | Stel VS , Smit JH , Pluijm SM , Visser M , Deeg DJ , Lips P ((2004) ) Comparison of the LASA Physical Activity Questionnaire with a 7-day diary and pedometer. J Clin Epidemiol 57: , 252–258. |
[33] | Ainsworth BE , Haskell WL , Herrmann SD , Meckes N , Bassett DR Jr. , Tudor-Locke C , Greer JL , Vezina J , Whitt-Glover MC , Leon AS ((2011) ) 2011 Compendium of Physical Activities: A second update of codes and MET values. Med Sci Sports Exerc 43: , 1575–1581. |
[34] | Radloff LS ((1977) ) The CES-D Scale: A self-report depression scale for research in the general population. Appl Psychol Meas 3: , 385–401. |
[35] | Vaccarino V , Badimon L , Bremner JD , Cenko E , Cubedo J , Dorobantu M , Duncker DJ , Koller A , Manfrini O , Milicic D , Padro T , Pries AR , Quyyumi AA , Tousoulis D , Trifunovic D , Vasiljevic Z , de Wit C , Bugiardini R , ESC Scientific Document Group Reviewers ((2020) ) Depression and coronary heart disease: 2018 ESC position paper of the working group of coronary pathophysiology and microcirculation developed under the auspices of the ESC Committee for Practice Guidelines. Eur Heart J 41: , 1687–1696. |
[36] | Murrough JW , Iacoviello B , Neumeister A , Charney DS , Iosifescu DV ((2011) ) Cognitive dysfunction in depression: Neurocircuitry and new therapeutic strategies. Neurobiol Learn Mem 96: , 553–563. |
[37] | Diabetes Mellitus (algorithm). https://www.lasa-vu.nl/themes/physical/diabetes-mellitus.htm |
[38] | Cardiovascular diseases (algorithm). https://www.lasa-vu.nl/themes/physical/cardiovascular-diseases.htm |
[39] | StataCorp. (2015) Stata Statistical Software: Release 14. StataCorp LP, College Station, TX. |
[40] | Li Y , Schneider JA , Bennett DA ((2007) ) Estimation of the mediation effect with a binary mediator. Stat Med 26: , 3398–3414. |
[41] | VanderWeele T ((2015) ) Explanation in causal inference: Methods for mediation and interaction. Oxford University Press, New York. |
[42] | Hayes AF ((2013) ) Introduction to Mediation, Moderation, and Conditional Process Analysis. A Regression-Based Approach. The Guilford Press, New York, NY. |
[43] | Twisk JWR ((2019) ) Multivariable modelling. In Applied Mixed Model Analysis. Cambridge University Press, Cambridge, pp. 58–77. |
[44] | Perneger TV ((1998) ) What’s wrong with Bonferroni adjustments. BMJ 316: , 1236–1238. |
[45] | Euser SM , van Bemmel T , Schram MT , Gussekloo J , Hofman A , Westendorp RG , Breteler MM ((2009) ) The effect of age on the association between blood pressure and cognitive function later in life. J Am Geriatr Soc 57: , 1232–1237. |
[46] | Nilsson LG , Nilsson E ((2009) ) Overweight and cognition. Scand J Psychol 50: , 660–667. |
[47] | van den Heuvel EG , van Schoor N , de Jongh RT , Visser M , Lips P ((2013) ) Cross-sectional study on different characteristics of physical activity as determinants of vitamin D status; inadequate in half of the population. Eur J Clin Nutr 67: , 360–365. |
[48] | Alwerdt J , Patterson AD , Sliwinski MJ ((2019) ) Gender differences in phytoestrogens and the relationship with speed of processing in older adults: A cross-sectional analysis of NHANES, 1999-2002. Nutrients 11: , 1780. |
[49] | Cohen J ((1988) ) Statistical Power Analysis for the Behavioral Sciences. Lawrence Erlbaum Associates, Mahwah, NJ. |
[50] | Mura T , Amieva H , Goldberg M , Dartigues JF , Ankri J , Zins M , Berr C ((2016) ) Effect size for the main cognitive function determinants in a large cross-sectional study. Eur J Neurol 23: , 1614–1626. |
[51] | Reas ET , Laughlin GA , Bergstrom J , Kritz-Silverstein D , Barrett-Connor E , McEvoy LK ((2017) ) Effects of sex and education on cognitive change over a 27-year period in older adults: The Rancho Bernardo Study. Am J Geriatr Psychiatry 25: , 889–899. |
[52] | Nebel RA , Aggarwal NT , Barnes LL , Gallagher A , Goldstein JM , Kantarci K , Mallampalli MP , Mormino EC , Scott L , Yu WH , Maki PM , Mielke MM ((2018) ) Understanding the impact of sex and gender in Alzheimer’s disease: A call to action. Alzheimers Dement 14: , 1171–1183. |
[53] | Durazzo TC , Meyerhoff DJ , Nixon SJ ((2010) ) Chronic cigarette smoking: Implications for neurocognition and brain neurobiology. Int J Environ Res Public Health 7: , 3760–3791. |
[54] | Dye L , Boyle NB , Champ C , Lawton C ((2017) ) The relationship between obesity and cognitive health and decline. Proc Nutr Soc 76: , 443–454. |
[55] | Gogniat MA , Robinson TL , Mewborn CM , Jean KR , Miller LS ((2018) ) Body mass index and its relation to neuropsychological functioning and brain volume in healthy older adults. Behav Brain Res 348: , 235–240. |
[56] | Hayes SM , Alosco ML , Hayes JP , Cadden M , Peterson KM , Allsup K , Forman DE , Sperling RA , Verfaellie M ((2015) ) Physical activity is positively associated with episodic memory in aging. J Int Neuropsychol Soc 21: , 780–790. |
[57] | Voss MW , Soto C , Yoo S , Sodoma M , Vivar C , van Praag H ((2019) ) Exercise and hippocampal memory systems. Trends Cogn Sci 23: , 318–333. |
[58] | Wolters FJ , Zonneveld HI , Hofman A , van der Lugt A , Koudstaal PJ , Vernooij MW , Ikram MA , Heart-Brain Connection Collaborative Research Group ((2017) ) Cerebral perfusion and the risk of dementia: A population-based study. Circulation 136: , 719–728. |
[59] | Gupta V , Lipsitz LA ((2007) ) Orthostatic hypotension in the elderly: Diagnosis and treatment. Am J Med 120: , 841–847. |
[60] | Forte G , De Pascalis V , Favieri F , Casagrande M ((2019) ) Effects of blood pressure on cognitive performance: A systematic review. J Clin Med 9: , 34. |
[61] | van Dijk EJ , Prins ND , Vrooman HA , Hofman A , Koudstaal PJ , Breteler MM ((2008) ) Progression of cerebral small vessel disease in relation to risk factors and cognitive consequences: Rotterdam Scan study. Stroke 39: , 2712–2719. |
[62] | Zhou TL , Kroon AA , van Sloten TT , van Boxtel MPJ , Verhey FRJ , Schram MT , Kohler S , Stehouwer CDA , Henry RMA ((2019) ) Greater blood pressure variability is associated with lower cognitive performance. Hypertension 73: , 803–811. |
[63] | Song R , Xu H , Dintica CS , Song R , Pan K , Qi X , Buchman AS , Bennett DA , Xu W ((2020) ) Associations between cardiovascular risk, structural brain changes, and cognitive decline. J Am Coll Cardiol 75: , 2525–2534. |
[64] | MacKinnon DP , Fairchild AJ , Fritz MS ((2007) ) Mediation analysis. Annu Rev Psychol 58: , 593–614. |
[65] | Hulman A , Tabak AG , Nyari TA , Vistisen D , Kivimaki M , Brunner EJ , Witte DR ((2014) ) Effect of secular trends on age-related trajectories of cardiovascular risk factors: The Whitehall II longitudinal study 1985-2009. Int J Epidemiol 43: , 866–877. |
[66] | Moriarty F , Savva GM , Grossi CM , Bennett K , Fox C , Maidment I , Loke YK , Steel N , Kenny RA , Richardson K ((2020) ) Cognitive decline associated with anticholinergics, benzodiazepines, and Z-drugs: Findings from The Irish Longitudinal Study on Ageing (TILDA). medRxiv, 2020.2005.2009.20095661. |
[67] | Andrieu S , Coley N , Lovestone S , Aisen PS , Vellas B ((2015) ) Prevention of sporadic Alzheimer’s disease: Lessons learned from clinical trials and future directions. Lancet Neurol 14: , 926–944. |
[68] | Jongstra S , Harrison JK , Quinn TJ , Richard E ((2016) ) Antihypertensive withdrawal for the prevention of cognitive decline. Cochrane Database Syst Rev 11: , CD011971. |
[69] | Kivipelto M , Mangialasche F , Ngandu T ((2018) ) Lifestyle interventions to prevent cognitive impairment, dementia and Alzheimer disease. Nat Rev Neurol 14: , 653–666. |