Early Cognitive Training Rescues Remote Spatial Memory but Reduces Cognitive Flexibility in Alzheimer’s Disease Mice
Abstract
Background:
Spatial memory dysfunction has been demonstrated in mouse models of Alzheimer’s disease (AD) which is consistent with the clinical finding that the early signature of AD includes difficulties in the formation and/or storage of a memory. A stored memory—a long term memory—can be modulated via process called as memory retrieval that can either lead toward memory reconsolidation or even memory extinction.
Objective:
We aim to shed light on the fate of the spatial memory during memory reactivation and memory extinction using a water maze task.
Methods:
In Set-up I, we trained 3-month-old mice (wild-type mice and mice with cerebral β-amyloidosis) and assessed the fate of remote memory after four months of retention interval (RI). In Set-up II, we performed an early-extensive training at 2 months of age, retrained the same mice at 3 months of age, introduced four months of RI, and finally assessed remote spatial memory at 7 months of age.
Results:
We find in β-amyloidosis mice that memory reactivation problems were detectable at 7 months of age and were alleviated by cognitive overtraining. Similarly, forgetting of remote spatial memory was also minimized by cognitive overtraining. Finally, we show that the cognitive training facilitates the recovery of the reactivated spatial memory while reducing the ability to form new spatial memory in AD mice.
Conclusion:
This result may explain the rationality behind the cognitive reserve observed in AD patients and elderly with severe β-amyloidosis not corresponding to the actual low dementia symptoms.
INTRODUCTION
The mice that overexpress mutant human amyloid-β protein precursor (AβPP), presenilin (PS), and/or tau proteins have successfully recapitulated multiple neuropathological features of Alzheimer’s disease (AD) including amyloid-β (Aβ) deposits, neurofibrillary tangles, synaptic dysfunction, and gliosis [1–3]. Similarly, the pathology has also been correlated with the memory deficit observed in a battery of behavioral tests such as the Morris water maze (WM) task, elevated plus maze, fear conditioning, etc. [1, 3–5]. The famous Nun Study, however, shows that memory dysfunction in AD and Aβ load may not correspond to each other in their severity [6]. Risk factors such as low linguistic ability (idea density and grammatical complexity) in early life increases the risk for dementia [6], while intelligence lowers the risk [7]. Similarly, activities that induce cognitive stimulation lower cognitive dysfunction in elderly [8]. It has recently been shown that cognitive training employing a water maze (WM) task preserves fear related memory in the Tg2576 AD mouse model [5].
Memory studies conducted with AD mice assessed learning and memory shortly after training [3, 5, 9]. However, the fate of a memory (remote memory) remains unknown when the gap between training and testing, also called retention interval (RI), is prolonged. New memories are gradually stabilized by memory consolidation [10], which is a time dependent process [11, 12]. It is also clear that reactivation of previously consolidated memory makes it enter an active and labile state, and subsequent reconsolidation makes the memory to become more resistant to decay [13]. This process is called memory reconsolidation and is one of the least understood components in memory research. Retraining animals to the same test, the memory retrieval initiates the memory reconsolidation [14]. The retrieval of remote spatial memory is thought to be a hippocampal independent event and involves neocortical regions such as prefrontal and anterior cingulate cortex [15]. However, memory retrieval can also initiate the opposite process called extinction, which weakens the memory [16]. Studies have shown that the RI determines the fate of a memory. A shorter RI initiates memory reconsolidation whereas a longer RI results in memory extinction [17–19]. Additionally, memory extinction occurs on the basis of relevance or recency as well [20].
Multiple studies have been performed to assess the fate of a remote memory after brain structural damage with major focus on rats [21–23]. Only one study has reported to measure remote spatial memory in AD mice using Tg2576 mice [5]. Therefore, we studied the remote spatial memory in an APP/PS1 mouse model [1].
The fate of remote spatial memory, i.e., reactivation after prolonged RI, and spontaneous recovery of an original memory after extinction, has not been studied in any AD mouse model. Here, we trained or overtrained both wild-type and APPtg mice, introduced a RI of 4 months, and assessed the forgetting of remote spatial memory and memory reactivation at 7 months of age. Similarly, after assessing memory reactivation, we retrained those mice in a platform reversal task for additional five consecutive days to study the possibility of recovery of the original reactivated spatial memory. We find that in APP/PS1 mice that memory reactivation dysfunction appears as early as 7 months of age. We also find that forgetting of a remote spatial memory as well as memory reactivation dysfunction can be minimized by early overtraining of these mice. In overtrained mice (both wild type and APPtg), we also show that the reactivated spatial memory can be spontaneously recovered even after platform reversal task.
MATERIAL AND METHODS
Animals
All experiments were approved by local authorities and were in accordance with the guidelines for animal experiments of the EU (Directive 2010/63/EU). All mice were group housed at 21-23°C with food (RM3P- Product code: 801/700; Scanbur AS Norway) and water ad libitum under 12 h day/night cycle. Here, we used female 2-, 3-, and 7-month-old C57BL/6J and APP/PS1-21 (APPtg) mice. The characterization of APP/PS1-21 was published previously [1, 4]. In this study, we exclusively used hemizygous APP/PS1-21 (APPtg+/-) mice.
Experimental design
Set-up I
Using the WM task as described below, we started training both wild-type C57BL/6J and APPtg mice at 3 months of age and later assessed learning and memory at 7 months of age. At 7 months of age, we first assessed the remote spatial memory via retraining mice on the same platform position. From day 10 onwards, we performed a WM reversal task (platform shifted towards the opposite position) for five consecutive days and a final probe trial at day 15 as shown in Fig. 1.
Fig.1
Experimental design for the assessment of remote spatial memory using WM relearning and WM reversal task. Red circle indicated the platform position for normal WM task and WM reversal task.
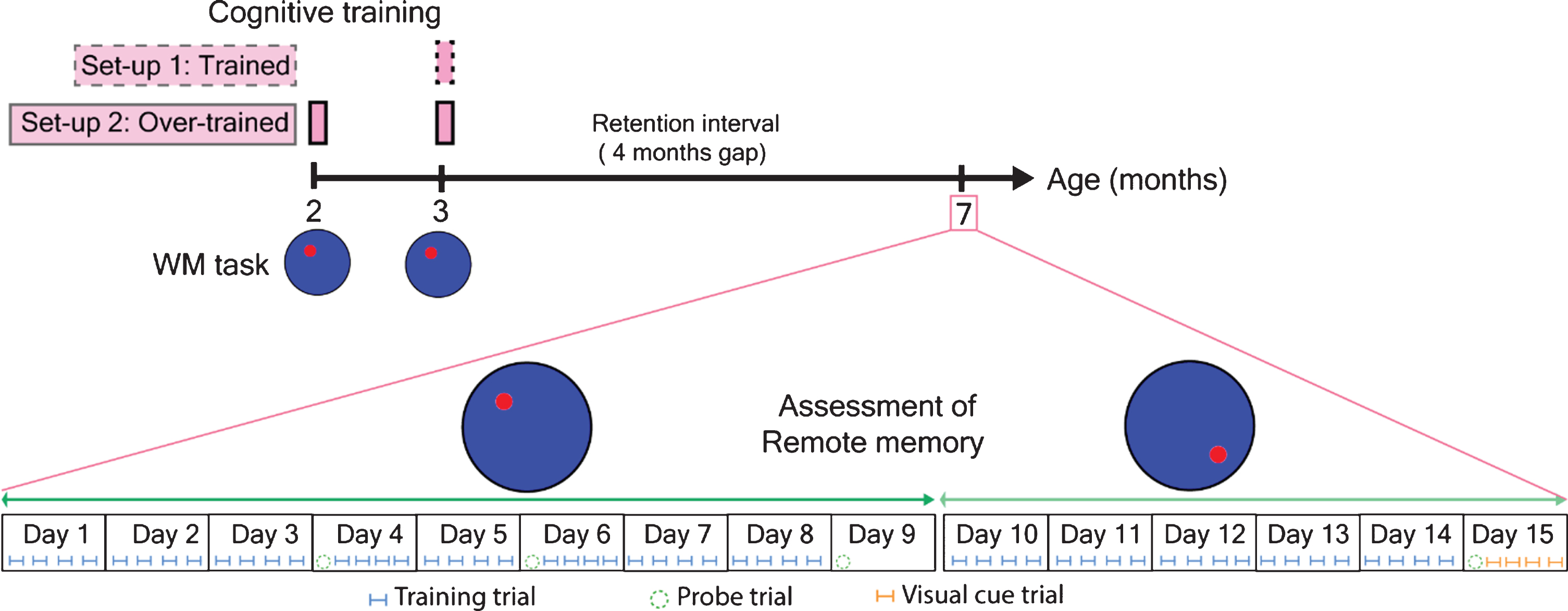
Set-up II
Here, we started training mice at 2 months of age and later overtrained at 3 months again. Finally, at 7 months, we assessed the performance of mice in the WM relearning task as well as in the WM reversal task, respectively, as shown in Fig. 1.
Morris water maze task
The WM task was used as a tool for cognitive training. The method has been described previously [24]. In brief, a circular water tank (color: white, height: 30 cm, diameter: 116 cm, and 75 cm above the floor) was located 30 cm away from walls/curtains (color: white). As the color of the water tank was white and the color of the plexiglass-platform was transparent, no additional color was added into water. A circular platform (height: 15 cm, and diameter: 10 cm) was fixed at a particular location during training trials. Additionally, the platform was submerged to a depth of 0.5-1.0 cm below the water surface. The water temperature was 22.5±1°C throughout the experiment. Similarly, the light intensity in a water pool was maintained at 65-70 lux and was provided through a diffuse light fixed above the water pool. Four distinct 2D-geometrical shapes were fixed on walls/curtains (115 cm above the ground) that act as distal spatial cues.
An individual mouse was allowed to swim four trials per day with an inter-trial interval (ITI) of 1 min for eight consecutive days. The experiment was stopped if a mouse sat on a hidden platform for 10 s or if the mouse swam for a maximum of 90 s per trial. Here, a mouse had to learn to find a hidden, fixed platform using distal spatial cues. To assess spatial memory, three individual probe trials with a trial duration of 30 s were given on day 4, day 6, and day 9, respectively. As a control experiment, additional four trials were given as visual cue trials on day 9.
The proximity was primarily used as a measure of learning and memory. Proximity [cm] was defined as the average position between the mouse and the hidden platform. Similarly, other measures—latency, track length, and % age time spent on the target quadrant—were additionally used as well. Latency [s] was defined as the time taken by the mouse to find and climb on the hidden platform. Track length [cm] was defined as the total distance swam by the mouse before it climbed on the platform. Finally, % age time spent on the target quadrant (% age s) was defined as the time spent by the mouse (%) on the target quadrant.
At the end of the WM task with 7-month-old mice, we omitted visual cue trials on day 9 as it might hamper the WM reversal task from day 10 onwards.
WM reversal task
A WM reversal task was used as a tool to study the remote spatial memory. The task was given from day 10 onwards after completion of the assessment of learning and memory on 7-month-old mice. Here, starting positions as well as platform position were rotated by 180°. The WM reversal task also consisted of normal training trials, a probe trial, and visual cue trials. During the training trials, each mouse was trained with four consecutive trials per day for five consecutive days in which each trial lasted for a maximum of 90 s with an ITI of 1 min. On day 15, a single probe trial of 30 s was given from a novel position. After 2 h of completion of a final probe trial, four visual cue trials were given to each mouse as described previously.
The navigation of a mouse during the training trials was classified into different search strategies [25] and the graphical representation were shown in Fig. 2 as:
Fig.2
Graphical representation and classification of a mouse trajectory into different search strategy. The search strategies were mathematically/statistically defined as: thigmotaxis: >35% of time (90 s) within closer wall zone (10 cm from the pool wall) and < 65% time in wider wall zone (16 cm from the pool wall); random search: >70% surface coverage; scanning: <70% surface coverage, >10% surface coverage, and < 0.7 SD (standard deviation) distance to the pool center; chaining: >65% of time within the annulus zone; perseverance: <0.45 SEM body angle, <0.40 SD mean distance to the previous goal; directed search: >80% of time in the goal corridor; focal search: <0.35 SEM (standard error of the mean) body angle, <0.25 SD mean distance to the present goal; and direct swim: 100% in the goal corridor; unclassified: the algorithm could not able to classify into above mentioned classification. An 18-cm wide goal corridor was set. Each column is a discrete search strategy that consists of three representative mice trajectories from training trials for visualization. Furthermore, a search strategy is divided into thigmotaxis, non-spatial search strategy (random, scanning, and chaining), and spatial search strategy (focal, directed, and direct). A blue line was a mouse trajectory and a red circle was a platform.
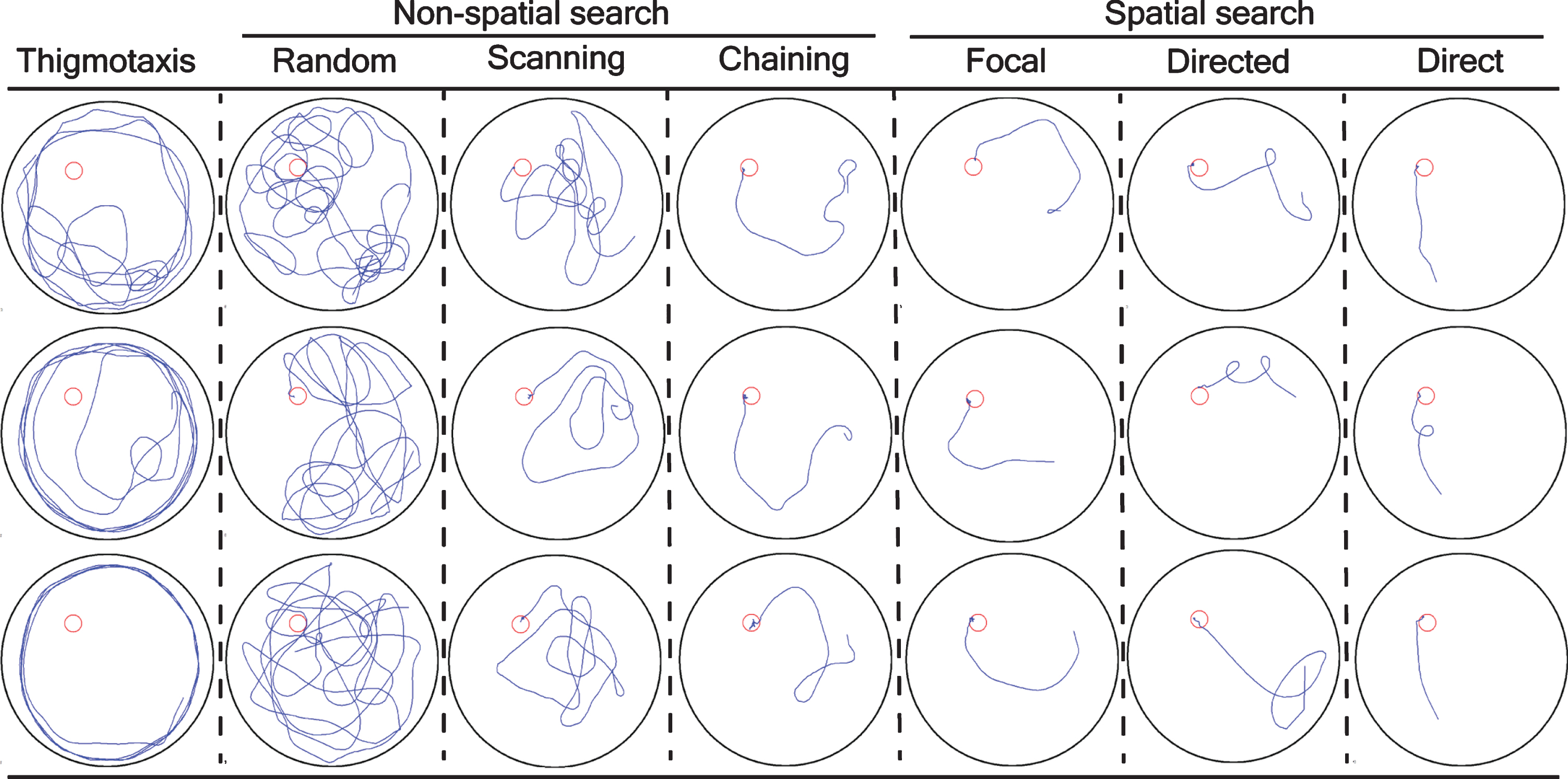
Finally, we performed exclusion criteria to remove unmotivated/non-learners from the final data analysis. A mouse will only be removed from data analysis if all criteria are met: 1) if more than 50% of trials of a mouse were unsuccessful, 2) if more than 50% of trials of a mouse had a wall factor value (% time spent in closer wall zone or thigmotaxis) more than 60, and 3) if latency was more than 30 s in at least three trials of visual cue trial experiment. Based on this criterion, the number of animals used in data analysis of each group for the experiments are shown in Table 1.
Table 1
Summary table showing number of animals used in the different groups for final data analysis of the WM experiments. The average age of animals when they entered the WM test were 2 months (60±4 days), 3 months (94±4 days), and 7 months (209±4 days).
Age (months) | |||||
2 | 3 | 7 | 7 | ||
Groups | WM task | WM task | WM task | WM reversal task | |
Set-up I | Trained C57BL/6J | 9 | 10 | 10 | |
Set-up I | Trained APPtg | 10 | 10 | 9 | |
Set-up II | Overtrained C57BL/6J | 11 | 11 | 11 | 11 |
Set-up II | Overtrained APPtg | 8 | 8 | 8 | 8 |
Statistics
Data processing and statistical analysis
In brief, an average performance of a mouse for a given day was calculated before final statistical analysis. The statistical evaluation for repeated measures longitudinal data was performed using a linear mixed model for MWM data [26] with the nlme package and function (lme) in R version 3.6.1 (https://www.r-project.org/) in which proximity is a function of day and group. Here, day was used as categorical variable and an individual animal’s variation was considered as random effect. The subsequent post hoc analysis was conducted with Tukey’s comparison using lsmeans package in R.
mixed model <- lme (data = training_data, proximity ~ group * day, random = ~1|animal)
For probe trials, we also performed a One-way ANOVA analysis using the lm function. Here, group denotes the group, based on a probe trial.
probe_trial <- lm (data = probe_data, proximity ~ group)
Learning score determination
The learning score system, modification of the Gallagher learning index [27, 28], was developed as follows:
In our set-up, we assumed a mouse was said to learn the WM training task if the proximity value was maximum 30 cm for all training trials in a given day. For a mouse that passed this criterion, a numeric score (TTC score) was given. As an example, if a mouse passed the criterion on day 5, a number 17 was given as trial 17 was the first trial of day 5. Similarly, if the mouse passed the criterion on day 2, a number 5 was given. If the mouse passed the criterion on day 8, a number 29 was given. If the mouse did not pass the criterion at all, then an arbitrary score (33) was given because our set-up consisted of only 32 trials (day 8), hence 33 was given. A multiplier value M was determined as a quotient of 33 (considered as an initialization value) and TTC score of a mouse.
Finally, a LS of a mouse was determined as a sum product of M and the proximity value of a probe trial. Finally, the LS was log10 transformed.
Because of non-Gaussian distribution, Kruskall-Wallis analysis was conducted to examine the difference on the learning score (log10 transformed) of different groups based on cognitive training. Later, a post-hoc Dunn’s test with Bonferroni adjustment for multiple group comparison using FSA package [30] in R.
Cluster analysis and heat map
The cluster analysis as well as heatmap for previous target crossing (PTC) data from the WM reversal task were generated using packages ComplexHeatmap [31] and dendextend [32] in R. In brief, each row is a group and each column is a trial. The data were center-scaled (z-transformation) across training trials. A Euclidean distance matrix was created and then the “complete” algorithm was performed for hierarchical agglomerative clustering.
Creation of a spatial intensity map from trail trajectories of the mice
A point pattern map of mice trajectories was generated from the intensity function of the point process in which an intensity is a function of the spatial location [33]. The intensity estimate (
To characterize the location and the size of a spatial hotspot, the mouse trajectory during a WM probe trial was recorded every 50 ms, and was saved as bitmap (bmp) image file (768 x 629 points) by the Viewer 3 software (Biobserve GmbH, Bonn, Germany). Next, all image files for a specific group were combined together at an offset value (0,0) using pillow library and the pixel coordinates (x,y) of mice trajectories in an combined image were extracted to a csv file using numpy library in Python (v3.7.4). The point pattern analyses were conducted using spatstat package in R (v3.6.1) [35] to analyze the spatial data and to generate the spatial density map. Here we used a default Gaussian kernel smoothing as an algorithm to reveal underlying spatial features [33]. Since the spatial data were taken from the water maze pool in which the frame of observation is restricted within a water tank, edge correction was also taken into consideration. Finally, the Gaussian kernel smoothing was conducted by selecting a proper bandwidth using smoothing parameter sigma (σ).
A two-dimensional window of observation (dimension: radius = 330 pixels, and center = 330, 315 pixels) was used to create a window area of 301786 square units. Next, a spatial point pattern map was generated using “density” function from randomly selected 39,000 pixel points from the mice trajectories. The average intensity was 0.129 points per square unit. Finally, the bandwidth (h = σ/2) was amplified nine times to visualize the spatial hotspot by selecting sigma (σ = 18).
RESULTS
7-month-old APPtg mice show dysfunction in memory reactivation of remote spatial memory
In order to study the fate of remote spatial memory in APP/PS1-21 mice, we designed the Set-up I experiment. Here, we trained both C57BL/6J and APPtg mice at 3 months of age using a WM task for eight consecutive days and found significant group differences during training trials [F(1,17)=5.12, p = 0.03] (Fig. 3a, left). Furthermore, by the end of the WM task on day 8, both groups were comparable in their performance [mean proximity values: trained C57BL/6J=16.8 cm and trained APPtg = 22.4 cm, two tailed unpaired t-test: p = 0.20].
Fig.3
Deficient spatial memory reactivation in 7-month-old APPtg mice. a, b) Set-up 1: mice were trained at 3 months of age using the WM task and their cognitive performance were reassessed at 7 months of age (right). Mice were trained for eight consecutive days to find a submerged platform in a pool filled with water. The average proximity of mice with respect to a hidden platform were used as a measure of spatial learning and memory. a, right) Mice that have been trained at 3 months of age again performed the WM task at 7 months of age. Mixed model analysis showed significant group difference between trained C57BL/6J and APPtg mice [7 months: F(1,18)=5.11, p = 0.036] during the retraining task. b) Assessment of spatial memory using probe trials at 7 months of age. During a probe trial, the platform was removed, and mice were allowed to swim from a novel starting position for 30 s and the proximity (cm) of mice from the former platform position was used as a measure of spatial memory. Two-tailed unpaired t-test was performed and did not show any group differences for any probe trial-day. Values are mean±95% confidence interval.
![Deficient spatial memory reactivation in 7-month-old APPtg mice. a, b) Set-up 1: mice were trained at 3 months of age using the WM task and their cognitive performance were reassessed at 7 months of age (right). Mice were trained for eight consecutive days to find a submerged platform in a pool filled with water. The average proximity of mice with respect to a hidden platform were used as a measure of spatial learning and memory. a, right) Mice that have been trained at 3 months of age again performed the WM task at 7 months of age. Mixed model analysis showed significant group difference between trained C57BL/6J and APPtg mice [7 months: F(1,18)=5.11, p = 0.036] during the retraining task. b) Assessment of spatial memory using probe trials at 7 months of age. During a probe trial, the platform was removed, and mice were allowed to swim from a novel starting position for 30 s and the proximity (cm) of mice from the former platform position was used as a measure of spatial memory. Two-tailed unpaired t-test was performed and did not show any group differences for any probe trial-day. Values are mean±95% confidence interval.](https://ip.ios.semcs.net:443/media/jad/2020/75-4/jad-75-4-jad200161/jad-75-jad200161-g003.jpg)
After four months of RI, we reassessed their learning and memory again using the WM task in the same environment at 7 months of age. First, we assessed the forgetting of the remote spatial memory from their performance of the first trial at day 1 but did not find any significant group difference [mean proximity values: trained C57BL/6J=37.8 cm and trained APPtg = 36.4 cm, two tailed unpaired t-test: p = 0.80] indicating a similar level of forgetting. However, assessing forgetting based on a single trial can be error prone. Therefore, we also assessed forgetting based on WM performance over all trials of day 1 and did not find any significant group difference on day 1 [mean proximity values: trained C57BL/6J=32.7 cm and trained APPtg = 39.0 cm, two tailed unpaired t-test: p = 0.22].
Second, we compared the overall performance of the mice during training trials and found a significant group difference [F(1,18)=5.11, p = 0.036] as in Fig. 3a (right). Finally, we analyzed the probe trials on days 4, 6, and 9, and found that the mean proximity values at day 4 for both trained APPtg and trained C57BL/6J were 41.5 cm and 40.5 cm, respectively. Although, the mean proximity values for both groups decreased at the third probe trial on day 9, the two-tailed unpaired t-test did not show any significant group difference in any probe trial-day (Fig. 3b). However, assessing learning and memory based on a single measure can be error-prone, hence we also assessed WM performance based on other measures such as latency, track length, and % age time spent on target quadrant. These additional assessments corroborated our result that APPtg and C57BL/6J differed significantly during WM reactivation task (Supplementary Figure 1).
Cognitive overtraining rescues forgetting and improves memory reactivation of remote spatial memory
Next, we wanted to observe the effect of early and extensive training on the forgetting and reactivation of a remote spatial memory in APPtg mice. Hence, we performed another set of experiments (Set-up II). Here, we trained mice at 2 months of age, retrained them at 3 months of age, and finally reassessed their performance at 7 months of age. First, we analyzed forgetting of a remote spatial memory from their performance on the first trial of day 1 and found that there was no significant group difference [mean proximity values: overtrained C57BL/6J=30.1 cm and overtrained APPtg = 21.7 cm, two tailed unpaired t-test: p = 0.07] indicating similar level of forgetting. Second, we also compared forgetting based on overall performance of day 1 and did not find any significant group difference [mean proximity values: overtrained C57BL/6J=25.9 cm and overtrained APPtg = 25.2 cm, two tailed unpaired t-test: p = 0.77]. Third, we compared their overall performance during training trials and also did not find any significant group difference [F(1,17)=0.63, p = 0.43] (Fig. 4b). Finally, performing three different probe trials on day 4, 6, and 9 showed that the mean proximity values for both groups in any probe-trial day hovered around 30 cm (Fig. 4b). Two-tailed unpaired t-test also did not reveal any significant group difference in any probe-trial-day (Fig. 4b). Assessing WM performance based on a single measure can be error-prone. Hence, we also measured WM performance based on other measures such as latency, track length, and % age time spent on target quadrant. Using these measures, we did not find any significant group difference during training trials and probe trials (Supplementary Figure 2).
Fig.4
Cognitive overtraining rescues forgetting and improves memory reactivation in APPtg mice. a) In Set-up II (over-trained group), we started training mice at 2 months of age, retrained them at 3 months, and assessed the WM performance at 7 months of age. At 2 months, we did not find any significant difference between overtrained C57BL/6J and overtrained APPtg [F(1,17)=1.83, p = 0.19]. Retraining of the same animals at 3 months of age also did not show any significant group difference [F(1,17)=0.80, p = 0.38]. Finally, the effect of cognitive overtraining was assessed at 7 months of age and no group difference was observed between overtrained C57BL/6J and overtrained APPtg mice [F(1,17)=0.63, p = 0.43]. b) Performance of animals during probe trials. Three independent probe trials were given before normal training trials on day 4, 6, and 9, respectively, in which mice were allowed to swim for 30 s. Two-tailed unpaired t-tests were performed between groups on each probe trial. Proximity (in cm) was used as a measure of spatial learning and memory. Overtrained C57BL/6J: 7 months of age: 11 animals and overtrained APPtg: 8 animals. Values are mean±95% confidence interval.
![Cognitive overtraining rescues forgetting and improves memory reactivation in APPtg mice. a) In Set-up II (over-trained group), we started training mice at 2 months of age, retrained them at 3 months, and assessed the WM performance at 7 months of age. At 2 months, we did not find any significant difference between overtrained C57BL/6J and overtrained APPtg [F(1,17)=1.83, p = 0.19]. Retraining of the same animals at 3 months of age also did not show any significant group difference [F(1,17)=0.80, p = 0.38]. Finally, the effect of cognitive overtraining was assessed at 7 months of age and no group difference was observed between overtrained C57BL/6J and overtrained APPtg mice [F(1,17)=0.63, p = 0.43]. b) Performance of animals during probe trials. Three independent probe trials were given before normal training trials on day 4, 6, and 9, respectively, in which mice were allowed to swim for 30 s. Two-tailed unpaired t-tests were performed between groups on each probe trial. Proximity (in cm) was used as a measure of spatial learning and memory. Overtrained C57BL/6J: 7 months of age: 11 animals and overtrained APPtg: 8 animals. Values are mean±95% confidence interval.](https://ip.ios.semcs.net:443/media/jad/2020/75-4/jad-75-4-jad200161/jad-75-jad200161-g004.jpg)
Finally, in order to understand the effect of cognitive training on remote spatial memory and WM performance of various experimental groups, we re-performed statistical analysis between all possible groups during training trials performed at 7 months of age. First, we compared the performance of all groups of the first trial of day 1 and found significant pairwise difference between overtrained APPtg and trained APPtg (Table 2). Second, measuring forgetting from a single trial could be error-prone hence, we compared forgetting between all experimental groups on the overall day 1 data and found that cognitive overtraining not only significantly rescued forgetting of APPtg mice (row 2, Table 3), but also made forgetting of APPtg comparable to C57BL/6J (row 1, Table 3). We also assessed forgetting (first trial or first day) between all groups based on latency, track length, and % age time spent on target quadrant, and found that cognitive overtraining could rescue forgetting of APPtg mice (Supplementary Tables 1 and 2).
Table 2
Summary table of one-way ANOVA followed by post hoc Tukey’s comparison for different groups in a first trial of day 1. Proximity were used as a measure of learning and memory and values were taken from 7-month-old mice. Red-bold values are p < 0.05.
Main effect on Trial 1: [F(3,35)=3.87, p = 0.01] | ||||
Group | estimate | t-ratio | p | |
1 | Overtrained APPtg versus Overtrained C57BL/6J | –8.48 | –1.64 | 0.36 |
2 | Overtrained APPtg versus trained APPtg | –14.76 | –2.81 | 0.03 |
3 | Overtrained C57BL/6J versus trained C57BL/6J | –7.67 | –1.58 | 0.39 |
4 | Trained APPtg versus trained C57BL/6J | –1.39 | –0.28 | 0.99 |
Table 3
Summary table of one-way ANOVA followed by post hoc Tukey’s comparison for different groups in day 1. Proximity were used as a measure of learning and memory and values were taken from 7-month-old mice. Red-bold values are p < 0.05
Main effect on Day 1: [F(3,35)=5.64, p = 0.002] | ||||
Group | estimate | t-ratio | p | |
1 | Overtrained APPtg versus Overtrained C57BL/6J | –0.63 | –0.16 | 0.99 |
2 | Overtrained APPtg versus trained APPtg | –13.74 | –3.14 | 0.008 |
3 | Overtrained C57BL/6J versus trained C57BL/6J | –6.88 | –1.85 | 0.26 |
4 | Trained APPtg versus trained C57BL/6J | 6.22 | 1.64 | 0.37 |
Third, we compared the overall performance between all groups and found that the main impact of cognitive overtraining can be observed in APPtg mice as overtraining makes APPtg mice perform like C57BL/6J littermates (rows 1 and 2, Table 4; Supplementary Tables 3–5).
Table 4
Summary table showing linear mixed model followed by post hoc Tukey’s comparison on repeated measures data. Proximity were used as a measure of learning and memory and values were taken from training trials of 7-month-old mice. Red-bold values are p < 0.05
Main effect on overall training trials: [F(3,35)=8.04, p = 0.0003] | ||||
Group | estimate | t-ratio | p | |
1 | Overtrained APPtg versus Overtrained C57BL/6J | 0.81 | 0.30 | 0.99 |
2 | Overtrained APPtg versus trained APPtg | –10.55 | –3.85 | 0.002 |
3 | Overtrained C57BL/6J versus trained C57BL/6J | –3.52 | –1.40 | 0.50 |
4 | Trained APPtg versus trained C57BL/6J | 7.83 | 3.04 | 0.022 |
The conventional data analysis of a WM task does not integrate WM performance of training trials and probe trials. Hence, it is difficult to interpret such results. Therefore, we integrated training trials as well as probe trial(s) of each mouse to generate a learning score (LS) that enables interpretation and visualization of many groups. For this, a LS for each group (Set-up I and Set-up II) was calculated. Overall, the cognitive overtraining significantly increased the LS of both C57BL/6J and APPtg mice (Fig. 5). As shown in Fig. 5 (left), the LS of Probe trial 1 separated two groups: The first group consisted of overtrained C57BL/6J, overtrained APPtg, and trained C57BL/6J mice, as these groups are statistically not different when compared to each other. The second group consisted of a single member—trained APPtg mice, which is statistically significant to the other three groups (Fig. 5 left).
Fig.5
Effect of cognitive overtraining in the learning score of 7-month-old mice. The learning score (LS) of a mouse was determined for probe trial 1, probe trials 1 and 2, probe trials 2 and 3, and probe trials 1, 2, and 3, respectively. The LS data were log10 transformed and visualized as violin plot cum boxplot in which a circular dot (cyan color) represents the mean and the horizontal line inside the boxplot denotes the median. For probe trial(s), a Kruskall-Wallis test was performed on a log10 transformed LS data. In case of significant group differences, a post hoc Dunn’s test with Bonferroni correction was performed for multiple pairwise comparisons at alpha level of 0.05. The significant p values were denoted alongside figure. Details: For probe trial 1, a Kruskal-Wallis test showed a significant group difference [χ2(3)=12.11, p = 0.006]. For probe trials 1 and 2, we also found a significant group difference [χ2(3)=11.21, p = 0.01]. For probe trials 2 and 3, we also found a significant group difference [χ2(3)=9.80, p = 0.02]. Finally, we also found a significant group difference for probe trials 1, 2, and 3 [χ2(3)=11.00, p = 0.01]. Number of animals for each group were as follows: overtrained C57BL/6J N = 10; overtrained APPtg N = 8; trained C57BL/6J N = 10; trained APPtg N = 10.
![Effect of cognitive overtraining in the learning score of 7-month-old mice. The learning score (LS) of a mouse was determined for probe trial 1, probe trials 1 and 2, probe trials 2 and 3, and probe trials 1, 2, and 3, respectively. The LS data were log10 transformed and visualized as violin plot cum boxplot in which a circular dot (cyan color) represents the mean and the horizontal line inside the boxplot denotes the median. For probe trial(s), a Kruskall-Wallis test was performed on a log10 transformed LS data. In case of significant group differences, a post hoc Dunn’s test with Bonferroni correction was performed for multiple pairwise comparisons at alpha level of 0.05. The significant p values were denoted alongside figure. Details: For probe trial 1, a Kruskal-Wallis test showed a significant group difference [χ2(3)=12.11, p = 0.006]. For probe trials 1 and 2, we also found a significant group difference [χ2(3)=11.21, p = 0.01]. For probe trials 2 and 3, we also found a significant group difference [χ2(3)=9.80, p = 0.02]. Finally, we also found a significant group difference for probe trials 1, 2, and 3 [χ2(3)=11.00, p = 0.01]. Number of animals for each group were as follows: overtrained C57BL/6J N = 10; overtrained APPtg N = 8; trained C57BL/6J N = 10; trained APPtg N = 10.](https://ip.ios.semcs.net:443/media/jad/2020/75-4/jad-75-4-jad200161/jad-75-jad200161-g005.jpg)
Fig.6
Performance of WM task in WM reversal task. a) After performing a usual third probe trial on day 9, we skipped a normal visual cue trial since removal of distal cues during visual cue trial may alter the performance during the WM reversal task. From day 10 onwards, we rotated the platform as well as starting positions by 180° for five consecutive days. ANOVA on a mixed model showed no significant effect of cognitive training on proximity at the p-value<0.05 for four different training groups [F(3,34)=0.87, p = 0.46]. b) WM performance of different groups as a function of training trials for day 10 and day 11. c) Assessment of spatial memory in the WM reversal task on day 15. Mice were allowed to swim for 30 s in a water pool in which the platform was removed. A one-way ANOVA did not reveal significant effects of cognitive training on proximity during probe trial at the p-value<0.05 for four different training groups [F(3,34)=0.78, p = 0.51]. d) Performance of mice in visual cue trials on day 15. Latency and speed were used as measures to assess visual acuity and motor performance. One-way ANOVA analysis did not show any significant group difference in visual cue trials [latency: F(3,34)=1.35, p = 0.27; speed: F(3,34)=1.29, p = 0.29]. Values are mean±95% confidence interval.
![Performance of WM task in WM reversal task. a) After performing a usual third probe trial on day 9, we skipped a normal visual cue trial since removal of distal cues during visual cue trial may alter the performance during the WM reversal task. From day 10 onwards, we rotated the platform as well as starting positions by 180° for five consecutive days. ANOVA on a mixed model showed no significant effect of cognitive training on proximity at the p-value<0.05 for four different training groups [F(3,34)=0.87, p = 0.46]. b) WM performance of different groups as a function of training trials for day 10 and day 11. c) Assessment of spatial memory in the WM reversal task on day 15. Mice were allowed to swim for 30 s in a water pool in which the platform was removed. A one-way ANOVA did not reveal significant effects of cognitive training on proximity during probe trial at the p-value<0.05 for four different training groups [F(3,34)=0.78, p = 0.51]. d) Performance of mice in visual cue trials on day 15. Latency and speed were used as measures to assess visual acuity and motor performance. One-way ANOVA analysis did not show any significant group difference in visual cue trials [latency: F(3,34)=1.35, p = 0.27; speed: F(3,34)=1.29, p = 0.29]. Values are mean±95% confidence interval.](https://ip.ios.semcs.net:443/media/jad/2020/75-4/jad-75-4-jad200161/jad-75-jad200161-g006.jpg)
Mice can solve the WM task using egocentric navigation
Although our data suggested that cognitive overtraining with the WM task improved memory reactivation, we do not know whether the reactivated memory can still be preserved even after performing a WM reversal task. To address this question, we rotated the platform position as well as the starting positions by 180° from day 10 onwards and performed training trials for the next five consecutive days. While assessing the WM performance, we assume that all mice should enter this WM reversal task with similar level of learning and memory. Therefore, we compared the performance of all groups on day 8 of WM reactivation task and found out that all groups performed at comparable level (proximity value below 25 cm) at the end of the memory reactivation task (Tables 5 and 6). Similarly, we also compared the spatial memory of all groups on day 9, found comparable performance between experimental groups (Supplementary Tables 4 and 5).
Table 5
Summary table of one-way ANOVA followed by post hoc Tukey’s comparison for different groups at day 8 from 7-month-old mice. Performance was assessed by proximity. Red-bold values are p < 0.05
Main effect on day 8: [F(3,35)=3.72, p = 0.02] | ||||
Group | estimate | t-ratio | p | |
1 | Overtrained APPtg versus Overtrained C57BL/6J | –1.05 | –0.34 | 0.98 |
2 | Overtrained APPtg versus trained APPtg | –8.66 | –2.79 | 0.04 |
3 | Overtrained C57BL/6J versus trained C57BL/6J | 0.21 | 0.07 | 0.99 |
4 | Trained APPtg versus trained C57BL/6J | 7.83 | 2.67 | 0.052 |
Table 6
Summary table of proximity values of different groups at the first day and the last day of a WM task and a WM reversal task. SD is standard deviation
![]() |
In WM reversal task, we found that all groups quickly learned the task as early as by the second day (day 11) as proximity values hovered around 30 cm (Fig. 6a, b and Supplementary Figure 3a-c, respectively). Similarly, we did not find any significant group difference during training trials [F(3,34)=0.87, p = 0.46] (Fig. 6a, Supplementary Figure 3a-c, respectively). However, the overtrained groups performed worse as compared to trained groups on the first day (day 10) (Fig. 6b). Similarly, we assessed spatial memory during the probe trial on day 15 and found that the performance worsened for all groups as proximity and % age time spent on target quadrant hovered around 40 cm and 25%, respectively. In addition, one-way ANOVA analysis did not show any difference between groups (Fig. 6c and Supplementary Figure 3d). Thus, the probe trial assessment indicates that the cognitive training did not help improve the cognitive flexibility. At the end of the WM reversal task, we performed visual cue trials and showed that both latency and speed were comparable in all groups (Fig. 6d).
One important observation we found in WM reversal task was that all groups showed comparable performance at the end of the training trials (Fig. 6a, Supplementary Fig. 3a-c, respectively, and Table 6). However, we did not find improvement in a probe trial (Fig. 6c and Supplementary Figure 3d). In order to understand the causality behind this contradictory result, we assessed search strategies of each group. Our particular interest was the evolution of spatial search strategy during a normal WM task and a WM reversal task. As shown in Figure 7b, the spatial search strategy gradually increased as a function of training trials in both trained C57BL/6J and trained APPtg. In Set-up II, the spatial search dominated in the overall strategy for overtrained mice from day 4 onwards (Fig. 7b). By day 8, both overtrained C57BL/6J and overtrained APPtg exploited spatial search in 60% of total training trials (Fig. 7b).
Fig.7
Percentage-stacked-area plot of different strategies adopted by different groups of 7-month-old mice. a) Schema showing the platform position during the WM task and WM reversal task. b) and c) strategies adopted by mice as a function of training trials during the WM task and WM reversal task.
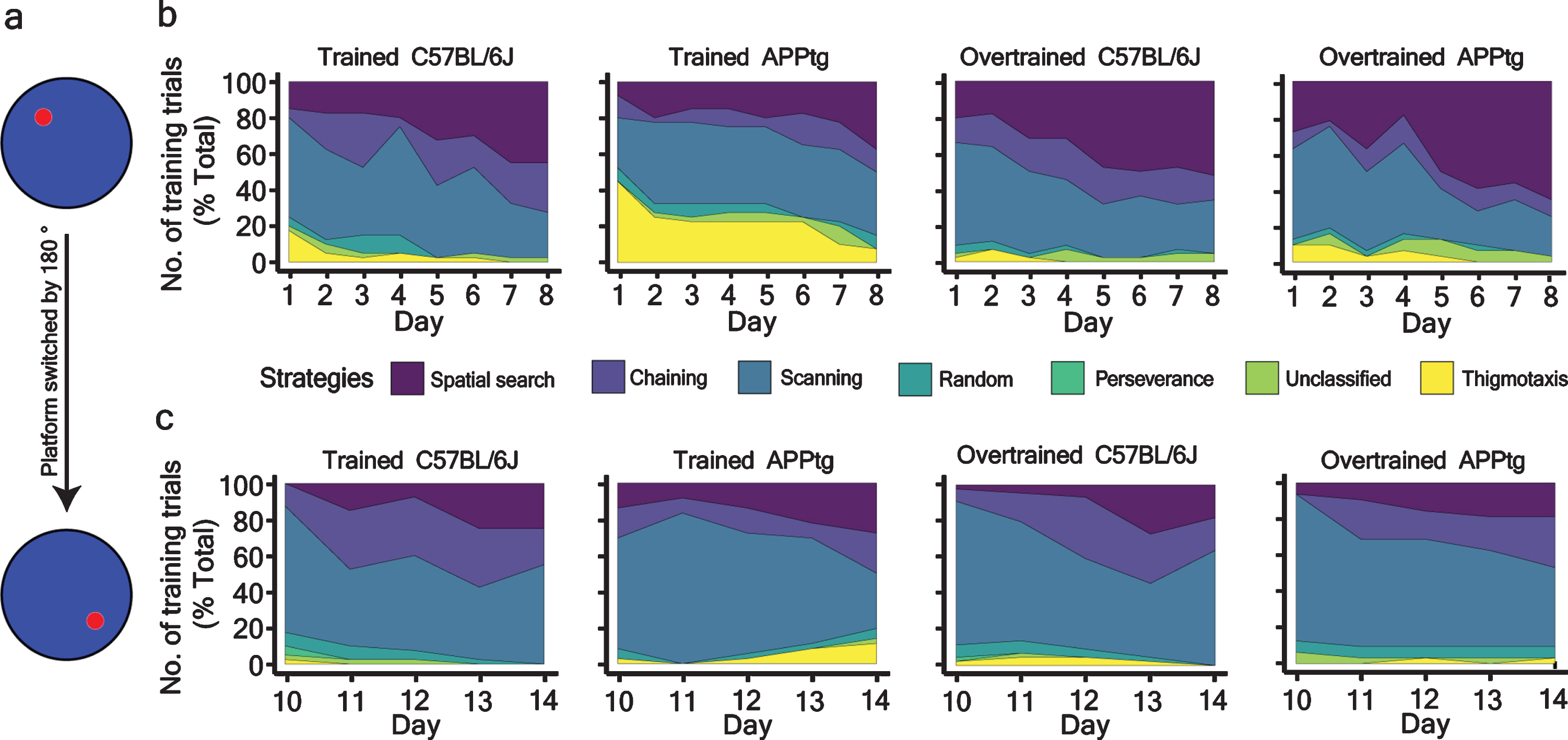
When the platform position had been changed for the WM reversal task, the dominance of spatial search was taken over by non-spatial search (chaining, scanning, random) as all groups of mice employed non-spatial search in more than 80% of total training trials irrespective of the training day (Fig. 7c). This search strategy showed that mice can successfully complete the WM task using non-spatial search strategies and corroborates previous findings that rodents can learn the WM task using egocentric (non-spatial) navigation as well [36, 37].
Cognitive training facilitates spontaneous recovery of reactivated spatial memory
One logical explanation behind the reappearance of non-spatial search strategies during the WM reversal task is that mice retain memory of the previous platform position. Therefore, we assessed the average number of visits of the previous target area as a function of training day. In fact, cognitive overtraining mainly affected the first day of WM reversal task as mice from Set-up II (overtrained) groups had more previous target crossing (PTC) on the first day, i.e., day 10 (Fig. 8a). Later, PTC became comparable with its normal trained counterparts from third day onwards (Fig. 8a). ANOVA of a mixed model of repeated measures showed significant group difference [F(3,34)=3.81, p = 0.01].
Fig.8
Previous target crossing in the WM reversal task. a) Memory retention of the previous task was assessed as previous target area crossing as a function of training day. ANOVA of a mixed model showed a significant group difference [F(3,34)=3.81, p = 0.01]. During pairwise comparison between groups, only overtrained C57BL/6J versus trained APPtg comparison gave significant difference (b = 0.54, SE = 0.17, t(34)=3.05, p = 0.02). Similarly, pairwise comparison between groups on day 10 (first day of WM reversal task) also showed significant group differences [overtrained APPtg versus trained APPtg (b = 0.43, SE = 0.36, t(34)=1.19, p = 0.63); overtrained APPtg versus trained C57BL/6J (b=-0.34, SE = 0.35, t(34)=-0.96, p = 0.76); overtrained C57BL/6J versus trained APPtg (b = 1.89, SE = 0.33, t(34)=5.61, p < 0.0001); overtrained C57BL/6J versus overtrained APPtg (b=–1.45, SE = 0.34, t(34)=-4.18, p = 0.001); overtrained C57BL/6J versus trained C57BL/6J (b = 1.11, SE = 0.32, t(34)=3.39, p = 0.009); and trained APPtg versus trained C57BL/6J (b=-0.77, SE = 0.34, t(34)=-2.25, p = 0.12)]. b) Hierarchical clustering of different training groups in the WM reversal task. Each row represents the training group, and each column represents the training trials. Training groups were sorted row-wise by similarity. Dendrogram represents similarity between different training groups. b, estimate; SE, standard error; t, t-ratio; p, p-value. Values are mean±95% confidence interval.
![Previous target crossing in the WM reversal task. a) Memory retention of the previous task was assessed as previous target area crossing as a function of training day. ANOVA of a mixed model showed a significant group difference [F(3,34)=3.81, p = 0.01]. During pairwise comparison between groups, only overtrained C57BL/6J versus trained APPtg comparison gave significant difference (b = 0.54, SE = 0.17, t(34)=3.05, p = 0.02). Similarly, pairwise comparison between groups on day 10 (first day of WM reversal task) also showed significant group differences [overtrained APPtg versus trained APPtg (b = 0.43, SE = 0.36, t(34)=1.19, p = 0.63); overtrained APPtg versus trained C57BL/6J (b=-0.34, SE = 0.35, t(34)=-0.96, p = 0.76); overtrained C57BL/6J versus trained APPtg (b = 1.89, SE = 0.33, t(34)=5.61, p < 0.0001); overtrained C57BL/6J versus overtrained APPtg (b=–1.45, SE = 0.34, t(34)=-4.18, p = 0.001); overtrained C57BL/6J versus trained C57BL/6J (b = 1.11, SE = 0.32, t(34)=3.39, p = 0.009); and trained APPtg versus trained C57BL/6J (b=-0.77, SE = 0.34, t(34)=-2.25, p = 0.12)]. b) Hierarchical clustering of different training groups in the WM reversal task. Each row represents the training group, and each column represents the training trials. Training groups were sorted row-wise by similarity. Dendrogram represents similarity between different training groups. b, estimate; SE, standard error; t, t-ratio; p, p-value. Values are mean±95% confidence interval.](https://ip.ios.semcs.net:443/media/jad/2020/75-4/jad-75-4-jad200161/jad-75-jad200161-g008.jpg)
Next, we performed hierarchical cluster analysis to observe the sorting effect of cognitive overtraining on different groups via PTC values. Figure 8b shows that cognitive training created two clusters: 1) one cluster consists of overtrained C57BL/6J, overtrained APPtg, and trained C57BL/6J and 2) another cluster consisted of trained APPtg. This finding corroborated our result as described in Fig. 5.
Since the previous target area and the starting position for the probe trial in the WM reversal task were located in the same quadrant, it is likely that mice could randomly visit the previous target area during a probe trial. Because of such experimental design, the generated PTC value could be error prone. Therefore, we performed a point pattern analysis of mice trajectories to create a spatial intensity map for every experimental group to observe their average x, y-position in the probe trial. We found that in Set-up I, localization hotspots were visible in the periphery of both platforms (former as well as current) in trained C57BL/6J as well as trained APPtg (Fig. 9b, c). In case of Set-up II, both overtrained C57BL/6J as well as overtrained APPtg showed a single localization hotspot in the periphery of the previous platform position (Fig. 9d, e). This demonstrates that cognitive overtraining strengthened the memory reactivation/reconsolidation as well as facilitated the spontaneous recovery of reactivated memory (Fig. 9d, e). Interestingly, it also shows that the cognitive overtraining reduces the cognitive flexibility/plasticity.
Fig.9
Spatial intensity (rate) map of different experimental groups in probe trial during the normal WM task and the WM reversal task respectively in 7-month-old mice. Left, accumulated mice trajectories per group (blue lines) with indicated platform position (red colored circle). Right, color-coded spatial rate map with peak rate. a) Platform position during a probe trial of normal WM and WM reversal tasks. A single probe trial was conducted before normal training trials on day 4, 6, 9, and 15. b-e) Mice trajectories and its corresponding spatial intensity map on probe trial on the different days of a normal WM task as well on the WM reversal task.
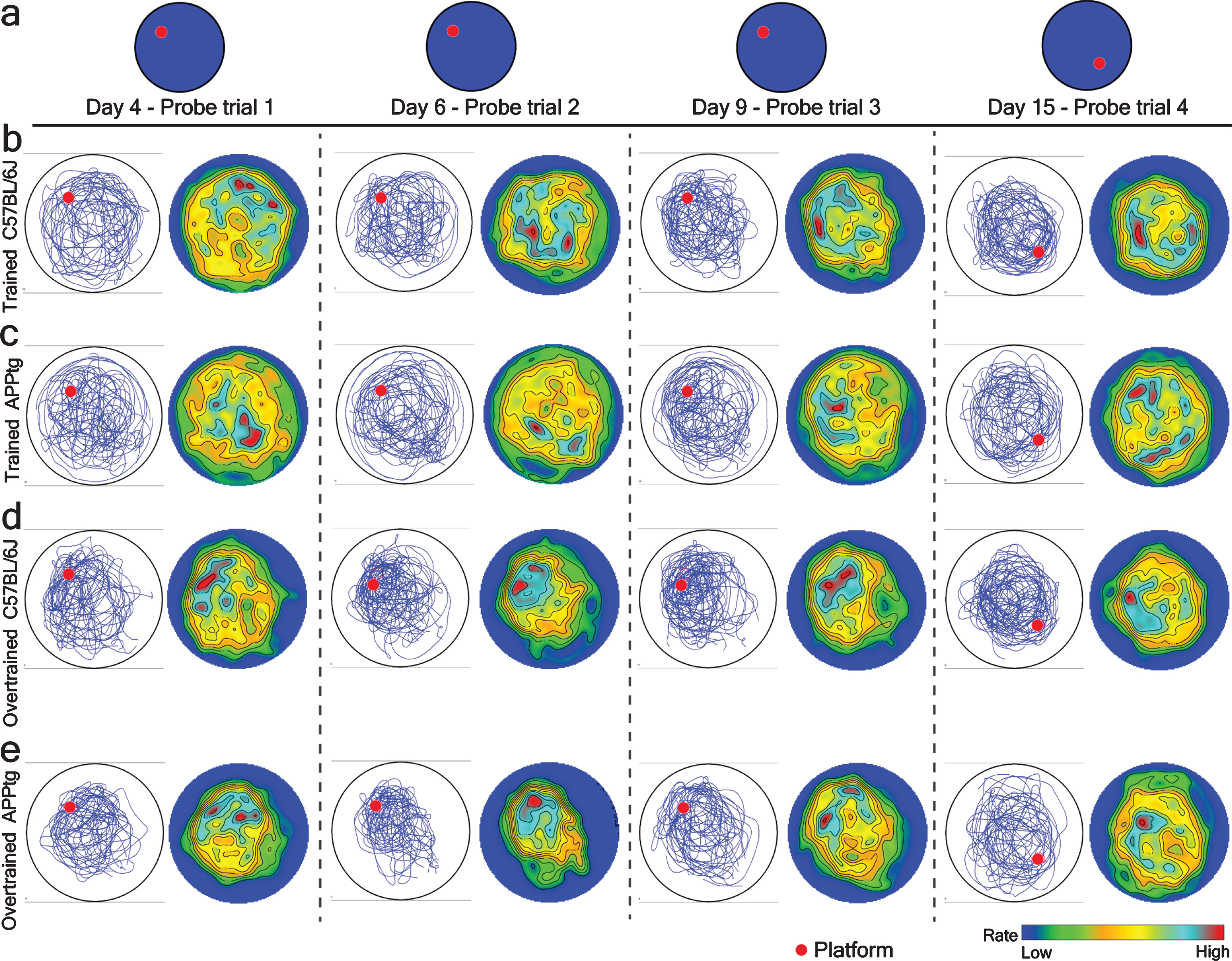
DISCUSSION
In this study, we found in APPtg mice that cognitive training (overtraining) not only improved the recall of remote spatial memory but also improved the spatial memory reconsolidation. Multiple studies show that lifestyle interventions such as environmental enrichment and exercise rescue spatial learning and memory in WM task [38, 39]. However, some studies have also reported that exercise does not improve the spatial learning and memory in AD mice [40]. Rather, environmental enrichment improves the WM performance of AD mice [41].
APP/PS1-21 female hemizygous mice had been used in this study because of early onset of disease pathology and less variability in Aβ levels [1]. Aβ accumulation starts as early as 6 weeks in the cortex and by 3 months, Aβ begins to appear in hippocampus [1]. The APP/PS1-21 mice show age-dependent progressive neuropathological features that cause cognitive impairment. It was also reported that spatial memory dysfunction appears from 6.5 months of age in WM task [4].
Limited studies have been performed to study the remote memories in rodents. Ramos et al. spatially overtrained rats in radial arm maze for 40 days (training starts from postnatal 28 days and continued for 5 days a week for a total of 8 weeks, with eight daily trials) [23]. After one day or seventy days following the last training trial (RI), bilateral dorsal hippocampi were damaged. When memory was assessed, dysfunction in memory reactivation was observed in both cases [23]. Similarly, in a WM task, both recent and remote memories are reported to be severely impaired with the loss of temporally graded retrograde amnesia after hippocampal damage [21, 22]. Jiang and colleagues [5] studied the fear-related remote memory in Tg2576-AD mice by cognitive overtraining using a WM task. The cognitive training was conducted at 8 months of age and assessment of remote memory was conducted at 9 months with an RI of 28 days. They found that spatial overtraining of Tg2576-AD mice rescued the fear-related remote memory [5].
For the first time, we here assess the impact of spatial training on remote spatial memory in an AD mouse model. In Set-up I, we started training mice at 3 months (first appearance of Aβ in hippocampus) and assessed remote spatial memory as well as WM performance at 7 months of age. In Set-up II, we performed early and extensive training of mice. Here, we started training animals at 2 months of age (before the appearance of Aβ plaques in hippocampus), again retrained at 3 months, and finally assessed remote spatial memory at 7 months. In both Set-ups, the RI was 4 months. We found that overtraining of mice (C57BL/6J and APPtg) rescued forgetting of remote spatial memory (Tables 2–4).
We are not aware of a study that revealed memory reactivation dysfunction at this early age in any AD mouse model. The memory reactivation of a spatial memory was previously studied on 16-month-old PDAPP mice (Alzheimer’s mouse model). Daumas and colleagues [9] trained PDAPP mice using a WM task so that they showed equivalent level of WM performance at the end of the task. When they retrained after 1.75 months of RI, PDAPP just needed four retraining trials to reactivate the spatial memory to an equivalent level of wild-type mice [9]. However, another study was conducted using APP/PS1-21 mice, which revealed a dysfunction of memory reactivation at 11 months of age [4]. Using the same AD mouse model (APP/PS1-21 mice), we, however, observed a dysfunction of spatial memory reactivation as early as 7 months of age (Fig. 3). Additionally, we also found that this reactivation dysfunction could be mitigated via cognitive overtraining (Fig. 4 and Table 4).
A WM reversal task involves the relocation of the platform to another quadrant [42] and initiates the reversal learning. The reversal learning requires extinction of the previous memory [43]. Extinction is suppression of a response in absence of a reinforcer [44]. The classic example is the extinction of a previous spatial memory in a WM task by performing probe trials multiple times. During extinction of spatial memory, the triangulation between the platform position and the distal cues is severed [45]. It has also been shown that extinction does not involve the deletion of memory established during acquisition [46] as it does not involve protein synthesis in the CA1 region of the hippocampus.
The important application of the WM reversal task is the study of the previous spatial memory via memory extinction. For example, spontaneous recovery of a previous memory was first demonstrated in mice by Lattel et al. in 2003 [47]. Similarly, a virtual WM reversal task in human also shows spontaneous recovery of previous memory [43]. This spontaneous recovery of previous memory, however, depends upon parameters such as the frequency of reinforcers [47] and incomplete extinction [48]. Using rats as model system, Rossato and colleagues [48] found that during incomplete extinction, the retrieval of original memory induces hippocampal protein synthesis indicating the role of hippocampus in retrieval.
In our study, the high proximity or low % age time spent on target quadrant values of all groups during the probe trial after the WM reversal task (Fig. 6c and Supplementary Figure 3d) is an indication that extinction was incomplete. In such scenario, we expected to observe the spontaneous recovery of previous memory in all groups based on Rossato and colleagues. Both studies [47, 48] used percentage time spent in the respective quadrant area as a measure of memory of previous and current memory. A spatial intensity/rate map, however, estimates the pixel counts of mouse trajectories in x and y coordinates of the water maze pool, hence, it might be more precise as compared to findings based on conventional measures. Using point pattern analysis of mice trajectories, our study showed that trained C57BL/6J and trained APPtg mice tend to show affinity for both previous platform area (previous WM task) as well as new platform area (WM reversal task) (Fig. 9b, c). However, when cognitive overtraining was performed, a spontaneous recovery of previous memory dominated not only for C57BL/6J mice, but also for APPtg mice (Fig. 9d, e). Thus, our findings show that spontaneous recovery of the previous and current memory appear discretely during incomplete extinction (Fig. 9b, c) which contradicts previous studies. This finding, therefore, provides an important update in the current understanding of spontaneous recovery of memory. Similarly, it can also be useful to study cognitive stimulation paradigms to preserve memory in AD patients as we showed in APPtg mice that subjects with cognitive overtraining can perform cognitively normal despite Aβ pathology.
Cognitive reserve and cognitive training via WM task
In the Nun Study, Snowdon et al. showed that the severity of brain β-amyloidosis and the clinical manifestation does not follow a one-by-one relationship, and that the clinical effects of AD can be averted despite high Aβ load [6]. Low linguistic ability in early life increases the risk of dementia [6], while intelligence lowers the risk [8] indicating the importance of cognitive training and intervention to lower cognitive dysfunction in elderly [8]. These epidemiological studies corroborate a cognitive reserve, a hypothesized protective system of the adult brain that minimizes cognitive decline [49]. Recently, the AlzForum [50] highlighted the issues of intelligence and education in relation with the likelihood of developing AD demanding new approaches to study and to prevent AD.
Physical exercise intervention shows an improvement or rescue of cognitive dysfunction in AD mouse models [40] with one exception [41]. Measures such as active lifestyle, education, or physical exercise help to rescue the cognitive function in elderly [40]. Similarly, earlier report from Nun Study points possibility of the positive association between the genetic risk factor such as ɛ4 allele of apolipoprotein E (APOE ɛ4) and dementia [51]. In this unfavorable marriage, a review shows several studies indicating positive interaction between APOE ɛ4 and gender [52]. Specifically, cognitive dysfunction is more pronounced in female with heterozygous APOE ɛ4 than in male counterparts [52]. Among homozygotes, however, the interaction is more pronounced in male than in female [53], indicating that APOE ɛ4 associated cognitive decline varies with gender and zygosity of APOE ɛ4 [54]. A study shows that certain lifestyle (education) has the protective effect on the cognitive function of elderly despite being a carrier of APOE ɛ4 [55].
There has not been any study conducted so far regarding the impact of cognitive overtraining in the rescue of remote spatial memory as well as reactivation of spatial memory. Therefore, we performed this study and showed that cognitive overtraining helps rescue these aspects of cognitive dysfunction in an AD mouse model.
This may be the first empirical evidence in mice that indicates the importance of cognitive overtraining in preserving a cognitive reserve: early cognitive training can prevent/decelerate cognitive dysfunction. This finding further suggests that the lack of cognitive training has a causal relation to later cognitive dysfunction. Cognitive overtraining, however, seems to be playing a double-edged role. During the probe trial on day 15 of the WM reversal task (Fig. 6c and Supplementary Figure 3d), all groups performed worst (Fig. 6c and Supplementary Figure 3d) indicating an inverse relationship between cognitive training and cognitive flexibility. Further studies are needed to elucidate the relationship between cognitive overtraining and neuronal plasticity.
In summary, the preservation of the cognitive performance of a learnt task even with severe β-amyloidosis in mice may be used as an indication on how to prevent habituation difficulties of elderly to new and foreign environments, e.g., nursery homes.
ACKNOWLEDGMENTS
We would like to thank Ivan Eiriz and Thomas Brüning for technical support.
The work of J.P. was supported by the following grants: Deutsche Forschungsgemeinschaft, Germany (DFG PA930/12); Wirtschaftsministerium Sachsen-Anhalt, Germany (EFRE, ZS/2016/05/78617); Leibniz Association, Germany (Leibniz-Wettbewerb SAW-2015-IPB-2); Latvian Council of Science FLPP, Latvia (lzp-2018/1-0275); Nasjonalforeningen, Norway (16154); HelseSØ, Norway (2016062, 2019054, 2019055); Norsk forskningsrådet, Norway (251290 FRIMEDIO, 260786 PROP-AD); Horizon 2020, European Union (643417 (PROP-AD)).
PROP-AD is an EU Joint Programme - Neurodegenerative Disease Research (JPND) project. The project is supported through the following funding organizations under the aegis of JPND (http://www.jpnd.eu). This project has received funding from the European Union’s Horizon 2020 research and innovation program under grant agreement #643417 (JPco-fuND).
Authors’ disclosures available online (https://www.j-alz.com/manuscript-disclosures/20-0161r1).
SUPPLEMENTARY MATERIAL
[1] The supplementary material is available in the electronic version of this article: https://dx.doi.org/10.3233/JAD-200161.
REFERENCES
[1] | Radde R , Bolmont T , Kaeser SA , Coomaraswamy J , Lindau D , Stoltze L , Calhoun ME , Jaggi F , Wolburg H , Gengler S , Haass C , Ghetti B , Czech C , Holscher C , Mathews PM , Jucker M ((2006) ) Abeta42-driven cerebral amyloidosis in transgenic mice reveals early and robust pathology. EMBO Rep 7: , 940–946. |
[2] | Richard BC , Kurdakova A , Baches S , Bayer TA , Weggen S , Wirths O ((2015) ) Gene dosage dependent aggravation of the neurological phenotype in the 5XFAD mouse model of Alzheimer’s disease. J Alzheimers Dis 45: , 1223–1236. |
[3] | Ohno M ((2009) ) Failures to reconsolidate memory in a mouse model of Alzheimer’s disease. Neurobiol Learn Mem 92: , 455–459. |
[4] | Serneels L , Van Biervliet J , Craessaerts K , Dejaegere T , Horre K , Van Houtvin T , Esselmann H , Paul S , Schafer MK , Berezovska O , Hyman BT , Sprangers B , Sciot R , Moons L , Jucker M , Yang Z , May PC , Karran E , Wiltfang J , D’Hooge R , De Strooper B ((2009) ) gamma-Secretase heterogeneity in the Aph1 subunit: relevance for Alzheimer’s disease. Science 324: , 639–642. |
[5] | Jiang X , Chai GS , Wang ZH , Hu Y , Li XG , Ma ZW , Wang Q , Wang JZ , Liu GP ((2015) ) Spatial training preserves associative memory capacity with augmentation of dendrite ramification and spine generation in Tg2576 mice. Sci Rep 5: , 9488. |
[6] | Snowdon DA , Kemper SJ , Mortimer JA , Greiner LH , Wekstein DR , Markesbery WR ((1996) ) Linguistic ability in early life and cognitive function and Alzheimer’s disease in late life. Findings from the Nun Study. JAMA 275: , 528–532. |
[7] | Huang AR , Strombotne KL , Horner EM , Lapham SJ ((2018) ) Adolescent cognitive aptitudes and later-in-life Alzheimer disease and related disorders. JAMA Netw Open 1: , e181726. |
[8] | Anderson EL , Howe LD , Wade KH , Ben-Shlomo Y , Hill WD , Deary IJ , Sanderson EC , Zheng J , Korologou-Linden R , Stergiakouli E ((2018) ) Education, intelligence and Alzheimer’s disease: Evidence from a multivariable two-sample Mendelian randomization study. bioRxiv 401042. |
[9] | Daumas S , Sandin J , Chen KS , Kobayashi D , Tulloch J , Martin SJ , Games D , Morris RG ((2008) ) Faster forgetting contributes to impaired spatial memory in the PDAPP mouse: deficit in memory retrieval associated with increased sensitivity to interference? Learn Mem 15: , 625–632. |
[10] | McGaugh JL ((2000) ) Memory–a century of consolidation. Science 287: , 248–251. |
[11] | Kandel ER ((2001) ) The molecular biology of memory storage: a dialogue between genes and synapses. Science 294: , 1030–1038. |
[12] | Bontempi B , Laurent-Demir C , Destrade C , Jaffard R ((1999) ) Time-dependent reorganization of brain circuitry underlying long-term memory storage. Nature 400: , 671–675. |
[13] | MacLeod S , Reynolds MG , Lehmann H ((2018) ) The mitigating effect of repeated memory reactivations on forgetting. NPJ Sci Learn 3: , 9. |
[14] | Nader K ((2003) ) Memory traces unbound. Trends Neurosci 26: , 65–72. |
[15] | Maviel T , Durkin TP , Menzaghi F , Bontempi B ((2004) ) Sites of neocortical reorganization critical for remote spatial memory. Science 305: , 96–99. |
[16] | Suzuki A , Josselyn SA , Frankland PW , Masushige S , Silva AJ , Kida S ((2004) ) Memory reconsolidation and extinction have distinct temporal and biochemical signatures. J Neurosci 24: , 4787–4795. |
[17] | Debiec J , LeDoux JE , Nader K ((2002) ) Cellular and systems reconsolidation in the hippocampus. Neuron 36: , 527–538. |
[18] | Eisenberg M , Kobilo T , Berman DE , Dudai Y ((2003) ) Stability of retrieved memory: inverse correlation with trace dominance. Science 301: , 1102–1104. |
[19] | Sara SJ ((2000) ) Retrieval and reconsolidation: toward a neurobiology of remembering. Learn Mem 7: , 73–84. |
[20] | Hardt O , Nader K , Nadel L ((2013) ) Decay happens: the role of active forgetting in memory. Trends Cogn Sci 17: , 111–120. |
[21] | Clark RE , Broadbent NJ , Squire LR ((2005) ) Hippocampus and remote spatial memory in rats. Hippocampus 15: , 260–272. |
[22] | Clark RE , Broadbent NJ , Squire LR ((2007) ) The hippocampus and spatial memory: findings with a novel modification of the water maze. J Neurosci 27: , 6647–6654. |
[23] | Ramos JM ((2009) ) Remote spatial memory and the hippocampus: effect of early and extensive training in the radial maze. Learn Mem 16: , 554–563. |
[24] | Paarmann K , Prakash SR , Krohn M , Mohle L , Brackhan M , Bruning T , Eiriz I , Pahnke J ((2019) ) French maritime pine bark treatment decelerates plaque development and improves spatial memory in Alzheimer’s disease mice. Phytomedicine 57: , 39–48. |
[25] | Ruediger S , Spirig D , Donato F , Caroni P ((2012) ) Goal-oriented searching mediated by ventral hippocampus early in trial-and-error learning. Nat Neurosci 15: , 1563–1571. |
[26] | Young ME , Clark MH , Goffus A , Hoane MR ((2009) ) Mixed effects modeling of Morris water maze data: Advantages and cautionary notes. Learn Motiv 40: , 160–177. |
[27] | Tomas Pereira I , Burwell RD ((2015) ) Using the spatial learning index to evaluate performance on the water maze. Behav Neurosci 129: , 533–539. |
[28] | Gallagher M , Burwell R , Burchinal M ((2015) ) Severity of spatial learning impairment in aging: Development of a learning index for performance in the Morris water maze. Behav Neurosci 129: , 540–548. |
[29] | Chen G , Chen KS , Knox J , Inglis J , Bernard A , Martin SJ , Justice A , McConlogue L , Games D , Freedman SB , Morris RG ((2000) ) A learning deficit related to age and beta-amyloid plaques in a mouse model of Alzheimer’s disease. Nature 408: , 975–979. |
[30] | Ogle D , Wheeler P , Dinno A ((2018) ) FSA: fisheries stock analysis. R package version 0.8. 20. Chapman & Hall/CRC, Boca Raton. |
[31] | Gu Z , Eils R , Schlesner M ((2016) ) Complex heatmaps reveal patterns and correlations in multidimensional genomic data. Bioinformatics 32: , 2847–2849. |
[32] | Galili T ((2015) ) dendextend: an R package for visualizing, adjusting and comparing trees of hierarchical clustering. Bioinformatics 31: , 3718–3720. |
[33] | Baddeley A , Rubak E , Turner R ((2015) ) Spatial point patterns: methodology and applications with R, Chapman and Hall/CRC. |
[34] | Gelfand AE , Diggle PJ , Fuentes M , Guttorp P ((2010) ) Handbook of spatial statistics. Chapman & Hall/CRC, Boca Raton, FL. |
[35] | Baddeley A , Turner R ((2005) ) spatstat: AnRPackage for analyzing spatial point patterns. J Stat Softw 12: , doi: 10.18637/jss.v012.i06. |
[36] | Knierim JJ , Hamilton DA ((2011) ) Framing spatial cognition: neural representations of proximal and distal frames of reference and their roles in navigation. Physiol Rev 91: , 1245–1279. |
[37] | Hamilton DA , Akers KG , Weisend MP , Sutherland RJ ((2007) ) How do room and apparatus cues control navigation in the Morris water task? Evidence for distinct contributions to a movement vector. J Exp Psychol Anim Behav Process 33: , 100–114. |
[38] | Cho J , Shin MK , Kim D , Lee I , Kim S , Kang H ((2015) ) Treadmill running reverses cognitive declines due to Alzheimer disease. Med Sci Sports Exerc 47: , 1814–1824. |
[39] | Garcia-Mesa Y , Pareja-Galeano H , Bonet-Costa V , Revilla S , Gomez-Cabrera MC , Gambini J , Gimenez-Llort L , Cristofol R , Vina J , Sanfeliu C ((2014) ) Physical exercise neuroprotects ovariectomized 3xTg-AD mice through BDNF mechanisms. Psychoneuroendocrinology 45: , 154–166. |
[40] | Shepherd A , Zhang TD , Zeleznikow-Johnston AM , Hannan AJ , Burrows EL ((2018) ) Transgenic mouse models as tools for understanding how increased cognitive and physical stimulation can improve cognition in Alzheimer’s disease. Brain Plast 4: , 127–150. |
[41] | Wolf SA , Kronenberg G , Lehmann K , Blankenship A , Overall R , Staufenbiel M , Kempermann G ((2006) ) Cognitive and physical activity differently modulate disease progression in the amyloid precursor protein (APP)-23 model of Alzheimer’s disease. Biol Psychiatry 60: , 1314–1323. |
[42] | Vorhees CV , Williams MT ((2006) ) Morris water maze: procedures for assessing spatial and related forms of learning and memory. Nat Protoc 1: , 848–858. |
[43] | Luna D , Martínez H ((2015) ) Spontaneous recovery of human spatial memory in a virtual water maze. Psicologica 36: , 283–308. |
[44] | Myers KM , Davis M ((2002) ) Behavioral and neural analysis of extinction. Neuron 36: , 567–584. |
[45] | Lattal KM , Abel T ((2001) ) Different requirements for protein synthesis in acquisition and extinction of spatial preferences and context-evoked fear. J Neurosci 21: , 5773–5780. |
[46] | Bouton ME ((1993) ) Context, time, and memory retrieval in the interference paradigms of Pavlovian learning. Psychol Bull 114: , 80–99. |
[47] | Lattal KM , Mullen MT , Abel T ((2003) ) Extinction, renewal, and spontaneous recovery of a spatial preference in the water maze. Behav Neurosci 117: , 1017–1028. |
[48] | Rossato JI , Bevilaqua LR , Medina JH , Izquierdo I , Cammarota M ((2006) ) Retrieval induces hippocampal-dependent reconsolidation of spatial memory. Learn Mem 13: , 431–440. |
[49] | Stern Y ((2002) ) What is cognitive reserve? Theory and research application of the reserve concept. J Int Neuropsychol Soc 8: , 448–460. |
[50] | Dickey Zakaib G ((2018) ) Intelligence matters more for brain reserve, but education helps. Alzforum, https://www.alzforum.org/news/research-news/intelligence-matters-more-brain-reserve-education-helps |
[51] | Riley KP , Snowdon DA , Saunders AM , Roses AD , Mortimer JA , Nanayakkara N ((2000) ) Cognitive function and apolipoprotein E in very old adults: findings from the Nun Study. J Gerontol B Psychol Sci Soc Sci 55: , S69–75. |
[52] | Ungar L , Altmann A , Greicius MD ((2014) ) Apolipoprotein E, gender, and Alzheimer’s disease: an overlooked, but potent and promising interaction. Brain Imaging Behav 8: , 262–273. |
[53] | Lehmann DJ , Refsum H , Nurk E , Warden DR , Tell GS , Vollset SE , Engedal K , Nygaard HA , Smith AD ((2006) ) Apolipoprotein E epsilon4 and impaired episodic memory in community-dwelling elderly people: a marked sex difference. The Hordaland Health Study. J Neurol Neurosurg Psychiatry 77: , 902–908. |
[54] | Swan GE , Lessov-Schlaggar CN , Carmelli D , Schellenberg GD , La Rue A ((2005) ) Apolipoprotein E epsilon4 and change in cognitive functioning in community-dwelling older adults. J Geriatr Psychiatry Neurol 18: , 196–201. |
[55] | de Oliveira FF , de Almeida SS , Chen ES , Smith MC , Naffah-Mazzacoratti MDG , Bertolucci PHF ((2018) ) Lifetime risk factors for functional and cognitive outcomes in patients with Alzheimer’s disease. J Alzheimers Dis 65: , 1283–1299. |