Staging Alzheimer’s Disease Risk by Sequencing Brain Function and Structure, Cerebrospinal Fluid, and Cognition Biomarkers
Abstract
This study aims to develop a composite biomarker that can accurately measure the sequential biological stages of Alzheimer’s disease (AD) on an individual level. We selected 144 subjects from the Alzheimer’s Disease Neuroimaging Initiative 2 datasets. Ten biomarkers, from brain function and structure, cerebrospinal fluid, and cognitive performance, were integrated using the event-based probabilistic model to estimate their optimal temporal sequence (Soptimal). We identified the numerical order of the Soptimal as the characterizing Alzheimer’s disease risk events (CARE) index to measure disease stage. The results show that, in the Soptimal, hippocampal and posterior cingulate cortex network biomarkers occur first, followed by aberrant cerebrospinal fluid amyloid-β and p-tau levels, then cognitive deficit, and finally regional gray matter loss and fusiform network abnormality. The CARE index significantly correlates with disease severity and exhibits high reliability. Our findings demonstrate that use of the CARE index would advance AD stage measurement across the whole AD continuum and facilitate personalized treatment of AD.
INTRODUCTION
Current literature regards sporadic Alzheimer’s disease (AD) as a clinical entity arising from a series of pathophysiological events related to amyloidosis and neurodegeneration. These events, measured by corresponding AD biomarkers, are believed to occur in a temporally ordered manner along with disease progression [1]; however, a detailed sequence remains ambiguous. Disentangling the temporal relationship among AD biomarkers provides insight into the evolution of AD pathogenesis. From a clinical perspective, an established AD biomarkers sequence would provide a template for defining an individual’s AD stage and dictating stage-dependent therapeutic strategies, especially with regard to facilitating secondary prevention of AD [2]. Therefore, estimating the optimal temporal order (Soptimal) of AD biomarkers is essential in uncovering AD development processes and designing effective treatment strategies.
After decades of research, we now understand AD development to be complex in nature, with a series of causal sequences between pathologies and functions. An earlier concept recognized amyloid-β (Aβ) deposition as the earliest AD trigger, causing downstream neurodegeneration and cognitive deficit in turn [3]. However, this linear pathway concept appears to be flawed given that Aβ removal has proven ineffective in improving clinical outcomes [4, 5]. Recent studies indicate several neurodegenerative biomarkers arise upstream in AD. Specifically, neural dysfunction would induce Aβ pathologies [6], and the soluble Aβ peptides can further exacerbate neural dysfunction before fibrillar Aβ deposits [7]. This corroborates observations of aberrant hippocampal hyperactivity and default mode network (DMN) hypoconnectivity or hypometabolism in apolipoprotein E (APOE) ɛ4 carriers without detectable Aβ deposition [8–10]. Tau pathology also is required in mediating Aβ toxicity [11]. These diverse pathophysiological events constitute the temporal-dependent process underlying AD development [4]. Our study focused on determining the Soptimal of those pathophysiological events represented by corresponding dynamic biomarkers, in order to accurately stage each individual across the whole AD spectrum.
Three major technical challenges impede the determination of the Soptimal among AD biomarkers. First, the conventional symptom-based group definition involves biologically heterogeneous populations and, therefore, poses a great challenge for disease staging [12]. Second, the dichotomizations of biomarker values by “cut-off point” thresholds appear to deviate from the continuous nature of insidious AD progression. Also, the cut-off points are difficult to standardize across laboratories [13]. Third, the Soptimal determination among multiple biomarkers generally requires a large cohort with a long follow-up period to link preclinical to advanced AD stages, complicating study design and significantly raising costs. System biology is an emerging strategy to unravel temporal relationships among biomarkers and predict disease progression related to AD by modeling approaches [14], thus exhibiting great potential in addressing the above challenges. Specifically, the event-based probabilistic (EBP) model can learn the temporal order of biomarkers to describe disease progression from large cross-sectional datasets based on the Bayesian theory [15, 16]. The EBP model is a decision-making tool that determines the order of a complex system of events by estimating the probabilities of occurrence and nonoccurrence of a series of events, rather than by dichotomizing biomarker status based on the cut-off point threshold.
Our study extended the EBP model innovatively by integrating functional, structural, biofluid, and cognitive biomarkers to determine the Soptimal, which links the appearance of any specific biomarkers in asymptomatic individuals to the subsequent emergence of clinical symptomatology across the whole continuum of the AD development process.
MATERTIALS AND METHODS
Subject information
This study employed data from the Alzheimer’s Disease Neuroimaging Initiative 2 (ADNI 2) database. ADNI initially was launched in 2003 by the National Institute on Aging (NIA), the National Institute of Biomedical Imaging and Bioengineering (NIBIB), the US Food and Drug Administration [17], private pharmaceutical companies, and nonprofit organizations, as a $60 million, five-year public-private partnership. Michael W. Weiner, MD, from San Francisco Veterans Affairs Medical Center and University of California-San Francisco, is the principal investigator of ADNI. On October 3, 2014, we downloaded ADNI 2 datasets from the Laboratory of Neuro Imaging (LONI), which included 225 subjects with four groups of clinical diagnoses (cognitively normal [CN], early mild cognitive impairment [EMCI], late MCI [LMCI], and AD). Specific inclusion and exclusion criteria of the four groups are described in detail in the ADNI 2 procedures manual (http://adni.loni.usc.edu/wp-content/uploads/2008/07/adni2-procedures-manual.pdf). Specifically, the EMCI subjects exhibited a 1–1.5 standard deviation (SD) decline in neuropsychological memory performance, whereas the LMCI subjects’ decline was 1.5 SD or greater. Briefly, the EMCI inclusion criteria are described as follows: 1) subjective memory concern; 2) declined delayed recall scores in logical memory test (9–11 points for subjects with 16 or more education years, 5–9 points for subjects with 8–15 education years, and 3–6 points for subjects with 0–7 education years); 3) Mini-Mental State Examination (MMSE) scores between 24 and 30; 4) Clinical Dementia Rating score = 0.5; 5) normal general cognition. Of the 225 subjects total, we selected 144 subjects based on the following requirements: First, all subjects had at least one resting-state functional connectivity magnetic resonance imaging (R-fMRI) scan with corresponding anatomical scans. Second, all subjects had cerebrospinal fluid (CSF) Aβ and phosphorylated tau (p-tau) concentration values. Third, all subjects had scores on the MMSE, modified 13-item Alzheimer’s Disease Assessment Scale-Cognitive Subscale (ADAS-Cog), and Rey Auditory Verbal Learning Test (AVLT) (immediate recall score, i.e., the sum of trials 1 to 5). Together, the 144 subjects consisted of 45 CN, 42 EMCI, 32 LMCI, and 25 AD subjects (Table 1).
Imaging acquisition
The ADNI data acquisition process is described at http://adni.loni.usc.edu/. Briefly, R-fMRI datasets were scanned on 3.0 Tesla (T) magnetic resonance imaging [18] scanners (Philips, Netherlands). During the resting-state acquisitions, no specific cognitive tasks were performed, and the participants were instructed to relax with their eyes open inside the scanner. Axial R-fMRI images of the whole brain were obtained in seven minutes with a single-shot gradient echo planar imaging (EPI) sequence. High-resolution magnetization-prepared rapid gradient-echo (MP-RAGE) 3-D sagittal images also were acquired.
Resting-state image preprocessing
Conventional preprocessing steps were conducted using Analysis of Functional NeuroImages (AFNI) software (http://afni.nimh.nih.gov/afni/), SPM8 (Wellcome Trust, London, United Kingdom), and MATLAB (MathWorks, Natick, Massachusetts). The preprocessing allows for T1-equilibration (removing the first 15 seconds of R-fMRI data); slice-acquisition-dependent time shift correction (3dTshift); motion correction (3dvolreg); detrending (3dDetrend); despiking (3dDespike); spatial normalization (original space to the Montreal Neurological Institute [MNI] space, SPM8); averaging white matter and CSF signal retrieval (3dROIstats) using standard SPM white matter and CSF mask in the MNI space; white matter, CSF signal, and motion effect removal (3dDeconvolve); global signal removal necessity check (the global signal will be removed if necessary) [19]; and low-frequency band-pass filtering (3dFourier, 0.015–0.1 Hz).
Biomarkers
We selected 10 well-studied AD biomarkers from only three examinations: neuropsychological assessment, MRI scan, and CSF, each representing an event that occurs along with AD progression. These biomarkers include three region-basedR-fMRI functional connectivity indices (FCI) from the hippocampus (HIPFCI), posterior cingulate cortex (PCCFCI), and fusiform gyrus (FUSFCI); two gray matter concentration indices (GMI) from the hippocampus (HIPGMI) and fusiform gyrus (FUSGMI); two CSF biomarkers of Aβ and p-tau levels; and three cognitive markers of MMSE, ADAS-Cog (ADAS), and AVLT scores. Detailed methods to extract FCI and GMI indices are provided in the Supplementary Methods 1 and 2.
Event-based probabilistic model
The Soptimal is determined by the EBP model. The conceptual frameworks of the EBP model, initially developed and applied to study seriation in determining the temporal order of fossil occurrence in paleontology [20], were further developed to treat disease progression (e.g., AD) as a sequence of events in which different biomarkers become abnormal in a temporally ordered manner using cross-sectional datasets [16, 21]. The EBP model does not make any a priori assumptions about the sequence in which these biomarker events occur, except that the sequence is consistent for all subjects. Rather, the EBP model estimates the probability of the event sequences using real-world data. The mathematical detail of the EBP model is described in the Supplementary Methods 3–5. This study used 45 CN and 25 AD subjects to determine the Soptimal. Note that the EMCI and LMCI subjects were excluded in determining the Soptimal so that they could be used as an independent validation.
CARE index and individual AD risk stage
We numbered each of the 10 biomarker events by order of occurrence in the Soptimal; collectively, these events comprise the index for characterizing Alzheimer’s disease risk events (CARE), or “CARE Index.” Each individual’s AD risk stage is defined as that at which k had the highest likelihood value at the Soptimal in equation 6 (Supplementary Methods 6). Each individual’s k value corresponds to a score on the CARE index.
Statistical analysis
We used one-way analysis of variance (ANOVA) to compare demographic information and clinical data among the four clinically defined groups (45 CN, 42 EMCI, 32 LMCI, and 25 AD). Then, we applied ANOVA to detect differences in CARE index scores among the four groups. The sources of the among-group differences were further identified by post-hoc multiple comparison procedure. (For ANOVA, the statistical significance level was set at p< 0.05; for post-hoc comparisons, the statistical significance level was set at Tukey-Kramer corrected p< 0.05.) In addition, Auditory Verbal Learning Test-30-min delayed recall (AVLT30 min) scores were used to validate the association of the CARE index score with disease severity. Specifically, we employed multiple linear regression models to estimate the relationships between the CARE index score and AVLT30 min score in EMCI and LMCI groups separately. We also examined such a relationship across the four groups using a logistic model (Supplementary Methods 7).
RESULTS
Subject information
As shown in Table 1, the four groups had no significant difference in demographic information except education years (F = 2.93, p = 0.04). By contrast, the MMSE, ADAS-Cog, and AVLT scores, as well as the CSF Aβ and p-tau levels, exhibited significant differences among groups.
Distribution of each biomarker value
In all 10 plotted and fitted biomarker event distributions (Fig. 1), we imposed the condition that distributions with lower values be abnormal (i.e., event occurred) and distributions with higher values be normal (i.e., event did not occur). Therefore, the values for those biomarkers that defined a higher value as abnormal in nature, including the ADAS-Cog score, p-tau level, HIPFCI, and FUSFCI, were multiplied by (–1).
Soptimal of events
The Soptimal represented by the 10 biomarkers was obtained and is presented in Fig. 2A. The first two disease events are represented by the two functional biomarkers: increased HIPFCI (CARE index score 1) and decreased PCCFCI (CARE index score 2). The next two are CSF biomarkers: decreased Aβ (CARE index score 3) and increased p-tau (CARE index score 4). The subsequent events are a mix of cognitive biomarkers (decreased MMSE score [CARE index score 5], increased ADAS-Cog score [CARE index score 6], decreased HIPGMI [CARE index score 7]), decreased AVLT score [CARE index score 8], and decreased FUSGMI [CARE index score 9]). The last event is increased functional biomarker FUSFCI (CARE index score 10). Note that the optimal order of biomarker events (i.e., Soptimal), calculated from the CN and AD groups, has a perfect diagonal pattern in the matrix (Fig. 2A). This result, obtained from application of the modified k-mean Gaussian mixture model fitting and our new greedy algorithm, minimized event uncertainty. To estimate the uncertainty in the obtained Soptimal, we performed the bootstrap procedure, wherein we resampled the data 500 times; for each bootstrap sample, we reestimated the optimal sequence Soptimal. The uncertainty in the estimated optimal sequence is illustrated in Fig. 2B. We observed that the event sequence uncertainty primarily existed within three distinct event clusters: 1) the early event cluster, including HIPFCI and PCCFCI; 2) the middle event cluster, including CSF biomarkers, cognitive performance, and HIPGMI; and 3) the later event cluster, including FUSFCI and FUSGMI. Note that there is negligible overlap among these three event clusters.
Association of the CARE index with clinical stages
Using the order of the EBP model-based biomarker events, we obtained a CARE index score for each subject regardless of the subject’s clinical stage. As an example, we have selected four typical subjects, one from each of the CN, EMCI, LMCI, and AD groups, to illustrate the distribution of the normalized likelihoods at each position on the CARE index (Fig. 3A). The AD risk for each of the four subjects was determined by the position of the subject’s highest likelihood value on the CARE index, where a score of 1 is associated with a CN subject, 4 with an EMCI subject, 7 with an LMCI subject, and 9 with an AD subject. Moreover, the curve provides likelihood values at other CARE index scores, showing each subject’s risk of developing AD. For example, the LMCI subject had a relatively high likelihood value (close to 0.6) at CARE index scores 7, 8, and 9, in addition to the highest likelihood value (0.65) at CARE index score 6. This suggests that this LMCI subject has a high risk of progressing from LMCI to AD-type dementia. Information of this nature may facilitate individual clinical inference.
Specifically, we found that all but one of the CN subjects have a CARE index score less than or equal to 6, while all AD subjects have a CARE index score greater than or equal to 6 (Fig. 3B). Similarly, the CARE index scores for EMCI and LMCI groups were between those of the CN and AD groups (Fig. 3C). With regard to CARE index score differences among groups (Fig. 3D), the median CARE index scores of the CN, EMCI, LMCI, and AD groups were 2, 4, 6, and 9, respectively. The CN group exhibited a lower CARE index score than the EMCI (p < 0.005), LMCI (p < 0.5×10- 6), and AD (p < 0.1×10- 6) groups. The AD group showed a higher CARE index score than the EMCI (p < 0.5×10- 4) and LMCI (p < 0.5×10- 4) groups. In addition, the EMCI group showed a lower CARE index score than the LMCI (p < 0.02) group. Note that the correspondence between CARE index scores and clinical stages exists across all groups, including EMCI and LMCI subjects who were not involved in the estimation of Soptimal. Such consistency makes it possible to use the CARE index to estimate biomarker-based AD stages for individual subjects.
AVLT30 min scores correlated with CARE index scores
The degree of disease severity, represented by the AVLT30 min score, was significantly correlated with the CARE index score (Fig. 4). With regard to individual clinical groups, regression lines are significant for the EMCI (p≤0.0042, R2 = 0.19) and LMCI (p≤0.0166, R2 = 0.18) groups, shown in the left and middle panels of Fig. 4, respectively. The full nonlinear regression model is also significant (p≤1.16×10- 12), shown in the right panel of Fig. 4. The higher the CARE index score, the lower the AVLT30 min score and the more severe the disease. This relationship is statistically valid across and within the subject groups. The curve fitting was estimated using a nonlinear least squares algorithm, yielding b0 = 2, A = 15, b2 = –0.33, b3 = 0.22, and b4 = 1.39 for the equation S.8. The F-statistic for the full exponential model fit was F[4,139] = 19.3283, with a corresponding p-value = 1.16×10- 12.
Intrasubject repeatability of CARE index measurement
Figure 5 shows the relationship between each subject’s CARE index score at baseline and at the second visit within six months. The stage consistency reached 89% with a slope of 1.04, indicating significant intrasubject repeatability (p < 1.69e-016). This high degree of intrasubject CARE index score consistency indicates that the biomarker-based CARE index system is very robust. For the method of repeatability, please refer to the Supplementary Methods 8.
DISCUSSION
Our study demonstrated that, given the availability of multimodal biomarkers measured by only three examinations, neuroimaging (brain function and structure), biological fluid (CSF), and cognitive assessments, the real-world cross-sectional datasets from cohorts comprising the whole AD continuum could be used to determine the AD development sequence. This accomplishment is significant because such a study would otherwise require rich resources involving a large sample size and a longitudinal design with high costs and a protracted period. The major finding of this study is that when multiple AD biomarkers are temporally ordered, functional abnormalities in the HIP and PCC networks comprise the earliest event, even antedating detectable CSF Aβ and p-tau abnormalities. This finding sheds light on the link between preclinical AD status and symptomatic onset and can be applied to accurately identify progressive AD trajectories, given the condition that disease onset remains insidious and no single biomarker serves as a predictor for future cognitive decline.
Destabilized brain function can serve as a critical contributor to AD cognitive deterioration [22, 23]. Of the various brain networks, the DMN and the hippocampal functional connectivity network (HFCN) are closely associated with both AD pathologies and clinical symptoms [2]. With regard to the HFCN, major studies have observed hippocampal hyperactivity at the early AD stage [8, 24] and identified it as a potential indicator of impending cognitive impairment [25]. It is assumed that early-stage hippocampal hyperactivity may be related to Aβ-induced hippocampal synaptic excitotoxicity, tau accumulation [22], or mitochondrial dysfunction [26]. With respect to the DMN, it overlaps broadly with Aβ deposition, and the overlap is possibly attributed to continuously high neural activity in the DMN regions that advance Aβ accumulation [27]. Studies convergently demonstrate DMN hypoconnectivity and hypometabolism in AD-continuum subjects including asymptomatic APOE ɛ4 carriers [28], amnestic mild cognitive impairment (MCI) subjects [29], and AD-type dementia subjects [30]. Accordingly, current findings indicate that aberrant DMN and hippocampal connectivity strength could be among the earliest events to trigger AD and may represent initial disease targets.
An unresolved issue in evaluating AD biomarker utilities is how their changes influence the cognitive decline trajectory and clinical onset. Although earlier studies suggest amyloidosis impairment on cognitive function [31], this association remains weak and controversial [32]. This study observed that cognitive impairment, indexed by MMSE, ADAS-Cog, and AVLT scores, occurred following abnormal brain network connectivity, CSF Aβ levels, and p-tau levels. It indicates that clinical AD symptoms emerge as downstream events following brain amyloidosis and neurodegenerative changes. Also, this event sequence provides evidence to support a recent concept that neither amyloidosis nor neurodegeneration is sufficient in isolation to cause the AD pathophysiological and clinical cascade [33]; rather, the co-occurrence of amyloidosis and neurodegeneration significantly advances gray matter atrophy [34, 35], accelerates cognitive deterioration [36, 37], and increases MCI or dementia hazard in cognitively normal elderly cohorts [38]. Mechanistically, emerging biological studies indicate that Aβ and tau interaction is a driving force in AD development. Aβ accumulation advances tau disease progression in that both enhance tauopathy [39] and potentiate tau impairment on brain function [40]. Further, tau is a required factor in Aβ-induced neurotoxicity [11, 41]. Clearly, current findings regarding the Soptimal support the notion that, while amyloidosis and neurodegeneration arise independently, once both are present they interact to advance the AD pathophysiological cascade and are a key mechanism in transforming normal aging into AD [33, 42].
The conventional symptom-based AD staging system is limited in effectively facilitating disease prevention, diagnosis, and treatment. This limitation can be attributed to remarkably inter-subject biological heterogeneity within each stage and relatively low temporal resolution of the three stages (cognitive normal, MCI, and dementia) in illustrating continuous AD progression. The estimated Soptimal and derived CARE index discussed herein would address these attributions by characterizing the risk of the 10 AD events at the individual level through neither clinical diagnosis information nor a specific biomarker cut-off point. This study demonstrates that the CARE index scores closely parallel disease severity through the whole AD continuum, as indicated by its gradual increase from CN to MCI to AD at the symptom-defined group level and close correlation with episodic memory performance. The higher the CARE index score, the more advanced and severe the disease. Accordingly, the CARE index may serve as a surrogate to indicate the AD development process and facilitate clinical trials by a) identifying AD-risk subjects who do not yet have any clinical symptoms; b) staging and categorizing patient populations based on their CARE index scores to enrich response rates, as has been demonstrated in oncology; and c) monitoring and evaluating treatment efficacy through individual subjects’ changes in CARE index scores. This personalized medicine technique would be particularly beneficial in assessing the efficacy of promising secondary prevention interventions in patients at the earliest discernible stage of AD.
This study has four limitations. First, this is the first attempt to incorporate the resting-state functional connectivity HIPFCI and PCCFCI biomarkers into the EBP model. Future studies could integrate other functional and structural biomarkers, including the executive control network, salience network, and insular network, into the biomarker sequence to characterize the trajectory of the neural network changes with AD progression. Second, currently available datasets include only 45 CN and 25 AD subjects, which limits the representative probability distributions of biomarkers generated to accurately estimate the temporal order of biomarkers. The Soptimal determined from a larger sample size may be different from the current results. Third, this study relies on the assumption that all subjects follow a single optimal event sequence, despite heterogeneous pathways of sporadic AD development [1, 43]. A recent study introduced a cluster of subjects that may follow different event sequences in their disease progression [44]. Fourth, the current-event-based model used a naïve Bayesian model that assumes the different biomarkers are independent measurements. The assumption is not always true. However, in practice, a naïve Bayesian system can work surprisingly well, even when the independence assumption is not true [45]. Therefore, further studies with larger sample sizes, additional biomarker events, and updated analysis, are required to enhance our understanding of AD pathogenesis and improve detection of AD progression on an individual level.
ACKNOWLEDGMENTS
This work was supported by US National Institutes of Health grants R01 AG020279 and R44 AG035405. We sincerely thank Ms. Lydia Washechek, BA, for editorial assistance.
Data collection and sharing for this project was funded by the Alzheimer’s Disease Neuroimaging Initiative (ADNI) (National Institutes of Health [NIH] Grant U01 AG024904 [PI: MW Weiner]) and DOD ADNI (Department of Defense award number W81XWH-12-2-0012). ADNI is funded by the National Institute on Aging (NIA) and the National Institute of Biomedical Imaging and Bioengineering (NIBIB), and through generous contributions from the following: AbbVie, Alzheimer’s Association; Alzheimer’s Drug Discovery Foundation; Araclon Biotech; BioClinica, Inc.; Biogen; Bristol-Myers Squibb Company; CereSpir, Inc.; Cogstate; Eisai Inc.; Elan Pharmaceuticals, Inc.; Eli Lilly and Company; EuroImmun; F. Hoffmann-La Roche Ltd and its affiliated company Genentech, Inc.; Fujirebio; GE Healthcare; IXICO Ltd.; Janssen Alzheimer Immunotherapy Research & Development, LLC.; Johnson & Johnson Pharmaceutical Research & Development LLC.; Lumosity; Lundbeck; Merck & Co., Inc.; Meso Scale Diagnostics, LLC.; NeuroRx Research; Neurotrack Technologies; Novartis Pharmaceuticals Corporation; Pfizer Inc.; Piramal Imaging; Servier; Takeda Pharmaceutical Company; and Transition Therapeutics. The Canadian Institutes of Health Research is providing funds to support ADNI clinical sites in Canada. Private sector contributions are facilitated by the Foundation for the National Institutes of Health (http://www.fnih.org). The grantee organization is the Northern California Institute for Research and Education, and the study is coordinated by the Alzheimer’s Therapeutic Research Institute at the University of Southern California. ADNI data are disseminated by the Laboratory for Neuro Imaging at the University of California.
Authors’ disclosures are available online (http://j-alz.com/manuscript-disclosures/16-0537r1).
Appendices
The supplementary material is available in the electronic version of this article: http://dx.doi.org/10.3233/JAD-160537.
REFERENCES
[1] | Jack CR Jr , Knopman DS , Jagust WJ , Petersen RC , Weiner MW , Aisen PS , Shaw LM , Vemuri P , Wiste HJ , Weigand SD , Lesnick TG , Pankratz VS , Donohue MC , Trojanowski JQ ((2013) ) Tracking pathophysiological processes in Alzheimer’s disease: An updated hypothetical model of dynamic biomarkers. Lancet Neurol 12: , 207–216. |
[2] | Sperling R , Mormino E , Johnson K ((2014) ) The evolution of preclinical Alzheimer’s disease: Implications for prevention trials. Neuron 84: , 608–622. |
[3] | Jack CR Jr , Knopman DS , Jagust WJ , Shaw LM , Aisen PS , Weiner MW , Petersen RC , Trojanowski JQ ((2010) ) Hypothetical model of dynamic biomarkers of the Alzheimer’s pathological cascade. Lancet Neurol 9: , 119–128. |
[4] | Musiek ES , Holtzman DM ((2015) ) Three dimensions of the amyloid hypothesis: Time, space and wingmen. Nat Neurosci 18: , 800–806. |
[5] | Herrup K ((2015) ) The case for rejecting the amyloid cascade hypothesis. Nat Neurosci 18: , 794–799. |
[6] | Bero AW , Yan P , Roh JH , Cirrito JR , Stewart FR , Raichle ME , Lee JM , Holtzman DM ((2011) ) Neuronal activity regulates the regional vulnerability to amyloid-beta deposition. Nat Neurosci 14: , 750–756. |
[7] | Cummings DM , Liu W , Portelius E , Bayram S , Yasvoina M , Ho SH , Smits H , Ali SS , Steinberg R , Pegasiou CM , James OT , Matarin M , Richardson JC , Zetterberg H , Blennow K , Hardy JA , Salih DA , Edwards FA ((2015) ) First effects of rising amyloid-beta in transgenic mouse brain: Synatic transmission and gene expression. Brain 138(Pt 7): , 1992–2004. |
[8] | Filippini N , MacIntosh BJ , Hough MG , Goodwin GM , Frisoni GB , Smith SM , Matthews PM , Beckmann CF , Mackay CE ((2009) ) Distinct patterns of brain activity in young carriers of the APOE-epsilon4 allele. Proc Natl Acad Sci U S A 106: , 7209–7214. |
[9] | Sheline YI , Morris JC , Snyder AZ , Price JL , Yan Z , D’Angelo G , Liu C , Dixit S , Benzinger T , Fagan A , Goate A , Mintun MA ((2010) ) APOE4 allele disrupts resting state fMRI connectivity in the absence of amyloid plaques or decreased CSF Abeta42. J Neurosci 30: , 17035–17040. |
[10] | Reiman EM , Chen K , Alexander GE , Caselli RJ , Bandy D , Osborne D , Saunders AM , Hardy J ((2004) ) Functional brain abnormalities in young adults at genetic risk for late-onset Alzheimer’s dementia. Proc Natl Acad Sci U S A 101: , 284–289. |
[11] | Roberson ED , Scearce-Levie K , Palop JJ , Yan F , Cheng IH , Wu T , Gerstein H , Yu GQ , Mucke L ((2007) ) Reducing endogenous tau ameliorates amyloid beta-induced deficits in an Alzheimer’s disease mouse model. Science 316: , 750–754. |
[12] | Serrano-Pozo A , Qian J , Monsell SE , Blacker D , Gomez-Isla T , Betensky RA , Growdon JH , Johnson KA , Frosch MP , Sperling RA , Hyman BT ((2014) ) Mild to moderate Alzheimer dementia with insufficient neuropathological changes. Ann Neurol 75: , 597–601. |
[13] | Bartlett JW , Frost C , Mattsson N , Skillback T , Blennow K , Zetterberg H , Schott JM ((2012) ) Determining cut-points for Alzheimer’s disease biomarkers: Statistical issues, methods and challenges. Biomark Med 6: , 391–400. |
[14] | Rollo JL , Banihashemi N , Vafaee F , Crawford JW , Kuncic Z , Holsinger RM ((2016) ) Unraveling the mechanistic complexity of Alzheimer’s disease through systems biology. Alzheimers Dement 12: , 708–718. |
[15] | Fonteijn HM , Modat M , Clarkson MJ , Barnes J , Lehmann M , Hobbs NZ , Scahill RI , Tabrizi SJ , Ourselin S , Fox NC , Alexander DC ((2012) ) An event-based model for disease progression and its application in familial Alzheimer’s disease and Huntington’s disease. Neuroimage 60: , 1880–1889. |
[16] | Young AL , Oxtoby NP , Daga P , Cash DM , Fox NC , Ourselin S , Schott JM , Alexander DC , Alzheimer’s Disease Neuroimaging Initiative ((2014) ) A data-driven model of biomarker changes in sporadic Alzheimer’s disease. Brain 137: , 2564–2577. |
[17] | Barros-Battesti DM , Ramirez DG , Sampaio Jdos S , Famadas KM , Faccini JL , Nunes PH , Martins TF , Ogrzewalska M , Labruna MB , Marcili A , Barbieri Fda S ((2013) ) Description of larva of Amblyomma romitii (Acari: Ixodidae) by optical and scanning electron microscopy, including porotaxy and phylogenetic analysis. Exp Appl Acarol 60: , 271–280. |
[18] | Kirsten H , Teupser D , Weissfuss J , Wolfram G , Emmrich F , Ahnert P ((2007) ) Robustness of single-base extension against mismatches at the site of primer attachment in a clinical assay. J Mol Med (Berl) 85: , 361–369. |
[19] | Chen G , Chen G , Xie C , Ward BD , Li W , Antuono P , Li SJ ((2012) ) A method to determine the necessity for global signal regression in resting-state fMRI studies. Magn Reson Med 68: , 1828–1835. |
[20] | Puolamaki K , Fortelius M , Mannila H ((2006) ) Seriation in paleontological data using markov chain Monte Carlo methods.e. PLoS Comput Biol 2: , 6. |
[21] | Ziegler G , Penny WD , Ridgway GR , Ourselin S , Friston KJ , Alzheimer’s Disease Neuroimaging Initiative ((2015) ) Estimating anatomical trajectories with Bayesian mixed-effects modeling. Neuroimage 121: , 51–68. |
[22] | Palop JJ , Mucke L ((2010) ) Amyloid-beta-induced neuronal dysfunction in Alzheimer’s disease: From synapses toward neural networks. Nat Neurosci 13: , 812–818. |
[23] | Sanchez PE , Zhu L , Verret L , Vossel KA , Orr AG , Cirrito JR , Devidze N , Ho K , Yu GQ , Palop JJ , Mucke L ((2012) ) Levetiracetam suppresses neuronal network dysfunction and reverses synaptic and cognitive deficits in an Alzheimer’s disease model. Proc Natl Acad Sci U S A 109: , E2895–E2903. |
[24] | Sperling RA , Dickerson BC , Pihlajamaki M , Vannini P , LaViolette PS , Vitolo OV , Hedden T , Becker JA , Rentz DM , Selkoe DJ , Johnson KA ((2010) ) Functional alterations in memory networks in early Alzheimer’s disease. Neuromolecular Med 12: , 27–43. |
[25] | O’Brien JL , O’Keefe KM , LaViolette PS , DeLuca AN , Blacker D , Dickerson BC , Sperling RA ((2010) ) Longitudinal fMRI in elderly reveals loss of hippocampal activation with clinical decline. Neurology 74: , 1969–1976. |
[26] | Swerdlow RH , Burns JM , Khan SM ((2014) ) The Alzheimer’s disease mitochondrial cascade hypothesis: Progress and perspectives. Biochim Biophys Acta 1842: , 1219–1231. |
[27] | Buckner RL , Sepulcre J , Talukdar T , Krienen FM , Liu H , Hedden T , Andrews-Hanna JR , Sperling RA , Johnson KA ((2009) ) Cortical hubs revealed by intrinsic functional connectivity: Mapping, assessment of stability, and relation to Alzheimer’s disease. J Neurosci 29: , 1860–1873. |
[28] | Fleisher AS , Sherzai A , Taylor C , Langbaum JB , Chen K , Buxton RB ((2009) ) Resting-state BOLD networks versus task-associated functional MRI for distinguishing Alzheimer’s disease risk groups. Neuroimage 47: , 1678–1690. |
[29] | Sorg C , Riedl V , Muhlau M , Calhoun VD , Eichele T , Laer L , Drzezga A , Forstl H , Kurz A , Zimmer C , Wohlschlager AM ((2007) ) Selective changes of resting-state networks in individuals at risk for Alzheimer’s disease. Proc Natl Acad Sci U S A 104: , 18760–18765. |
[30] | Greicius MD , Srivastava G , Reiss AL , Menon V ((2004) ) Default-mode network activity distinguishes Alzheimer’s disease from healthy aging: Evidence from functional MRI. Proc Natl Acad Sci U S A 101: , 4637–4642. |
[31] | Mormino EC , Kluth JT , Madison CM , Rabinovici GD , Baker SL , Miller BL , Koeppe RA , Mathis CA , Weiner MW , Jagust WJ , Alzheimer’s Disease Neuroimaging Initiative ((2009) ) Episodic memory loss is related to hippocampal-mediated beta-amyloid deposition in elderly subjects. Brain 132: , 1310–1323. |
[32] | Villemagne VL , Burnham S , Bourgeat P , Brown B , Ellis KA , Salvado O , Szoeke C , Macaulay SL , Martins R , Maruff P , Ames D , Rowe CC , Masters CL , Australian Imaging Biomarkers Lifestyle (AIBL) Research Group ((2013) ) Amyloid beta deposition, neurodegeneration, and cognitive decline in sporadic Alzheimer’s disease: A prospective cohort study. Lancet Neurol 12: , 357–367. |
[33] | Chetelat G ((2013) ) Alzheimer disease: Abeta-independent processes-rethinking preclinical AD. Nat Rev Neurol 9: , 123–124. |
[34] | Desikan RS , McEvoy LK , Thompson WK , Holland D , Roddey JC , Blennow K , Aisen PS , Brewer JB , Hyman BT , Dale AM , Alzheimer’s Disease Neuroimaging Initiative ((2011) ) Amyloid-beta associated volume loss occurs only in the presence of phospho-tau. Ann Neurol 70: , 657–661. |
[35] | Fortea J , Vilaplana E , Alcolea D , Carmona-Iragui M , Sanchez-Saudinos MB , Sala I , Anton-Aguirre S , Gonzalez S , Medrano S , Pegueroles J , Morenas E , Clarimon J , Blesa R , Lleo A , Alzheimer’s Disease Neuroimaging Initiative ((2014) ) Cerebrospinal fluid beta-amyloid and phospho-tau biomarker interactions affecting brain structure in preclinical Alzheimer disease. Ann Neurol 76: , 223–230. |
[36] | Mormino EC , Betensky RA , Hedden T , Schultz AP , Amariglio RE , Rentz DM , Johnson KA , Sperling RA ((2014) ) Synergistic effect of beta-amyloid and neurodegeneration on cognitive decline in clinically normal individuals. JAMA Neurol 71: , 1379–1385. |
[37] | Wirth M , Madison CM , Rabinovici GD , Oh H , Landau SM , Jagust WJ ((2013) ) Alzheimer’s disease neurodegenerative biomarkers are associated with decreased cognitive function but not beta-amyloid in cognitively normal older individuals. J Neurosci 33: , 5553–5563. |
[38] | Knopman DS , Jack CR Jr , Wiste HJ , Weigand SD , Vemuri P , Lowe V , Kantarci K , Gunter JL , Senjem ML , Ivnik RJ , Roberts RO , Boeve BF , Petersen RC ((2012) ) Short-term clinical outcomes for stages of NIA-AA preclinical Alzheimer disease. Neurology 78: , 1576–1582. |
[39] | Lewis J , Dickson DW , Lin WL , Chisholm L , Corral A , Jones G , Yen SH , Sahara N , Skipper L , Yager D , Eckman C , Hardy J , Hutton M , McGowan E ((2001) ) Enhanced neurofibrillary degeneration in transgenic mice expressing mutant tau and APP. Science 293: , 1487–1491. |
[40] | Khan UA , Liu L , Provenzano FA , Berman DE , Profaci CP , Sloan R , Mayeux R , Duff KE , Small SA ((2014) ) Molecular drivers and cortical spread of lateral entorhinal cortex dysfunction in preclinical Alzheimer’s disease. Nat Neurosci 17: , 304–311. |
[41] | Rapoport M , Dawson HN , Binder LI , Vitek MP , Ferreira A ((2002) ) Tau is essential to beta-amyloid-induced neurotoxicity. Proc Natl Acad Sci U S A 99: , 6364–6369. |
[42] | Jack CR Jr ((2014) ) PART and SNAP. Acta Neuropathol 128: , 773–776. |
[43] | Jack CR Jr , Holtzman DM ((2013) ) Biomarker modeling of Alzheimer’s disease. Neuron 80: , 1347–1358. |
[44] | Young AL , Oxtoby NP , Huang J , Marinescu RV , Daga P , Cash DM , Fox NC , Ourselin S , Schott JM , Alexander DC , Alzheimer’s Disease Neuroimaging Initiative ((2015) ) Multiple orderings of events in disease progression. Inf Process Med Imaging 24: , 711–722. |
[45] | Russell SJ , Norvig P ((2003) ) Prentice Hall/Pearson Education, Upper Saddle River, NJ. Artificial intelligence: A Modern Approach. |
Figures and Tables
Fig.1
Probability distributions of normal (cyan) and abnormal (black) events measured by biomarkers from the AD and CN populations. The y-axis denotes the proportion of subjects, while the x-axis indicates the detected value of each biomarker measurement. The (–1) is employed to reverse the signs of the biomarker, indicating the left distribution is an event that occurred and the right distribution is an event that did not occur.
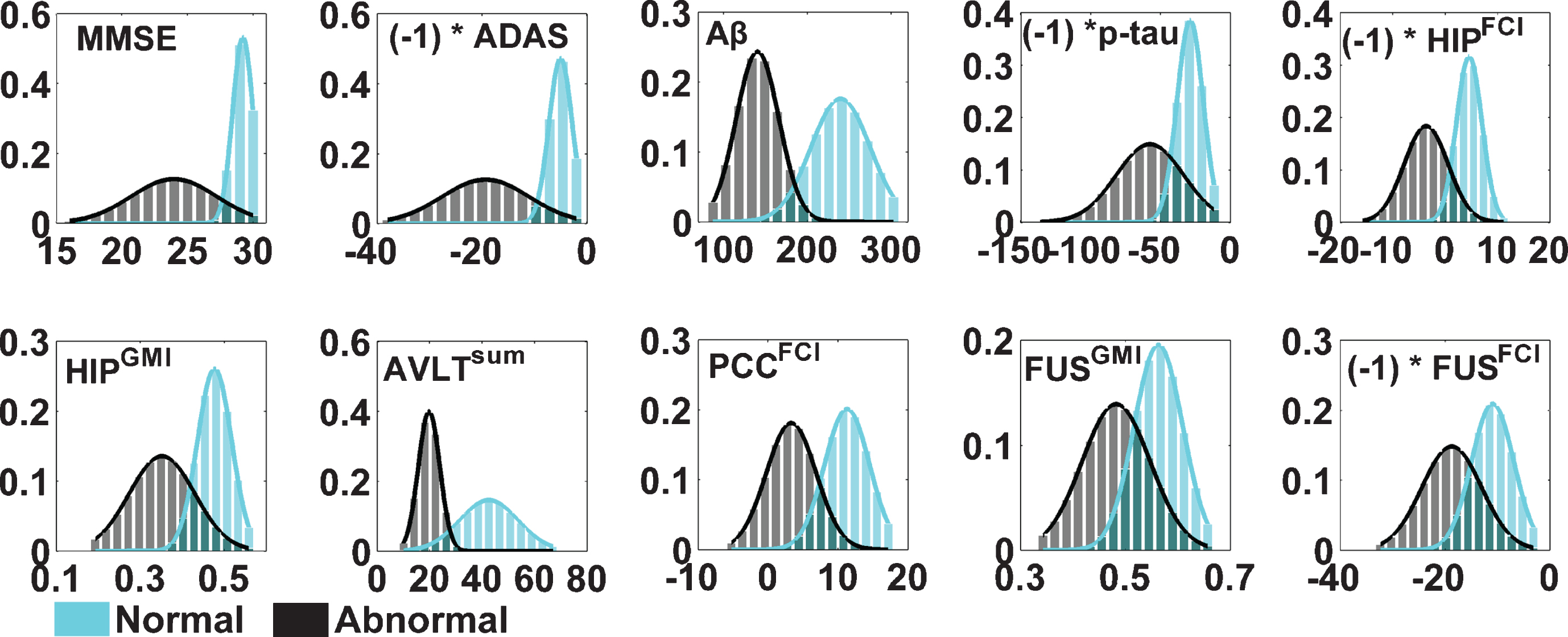
Fig.2
Optimal temporal order, Soptimal, of the 10 AD biomarkers estimated by the EBP model. A) The y-axis shows the Soptimal and the x-axis shows the CARE index score at which the corresponding event occurred. B) Bootstrap cross-validation of the Soptimal. Each entry in the matrix represents the proportion of the Soptimal during 500 bootstrap samples. The proportion values range from 0 to 1 and correspond to color, from white to black. The CARE index scores with their corresponding biomarkers follow: 1, increased HIPFCI; 2, decreased PCCFCI; 3, decreased Aβ concentration; 4, increased p-tau concentration; 5, decreased MMSE score; 6, increased ADAS score; 7, decreased HIPGMI; 8, decreased AVLT score; 9, decreased FUSGMI; 10, increased FUSFCI.
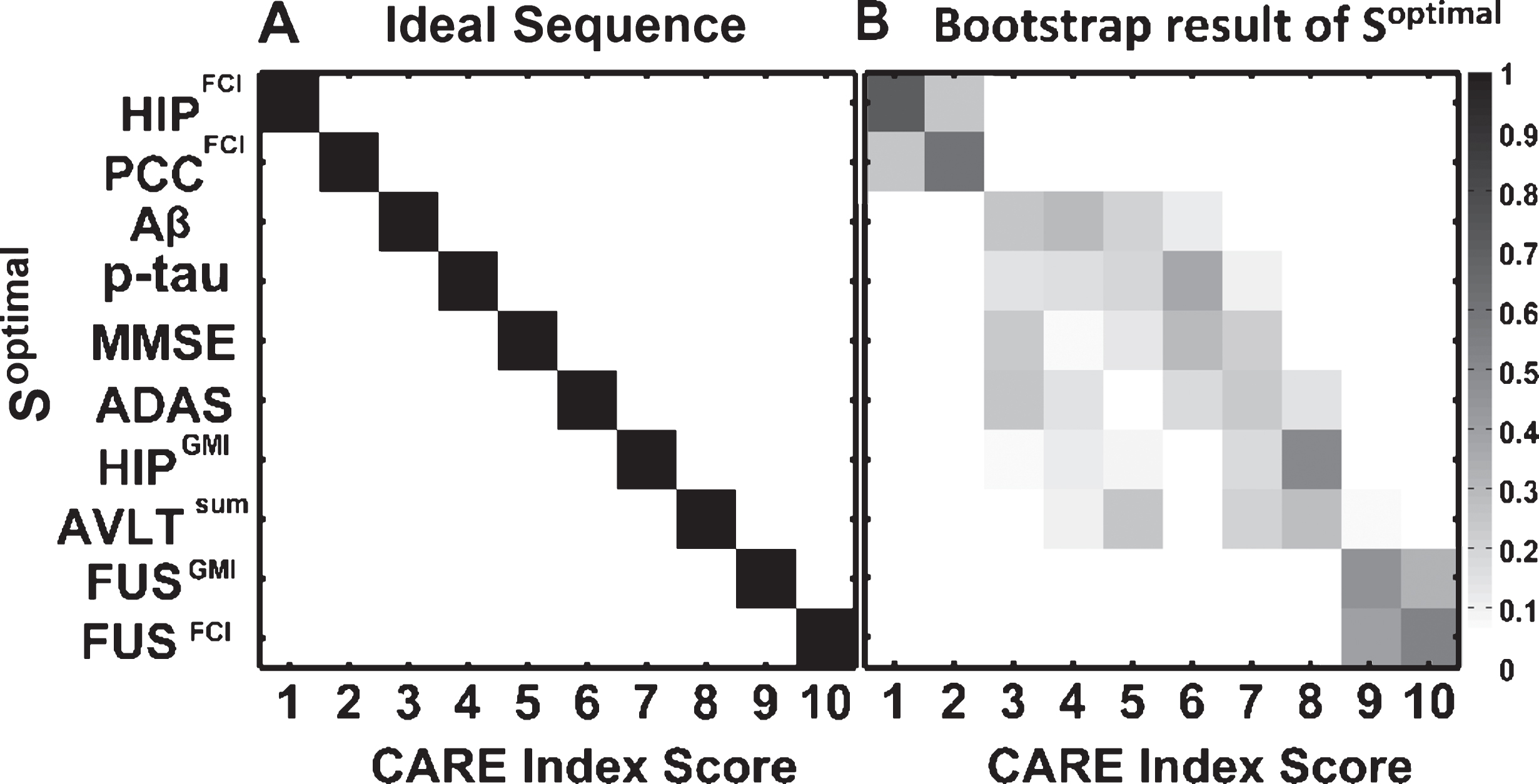
Fig.3
CARE index associated with AD clinical stages. A) Normalized likelihoods across the CARE index. The cyan, yellow, red, and black lines represent the likelihoods at each score on the CARE index for a CN subject, an EMCI subject, an LMCI subject, and an AD subject, respectively. B) CARE index distribution in CN and AD groups calculated from the EBP model. The CARE index is ordered by the maximum likelihood event sequence. Each score on the CARE index corresponds to the occurrence of a biomarker event. CARE index score 0 corresponds to no events having occurred and CARE index score 10 corresponds to all events having occurred. Both CN and AD groups showed heterogeneous index distributions. C) CARE index distributions in EMCI and LMCI groups. The proportion of EMCI (yellow) and LMCI (red) subjects at each CARE index score was plotted. D) A box plot of the CARE index score differences between groups. The median CARE index scores for CN, EMCI, LMCI, and AD groups are 2, 4, 6, and 9, respectively. The two-sample t-tests between CN and EMCI, CN and LMCI, EMCI and LMCI, and AD and LMCI showed significant differences. The red “+” denotes an outlier in the CN group.
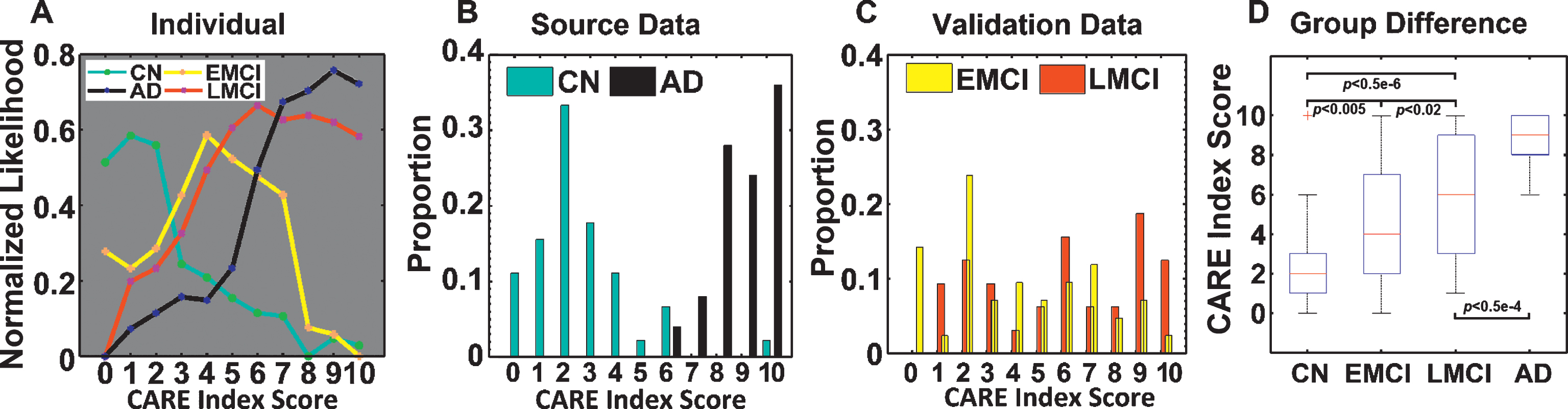
Fig.4
Correlations between CARE index score and episodic memory performance. Both the within-group linear regression model and across-groups nonlinear curve-fitting analysis demonstrated significantly negative correlations between the CARE index scores and AVLT30 min scores. The higher the CARE index score, the worse the episodic memory function. Note that, since the data are discrete, many individual data points overlap; for clarity, we perturbed each of the individual plot points by adding a small random displacement in the horizontal and vertical directions. The linear regression analysis used only the original (nonperturbed) data as an input.
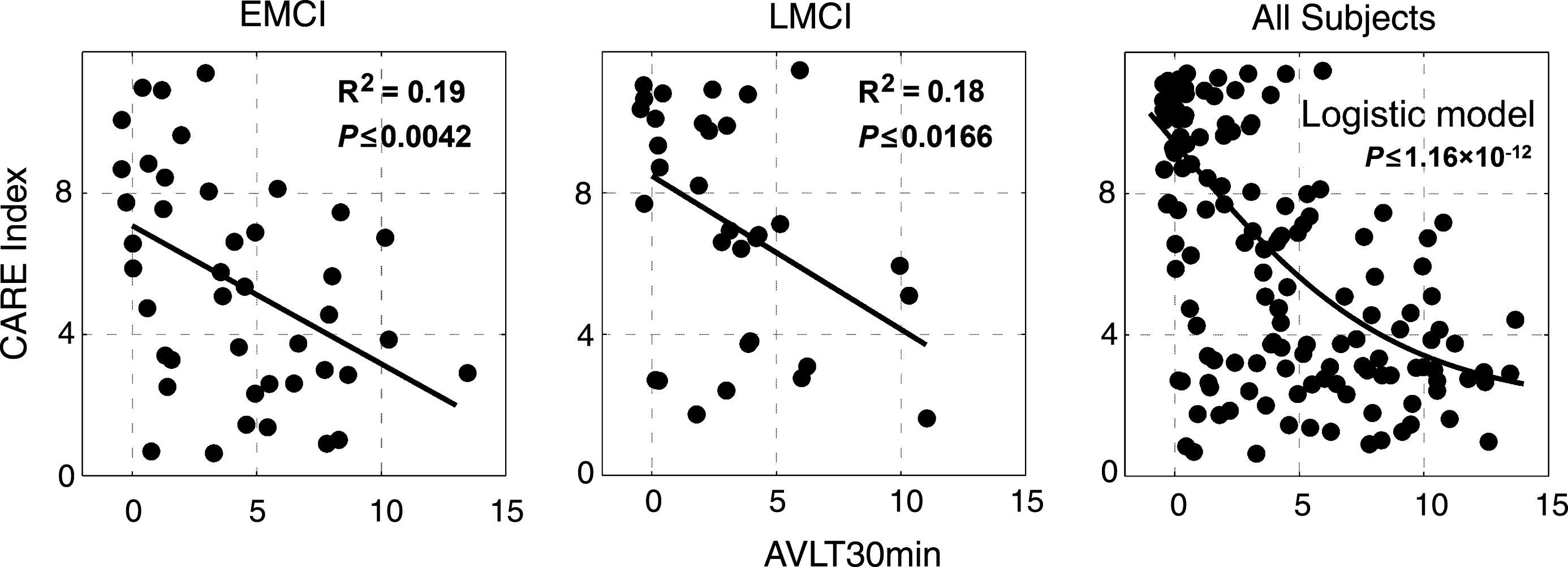
Fig.5
Intrasubject consistency of the CARE index score between repeated measures. The x-axis is individual’s baseline CARE index score, and the y-axis is the individual’s CARE index score from a measurement repeated within six months. Circle size and the number next to the circle represent the number of subjects falling on the same data point. The correlation value between two CARE index scores from repeated measurements is 89% with a slope of 1.04 (p < 1.69e-016).
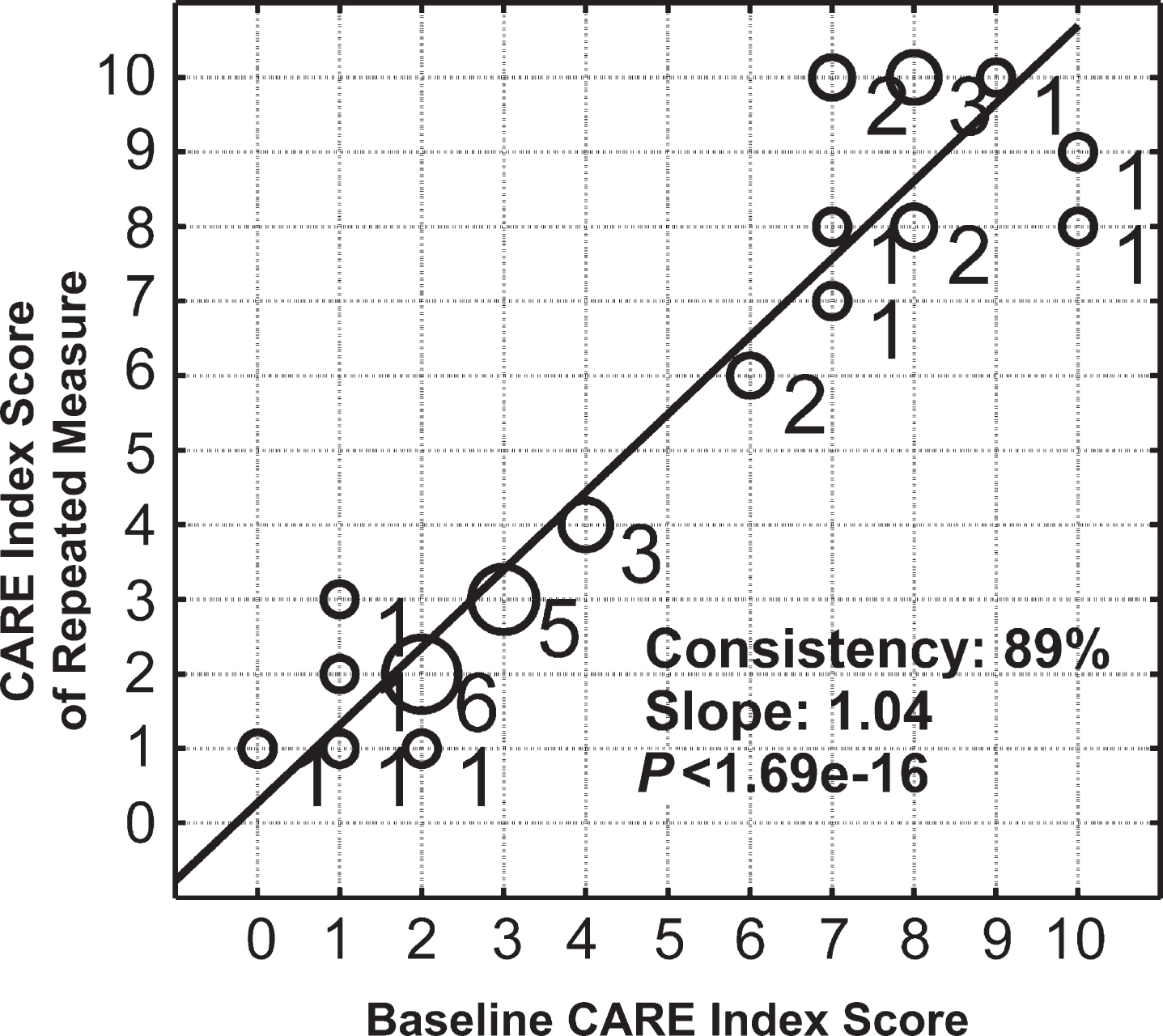
Table 1
Demographic and clinical data for all subjects
CN* | EMCI | LMCI | AD | F value | p-value | |
(n = 45) | (n = 42) | (n = 32) | (n = 25) | |||
Gender (M/F) | 20/25 | 19/22# | 20/12 | 13/12 | 0.98 | 0.40 |
Age (Yrs) | 73.6±6.4 | 71.5±6.9 | 72.1±8.1 | 73.9±6.9 | 0.98 | 0.41 |
Education (Yrs) | 16.3±2.5 | 15.1±2.4 | 16.8±2.4 | 15.7±2.8 | 2.93 | 0.04 |
MMSE | 28.8±1.3 | 28.0±1.8 | 27.5±1.9 | 22.6±2.8 | 63.13 | <7.0×10- 26 |
ADAS-Cog | 5.4±2.6 | 8.6±3.4 | 11.4±5.3 | 21.7±7.3 | 71.36 | <4.6×10- 28 |
Aβ (pg/ml) | 201.5±54.2 | 177.1±61.0 | 170.5±48.2 | 141.0±40.0 | 7.21 | <2.0×10- 4 |
p-tau (pg/ml) | 32.7±14.2 | 43.2±24.0 | 44.4±21.6 | 55.4±26.9 | 6.21 | <0.5×10- 3 |
AVLT | 44.6±10.4 | 37.3±10.4 | 32.7±7.6 | 22.6±7.1 | 31.48 | <1.3×10- 15 |
AVLT30min | 7.0±3.8 | 4.2±3.4 | 3.1±3.0 | 0.6±1.3 | 23.12 | <3.3×10- 12 |
*Including 18 significant memory concern subjects who were cognitively normal. #One EMCI subject’s gender information is unavailable. Clinical data are expressed as mean±standard deviation. F values and p-values were obtained by one-way analysis of variance. There were significant differences among the four groups for education years; MMSE, ADAS-Cog, and AVLT scores; and CSF Aβ and p-tau levels. Abbreviations: CN, cognitively normal; EMCI, early mild cognitive impairment; LMCI, late mild cognitive impairment; AD, Alzheimer’s disease; MMSE, Mini-Mental State Examination; ADAS-Cog, Alzheimer’s Disease Assessment Scale-Cognitive Subscale; AVLT30 min, Auditory Verbal Learning Test-30-min delayed recall; Aβ, β-amyloid; p-tau, phosphorylated tau; AVLT, Rey Auditory Verbal Learning Test.