Exploring Causal Relationships Between Gut Microbiota and Alzheimer’s Disease: A Bidirectional Mendelian Randomization Study
Abstract
Background:
Many observational studies have investigated the link between the gut microbiota and Alzheimer’s disease (AD), but the causality remains uncertain.
Objective:
This study aimed to evaluate the causal impact of gut microbiota on AD.
Methods:
A two-sample Mendelian randomization (MR) study was conducted employing summary data. Summary statistics for AD were from the latest genome-wide association study (cases and proxy cases: 85,934; controls: 401,577). Summary data for gut microbiota were acquired from MiBioGen consortium. Causal effect estimations primarily relied on the inverse variance weighting method along with the sensitivity analyses for testing for pleiotropy and heterogeneity. Additionally, reverse MR analyses were performed to examine potential reverse causality.
Results:
Seven gut microbiota were identified as associated with AD risk. Order Selenomonadales (odds ratio [OR] 1.13, 95% confidence interval [CI] 1.03–1.24, p = 0.01), Family Pasteurellaceae (OR 1.07, 95% CI 1.01–1.13, p = 0.01), and Genus Methanobrevibacter (OR 1.07, 95% CI 1.00–1.13, p = 0.04) were correlated with an elevated likelihood of AD, while Class Mollicutes (OR 0.87, 95% CI 0.79–0.95, p = 0.00), Genus Ruminiclostridium9 (OR 0.87, 95% CI 0.78–0.97, p = 0.01), Genus Clostridiuminnocuumgroup (OR 0.94, 95% CI 0.89–0.99, p = 0.03), and Genus Eggerthella (OR 0.94, 95% CI 0.89–1.00, p = 0.04) exerted beneficial impact in mitigating AD. No statistically significant reverse causality was found between AD and each of these seven specific gut microbiota species.
Conclusions:
This study unveiled a causal link between certain gut microbiota and AD, offering new insights for advancing clinical treatments.
INTRODUCTION
Alzheimer’s disease (AD) is a neurodegenerative disorder that accounts for 60–80% of cases of cognitive decline [1]. Regrettably, the etiology of AD is poorly understood [2]. Because the AD biomarkers testing is radioactive and invasive, the diagnosis of AD mainly relies on the assessment of clinical manifestations. Regarding treatment, despite substantial investments in the field, there remains a deficiency in effective medications capable of altering the pathological progression of AD [3]. Hence, further elucidating the pathological mechanisms of AD, exploring novel non-invasive early biomarkers, and developing effective treatment strategies are imperative.
Gut microbiota are the microorganisms in the digestive tracts of animals that play a crucial role in metabolic processes, immune modulation, and intestinal epithelial upkeep [4]. Lately, considerable attention has been directed towards the influence of gut microbiota on central nervous system function, commonly known as the gut-brain axis [5]. Growing evidence suggests that changes in the gut microbiome are linked to the initiation and progression of AD [6–9]. In an observational study, the gut microbiome composition in individuals with AD was found to be significantly different from that of asymptomatic controls. By characterizing the bacterial taxonomic makeup of fecal samples from subjects with and without diagnosed AD, Vogt et al. identified differences in bacterial abundance from phylum to genus in the microbiomes of AD participants, including Firmicutes Phylum decreased, Bacteroidetes increased, and Bifidobacteria decreased [10]. It was also discovered that some gut microbiota in individuals with AD differed at the taxonomic level from controls, such as Bacteroidetes, Actinobacteria, Ruminococcus, Lachnospiraceae, and Lunamonales, indicating potential involvement of gut microbiota in AD pathogenesis [11]. Furthermore, neuroinflammation in AD patients may be related to amyloidosis and peripheral inflammation caused by altered gut microbiota. Specifically, AD patients exhibited elevated levels of species of endobacteria with pro-inflammatory properties such as Escherichia/Shigella, alongside reduced levels of the anti-inflammatory microbial group E. rectale. [12]. In addition, gut dysbiosis can contribute to the occurrence and onset of AD through oxidative stress and insulin resistance [13]. However, the debate persists over whether variations in gut microbiota composition are a causative factor or a consequence of AD, with meta-analyses of observational studies yielding inconclusive findings [11]. The results in these studies may be affected by unmeasured confounding factors (e.g., health conditions), potentially leading to inaccuracies in reflecting the true causal effects.
Mendelian randomization (MR) is a methodological study design, comparable to a randomized controlled trial, that estimates causal effects by exploiting the random allocation of genetic variants [14]. By leveraging strongly associated single nucleotide polymorphisms (SNPs) in the role of instrumental variables (IVs) for inference, MR produces unbiased estimates even when there is unmeasured confounding factors. While the MR approach has been applied across various studies to investigate potential causal links between the gut microbiota and various diseases [15–18], evidence linking the gut microbiota to a causal role in AD is limited.
In this case, we conducted a two-sample bidirectional MR investigation employing summary level statistics obtained from the most extensive and current genome-wide association studies (GWASs) of common gut microbiota species and AD. By using multiple MR analysis methods and performing comprehensive sensitivity analyses, we found potential causal effects of the presence of 7 gut microbiota species on AD proxied by 1,947 SNPs. Specifically, Order Selenomonadales, Family Pasteurel-laceae, and Genus Methanobrevibacter were potentially linked with an elevated risk of AD. In contrast, Class Mollicutes, Genus Ruminiclostridium9, Genus Clostridiuminnocuumgroup, and Genus Eggerthella were shown to exert a protective influence on AD onset and development. Furthermore, no significant reverse causality was found between AD and these seven gut microbiota species. These findings have clinical implications, because they may provide novel avenues for advancing therapeutic approaches in clinical settings for AD and strategies to prevent AD.
MATERIALS AND METHODS
MR and assumptions
This study utilized two-sample MR with genetic instruments to explore potential causal links between gut microbiota and AD, leveraging data from the latest GWAS. SNPs associated with the composition of microorganisms inhabiting the gastrointestinal tract of humans were chosen as IVs. We employed a bidirectional design to test the association of gut microbiota with AD and to examine whether the gut microbiota composition difference among individuals is a downstream effect of AD.
MR relies on three assumptions: 1) Relevance: the IVs exhibit strong association with the exposure under scrutiny; 2) Effective random assignment: the genetic variants utilized as IVs are free from associations with the unmeasured potential confounders; 3) Exclusion restriction: the IVs solely influence the outcome by means of the exposure under investigation [19].
Genetic associations with gut microbiota
The genetic data regarding gut microbiota were sourced via the recent GWAS conducted by the international consortium MiBioGen [20, 21]. This comprehensive study incorporated sequencing data of 16 S ribosomal RNA genes and genotyping information from a total of 18,340 individuals across 24 cohorts from diverse nations, including Finland, Germany, the UK, etc. It aimed to investigate the connection involving autosomal human genetic variations and the gut microbiome, encompassing a comprehensive set of 211 taxa. Of these, 15 microbial taxa without particular species designation were excluded, leaving a total of 196 taxa for analysis.
The data source for AD
The summary statistics pertaining to AD were acquired via a recent GWAS carried out by the European Alzheimer and Dementia Biobank (EADB) consortium [22]. It encompasses a total of 39,106 individuals clinically diagnosed with AD, 46,828 individuals classified as proxy-ADD cases (individuals whose parent received a diagnosis of AD or dementia [23]) and 401,577 control individuals of European descent.
Genetic instruments selection
This study utilized SNPs that strongly and independently predicted exposures as genetic instruments. To broaden the scope of correlation findings, we chose to employ genetic instruments with a threshold of p < 1×10–5 [24]. To ensure the independence among the genetic instruments, a linkage disequilibrium screening (correlation coefficient less than 0.01 and within 5,000 kb) was performed using data from the European-based 1000 Genomes Project [25]. Taking into account the strand orientation, we excluded palindromic SNPs (such as those containing A/T or G/C bases, where the alleles on the forward strand match those on the reverse strand) [17].
Instrumental strength for the association between SNPs and exposure was assessed by F-statistic. F value is calculated by F = [R2×(N-2)]/(1- R2), R2 = [2×β2×EAF×(1-EAF)]/[2×β2×EAF× (1-EAF)+2×SE2×N×EAF×(1-EAF)], where N, β, EAF and SE represent the sample size, effect size of the SNP, effect allele frequency, and standard error of the estimator of β, respectively [26, 27]. SNPs with F-statistics below 10 were removed. Furthermore, exposures with fewer than three SNPs associated across the entire genome were eliminated, as per the criteria set for particular sensitivity analyses [28].
Statistical analysis
Mendelian randomization analysis
In the main analysis, we evaluated the causal relationships between gut microbiota and AD by using the inverse variance weighting (IVW) estimation [29]. Besides, to enhance the robustness of the research, we utilized four alternative approaches, including MR-Egger regression, weighted median (WM), maximum likelihood estimation (MLE), as well as weighted mode (WMODE) method.
Pleiotropy denotes the occurrence where genetic variants impact multiple phenotypes, potentially influencing result reliability. IVW estimation involves meta-analyzing variant-specific Wald ratios for each variant, assuming balanced pleiotropy [28]. The four alternative approaches mentioned above can address horizontal pleiotropy—an infringement of the exclusion restriction assumption—under varying assumptions about the causal structure of the pleiotropy. The WM estimator requires that more than 50% SNPs are unaffected by pleiotropy [30]. Besides, in MR-Egger analysis, it is assumed that the magnitude of the pleiotropic effect is independent of the strength of the association between the genetic variant and the exposure across all instruments [28]. The maximum likelihood estimation stands as a classical tool for point estimation with lower standard error than the IVW method [31]. Finally, the weighted mode method allows a relaxation of the instrumental variable assumptions even if the majority of instruments are invalid [32]. As IVW estimation is reputed to be more robust when multiple IVs are used [30], this study primarily relies on the IVW method, complemented by the other four approaches as supplementary analyses.
Reverse Mendelian randomization
Bidirectional MR involves conducting two analyses on the same pair of phenotypes, with the exposure and the outcome variables swapped. In the reverse MR context, AD was regarded as the exposure and SNPs that reach significance p < 5×10–8 were extracted as instruments. Based on previous MR analysis, gut microbiota significantly associated with AD risk were regarded as the outcome. Following this, we employed a similar two-sample MR analysis to test whether AD causes the gut microbiota composition changes.
Sensitivity analyses
Given the challenge of verifying the exclusion restriction assumption, extensive sensitivity analyses were carried out to ensure the consistency and reliability of the inference. We employed the MR-Egger intercept test [31] and the MR-pleiotropy residual sum and outlier (MR-PRESSO) global test [33] to detect data points that deviate significantly from the rest of the dataset and assess horizontal pleiotropy within the IVs. In both tests, a p-value greater than 0.05 suggests the absence of pleiotropy. According to Rucker model selection framework, goodness-of-fit heterogeneity statistics can be used to determine whether the IVW method and MR-Egger regression model are best supported by the data. Therefore, Cochran’s Q test was utilized to determine whether heterogeneity existed among IVs, that is, to assess whether the variability in effect sizes was greater than that expected by chance alone. Similarly, p > 0.05 indicates the absence of significant heterogeneity. When sensitivity analyses did not yield significant conclusions, that is, the MR assumptions were met without pleiotropy or heterogeneity effects, the IVW method was considered to be reliable.
Results were considered significant based on the following criteria [34, 35]: 1) the IVW method demonstrated a statistically significant outcome (p < 0.05); 2) according to the relationship between OR value and 1, the five aforementioned MR analysis approaches indicated constant directions; (3) sensitivity analyses did not provide significance (p > 0.05).
We depicted the detailed schematic overview of this MR study in Fig. 1. All statistical procedures were executed utilizing R software version 4.3.2 and the R packages in the data availability statement section (‘TwosampleMR’[33], ‘Mendelian randomization’ [36], and ‘MR-PRESSO’ [37]).
Fig. 1
Workflow for performing the two-sample bidirectional Mendelian randomization study. GWAS, genome-wide association study; MR, Mendelian randomization.
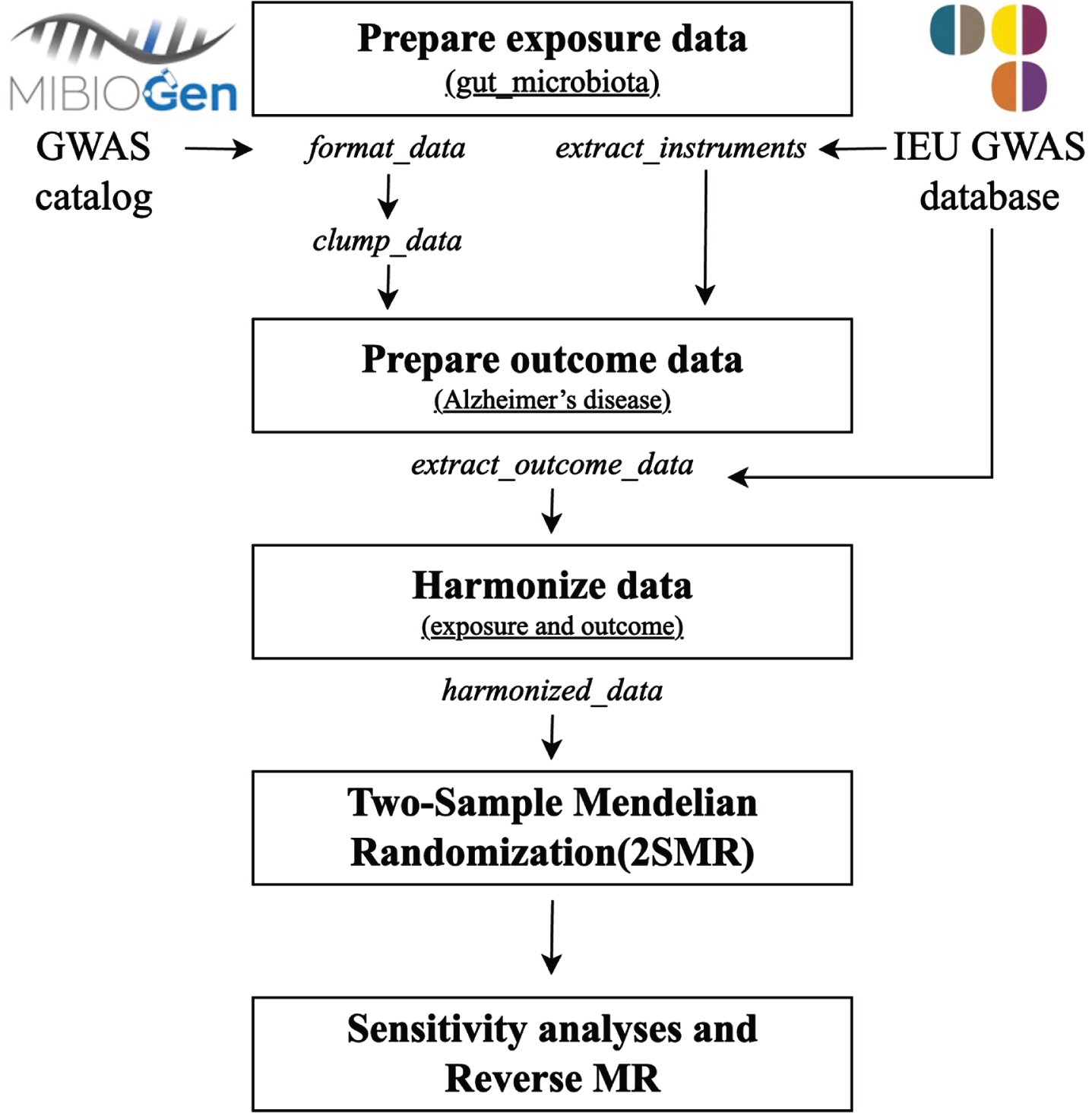
RESULTS
Collection of instrumental variable
After conducting quality control and removing exposures with fewer than 3 associated SNPs, we collected 1,947 SNPs jointly associated with AD and 183 taxa of gut microbiota, from fine to rough, comprising 111 genera, 29 families, 19 orders, 15 classes, and 9 phyla. Details of the SNPs and gut microbiota included are in Supplementary Table 1. The F-statistics of the IVs are all larger than 16.97, indicating a low likelihood of weak IVs bias.
Causal relationships between genetically proxied gut microbiota and AD
Figure 2 visually illustrates the preliminary findings regarding the relationships between the genetically proxied gut microbiota and the genetically proxied AD. Seven taxa of gut microbiota were identified as having a causal association with AD by applying the IVW method at the significance level 0.05 (Fig. 3). And the other four methods showed consistent directions with the IVW method.
Fig. 2
The causal effects of gut microbiota on Alzheimer’s disease were detected by five MR analysis methods. The numbers in the outermost circle are the IDs of each gut microbiota, and their correspondence with the names of the microbiota taxa is shown in Supplementary Table 2. Each red dot represents the estimated causal impact of the gut microbiota on AD using the IVW method, that is, the odds ratio (OR). The dashed line indicates OR = 1. OR < 1 indicates that the microbiota may have a protective effect on AD, otherwise they may be harmful. The circular heat map shows the p-values of five methods from the inside to the outside, including the p-values of WMODE, MLE, WM, MR-Egger and IVW, respectively. The p-value<0.05 is considered to be significant. Each separate block corresponds to a different level of gut microbiota, including phylum, class, order, family and genus. IVW, inverse variance weighting estimation; WMODE, weighted mode, MLE, maximum likelihood estimation; WM, weighted median.
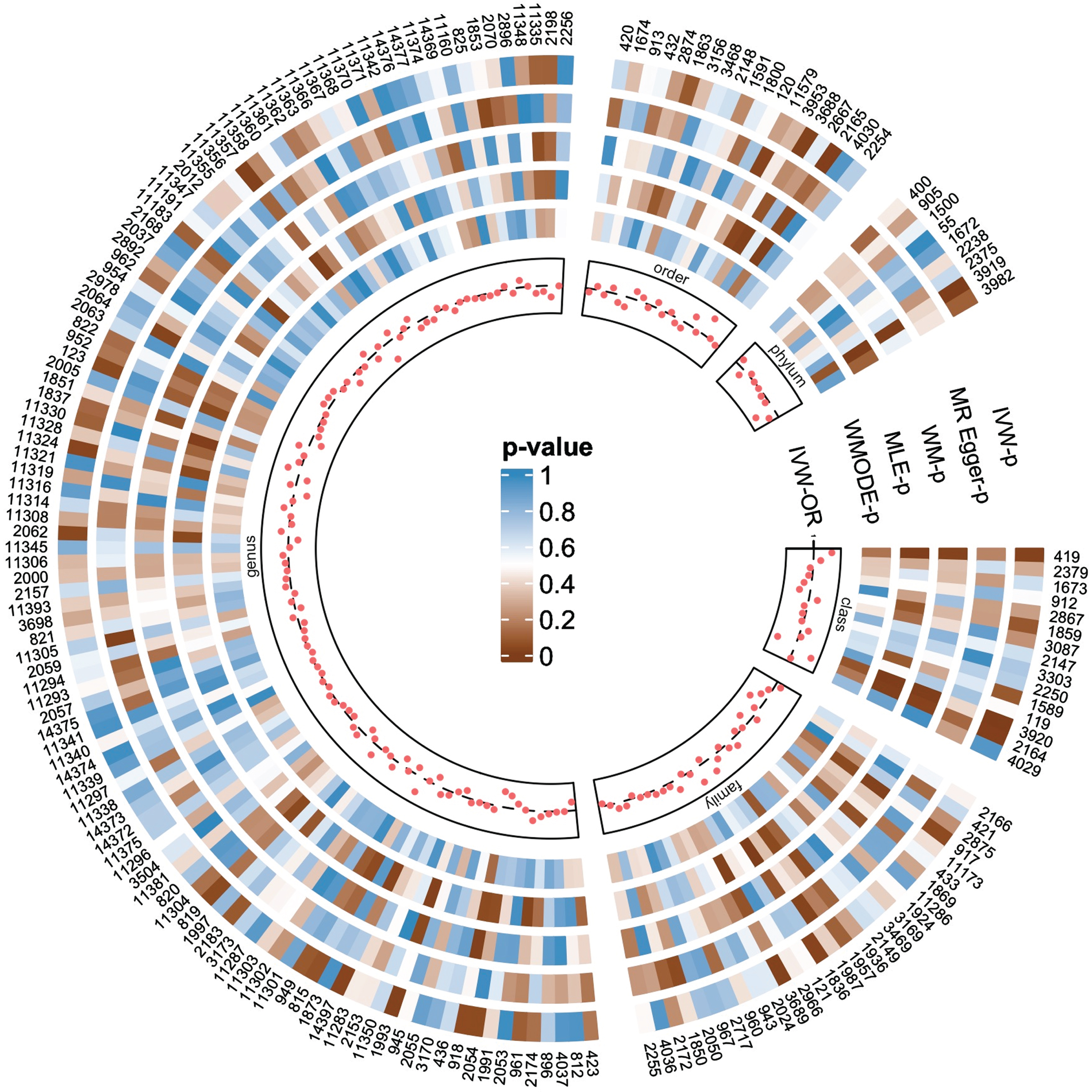
Fig. 3
Forest plot of MR estimate for the causal effect of seven gut microbiota on the risk of Alzheimer’s disease. OR, odds ratio; 95% CI, 95% confidence interval; IVW, inverse variance weighting estimation; WM, weighted median; MLE, maximum likelihood estimation; WMODE, weighted mode.

On the one hand, the IVW method showed three gut microbiota taxa had a positive causal effect on AD risk: Order Selenomonadales (OR 1.13, 95% CI 1.03–1.24, p = 0.01), Family Pasteurellaceae (OR 1.07, 95% CI 1.01–1.13, p = 0.01), and Genus Methanobrevibacter (OR 1.07, 95% CI 1.00–1.13, p = 0.04). These results suggest that the increased level of the three gut microbiota may result in an increased risk of AD. On the other hand, the IVW method showed four other gut microbiota taxa had a negative causal effect on AD risk: Class Mollicutes (OR 0.87, 95% CI 0.79–0.95, p = 0.00), Genus Ruminiclostridium9 (OR 0.87, 95% CI 0.78–0.97, p = 0.01), Genus Clostridiuminnocuumgroup (OR 0.94, 95% CI 0.89–0.99, p = 0.03), and Genus Eggerthella (OR 0.94, 95% CI 0.89–1.00, p = 0.04). These results suggest that these four other gut microbiota taxa may potentially exert a significant influence on resisting AD. The results of the four other methods, i.e., the MR-Egger, WM, MLE and WMODE, demonstrated consistency with the primary IVW analysis, hence increasing the credibility of the revealed causal associations. Although all causal effects were not significant after Bonferroni correction for multiple tests, the ps which were smaller than 0.05 across several methods might still indicate potential causal relationships. Details of the findings are in Supplementary Table 2.
Sensitivity analyses of pleiotropy and heterogeneity
The comprehensive outcomes of the sensitivity assessments are presented in Supplementary Table 2. According to the MR-Egger regression, there was no indication that the IVs associated with the seven significant gut microbiota taxa had any pleiotropic effect on AD, which was coincided with the analysis of MR-PRESSO. Moreover, Cochran’s Q tests also showed no evidence for any heterogeneity in the analysis (Table 1). These results further showed the robustness of the significant causal effects of the seven specific gut microbiota species on AD.
Table 1
Sensitivity analyses of MR results between gut microbiota and Alzheimer’s disease risk
Gut microbiota | Classification | Pleiotropy, p | Heterogeneity (Cochran’s Q test), p | ||
MR-Egger intercept | MR-PRESSO | IVW | MR-Egger | ||
Mollicutes | Class | 0.94 | 0.19 | 0.14 | 0.10 |
Pasteurellaceae | Family | 0.80 | 0.83 | 0.82 | 0.76 |
Clostridiuminnocuumgroup | genus | 0.96 | 0.76 | 0.75 | 0.65 |
Eggerthella | genus | 0.28 | 0.85 | 0.85 | 0.90 |
Methanobrevibacter | genus | 0.64 | 0.97 | 0.97 | 0.95 |
Ruminiclostridium9 | genus | 0.25 | 0.40 | 0.37 | 0.42 |
Selenomonadales | order | 0.38 | 0.70 | 0.65 | 0.65 |
IVW, inverse variance weighting estimation; MR-PRESSO, Mendelian randomization pleiotropy residual sum and outlier.
Reverse Mendelian randomization
In order to rule out the possibility of reverse causal effects and further validate the previous results, we performed a reverse MR analysis employing the IVW method. After removing the SNPs in linkage disequilibrium, we identified 141 SNPs possessing F-statistics exceeding 10, as showed in Supplementary Table 3.
No statistically significant reverse causality was found between AD and each of these seven specific gut microbiota species, including Class Mollicutes (p = 0.14), Family Pasteurellaceae (p = 0.62), Genus Clostridiuminnocuumgroup (p = 0.42), Genus Eggerthella (p = 0.06), Genus Methanobrevibacter (p = 0.52), Genus Ruminiclostridium9 (p = 0.93), and Order Selenomonadales (p = 0.33). Furthermore, we detected no horizontal pleiotropy in the rest of the examined gut microbiota (Supplementary Table 4). These results further indicated the absence of the bias induced by potential unknown confounders.
DISCUSSION
Our bidirectional MR study revealed potential causal effects of the presence of seven gut microbiota species on AD. Specifically, Order Selenomonadales, Family Pasteurellaceae, and Genus Methanobrevibacter were found to have a possible association with elevated likelihood of developing AD, while Class Mollicutes, Genus Ruminiclostridium9, Genus Clostridiuminnocuumgroup, and Genus Eggerthella exert a protective influence against the onset of AD. Furthermore, no statistically significant reverse causality was found between AD and each of these seven specific gut microbiota species. These findings suggested that these gut microbiota could offer novel avenues for the development of therapeutic and preventive strategies for AD.
Weighted median estimation is more robust than the IVW estimation if there exist pleiotropic IVs in MR studies. Based on this fact, we only focus on Selenomonadales and Mollicutes, which have showed a statistically significant difference in both the IVW method and weighted median estimation, in the literature validation. Chen et al. found that for the oral microbiomes, order Selenomonadales exhibited a gradual rise from individuals with normal cognition to those with mild AD, and subsequently to those with moderate AD [38]. This further corroborated our findings regarding the Selenomonadales group, which markedly elevates the risk of AD. Besides, Zhang et al. suggested that elevated fecal levels of Mollicutes could serve as valuable biomarkers for excluding [39].
To our knowledge, this study represents the initial exploration into the causality of gut microbiota and AD risk through MR approach. We uncovered a causal association between some common gut microbiota species and AD, with certain gut microbiota actively contributing to disease progression. A notable strength of our study lies in the rigorous implementation of MR methods, adeptly inferring the direction of causation, thereby yielding more precise conclusions about causal inferences.
While the MR design is generally less prone to confounding factors compared to other observational studies, there are limitations. First, relying on summary level statistics in GWAS databases instead of raw clinical data limits the capacity for further analyses on different forms of the disease. Second, because of the limited gut microbiota data sources, the same dataset is used for both selecting SNPs and estimating the impact size of the SNPs, resulting in potential selection bias. Third, the inclusion of primarily individuals with European descent in GWAS studies may lead to bias, as changes in the composition of the dominant intestinal microbiota, which are affected by different exposures such as dietary habits, may not be fully represented. Fourth, considering that AD typically manifests in older age, participants would need to have survived the exposure in order to be recruited, which may introduce bias. Finally, because the pathobiology of AD is complex, stringent multiple testing corrections might inadvertently overlook gut microbiota causally linked to AD. To address this concern, we opted not to apply multiple corrections.
In summary, this study unveiled a causal relationship between particular gut microbiota and the risk of AD. These findings have clinical implications, because they may provide novel avenues for advancing therapeutic approaches in clinical settings for AD and strategies to prevent AD. Whether AD has a causal impact on the composition of the gut microbiota needs more study.
AUTHOR CONTRIBUTIONS
Anqi Chen (Conceptualization; Formal analysis; Methodology; Writing – original draft); Yuquan Wang (Data curation; Formal analysis; Writing – original draft); Yue-Qing Hu (Conceptualization; Project administration; Supervision; Writing – review & editing).
ACKNOWLEDGMENTS
The authors thank the GWAS summary-level data, which have been downloaded from https://gwas.mrcieu.ac.uk/datasets. The authors thank all the reviewers and editors for their useful suggestions for improving this study.
FUNDING
This research was supported partially by the National Key R&D Program of China [2023YFF1205101].
CONFLICT OF INTEREST
The authors have no conflict of interest to report.
DATA AVAILABILITY
GWAS summary-level data is available at https://gwas.mrcieu.ac.uk/datasets.
The R package Mendelian Randomization is available at https://cran.r-project.org/web/packages/MendelianRandomization/index.html.
The R package TwosampleMR is available at https://github.com/MRCIEU/TwoSampleMR. The R package MR-PRESSO is available at https://github.com/rondolab/MR-PRESSO. The source codes to reproduce the analyses can be accessed in the Supplementary Material.
SUPPLEMENTARY MATERIAL
[1] The supplementary material is available in the electronic version of this article: https://dx.doi.org/10.3233/ADR-240071.
REFERENCES
[1] | Sosa-Ortiz AL , Acosta-Castillo I , Prince MJ ((2012) ) Epidemiology of dementias and Alzheimer’s disease. Res 43: , 600–608. |
[2] | Butterfield DA , Halliwell B ((2019) ) Oxidative stress, dysfunctional glucose metabolism and Alzheimer disease. Nat Rev Neurosci 20: , 148–160. |
[3] | Kodamullil AT , Zekri F , Sood M , Hengerer B , Canard L , McHale D , Hofmann-Apitius M ((2017) ) Trial watch: Tracing investment in drug development for Alzheimer disease. Nat Rev Drug Discov 16: , 819–820. |
[4] | Moszak M , Szulińska M , Bogdański P ((2020) ) You are what you eat—The relationship between diet, microbiota, and metabolic disorders—A review. Nutrients 12: , 1096. |
[5] | Cryan JF , O’riordan KJ , Cowan CSM , John F. Sandhu KV , Bastiaanssen TFS , Boehme M , Codagnone MG , Cussotto S , Fulling C , Golubeva AV , Guzzetta KE , Jaggar M , LongSmith CM , Lyte JM , Martin JA , Molinero-Perez A , Moloney G , Morelli VE , Morillas E , O’Connor R , Cruz-Pereira JS , Peterson VL , Rea K , Ritz NL , Sherwin E , Spichak S , Teichman EM , van de Wouw M , Ventura-Silva AP , WallaceFitzsimons SE , Hyland N , Clarke G , Dinan TG ((2019) ) The microbiota-gut-brain axis. Physiol Rev 99: , 1877–2013. |
[6] | Szablewski L ((2018) ) Human gut microbiota in health and Alzheimer’s disease. J Alzheimers Dis 62: , 549–560. |
[7] | Ticinesi A , Nouvenne A , Tana C , Prati B , Meschi T ((2019) ) Gut microbiota and microbiota-related metabolites as possible biomarkers of cognitive aging. In Reviews on Biomarker Studies in Aging and Anti-Aging Research, Guest PC, ed. Springer, Switzerland, pp. 129–154. |
[8] | Shen L , Ji HF ((2019) ) Associations between gut microbiota and Alzheimer’s disease: Current evidences and future therapeutic and diagnostic perspectives. J Alzheimers Dis 68: , 25–31. |
[9] | Jiang C , Li G , Huang P , Liu Z , Zhao B ((2017) ) The gut microbiota and Alzheimer’s disease. J Alzheimers Dis 58: , 1–15. |
[10] | Vogt NM , Kerby RL , Dill-McFarland KA , Harding SJ , Merluzzi AP , Johnson SC , Carlsson CM , Asthana S , Zetterberg H , Blennow K , Bendlin BB , Rey FE ((2017) ) Gut microbiome alterations in Alzheimer’s disease. Sci Rep 7: , 13537. |
[11] | Zhuang ZQ , Shen LL , Li WW , Fu X , Zeng F , Gui L , Lu Y , Cai M , Zhu C , Tan YL , Zheng P , Li HY , Zhu J , Zhou HD , Bu XL , Wang YJ ((2018) ) Gut microbiota is altered in patients with Alzheimer’s disease. J Alzheimers Dis 63: , 1337–1346. |
[12] | Cattaneo A , Cattane N , Galluzzi S , Provasi S , Lopizzo N , Festari C , Ferrari C , Guerra UP , Paghera B , Muscio C , Bianchetti A , Volta GD , Turla M , Cotelli MS , Gennuso M , Prelle A , Zanetti O , Lussignoli G , Mirabile D , Bellandi D , Gentile S , Belotti G , Villani D , Harach T , Bolmont T , Padovani A , Boccardi M , Frisoni GB , INDIA-FBP Group ((2017) ) Association of brain amyloidosis with pro-inflammatory gut bacterial taxa and peripheral inflammation markers in cognitively impaired elderly. Neurobiol Aging 49: , 60–68. |
[13] | Liu S , Gao J , Zhu M , Liu K , Zhang HL ((2020) ) Gut microbiota and dysbiosis in Alzheimer’s disease: Implications for pathogenesis and treatment. Mol Neurobiol 57: , 5026–5043. |
[14] | Birney E ((2021) ) Mendelian randomization. Cold Spring Harb Perspect Med 12: , a041302. |
[15] | Liu X , Tong X , Zou Y , Lin X , Zhao H , Tian L , Jie Z , Wang Q , Zhang Z , Lu H , Xiao L , Qiu X , Zi J , Wang R , Xu X , Yang H , Wang J , Zong Y , Liu W , Hou Y , Zhu S , Jia H , Zhang T ((2022) ) Mendelian randomization analyses support causal relationships between blood metabolites and the gut microbiome. Nat Genet 54: , 52–61. |
[16] | Li P , Wang H , Guo L , Gou X , Chen G , Lin D , Fan D , Guo X , Liu Z ((2022) ) Association between gut microbiota and preeclampsia-eclampsia: A two-sample Mendelian randomization study. BMC Med 20: , 443. |
[17] | Long Y , Tang L , Zhou Y , Zhao S , Zhu H ((2023) ) Causal relationship between gut microbiota and cancers: A two-sample Mendelian randomisation study. BMC Med 21: , 66. |
[18] | Zhuang Z , Gao M , Yang R , Liu Z , Cao W , Huang T ((2021) ) Causal relationships between gut metabolites and Alzheimer’s disease: A bidirectional Mendelian randomization study. Neurobiol Aging 100: , 119.e13–119.e16. |
[19] | Yeung CHC , Schooling CM ((2021) ) Systemic inflammatory regulators and risk of Alzheimer’s disease: A bidirectional Mendelian-randomization study. Int J Epidemiol 50: , 829–840. |
[20] | Wang J , Kurilshikov A , Radjabzadeh D , Turpin W , Croitoru K , Bonder MJ , Jackson MA , Medina-Gomez C , Frost F , Homuth G , Ruhlemann M , Hughes D , Kim HN , MiBioGen Consortium Initiative, Spector TD , Bell JT , Steves CJ , Timpson N , Franke A , Wijmenga C , Meyer K , Kacprowski T , Franke L , Paterson AD , Raes J , Kraaij R , Zhernakova A ((2018) ) Meta-analysis of human genome-microbiome association studies: The MiBioGen consortium initiative. Microbiome 6: , 101. |
[21] | Kurilshikov A , Medina-Gomez C , Bacigalupe R , Radjabzadeh D , Wang J , Demirkan A , Le Roy CI , Raygoza Garay JA , Finnicum CT , Liu X , Zhernakova DV , Bonder MJ , Hansen TH , Frost F , Rühlemann MC , Turpin W , Moon JY , Kim HN , Lüll K , Barkan E , Shah SA , Fornage M , Szopinska-Tokov J , Wallen ZD , Borisevich D , Agreus L , Andreasson A , Bang C , Bedrani L , Bell JT , Bisgaard H , Boehnke M , Boomsma DI , Burk RD , Claringbould A , Croitoru K , Davies GE , van Duijn CM , Duijts L , Falony G , Fu J , van der Graaf A , Hansen T , Homuth G , Hughes DA , Ijzerman RG , Jackson MA , Jaddoe VWV , Joossens M , Jørgensen T , Keszthelyi D , Knight R , Laakso M , Laudes M , Launer LJ , Lieb W , Lusis AJ , Masclee AAM , Moll HA , Mujagic Z , Qibin Q , Rothschild D , Shin H , Sørensen SJ , Steves CJ , Thorsen J , Timpson NJ , Tito RY , Vieira-Silva S , Völker U , Völzke H , Võsa U , Wade KH , Walter S , Watanabe K , Weiss S , Weiss FU , Weissbrod O , Westra HJ , Willemsen G , Payami H , Jonkers D , Arias Vasquez A , de Geus EJC , Meyer KA , Stokholm J , Segal E , Org E , Wijmenga C , Kim HL , Kaplan RC , Spector TD , Uitterlinden AG , Rivadeneira F , Franke A , Lerch MM , Franke L , Sanna S , D’Amato M , Pedersen O , Paterson AD , Kraaij R , Raes J , Zhernakova A ((2021) ) Large-scale association analyses identify host factors influencing human gut microbiome composition. Nat Genet 53: , 156–165. |
[22] | Bellenguez C , Kucukali F , Jansen IE , Kleineidam L , Moreno-Grau S , Amin N , Naj AC , Campos-Martin R , Grenier-Boley B , Andrade V , et al. ((2022) ) New insights into the genetic etiology of Alzheimer’s disease and related dementias. Nat Genet 54: , 412–436. |
[23] | Lake J , Warly Solsberg C , Kim JJ , Acosta-Uribe J , Makarious MB , Li Z , Levine K , Heutink P , Alvarado CX , Vitale D , Kang S , Gim J , Lee KH , Pina-Escudero SD , Ferrucci L , Singleton AB , Blauwendraat C , Nalls MA , Yokoyama JS , Leonard HL ((2023) ) Multi-ancestry meta-analysis and fine-mapping in Alzheimer’s disease. Mol Psychiatry 28: , 3121–3132. |
[24] | Sanna S , van Zuydam NR , Mahajan A , Kurilshikov A , Vich Vila A , Vosa U , Mujagic Z , Masclee AAM , Jonkers D , Oosting M , Joosten LAB , Netea MG , Franke L , Zhernakova A , Fu J , Wijmenga C , McCarthy MI ((2019) ) Causal relationships among the gut microbiome, short-chain fatty acids and metabolic diseases. Nat Genet 51: , 600–605. |
[25] | Genomes Project Consortium ((2010) ) A map of human genome variation from population scale sequencing. Nature 467: , 1061–1073. |
[26] | Burgess S , Thompson SG , CRP CHD Genetics Collaboration ((2011) ) Avoiding bias from weak instruments in Mendelian randomization studies. Int J Epidemiol 40: , 755–764. |
[27] | Zhang J , Wu G , Tang Y , Liu H , Ge X , Peng R , Cao J , Tu D , Su B , Jin S , Jiang G , Zhang C , Bai D ((2023) ) Causal associations between gut microbiota and primary biliary cholangitis: A bidirectional two-sample Mendelian randomization study. Front Microbiol 14: , 1273024. |
[28] | Sanderson E , Glymour MM , Holmes MV , Kang H , Morrison J , Munafò MR , Palmer T , Schooling CM , Wallace C , Zhao Q , Davey SG ((2022) ) Mendelian randomization. Nat Rev Methods Primers 2: , 6. |
[29] | Burgess S , Butterworth A , Thompson SG ((2013) ) Mendelian randomization analysis with multiple genetic variants using summarized data. Genet Epidemiol 37: , 658–665. |
[30] | Bowden J , Davey SG , Haycock PC , Burgess S ((2016) ) Consistent estimation in Mendelian randomization with some invalid instruments using a weighted median estimator. Genet Epidemiol 40: , 304–314. |
[31] | Burgess S , Scott RA , Timpson NJ , Davey SG , Thompson SG , EPIC-InterAct Consortium ((2015) ) Using published data in Mendelian randomization: A blueprint for efficient identification of causal risk factors. Eur J Epidemiol 30: , 543–552. |
[32] | Hartwig FP , Davey SG , Bowden J ((2017) ) Robust inference in summary data Mendelian randomization via the zero modal pleiotropy assumption. Int J Epidemiol 46: , 1985–1998. |
[33] | Verbanck M , Chen CY , Neale B , Do R ((2018) ) Detection of widespread horizontal pleiotropy in causal relationships inferred from Mendelian randomization between complex traits and diseases. Nat Genet 50: , 693–698. |
[34] | Sekirov I , Russell SL , Antunes LCM , Finlay BB ((2010) ) Gut microbiota in health and disease. Physiol Rev 90: , 859–904. |
[35] | Wang J , Luo R , Zhao X , Xia D , Liu Y , Shen T , Liang Y ((2023) ) Association between gut microbiota and primary ovarian insufficiency: A bidirectional two-sample Mendelian randomization study. Front Endocrinol 14: , 1183219. |
[36] | Hemani G , Zheng J , Elsworth B , Wade KH , Haberland V , Baird D , Laurin C , Burgess S , Bowden J , Langdon R , Tan VY , Yarmolinsky J , Shihab HA , Timpson NJ , Evans DM , Relton C , Martin RM , Davey SG , Gaunt TR , Haycock PC ((2018) ) The MR-base platform supports systematic causal inference across the human phenome. eLife 7: , e34408. |
[37] | Yavorska OO , Burgess S ((2017) ) MendelianRandomization: An R package for performing Mendelian randomization analyses using summarized data. Int J Epidemiol 46: , 1734–1739. |
[38] | Chen L , Xu X , Wu X , Cao H , Li X , Hou Z , Wang B , Liu J , Ji X , Zhang P , Li H ((2022) ) A comparison of the composition and functions of the oral and gut microbiotas in Alzheimer’s patients. Front Cell Infect Microbiol 12: , 942460. |
[39] | Zhang Z , Tan X , Sun X , Wei J , Li Q , Wu Z ((2022) ) Isoorientin affects markers of Alzheimer’s disease via effects on the oral and gut microbiota in APP/PS1 mice. J Nutr 152: , 140–152. |