Characterization of Depressive Symptoms in Dementia and Examination of Possible Risk Factors
Abstract
Background:
Depression in individuals with Alzheimer’s disease (AD) is common, distressing, difficult to treat, and inadequately understood. It occurs more frequently in AD than in older adults without dementia. The reasons why some patients develop depression during AD and others do not remain obscure.
Objective:
We aimed to characterize depression in AD and to identify risk factors.
Methods:
We used data from three large dementia focused cohorts: ADNI (n = 665 with AD, 669 normal cognition), NACC (n = 698 with AD, 711 normal cognition), and BDR (n = 757 with AD). Depression ratings were available using the GDS and NPI and in addition for BDR the Cornell. A cut-off of≥8 was used for the GDS and the Cornell Scale for Depression in Dementia,≥6 for the NPI depression sub-scale, and≥2 for the NPI-Q depression sub-scale. We used logistic regression to examine potential risk factors and random effects meta-analysis and an interaction term to look for interactions between each risk factor and the presence of cognitive impairment.
Results:
In individual studies there was no evidence of a difference in risk factors for depressive symptoms in AD. In the meta-analysis the only risk factor which increased the risk of depressive symptoms in AD was previous depression, but information on this was only available from one study (OR 7.78 95% CI 4.03–15.03).
Conclusion:
Risk factors for depression in AD appear to differ to those for depression per se supporting suggestions of a different pathological process, although a past history of depression was the strongest individual risk factor.
INTRODUCTION
Depression and Alzheimer’s disease (AD) are both common mental disorders. The incidence of depression is increased in those with AD compared to older people without dementia, it is distressing for patients, and may increase carer burden [1–3]. Up to 16% of individuals with AD develop depression during their illness [4]. Depression in dementia is difficult to treat, appears to have different symptomatology (e.g., less guilt/worthlessness), and currently available anti-depressants are ineffective [5, 6]. Separate diagnostic criteria have been proposed for depression in AD, but have not been adopted into routine practice [7].
A recent systematic review examining risk factors for depression in AD found only 7 studies, most of which were of relatively low quality. Family and personal history of mental health problems were both found to increase risk [8]. They concluded that the risk factors for depression in AD were largely similar to those for older adults without cognitive impairment, although sex and educational level did not appear to be risk factors for depression in AD. A family history of depression has been one of the most consistently reported risk factors for depression in AD, suggesting a possible genetic predisposition [9, 10].
The underlying biology of depression in AD remains poorly understood. It has been suggested that it may have a vascular component (e.g., [11, 12]). A large case series which used the Hamilton Depression Rating Scale found that depression in AD is not simply a symptom of the dementia [13]. Individuals with depression in AD are more likely than those with AD who are not depressed to develop psychotic symptoms and have also been shown to have increased cortical tangles, which suggests more severe AD pathology [3, 14]. They have also been shown to have reduced perfusion of frontal brain areas and reduced connectivity between the amygdala and the frontal cortex [15, 16].
The reasons why some patients develop depression during AD and others do not remain unknown. The biological mechanisms underlying depression in AD and whether this differs to depression in those without AD also remain unclear.
Aims and objectives
We aimed to characterize depression in individuals with AD using three large cohort studies, to identify risk factors for depression in AD and to assess whether these risk factors differed from those with normal cognition who develop depression.
METHODS
We obtained information from three large dementia focused cohort studies.
National Alzheimer’s Co-ordinating Center
The NACC (https://naccdata.org/) was established in 2005 and collates information from Alzheimer’s Disease Research Centers across the USA using uniform data sets. Individuals with AD, mild cognitive impairment (MCI), and normal are included. Each center has its own enrolment protocol. This can include clinician referral, self-referral, active recruitment by community organizations, and recruitment of volunteers with normal cognition. It is therefore not representative of the general US population. Participants are seen approximately annually.
Depressive symptoms were assessed using the Neuropsychiatric Inventory-Questionnaire (NPI-Q) and the Geriatric Depression Scale (GDS) [17, 18]. Unlike Alzheimer’s Disease Neuroimaging Initiative (ADNI) individuals with depressive symptoms at baseline were not excluded.
Because the current study was part of a larger analysis which included neuroimaging data [19], individuals were included in this study if they had “at least one MRI scan with calculated volumes available, had at least one visit with information on current/previous depression and either had a diagnosis of AD on at least one occasion, a diagnosis of impaired cognition during follow-up or normal cognition at all visits.” [19]. Data was obtained from the September 2019 data freeze.
Alzheimer’s Disease Neuroimaging Initiative
The ADNI (http://www.adni-info.org/) was established in 2004 and is a longitudinal study aimed at developing biomarkers and other indicators for the early detection of AD. It has clinical data including PET and MRI on > 800 individuals [20, 21]. Participants included in the trial had either normal cognition, MCI, or early AD at baseline. After the screening visit participants have assessments at 0, 3, 6, and 12 months before the interval between visits increases to every 6 months [20]. ADNI was carried out in phases; ADNI1 in 2004; ADNI-GO in 2009; ADNI 2 in 2011; and ADNI3 in 2016. Many individuals were seen in more than one phase with existing participants included in the recruitment for each successive phase. There are differences in the data collected in each phase. For example, the shorter form NPI-Q was used in ADNI 1 and ADNI-GO whereas the full NPI was used in ADNI 2 & 3. Individuals meeting criteria for depression either at entry to the study or in the 2 years preceding study entry were an excluded, meaning that very few participants had significant depressive symptoms at baseline. Other exclusion criteria included inability to speak English or Spanish, age < 55 or > 90 at study entry, learning disability, other unstable medical conditions, severe sensory impairment which would impair neuropsychological testing, unwillingness to undergo neuroimaging and lack of a study partner. At study entry participants had to have a Mini-Mental State Examination (MMSE) of > 19.
Depressive symptoms were measured using the GDS and NPI or NPI-Q [17, 18, 22]. The final dataset used in this analysis was downloaded in May 2021.
Brains for Dementia Research
Data was available for BDR via the Dementia Platforms UK portal (https://portal.dementiasplatform.uk). BDR was set up in 2007 to establish a network of brain bank facilities in England and Wales. Participants in BDR were recruited using advertisements in a wide range of media with support from Alzheimer’s Research UK and the Alzheimer’s Society. Participants agreed at study entry to donate their brains after their demise. Exclusion criteria included brain trauma/major stroke, healthy controls aged < 65 (except where partner of participant), insufficient command of the English language to be able to complete assessments and “being geographically too remote from an assessment center.” [23]. Participants are seen roughly annually by either a psychologist or research nurse. Depressive symptoms were measured using the NPI-Q, the GDS and the Cornell Scale for Depression in Dementia [17, 18, 24].
Definition of depressive symptoms
As in our linked study a cut-off of≥8 was used for the GDS and the Cornell Scale for Depression in Dementia [19]. These cut offs were chosen for the best balance of specificity and sensitivity [25, 26]. NPI sub-scale scores were calculated for depression by multiplying the severity and frequency items for each symptom [22]. A cut-off was used of≥2 for the NPI-Q and≥6 for the NPI depression sub-scale. The NPI cut-off of 6 was chosen to ensure that individuals would have to have had depressive symptoms often/frequently/very frequently and at least of moderate severity. Although the NPI is predominantly a screening tool it has previously been used to define clinically significant behavioral and psychological symptoms of dementia and to diagnose depression [27–29].
Depression caseness was defined as scoring above the threshold on either the NPI, GDS, or Cornell. Intermittent depression was defined as “one episode above threshold on any scale and persistent as≥2 episodes above threshold on any scale during follow-up” [19].
The GDS is self-rated and thus can only be completed by individuals who are less cognitively impaired and thus are able to understand the content, recall the previous week, and retain the information for long enough to complete the questionnaire. The Cornell is clinician rated and includes information from both patients and carers. While flawed as a diagnostic tool for depression the NPI allowed us to identify individuals with significant depressive symptoms who were unable to complete the GDS as they were too cognitively impaired [30]. The NPI has been previously used in multiple studies to define depression caseness in those with dementia [31–34], and an NPI depression subscale score of 4 or more has been used as a criterion for entry into clinical trials [35].
In those with normal cognition, the NPI was not used to define depression caseness as it has not been validated in this population.
Dataset exclusions
Because we aimed to study persistent depressive symptoms, we excluded participants with only one study visit and those who failed the screening visit. Individuals with treated depression (i.e., taking antidepressants at baseline but who never scored above the GDS threshold during follow-up) were excluded. This comprised 9 individuals in BDR. In NACC, 18 individuals were taking antidepressants at baseline but were not depressed and 105 were taking antidepressants at least 2 timepoints but never met depression caseness criteria. In older adult psychiatry it is common for antidepressants to be prescribed for 2 years to prevent relapse.
In NACC, in which some participants were seen multiple times before they developed cognitive difficulties, visits prior to the time at which they first developed MCI were not considered for the AD group.
Risk factors under investigation
In our risk factor analysis, we considered demographic and clinical variables which have previously been shown to be associated with the risk of an individual developing depression. These included female sex, years of education, smoking, alcohol consumption, stroke, previous psychiatric illness, previous depression, cardiovascular disease, diabetes, drug use, and neurological illnesses such as Parkinson’s disease [36–43]. Data was not available on all risk factors from each of the included studies.
Statistical analysis
All statistical analyses were carried out in STATA 14 except the meta-analysis, which was conducted in R. Chi squared tests were used to assess differences in baseline categorical variables. Parametric analyses were used wherever possible to assess between group differences in continuous variables. Data was examined for normal distribution using histograms, P normal and Q normal plots and where necessary the Shapiro Wilk test. Where data was not normally distributed and could not be transformed, the Kruskal Wallis test was used.
Logistic regression was used to examine the effects of risk factors on the risk of developing clinically significant depressive symptoms, either intermittent or persistent. In NACC age, sex, psychiatric history, history of depression, ethnicity, and years of education were co-variates in this analysis. In ADNI age, sex, ethnicity, psychiatric history, and years of education were used as co-variates. In BDR, age, sex, ethnicity, and years of education were available as co-variates. Random effects meta-analyses were conducted (to allow for between-cohort variations in data collection) to estimate the average effect of each risk factor on depression across the cohorts (where available). Imputation was not possible for any dataset as the data was missing not at random (Older individuals and those with dementia more likely to drop out of the study).
In a second model, to assess whether risk factors for depressive symptoms differed between individuals with AD and normal cognition, cognition (i.e., the presence/absence of dementia) was added to each logistic regression model as an interaction term.
To assess the effect of persistent low mood, we used trajectory modelling. This accounts for changes in depression scores over time. GDS scores were used as this was the most complete dataset. For the trajectory modelling in the NACC dataset Akaike information criterion (AIC), Bayes information criterion (BIC), and entropy (which assesses model homogeneity) were used to assess the model fit. The model chosen had the lowest AIC, BIC, confidence intervals, no groups with extremely low numbers of participants and an entropy of > 0.7 (see Supplementary Table 5). The odds of correct classification and the average posterior probability for each group were also assessed. Due to missing data, only the first 5 years of time in the study could be modelled in the trajectory analysis. Violin plots and logistic regression were used to assess the influence of trajectory group on NPI-Q sub scale scores. Because ADNI excluded individuals with clinically significant depressive symptoms at study entry and attrition led to missing data on the GDS later in the study, it was not possible to adequately model trajectories of depressive symptoms in ADNI (no model with entropy > 0.7). Due to lower numbers in BDR and individuals completing a mixture of the GDS and Cornell it was again not possible to produce an adequate trajectory model.
The primary outcome was the risk of developing depressive symptoms above the defined threshold. After the a priori analysis, secondary analyses were performed including the analysis of NPI sub scales. These were not included in the power calculation prior to study commencement.
Ethical approvals
All contributing ADCs are required to obtain informed consent from their participants and maintain their own separate IRB review and approval from their institution prior to submitting data to NACC. The NACC database itself is exempt from IRB review and approval.
As this study’s use of ADNI and BDR data fell into the category of secondary analysis of anonymized data, under UK law, no separate ethical approval was required for these analyses.
RESULTS
NACC
As shown in Table 1, 698 individuals with AD were included, 111 of whom met criteria for persistent depressive symptoms. Depressed individuals were more to have experienced depression in the last two years, to be female, and to be taking antidepressants. They also had slightly higher baseline NPI anxiety (mean difference 0.30 in persistent depression group) and apathy scores (mean difference 0.31 in persistent depression group). As shown in Supplementary Table 3, at baseline the AD +/–depression groups had similar scores on cognitive tests such as the MMSE.
Table 1
Baseline characteristics of the cohorts in this
NACC | ADNI | BDR | ||||||||||
AD no depN = 393 | AD intermittent depN = 185 | AD persistent depN = 111 | Statistical Evidence | AD, no depn = 447 | AD, intermittent depn = 153 | AD, persistent depn = 66 | Statistical Evidence | Dementia no depn = 490 | Dementia intermittent depn = 185 | Dementia persistent depn = 82 | Statistical Evidence | |
Age at baseline (mean (SD)) | 78.2 (8.5) | 76.1 (9.4) | 74.4 (10.3) | p = 0.935 | 74.5 (7.6) | 73.8 (7.1) | 74.6 (7.1) | p = 0.579 | 80.0 (9.2) | 77.8 (9.2) | 73.9 (11.3) | p < 0.001 |
Sex | p = 0.035 | p = 0.213 | p = 0.38 | |||||||||
male | 221 (56.2%) | 89 (48.1%) | 49 (44.1%) | 58.17% | 54.90% | 46.97% | 54.1% | 60.0% | 56.1% | |||
female | 172 (43.8%) | 96 (51.9%) | 62 (55.9%) | 41.83% | 45.10% | 53.03% | 45.9% | 40.0% | 43.9% | |||
Marital status | p = 0.30 | p = 0.24 | ||||||||||
married | 256 (65.1%) | 124 (67.0%) | 78 (70.3%) | 83.7% | 81.7% | 83.3% | p = 0.778 | 59.6% | 59.5% | 62.2% | ||
widowed | 80 (20.4%) | 30 (16.2%) | 17 (15.3%) | 10.1% | 10.5% | 10.6% | 25.1% | 25.4% | 20.7% | |||
divorced | 34 (8.7%) | 23 (12.4%) | 8 (7.2%) | 3.8% | 5.9% | 6.1% | 3.3% | 7.0% | 7.3% | |||
separated | 2 (0.5%) | 0 (0.0%) | 2 (1.8%) | |||||||||
other | 5.4% | 4.3% | 5.2% | 2.5% | 2.0% | 0.0% | 12.0% | 8.1% | 9.7% | |||
Ethnicity | p = 0.61 | p = 0.33 | ||||||||||
White | 331 (84.2%) | 165 (89.2%) | 91 (82.0%) | 94.2% | 93.5% | 92.4% | p = 0.974 | 84.3% | 92.0% | 98.8% | ||
Black or African American | 44 (11.2%) | 13 (7.0%) | 16 (14.4%) | 3.1% | 3.3% | 4.6% | 0.0% | 0.0% | 0.0% | |||
native American/Asian | 4.4% | 3.7% | 3.6% | 2.8% | 3.3% | 3.0% | 15.7% | 8.0% | 1.2% | |||
Prescribed an antidepressant | 0 (0.0%) | 59 (31.9%) | 38 (34.2%) | p < 0.001 | 0.0% | 2.6% | 3.0% | p = 0.001 | 0.0% | 4.3% | 7.3% | p = 0.012 |
at baseline | ||||||||||||
GDS score at baseline | 1.5 (1.5) | 2.7 (2.4) | 4.4 (3.5) | Kwallis χ2 = 77.5 p < 0.001 | 1.3 (1.1) | 2.0 (1.6) | 2.4 (1.8) | Kwallis p < 0.001 | 2.5 (2.1) | 4.5 (3.0) | 5.2 (3.3) | Kwallis χ2 = 47.21 p < 0.001 |
NPI depression score at baseline | 0.1 (0.3) | 0.8 (0.9) | 1.1 (1.0) | Kwallis χ2 = 161.4 p < 0.001 | 1.8 (1.0) | 2.6 (2.1) | 2.4 (1.8) | Kwallis p = 0.015 |
In the NACC, there were 711 individuals with normal cognition but numbers with either persistent depressive symptoms (n = 23) or intermittent depressive symptoms (n = 23) were low (see Supplementary Table 4).
ADNI
We included 666 individuals with AD (see Table 1). As shown in Supplementary Table 9, at baseline the AD +/–depression groups had similar scores on cognitive tests such as the MMSE. Few individuals had depressive symptoms at baseline, and depressive symptoms in this cohort developed during follow-up. There was no evidence of a sex difference in those with depression. Individuals with depressive symptoms had higher baseline MMSE scores, lower Clinical Dementia Rating sum of boxes, and a lower (better) Rey Auditory Verbal Learning Test forgetting score. Unlike the NACC cohort, there was no evidence of a difference in baseline NPI apathy and anxiety scores in individuals with depression.
There were 669 individuals without cognitive impairment (Supplementary Table 9), but above threshold depressive symptoms were again low (n = 44 intermittent and n = 10 persistent).
BDR
In the BDR cohort, 757 individuals with dementia were included in this study (Table 1). Unlike NACC and ADNI, probable clinical diagnoses are not available and therefore it was not possible to separate individuals with different types of dementia. Depressed individuals were younger (unlike NACC and ADNI), more likely to live alone/with family, had a slightly higher Hachinski Ischemic index score, have higher MMSE score and lower (better) Basic Activities of Daily Living score at baseline.
Risk factors for depressive symptoms in AD/dementia
In the BDR cohort (which included all dementias), there was evidence that being a smoker increased the risk of depressive symptoms but no evidence that years of education or medical co-morbidities altered the risk (Table 2, Supplementary Table 13, and Fig. 1). In the NACC cohort (Supplementary Table 5 and Fig. 1), history of previous depression/psychiatric disease and being female increased the risk of depression. There was again no evidence that years of education increased the risk. Using an interaction term in NACC, there was no evidence that risk factors for depressive symptoms in AD differed from those with normal cognition (Supplementary Tables 5 and 6), but the numbers with normal cognition and depressive symptoms were low. In the ADNI (Fig. 1, Supplementary Tables 1 and 10), there was only evidence that previous psychiatric illness increased the risk of depressive symptoms in AD.
Table 2
Summary of the risk factor analysis in individuals with dementia in the three cohorts used in this study. Note that information was not available on all co-variates for each study
Study | Risk factor | Total N | N depression | N no depression | Odds ratio | Standard error | Lower end of 95% CI | Upper end of 95% CI | Co-variates used |
NACC | Cardiovascular disease | 604 | 337 | 274 | 0.8569945 | 0.2088732 | 0.5315165 | 1.3817810 | age, gender, ethnicity, psych Hx, education years, Hx depression |
NACC | Diabetes | 605 | 339 | 274 | 0.9279423 | 0.2682048 | 0.5266192 | 1.6351040 | age, gender, ethnicity, psych Hx, education years, Hx depression |
NACC | Stroke or TIA | 601 | 336 | 273 | 1.3818290 | 0.4637573 | 0.7157833 | 2.6676390 | age, gender, ethnicity, psych Hx, education years, Hx depression |
NACC | Depression | 610 | 341 | 275 | 7.7849360 | 0.4637573 | 0.7157833 | 2.6676390 | age, gender, ethnicity, education years, Hx depression |
NACC | Psychiatric problems in past | 610 | 339 | 277 | 0.9937580 | 0.4731924 | 0.3908126 | 2.5269270 | age, gender, ethnicity, psych Hx, education years, |
NACC | Parkinson’s disease | 609 | 340 | 277 | 3.3123620 | 2.5942650 | 0.7136261 | 15.3746300 | age, gender, ethnicity, psych Hx, education years, Hx depression |
NACC | gender female vs. male | 610 | 364 | 296 | 1.5745180 | 0.2928298 | 1.0935550 | 2.2670160 | age, ethnicity, psych Hx, education years, Hx depression |
NACC | alcohol problems yes/no | 609 | 339 | 277 | 1.2890680 | 0.5554154 | 0.5540159 | 2.9993660 | age, gender, ethnicity, psych Hx, education years, Hx depression |
NACC | smoking continuous | 594 | 330 | 271 | 0.9913722 | 0.0064679 | 0.9787760 | 1.0041310 | age, gender, ethnicity, psych Hx, education years, Hx depression |
NACC | years of education | 610 | 364 | 296 | 0.9897973 | 0.0124916 | 0.9656145 | 1.0145860 | age, gender, ethnicity, psych Hx, education years, Hx depression |
ADNI | Psychiatric problems in past | 610 | 399 | 211 | 2.2038450 | 0.3945182 | 1.5516880 | 3.1300970 | age, gender, ethnicity, education years |
ADNI | Neurological problems | 610 | 399 | 211 | 0.9912748 | 0.1927211 | 0.6771824 | 1.4510500 | age, gender, ethnicity, Psych Hx, education years |
ADNI | Endocrine disease incl diabetes | 610 | 399 | 211 | 1.1156480 | 0.1988043 | 0.7867669 | 1.5820050 | age, gender, ethnicity, Psych Hx, education years |
ADNI | Cardiovascular disease | 610 | 399 | 211 | 1.2770240 | 0.2484025 | 0.8722205 | 1.8697000 | age, gender, ethnicity, Psych Hx, education years |
ADNI | alcohol problems yes/no | 610 | 399 | 211 | 1.5679050 | 0.6334404 | 0.7102816 | 3.4610580 | age, gender, ethnicity, Psych Hx, education years |
ADNI | smoker vs. non smoker | 610 | 399 | 211 | 1.2875260 | 0.2334726 | 0.9024097 | 1.8369950 | age, gender, ethnicity, Psych Hx, education years |
ADNI | gender female vs. male | 610 | 399 | 211 | 1.2195750 | 0.2210752 | 0.8548885 | 1.7398340 | age, gender, ethnicity, Psych Hx, education years |
ADNI | years of education | 610 | 399 | 211 | 1.0469540 | 0.0332799 | 0.9837173 | 1.1142560 | age, gender, ethnicity, Psych Hx, education years |
BDR | diabetes | 350 | 259 | 122 | 0.5400425 | 0.2317642 | 0.2328772 | 1.2523590 | age, gender, ethnicity, education years |
BDR | gender female vs. male | 397 | 263 | 142 | 0.9781605 | 0.2181267 | 0.6318222 | 1.5143470 | age, ethnicity, education years |
BDR | smoking mod vs. non | 341 | 227 | 119 | 1.5450070 | 0.4583438 | 0.8637972 | 2.7634330 | age, gender, ethnicity, education years |
BDR | smoking heavy vs. none | 341 | 227 | 119 | 1.7359670 | 0.5995392 | 0.8822014 | 3.4159790 | age, gender, ethnicity, education years |
BDR | alcohol problems yes/no | 344 | 234 | 115 | 1.0128510 | 0.3961941 | 0.4705255 | 2.1802570 | age, gender, ethnicity, education years |
BDR | years of education | 397 | 263 | 142 | 0.9210577 | 0.0319945 | 0.8604367 | 0.9859496 | age, gender, ethnicity |
BDR | Cardiovascular disease | 349 | 234 | 121 | 0.9424967 | 0.3238102 | 0.4806574 | 1.8480940 | age, gender, ethnicity, education years |
BDR | Parkinson’s disease | 344 | 232 | 121 | 1.3942030 | 0.7819150 | 0.4644552 | 4.1851200 | age, gender, ethnicity, education years |
BDR | Stroke | 323 | 222 | 106 | 1.4276510 | 0.6023836 | 0.6244042 | 3.2642120 | age, gender, ethnicity, education years |
There was again no evidence of an interaction between depressive symptoms and cognitive impairment in any individual study for any of the risk factors examined either in the crude analysis (Supplementary Tables 5, 6, 10, 11, and 14) or when adjustment for co-variates was performed.
Fig. 1
Random effects meta-analysis of the effect of risk factors under investigation for their effect on the development of depression (either intermittent or persistent) in AD from the NACC, ADNI and BDR cohorts. Data was not available for all risk factors in each study. Red boxes represent point estimate (odds ratio) and horizontal lines the 95% confidence intervals. Black diamonds are pooled estimates for each risk factor. Estimates above 1 (on the right-hand side of the y-axis) suggest an increased risk for depression, while estimates below 1 (on the left-hand side of the y-axis) suggests a decreased risk for depression. * ADNI is endocrine diseases including diabetes. †is smoking as smoking years in NACC, whereas in ADNI and BDR it was smoker versus non-smoker ‡Stroke or TIA in NACC.
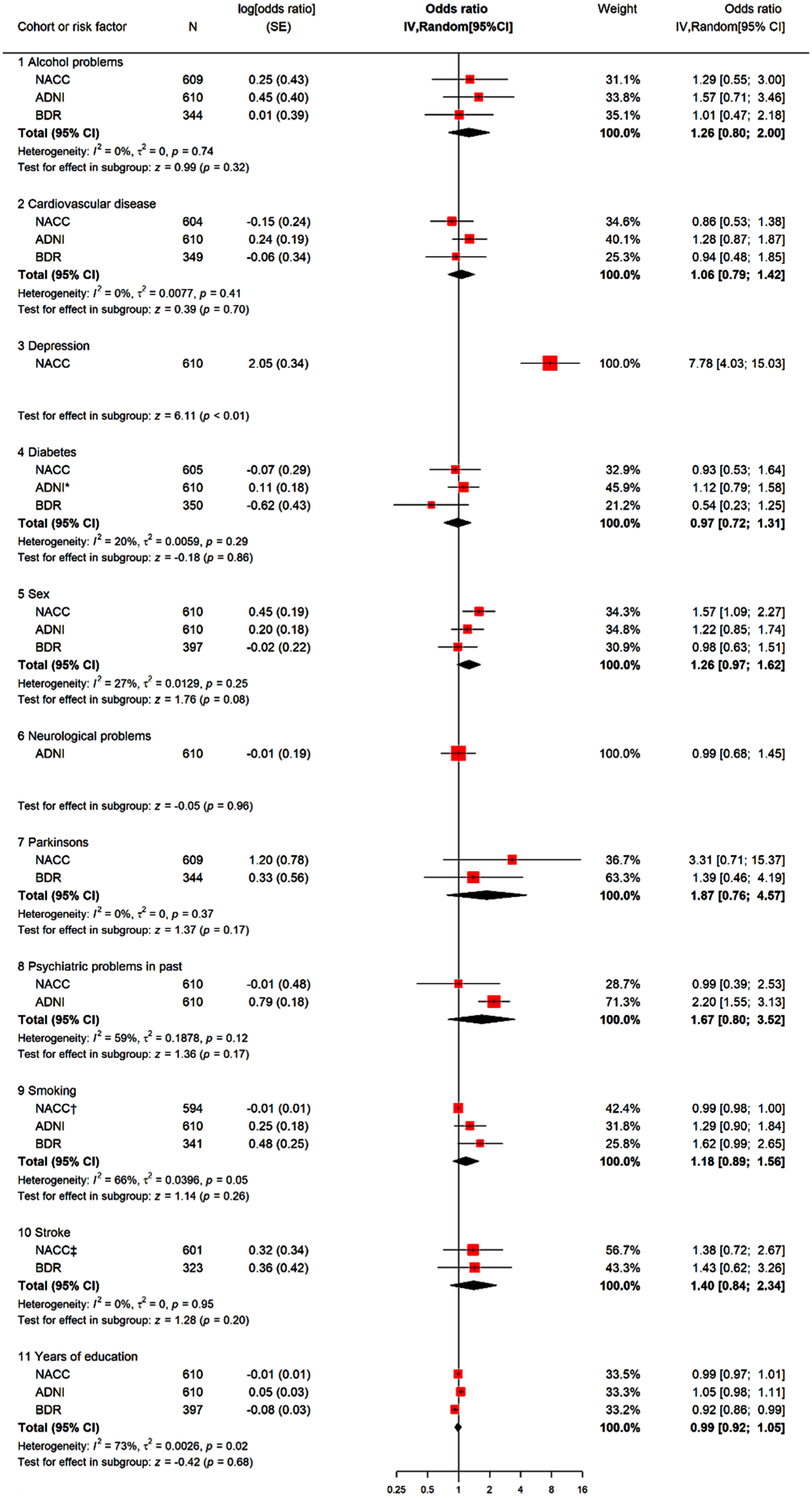
For ease of presentation, odds ratios for each risk factor and cohort, as well as pooled estimates from random-effects meta-analyses (where possible) are presented in Fig. 1. For the risk factors where meta-analyses were possible, there was no strong evidence that any risk factor studied increased the risk of depressive symptoms in those with dementia. It was not possible to meta-analyze the effect of previous depression as data on this risk factor was only available from one study (NACC; OR 7.78, 95% CI 4.03 to 15.03). To explore mediation, we removed previous depression as a co-variate from the NACC analysis (Supplementary Figure 1) and re-ran the meta-analyses. Most effect estimates were unchanged, apart from previous psychiatric problems, which increased the risk of depressive symptoms in AD (OR 2.27, 95 % CI 1.64 to 3.13).
Trajectory modelling in the NACC cohort
Using GDS scores, it was possible to model longitudinal depressive symptoms in the NACC cohort. Because GDS completion requires better cognition there may be a bias towards individuals with higher cognitive performance in the groups with higher depression scores. A 5-group model was the best fit (Supplementary Table 7). The two groups with low depression scores throughout appear to be very similar but the model scores for a 4-group model were inferior. Broadly speaking there were individual with low depression scores at baseline whose scores remained low, those with high scores at baseline who remained depressed and individuals whose GDS scores either decreased or increased during 5-year follow-up. Using violin plots (Fig. 2), it appears that individuals with GDS scores that remained high appeared to be more likely to experience apathy (OR 4.30 (95% CI 1.40 to 13.18) p = 0.011), anxiety, and depressive symptoms on the NPI during follow-up.
Fig. 2
GDS trajectory analysis in NACC (A). Individuals with persistent depressive symptoms during follow-up (group 5) appeared to have more apathy (C), anxiety (D), and NPI rated depression (B) during 5-year follow-up.
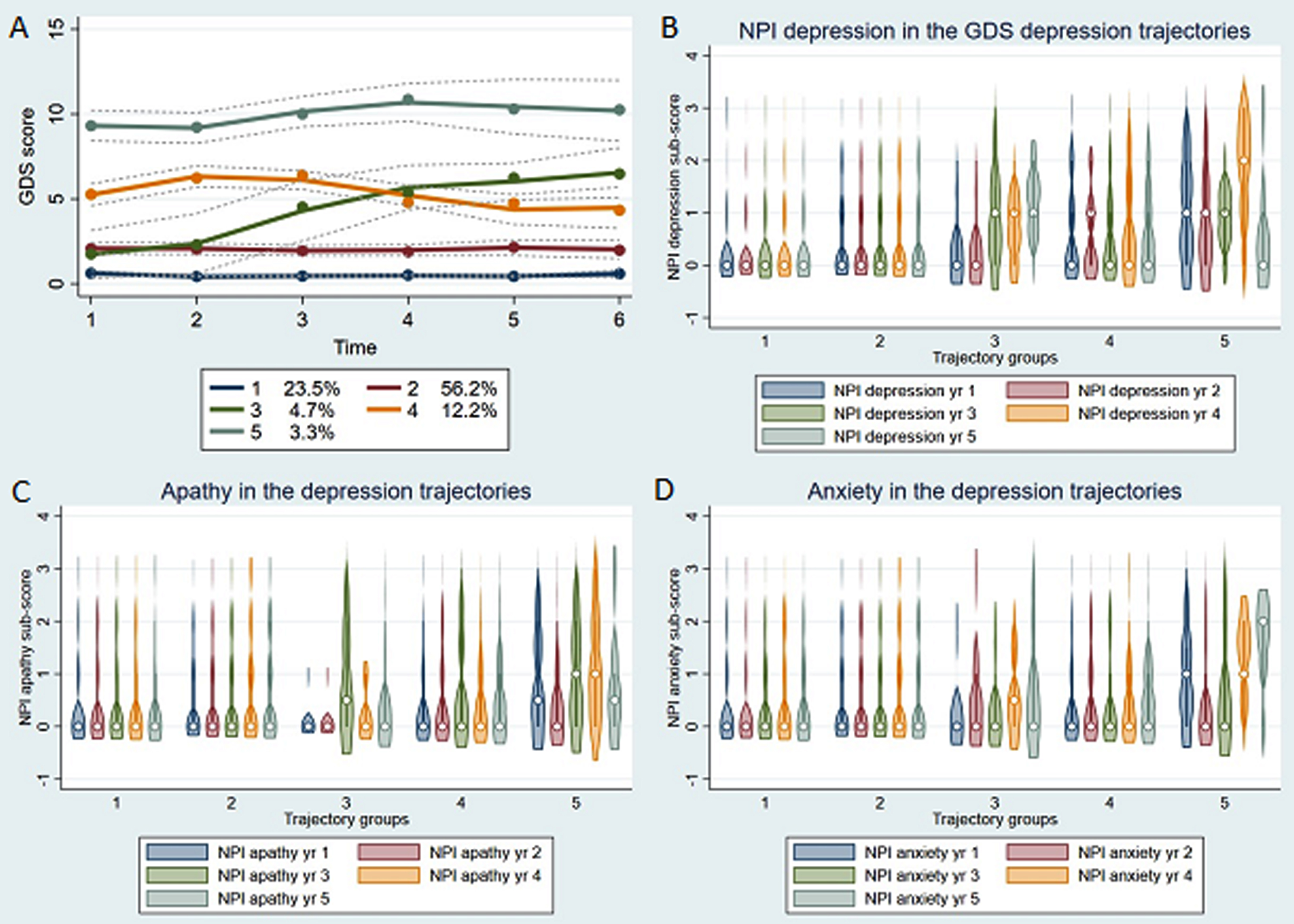
Principal component analysis in the NACC cohort
In our previous study, we used polychoric principal component analysis including NPI and GDS scores to allow for the semi categorical nature of the NPI three factors emerged: factor 1 (the majority of the NPI sub scales), factor 2 (NPI depression, anxiety, and some loading for apathy) and factor 3 (GDS depression) [19].
Although it was not possible to perform trajectory modelling in either ADNI or BDR, it was possible to examine NPI symptoms during follow-up in the BDR cohort (Supplementary Figure 4). The same effect on apathy and anxiety was not seen. This may be because BDR includes many different types of dementia, or a true non replication. Using logistic regression to examine models to examine risk factors for clinically significant NPI symptoms in BDR (Supplementary Table 14), almost all NPI sub-scales were more likely to be clinically significant in those with persistent depression, with some evidence that pathological diagnosis altered this relationship.
DISCUSSION
We have examined three large dementia focused cohorts to identify risk factors for depressive symptoms in AD. In individual studies, using interaction terms, we found no evidence that the risk factors differed from depression per se. A history of previous depression was the risk factor with the best evidence of effect but data on this was only available from one study. There were differences between cohorts (Table 4) that may have been due to recruitment criteria. Although our formal test for whether risk factors for depression differed between those with and without AD was not significant, in the random effects meta-analysis only previous depression increased the risk of depressive symptoms in AD. Information on this was only however available from one study. Other risk factors under examination which have been shown to increase the risk of depression, did not increase the risk of depressive symptoms in AD in the current study.
A previous systematic review which included over 2200 individuals found that only previous psychiatric history and greater cognitive impairment were predictive of depression in dementia. Like us they found no evidence that sex, diabetes, or cardiovascular risk increased the risk of depression [8]. Our study adds to the evidence that risk factors for depression appear to be different to the wider older adult population. For example, female sex, alcohol use, and stroke are well established as risk factors for depression in the general population.
We have not formally commented on the rates of depression in each cohort as they are not representative of the general population. It was noticeable, however, that depression was more common in those with AD in each cohort, but as controls in these cohorts are volunteers who may differ from the wider population (e.g., more highly educated), this may account for some of the difference observed.
In our previous study, we found evidence using a post hoc principal component analysis that depression in AD may be linked to apathy and anxiety more than other NPI sub scales [19]. In the current study, we demonstrated in the BDR cohort that almost all NPI sub scales were more likely to be clinically significant in those with depressive symptoms. Depressive symptoms have been previously shown to vary during follow-up [44, 45]. It has also previously been demonstrated that factor analysis may yield different combinations at baseline to during follow-up [46, 47]. It has been suggested that future research should consider pairs or small groups of symptoms when attempting to understand the underlying biology.
Strengths of this study include the use of multiple cohorts, the use of higher thresholds than some previous studies to define depression so that only clinically significant depressive symptoms were included, the larger size compared to some previous studies and the length of follow-up data available. This allowed a more longitudinal view of depression in AD. Limitations include the relatively low numbers of individuals without dementia who developed depressive symptoms (although this is consistent with the known prevalence) and the low numbers of individuals with persistent depressive symptoms in ADNI (due to the study entry criteria). Due to nature of recruitment for NACC, BDR, and ADNI, individuals with normal cognition in these studies are likely not to be representative of the normal population. In addition, low numbers of individuals with normal cognition, including those with normal cognition and depressive symptoms, are likely to have limited the power of some of our analyses, particularly the analysis using the presence/absence of dementia as an interaction term to assess whether the risk factors for depressive symptoms differed between those with and without dementia. Numbers were especially low for those with normal cognition and depression in ADNI. This limits our conclusions on whether the risk factors for depressive symptoms truly differ between those with and without AD. While we have carried out some secondary, post hoc analyses we have clearly identified which analyses these were and have advised that the results be treated with caution until replicated.
Future work should include a more detailed examination of depression in AD in relation to vascular risk factors in larger cohorts or in a meta-analysis, examination of regional atrophy and examination of the genetic underpinnings of depression in AD.
To conclude, we have used data from three large cohorts to study depression in AD. We have identified that a past history of depression is the strongest risk factor for the development of depressive symptoms in AD.
ACKNOWLEDGMENTS
We are immensely grateful to the participants, volunteers, and staff of NACC, ADNI, and BDR without whom this research would not have been possible.
FUNDING
LS is funded by a junior fellowship from the Alzheimer’s Society (grant no. 518) and by the Elizabeth Blackwell Institute (University of Bristol) and the Wellcome Trust Strategic Institutional Support fund. ML has received research funding from the MS Society, Parkinson’s UK, and the NIHR HTA. CB is funded by the NIHR. JP is supported by a British Heart Foundation Accelerator Award (AA/18/7/34219) and works in a Unit that is supported by the University of Bristol and UK Medical Research Council (MC_UU_00011/6).
Data collection and sharing for this project was funded by the Alzheimer’s Disease Neuroimaging Initiative (ADNI) (National Institutes of Health Grant U01 AG024904) and DOD ADNI (Department of Defense award number W81XWH-12-2-0012). ADNI is funded by the National Institute on Aging, the National Institute of Biomedical Imaging and Bioengineering, and through generous contributions from the following: AbbVie, Alzheimer’s Association; Alzheimer’s Drug Discovery Foundation; Araclon Biotech; BioClinica, Inc.; Biogen; Bristol-Myers Squibb Company; CereSpir, Inc.; Cogstate; Eisai Inc.; Elan Pharmaceuticals, Inc.; Eli Lilly and Company; EuroImmun; F. Hoffmann-La Roche Ltd and its affiliated company Genentech, Inc.; Fujirebio; GE Healthcare; IXICO Ltd.; Janssen Alzheimer Immunotherapy Research & Development, LLC.; Johnson & Johnson Pharmaceutical Research & Development LLC.; Lumosity; Lundbeck; Merck & Co., Inc.; Meso Scale Diagnostics, LLC.; NeuroRx Research; Neurotrack Technologies; Novartis Pharmaceuticals Corporation; Pfizer Inc.; Piramal Imaging; Servier; Takeda Pharmaceutical Company; and Transition Therapeutics. The Canadian Institutes of Health Research is providing funds to support ADNI clinical sites in Canada. Private sector contributions are facilitated by the Foundation for the National Institutes of Health (http://www.fnih.org). The grantee organization is the Northern California Institute for Research and Education, and the study is coordinated by the Alzheimer’s Therapeutic Research Institute at the University of Southern California. ADNI data are disseminated by the Laboratory for Neuro Imaging at the University of Southern California.
The NACC database is funded by NIA/NIH Grant U24 AG072122. NACC data are contributed by the NIA-funded ADCs: P50 AG005131 (PI James Brewer, MD, PhD), P50 AG005133 (PI Oscar Lopez, MD), P50 AG005134 (PI Bradley Hyman, MD, PhD), P50 AG005136 (PI Thomas Grabowski, MD), P50 AG005138 (PI Mary Sano, PhD), P50 AG005142 (PI Helena Chui, MD), P50 AG005146 (PI Marilyn Albert, PhD), P50 AG005681 (PI John Morris, MD), P30 AG008017 (PI Jeffrey Kaye, MD), P30 AG008051 (PI Thomas Wisniewski, MD), P50 AG008702 (PI Scott Small, MD), P30 AG010124 (PI John Trojanowski, MD, PhD), P30 AG010129 (PI Charles DeCarli, MD), P30 AG010133 (PI Andrew Saykin, PsyD), P30 AG010161 (PI David Bennett, MD), P30 AG012300 (PI Roger Rosenberg, MD), P30 AG013846 (PI Neil Kowall, MD), P30 AG013854 (PI Robert Vassar, PhD), P50 AG016573 (PI Frank LaFerla, PhD), P50 AG016574 (PI Ronald Petersen, MD, PhD), P30 AG019610 (PI Eric Reiman, MD), P50 AG023501 (PI Bruce Miller, MD), P50 AG025688 (PI Allan Levey, MD, PhD), P30 AG028383 (PI Linda Van Eldik, PhD), P50 AG033514 (PI Sanjay Asthana, MD, FRCP), P30 AG035982 (PI Russell Swerdlow, MD), P50 AG047266 (PI Todd Golde, MD, PhD), P50 AG047270 (PI Stephen Strittmatter, MD, PhD), P50 AG047366 (PI Victor Henderson, MD, MS), P30 AG049638 (PI Suzanne Craft, PhD), P30 AG053760 (PI Henry Paulson, MD, PhD), P30 AG066546 (PI Sudha Seshadri, MD), P20 AG068024 (PI Erik Roberson, MD, PhD), P20 AG068053 (PI Marwan Sabbagh, MD), P20 AG068077 (PI Gary Rosenberg, MD), P20 AG068082 (PI Angela Jefferson, PhD), P30 AG072958 (PI Heather Whitson, MD), P30 AG072959 (PI James Leverenz, MD).
CONFLICT OF INTEREST
LS has no relevant conflicts of interest to disclose. She has previously received a travel award from the RCPsych/Gatsby Foundation and is the finance office for the Academic Faculty of the Royal College of Psychiatrists. She has received research funding in the past from the Mason Medical Research Foundation, the David Telling Charitable Trust, BRACE Alzheimer’s Research and the British Neuropathological Society.
ML has no relevant conflicts of interest to disclose. He has received research funding from the MS Society, Parkinson’s UK and the NIHR HTA.
JP has no relevant conflicts of interest to disclose.
During the last 3 years, Clive Ballard has received consulting fees from Acadia pharmaceutical company, AARP, Addex pharmaceutical company, Eli Lily, Enterin pharmaceutical company, GWPharm, H.Lundbeck pharmaceutical company, Novartis pharmaceutical company, Janssen Pharmaceuticals, Johnson and Johnson pharmaceuticals, Novo Nordisk pharmaceutical comapny, Orion Corp pharmaceutical company, Otsuka America Pharm Inc, Sunovion Pharm. Inc, Suven pharmaceutical company, Roche pharmaceutical company, Biogen pharmaceutical company, Synexus clinical research organization and tauX pharmaceutical company and research funding from synexus clinical research organization, Roche pharmaceutical company, Novo Nordisk pharmaceutical company, Novartis pharmaceutical company, Medical research council (UK), Wellcome trust (UK), National Institute for Health Research (UK), National Institute for Health (US), IMI (Eu), Michael J Fox foundation (US), Alzheimer’s Disease Drug Discovery foundation (US), Alzheimer’s Society (UK), Parkinson’s Society (UK), Alzheimer’s Research UK, the Gilling’s foundation and BRACE (UK)
DATA AVAILABILITY
The data used in this study was obtained from ADNI (www.loni.ucla.edu/ADNI), the NACC (https://naccdata.org/), and Dementia Platforms UK (https://portal.dementiasplatform.uk). Data is available free of charge to bona fide researchers who submit a research proposal.
SUPPLEMENTARY MATERIAL
[1] The supplementary material is available in the electronic version of this article: https://dx.doi.org/10.3233/ADR-239000.
REFERENCES
[1] | Sousa MFB , Santos RL , Turró-Garriga O , Dias R , Dourado MCN , Conde-Sala JL ((2016) ) Factors associated with caregiver burden: Comparative study between Brazilian and Spanish caregivers of patients with Alzheimer’s disease (AD). Int Psychogeriatr 28: , 1363–1374. |
[2] | Dudas R , Malouf R , McCleery J , Dening T ((2018) ) Antidepressants for treating depression in dementia. Cochrane Database Syst Rev 8: , CD003944. |
[3] | Zubenko GS , Zubenko WN , McPherson S , Spoor E , Marin DB , Farlow MR , Smith GE , Geda YE , Cummings JL , Petersen RC , Sunderland T ((2003) ) A collaborative study of the emergence and clinical features of the major depressive syndrome of Alzheimer’s disease. Am J Psychiatry 160: , 857–866. |
[4] | Asmer MS , Kirkham J , Newton H , Ismail Z , Elbayoumi H , Leung RH , Seitz DP ((2018) ) Meta-analysis of the prevalence of major depressive disorder among older adults with dementia. J Clin Psychiatry 79: , 17r11772. |
[5] | Banerjee S , Hellier J , Romeo R , Dewey M , Knapp M , Ballard C , Baldwin R , Bentham P , Fox C , Holmes C , Katona C , Lawton C , Lindesay J , Livingston G , McCrae N , Moniz-Cook E , Murray J , Nurock S , Orrell M , O’Brien J , Poppe M , Thomas A , Walwyn R , Wilson K , Burns A ((2013) ) Study of the use of antidepressants fordepression in dementia: The HTA-SADD trial–a multicentre,randomised, double-blind, placebo-controlled trial of the clinicaleffectiveness and cost-effectiveness of sertraline and mirtazapine. Health Technol Assess 17: , 1–166. |
[6] | Haupt M , Kurz A , Greifenhagen A ((1995) ) Depression in Alzheimer’s disease: Phenomenological features and association with severity and progression of cognitive and functional impairment. Int J Geriatr Psychiatry 10: , 469–476. |
[7] | Olin JT , Schneider LS , Katz IR , Meyers BS , Alexopoulos GS , Breitner JC , Bruce ML , Caine ED , Cummings JL , Devanand DP , Krishnan KRR , Lyketsos CG , Lyness JM , Rabins PV , Reynolds CF , Rovner BW , Steffens DC , Tariot PN , Lebowitz BD ((2002) ) Provisional diagnostic criteria for depression of Alzheimer disease. Am J Geriatr Psychiatry 10: , 125–128. |
[8] | Steck N , Cooper C , Orgeta V ((2018) ) Investigation of possible risk factors for depression in Alzheimer’s disease: A systematic review of the evidence. J Affect Disord 236: , 149–156. |
[9] | Pearlson G , Ross C , Lohr W , Royner B , Chase G , Folstein M ((1990) ) Association between family history of affective disorder and the depressive syndrome of Alzheimer’s disease. Am J Psychiatry 147: , 452–456. |
[10] | Strauss M , Ogrocki P ((1996) ) Confirmation of an association between family history of affective disorder and the depressive syndrome in Alzheimer’s disease. Am J Psychiatry 153: , 1340–1342. |
[11] | Lee JJ , Lee EY , Lee SB , Park JH , Kim TH , Jeong H-G , Kim JH , Han JW , Kim KW ((2015) ) Impact of white matter lesions on depression in the patients with Alzheimer’s disease. Psychiatry Invest 12: , 516–522. |
[12] | Anor CJ , O’Connor S , Saund A , Tang-Wai DF , Keren R , Tartaglia MC ((2017) ) Neuropsychiatric symptoms in Alzheimer disease, vascular dementia, and mixed dementia. Neurodegener Dis 17: , 127–134. |
[13] | Chemerinski E , Petracca G , Sabe L , Kremer J , Starkstein SE ((2001) ) The specificity of depressive symptoms in patients with Alzheimer’s disease. Am J Psychiatry 158: , 68–72. |
[14] | Rapp MA , Schnaider-Beeri M , Purohit DP , Perl DP , Haroutunian V , Sano M ((2008) ) Increased neurofibrillary tangles in patients with Alzheimer disease with comorbid depression. Am J Geriatr Psychiatry 16: , 168–174. |
[15] | Levy-Cooperman N , Burhan AM , Rafi-Tari S , Kusano M , Ramirez J , Caldwell C , Black SE ((2008) ) Frontal lobe hypoperfusion and depressive symptoms in Alzheimer disease. J Psychiatry Neurosci 33: , 218–226. |
[16] | Guo Z , Zhang J , Liu X , Hou H , Cao Y , Wei F , Li J , Chen X , Shen Y , Chen W ((2015) ) Neurometabolic characteristics in the anterior cingulate gyrus of Alzheimer’s disease patients with depression: A (1)H magnetic resonance spectroscopy study. BMC Psychiatry 15: , 306. |
[17] | Kaufer DI , Cummings JL , Ketchel P , Smith V , MacMillan A , Shelley T , Lopez OL , DeKosky ST ((2000) ) Validation of the NPI-Q, a briefclinical form of the Neuropsychiatric Inventory. JNeuropsychiatry Clin Neurosci 12: , 233–239. |
[18] | Yesavage JA , Brink TL , Rose TL , Lum O , Huang V , Adey M , Leirer VO ((1982) ) Development and validation of a geriatric depression screening scale: A preliminary report. J Psychiatr Res 17: , 37–49. |
[19] | Sinclair LI , Ballard CG ((2023) ) Persistent depressive symptoms are associated with frontal regional atrophy in patients with Alzheimer’s disease.e. Int J Geriatr Psychiatry 38: , 5858. |
[20] | Petersen RC , Aisen PS , Beckett LA , Donohue MC , Gamst AC , Harvey DJ , Jack CR , Jagust WJ , Shaw LM , Toga AW , Trojanowski JQ , Weiner MW ((2010) ) Alzheimer’s Disease Neuroimaging Initiative (ADNI): Clinical characterization. Neurology 74: , 201–209. |
[21] | Misra C , Fan Y , Davatzikos C ((2009) ) Baseline and longitudinal patterns of brain atrophy in MCI patients, and their use in prediction of short-term conversion to AD: Results from ADNI. Neuroimage 44: , 1415–1422. |
[22] | Cummings JL , Mega M , Gray K , Rosenberg-Thompson S , Carusi DA , Gornbein J ((1994) ) The Neuropsychiatric Inventory: Comprehensive assessment of psychopathology in dementia. Neurology 44: , 2308–2314. |
[23] | Francis PT , Costello H , Hayes GM ((2018) ) Brains for Dementia Research: Evolution in a longitudinal brain donation cohort to maximize current and future value. J Alzheimers Dis 66: , 1635–1644. |
[24] | Alexopoulos GS , Abrams RC , Young RC , Shamoian CA ((1988) ) Cornell Scale for Depression in Dementia. Biol Psychiatry 23: , 271–284. |
[25] | Pocklington C , Gilbody S , Manea L , McMillan D ((2016) ) The diagnostic accuracy of brief versions of the Geriatric Depression Scale: A systematic review and meta-analysis. Int J Geriatr Psychiatry 31: , 837–857. |
[26] | Goodarzi ZS , Mele BS , Roberts DJ , Holroyd-Leduc J ((2017) ) Depression case finding in individuals with dementia: A systematic review and meta-analysis. J Am Geriatr Soc 65: , 937–948. |
[27] | Caputo M , Monastero R , Mariani E , Santucci A , Mangialasche F , Camarda R , Senin U , Mecocci P ((2008) ) Neuropsychiatric symptoms in 921 elderly subjects with dementia: A comparison between vascular and neurodegenerative types. Acta Psychiatr Scand 117: , 455–464. |
[28] | Vik-Mo AO , Giil LM , Borda MG , Ballard C , Aarsland D ((2020) ) The individual course of neuropsychiatric symptoms in people with Alzheimer’s and Lewy body dementia: 12-year longitudinal cohort study. Br J Psychiatry 216: , 43–48. |
[29] | Vik-Mo AO , Giil LM , Ballard C , Aarsland D ((2018) ) Course of neuropsychiatric symptoms in dementia: 5-year longitudinal study. Int J Geriatr Psychiatry 33: , 1361–1369. |
[30] | Gallo JL , Schmidt KS , Libon DJ ((2009) ) An itemized approach to assessing behavioral and psychological symptoms in dementia. Am J Alzheimers Dis Other Demen 24: , 163–168. |
[31] | Fischer CE , Kortebi I , Karameh WK , Kumar S , Gallagher D , Golas A , Munoz D , Barfett J , Butters MA , Bowie CR , Flint A , Rajji T , Herrmann N , Pollock BG , Mulsant B , Schweizer TA , Mah L ((2019) ) Examining the link between cardiovascular risk factors and neuropsychiatric symptoms in mild cognitive impairment and major depressive disorder in remission. J Alzheimers Dis 67: , 1305–1311. |
[32] | Steffens DC , Fisher GG , Langa KM , Potter GG , Plassman BL ((2009) ) Prevalence of depression among older Americans: The Aging, Demographics and Memory Study. Int Psychogeriatr 21: , 879–888. |
[33] | Fritze F , Ehrt U , Sønnesyn H , Kurz M , Hortobágyi T , Nore SP , Ballard C , Aarsland D ((2011) ) Depression in mild dementia:Associations with diagnosis, APOE genotype and clinical features. Int J Geriatr Psychiatry 26: , 1054–1061. |
[34] | Arbus C , Gardette V , Bui E , Cantet C , Andrieu S , Nourhashémi F , Schmitt L , Vellas B ((2010) ) Antidepressant use in Alzheimer’s diseasepatients: Results of the REAL.FR cohort. Int Psychogeriatr 22: , 120–128. |
[35] | Schneider LS , Tariot PN , Lyketsos CG , Dagerman KS , Davis KL , Davis S , Hsiao JK , Jeste DV , Katz IR , Olin JT , Pollock BG , Rabins PV , Rosenheck RA , Small GW , Lebowitz B , Lieberman JA ((2001) ) National Institute of Mental Health Clinical Antipsychotic Trials of Intervention Effectiveness (CATIE): Alzheimer disease trial methodology. Am J Geriatr Psychiatry 9: , 346–360. |
[36] | Schubert DS , Foliart RH ((1993) ) Increased depression in multiple sclerosis patients. A meta-analysis. Psychosomatics 34: , 124–130. |
[37] | Dafer RM , Rao M , Shareef A , Sharma A ((2008) ) Poststroke depression. Top Stroke Rehabil 15: , 13–21. |
[38] | Cole MG , Dendukuri N ((2003) ) Risk factors for depression among elderly community subjects: A systematic review and meta-analysis. Am J Psychiatry 160: , 1147–1156. |
[39] | Carney RM , Freedland KE ((2008) ) Depression in patients with coronary heart disease.S. Am J Med 121: , 20–27. |
[40] | Andreoulakis E , Hyphantis T , Kandylis D , Iacovides A ((2012) ) Depression in diabetes mellitus: A comprehensive review. Hippokratia 16: , 205–214. |
[41] | Antar T , Morris HR , Faghri F , Leonard HL , Nalls MA , Singleton AB , Iwaki H ((2021) ) Longitudinal risk factors for developing depressive symptoms in Parkinson’s disease. J Neurol Sci 429: , 117615. |
[42] | Colledge S , Larney S , Peacock A , Leung J , Hickman M , Grebely J , Farrell M , Degenhardt L ((2020) ) Depression, post-traumatic stress disorder, suicidality and self-harm among people who inject drugs: A systematic review and meta-analysis. Drug Alcohol Depend 207: , 107793. |
[43] | Hasin DS , Goodwin RD , Stinson FS , Grant BF ((2005) ) Epidemiology of major depressive disorder: Results from the National Epidemiologic Survey on Alcoholism and Related Conditions. Arch Gen Psychiatry 62: , 1097–1106. |
[44] | Eustace A , Coen R , Walsh C , Cunningham CJ , Walsh JB , Coakley D , Lawlor BA ((2002) ) A longitudinal evaluation of behavioural and psychological symptoms of probable Alzheimer’s disease. Int J Geriatr Psychiatry 17: , 968–973. |
[45] | Haupt M , Kurz A , Jänner M ((2000) ) A 2-year follow-up of behavioural and psychological symptoms in Alzheimer’s disease. Dement Geriatr Cogn Disord 11: , 147–152. |
[46] | Bettney L , Butt S , Morris J , Connolly A , McCollum C , Burns A , Purandare N ((2012) ) Investigating the stability of neuropsychiatric sub-syndromes with progression of dementia: A 2-year prospective study. Int J Geriatr Psychiatry 27: , 1118–1123. |
[47] | Connors MH , Seeher KM , Crawford J , Ames D , Woodward M , Brodaty H ((2018) ) The stability of neuropsychiatric subsyndromes in Alzheimer’s disease. Alzheimers Dement 14: , 880–888. |