Assessing the Potential of EEG in Early Detection of Alzheimer’s Disease: A Systematic Comprehensive Review (2000–2023)
Abstract
Background:
As the prevalence of Alzheimer’s disease (AD) grows with an aging population, the need for early diagnosis has led to increased focus on electroencephalography (EEG) as a non-invasive diagnostic tool.
Objective:
This review assesses advancements in EEG analysis, including the application of machine learning, for detecting AD from 2000 to 2023.
Methods:
Following PRISMA guidelines, a search across major databases resulted in 25 studies that met the inclusion criteria, focusing on EEG’s application in AD diagnosis and the use of novel signal processing and machine learning techniques.
Results:
Progress in EEG analysis has shown promise for early AD identification, with techniques like Hjorth parameters and signal compressibility enhancing detection capabilities. Machine learning has improved the precision of differential diagnosis between AD and mild cognitive impairment. However, challenges in standardizing EEG methodologies and data privacy remain.
Conclusions:
EEG stands out as a valuable tool for early AD detection, with the potential to integrate into multimodal diagnostic approaches. Future research should aim to standardize EEG procedures and explore collaborative, privacy-preserving research methods.
INTRODUCTION
Despite numerous reviews on Alzheimer’s disease (AD) and its diagnosis using electroencephalography (EEG), the rapid evolution of technology and analytical methods from 2000 to 2023 demands a fresh examination. This review is motivated by the continuous advancements in EEG technology, signal processing techniques, and the integration of machine learning algorithms which have significantly improved the diagnostic accuracy of EEG for AD. The novelty of this content lies in the comprehensive analysis of these advancements, highlighting the transition from traditional EEG analysis to sophisticated, automated approaches that promise earlier and more accurate diagnoses.
Recent contributions to the field have introduced new dimensions to the analysis and interpretation of EEG signals. For instance, the application of Hjorth parameters has been shown to effectively characterize the complexity of EEG dynamics in the early stages of AD, potentially serving as a non-invasive and efficient biomarker for the disease.1,2 Furthermore, the enhanced compressibility of EEG signals observed in AD patients underscores the altered neurodynamic and opens up new pathways for compact signal representation and analysis.3
Innovative methodologies that leverage the full spectrum of EEG signal characteristics are continually emerging. One such approach includes an automated differential diagnosis mechanism, which offers a novel perspective on discriminating between mild cognitive impairment (MCI) and AD, employing advanced signal processing and classification techniques.4 Complementary to this, complexity analysis of EEG signals presents a compelling argument for its inclusion in diagnostic protocols, enriching the analytical toolkit available for AD research.5
AD stands as the archetype of neurodegenerative disorders, representing the primary cause of dementia in the elderly population.6 As a progressive disease, it erodes memory and cognitive faculties, leading to eventual loss of independence and personality changes. The pathophysiology of AD is complex and multifaceted, involving hallmark features such as amyloid-β plaques, tau tangles, synaptic dysfunction, and neuronal loss.7 The growing prevalence of AD with an aging global demographic has underscored an urgent need for early diagnostic tools to arrest or mitigate the progression of the disease. The significance of AD is not limited to its clinical manifestations; it also exerts a profound socioeconomic impact, straining healthcare resources and affecting the quality of life of patients and caregivers alike.2,8
Efficacy of EEG in the early detection
The early diagnosis of AD is critical, as it provides a window for potential interventions that could delay the onset of severe symptoms, preserve function, and extend periods of independence.9 Despite extensive research, definitive diagnosis is often reached at a stage where therapeutic options are limited in their efficacy.10,11 Current diagnostic modalities such as magnetic resonance imaging (MRI) and positron emission tomography (PET) scans, while useful, are expensive and not universally accessible. Moreover, these techniques often identify the disease once significant brain changes have occurred, which is typically beyond the stage where interventions are most likely to be beneficial.12,13
Amidst this landscape, EEG emerges as a potential beacon of hope. It is a well-established neurophysiological technique that records electrical activity of the brain and has shown promise in detecting the subtle changes in brain function that precede overt clinical symptoms.2 Research has consistently demonstrated alterations in EEG patterns in individuals with AD compared to healthy controls, including changes in rhythm synchrony, power spectrum, and connectivity.14 These findings have sparked interest in the development of EEG as a tool for the early diagnosis and monitoring of AD.
The purpose of this comprehensive review is to systematically explore and evaluate the advancements and the potential of EEG as a diagnostic tool for AD spanning from the year 2000 to 2023. This period has been marked by significant advancements in both the understanding of AD pathology and neuroimaging technologies, with EEG analysis methodologies becoming increasingly sophisticated. The review aims to synthesize findings from studies that have employed EEG to identify biomarkers of AD, with a focus on the evolution of these methodologies over time. The objective is to determine the of AD, to assess the progression of the disease, and to understand the potential EEG holds as a routine screening tool in clinical settings. Furthermore, the review intends to identify patterns within EEG data that are indicative of AD and to correlate these patterns with disease progression and response to treatment, thereby facilitating individualized patient care strategies.
The rationale for choosing the timeline from 2000 to 2023 is to encapsulate a period of rapid evolution in EEG technology and analysis techniques, alongside a parallel growth in the understanding of AD. This timeline allows for an examination of the initial hypotheses regarding EEG’s diagnostic potential, through to the recent innovations that have transformed this potential into tangible diagnostic applications.15 EEG has been selected for focus due to its unique advantages over other diagnostic modalities. It is relatively low-cost, widely available, and can be performed with minimal discomfort to patients, making it an attractive option for widespread screening.16 Moreover, EEG is sensitive to the earliest changes in brain function, often preceding structural changes seen on MRI or PET scans, thus offering the possibility of an earlier diagnosis of AD.17–19 The review will also underscore the importance of EEG in the broader context of an integrative diagnostic approach. By combining EEG findings with other clinical and biomarker data, there is potential to enhance the diagnostic accuracy and provide a more comprehensive understanding of AD, thus supporting the impetus for further research and innovation in this area.
AD continues to present a formidable challenge in the realm of neurodegenerative disease research, necessitating early and precise diagnostic capabilities for effective management of its progression. EEG, with its non-invasive nature and profound sensitivity to the brain’s electrical dynamics, has advanced as a cornerstone in the investigative exploration of AD. The background of EEG research in AD diagnosis between 2000 and 2023 represents an epoch of transformative development, marked by a synergy of enhanced comprehension of AD’s complexities and technological evolutions in EEG analysis. This epoch has been characterized by a steadily growing recognition of EEG’s ability to detect the early and subtle neurophysiological changes hallmark to AD, establishing EEG as a non-invasive, cost-effective, and widely accessible diagnostictool.
The evolution of the EEG landscape over the past decades has been propelled by pioneering studies focusing on characteristic EEG alterations in AD, which laid the foundational groundwork for the field. These include decreased power across specific frequency bands and disrupted functional connectivity, which have provided early insights into the disease’s impact on brain function.11,20 Building upon these initial discoveries, further research has underscored the diagnostic value of quantitative EEG and the analysis of mutual information in AD patients, progressively unraveling the intricate effects of the disease on neural dynamics.20,21
An enhanced compressibility of EEG signals in AD patients has been noted, a finding that adds a new dimension to signal analysis by highlighting the altered neurodynamic properties of AD, suggesting a potential for novel data representation and analysis methods.3 Moreover, novel methodologies employing Hjorth parameters have emerged, offering a promising direction for the early detection of AD from EEG signals and bolstering the argument for EEG’s place in routine screening.1
Advancements in analytical approaches have facilitated the emergence of automated differential diagnosis methodologies that offer refined mechanisms to distinguish between MCI and AD, leveraging advanced signal processing and classification techniques.4 Additionally, the examination of EEG signal complexity provides a compelling argument for its inclusion in diagnostic protocols, which is a testament to the analytical toolkit available for AD research that is ever-enriching.5
The modern landscape of EEG research in AD diagnosis is further characterized by a theoretical framework that aligns with the neurophysiological alterations associated with the disease. The potential of EEG to capture these changes has only grown more promising with the integration of innovative machine learning algorithms and sophisticated analytical methods. The emphasis on integrative diagnostic strategies, combining EEG with other biomarkers and brain imaging techniques, has seen significant progress, aiming to establish a multimodal approach that enhances diagnostic specificity and sensitivity for AD.22,23
Current challenges in AD detection, such as the necessity for explainable artificial intelligence (XAI) to justify machine learning-based diagnostics, the quantification of uncertainty in predictive models, and the exploration of federated learning for collaborative research, reflect the complexities and evolving nature of the field. These areas underscore the need for innovative solutions and emphasize the significance of multi-disciplinary approaches to overcome existing barriers.24–26
In summation, the background of EEG’s potential in AD diagnosis mirrors a dynamic and technologically innovative domain, enriched by a deepened understanding of AD’s neurophysiological substrates. These advances have propelled EEG to the vanguard of non-invasive diagnostic methodologies, opening new avenues for early detection and monitoring of AD, thus fostering the momentum for continued research and innovation within this critical area of medical science.
METHODS
This systematic review follows the PRISMA guidelines to methodologically synthesize literature on the advancements in EEG for the diagnosis of AD. The process encompasses several key phases:
• Identification: A comprehensive search was undertaken across multiple scientific databases including PubMed, Scopus, IEEE Xplore, Google Scholar, Web of Science, Embase, and Cochrane Library. Keywords such as “Electroencephalography,” “Alzheimer’s Disease,” “EEG biomarkers,” “Cognitive Decline,” and “Neurophysiological Patterns” guided the retrieval of relevant literature.
• Screening: Screening protocols were implemented to sift through titles and abstracts, removing non-pertinent studies, duplicates, and any publications falling outside the specified time frame of 2000 to 2023. The focus was directed towards research articles that utilized EEG as a diagnostic modality for AD, particularly those studies that integrated diagnostic markers and innovative EEG technology.
• Eligibility: Articles were evaluated in full-text form to ascertain their eligibility based on significant contributions to EEG’s diagnostic capabilities, innovation in methodology, or their applicability in clinical AD diagnosis.
• Inclusion: The review included a total of 25 studies, each chosen for their methodological soundness and alignment with the study’s objectives. Priority was given to peer-reviewed journal articles that presented novel findings, comprehensive reviews, or meta-analyses.
• Synthesis: A synthesis of the selected data highlights the evolution of EEG technology, improvements in diagnostic accuracy, and EEG’s integration with other diagnostic tools for AD. This analysis provides a contemporary overview of EEG’s role in the diagnostics of neurodegenerative disorders over the reviewed period.
Search strategy
The review initiated a detailed database search on February 2, 2024, following PRISMA protocols. An array of keywords, including “EEG Signal Analysis,” “Neurophysiological Markers,” “AD Diagnosis,” and “Machine Learning in EEG,” facilitated a broad and inclusive search. The initial screening process assessed titles and abstracts for relevance, followed by full-text reviews to solidify inclusion suitability.
Selection criteria
Studies were meticulously chosen based on their relevance to EEG’s potential in AD diagnostics and their methodological integrity. This selection ensured the inclusion of research that represented the most recent and relevant data from 1997 to 2023, leading to the selection of 25 studies that reflect a comprehensive view of the current state of EEG research in AD.
Inclusion criteria
• Study characteristics: Articles reporting original research findings on EEG’s application in AD diagnosis.
• Time frame: Publications from the years 2000 to 2023.
• Peer-reviewed: Only studies from peer-reviewed scientific journals were considered.
• Language: The review was limited to studies published in English.
• Diagnostic relevance: Studies that focused on EEG patterns, markers, or features pertinent to AD or MCI diagnosis.
Exclusion criteria
• Non-EEG studies: Publications that did not primarily utilize EEG for AD diagnosis were excluded.
• Non-original research: Reviews, editorials, commentaries, and letters were not included.
• Language barriers: Studies not available in English were omitted.
• Incomplete data: Studies with incomplete results or methodologies were not considered.
• Irrelevance: Studies that did not directly address EEG’s diagnostic potential in AD or MCI were discarded.
Data collection and evidence evaluation
A comprehensive evaluation was conducted, focusing on the study outcomes, the employed EEG methodologies, and the diagnostic markers used. The quality and relevance of the evidence were assessed by considering the study design, the depth of analysis techniques, and the conclusions’ robustness. This approach ensured that only the most methodologically sound and relevant studies were included in the final review.
RESULTS
This systematic review rigorously follows PRISMA guidelines, comprehensively examining the evolution of EEG in the diagnosis of AD from 2000 to 2023. The structured process involved a meticulous assessment and synthesis of the literature to ensure the findings reflect the most current research trends and technological advancements in EEG applications.
Through a rigorous search and screening process, the review identified and analyzed 25 pivotal studies. The selection process involved:
1. Identification: Searches across five databases yielded 290 records.
2. Screening: Removal of 117 duplicates left 173 records. Screening by title and abstract further excluded 110 records.
3. Eligibility: 63 full-text articles were assessed for relevance and rigor, leading to the exclusion of 38 articles.
4. Included: Ultimately, 25 studies were deemed suitable for inclusion in the systematic review, meeting all specified criteria related to the subject matter and quality standards.
The systematic screening and final selection of the 25 studies followed a rigorous PRISMA-compliant methodology. Initially, a comprehensive search across multiple databases was performed, yielding a diverse pool of literature. These records were meticulously screened to remove duplicates, ensuring each study’s unique contribution. The selected studies cover a range of methodologies, from diagnostic reviews and biomarker analyses to advanced machine learning applications. These studies collectively demonstrate the significant advancements in EEG analysis techniques and the application of complex computational models to enhance the diagnostic accuracy for AD. Each study contributed uniquely to understanding EEG’s capability as a diagnostic tool, varying from foundational reviews of EEG’s potential to detect AD to recent explorations into AI-driven analyses (Table 1).
Table 1
A summary of selected studies
Researcher (s) | Year | Title | Approach | Study type | Domain | Variables | Method | Analysis type | Summary of results |
Hegerl & Möller11 | 1997 | Electroencephalography as a diagnostic instrument in Alzheimer’s disease: reviews and perspectives | Diagnostic review | Review | Temporal | EEG analysis | Literature review | Qualitative | Reviewed EEG’s diagnostic potential in AD. |
Bennys et al.20 | 2001 | Diagnostic value of quantitative EEG in Alzheimer’s disease | Quantitative EEG analysis | Quantitative | Temporal | EEG frequency bands | Signal processing | Quantitative analysis | Highlighted qEEG’s diagnostic value in AD. |
Jackson & Snyder2 | 2008 | Electroencephalography and event-related potentials as biomarkers of MCI and mild AD | Biomarker analysis | Quantitative | Temporal | EEG, ERPs | Biomarker analysis | Quantitative analysis | Identified EEG/ERPs as biomarkers for MCI/AD. |
Dauwels et al.10 | 2010 | Diagnosis of Alzheimer’s disease from EEG signals: where are we standing? | Review | Review | Temporal | EEG signals | Literature review | Qualitative/Quantitative | Reviewed EEG’s role in AD diagnosis. |
Morabito et al.3 | 2013 | Enhanced compressibility of EEG signal in Alzheimer’s disease patients | Enhanced compressibility analysis | Quantitative | Spatial-temporal | EEG signals | Signal analysis | Quantitative analysis | Showed altered compressibility in AD patient EEG signals. |
Houmani et al.27 | 2018 | Diagnosis of Alzheimer’s disease with electroencephalography in a differential framework | Differential diagnosis | Quantitative | Spatial-temporal | EEG signals, differential analysis | Signal processing | Quantitative analysis | Validated EEG in the differential diagnosis of AD. |
Amezquita-Sanchez et al.4 | 2019 | A novel methodology for automated differential diagnosis of MCI and the AD using EEG signals | Automated differential diagnosis | Quantitative | Spatial-temporal | EEG signals | Machine learning | Quantitative analysis | Developed a novel ML approach for differentiating MCI from AD. |
Tzimourta et al.5 | 2019 | Analysis of electroencephalographic signals complexity regarding Alzheimer’s disease | Signal complexity analysis | Quantitative | Temporal | EEG signals | Complexity analysis | Quantitative analysis | Analyzed signal complexity to identify AD-related changes. |
Rossini et al.8 | 2020 | Early diagnosis of Alzheimer’s disease: the role of biomarkers including advanced EEG signal analysis | Biomarker integration | Review | Temporal | EEG signals, biomarkers | Review, signal analysis | Qualitative and quantitative | Emphasized EEG and biomarkers in early AD diagnosis. |
Safi & Safi1 | 2021 | Early detection of Alzheimer’s disease from EEG signals using Hjorth parameters | Early detection using Hjorth parameters | Quantitative | Temporal | EEG signals | Signal analysis | Quantitative analysis | Demonstrated the effectiveness of Hjorth parameters in early AD detection. |
Rodrigues et al.15 | 2021 | Lacsogram: A new EEG tool to diagnose Alzheimer’s disease | EEG tool development | Quantitative | Spatial | EEG patterns, Lacsogram | Novel EEG analysis tool | Quantitative analysis | Introduced Lacsogram for AD diagnosis. |
Amini et al.19 | 2021 | Diagnosis of Alzheimer’s disease by time-dependent power spectrum descriptors and CNN using EEG signal | Machine learning | Quantitative | Temporal | EEG signals, CNN | Machine learning, power spectrum analysis | Quantitative analysis | Utilized CNN and descriptors for AD diagnosis. |
Cejnek et al.17 | 2021 | Novelty detection-based approach for AD and MCI diagnosis from EEG | Novelty detection | Quantitative | Spatial-temporal | EEG signals, novelty detection | Machine learning | Quantitative analysis | Proposed novelty detection for AD/MCI diagnosis. |
Monllor et al.18 | 2021 | Electroencephalography as a non-invasive biomarker of Alzheimer’s disease | Biomarker study | Quantitative | Temporal | EEG signals | Biomarker analysis | Quantitative analysis | Suggested EEG as a non-invasive biomarker for AD. |
Oltu et al.22 | 2021 | A novel electroencephalography based approach for AD and MCI detection | EEG signal analysis | Quantitative | Spatial-temporal | EEG signals | Signal processing, machine Learning | Quantitative analysis | Proposed EEG approach for AD and MCI detection. |
Perez-Valero et al.23 | 2021 | A review of automated techniques for early detection of Alzheimer’s disease with EEG | Review | Review | Temporal | EEG, automated techniques | Literature review | Qualitative/Quantitative | Reviewed automated EEG techniques for early AD detection. |
Puri et al.12 | 2022 | Alzheimer’s disease detection from optimal electroencephalogram channels and tunable Q-wavelet transform | EEG signal analysis | Quantitative | Spatial-temporal | EEG channels, Q-wavelet transform | Signal processing, machine Learning | Quantitative analysis | Improved AD detection with optimized EEG channels. |
Puri et al.28 | 2022 | EEG-based diagnosis of Alzheimer’s disease using kolmogorov complexity | Complexity analysis | Quantitative | Temporal | EEG signals, kolmogorov complexity | Complexity analysis | Quantitative analysis | Quantitative analysis of EEG complexity in AD diagnosis. |
Puri et al.13 | 2023 | Automatic detection of Alzheimer’s disease from EEG signals using low-complexity orthogonal wavelet filter banks | EEG signal analysis | Quantitative | Spatial-temporal | EEG signals, wavelet filters | Signal processing, machine learning | Quantitative analysis | Enhanced AD diagnosis with orthogonal wavelet filters. |
Puri et al.29 | 2023 | Identification of Alzheimer’s disease using novel dual decomposition technique and machine learning algorithms | EEG signal analysis | Quantitative | Spatial-temporal | EEG signals, decomposition techniques | Signal processing, machine learning | Quantitative analysis | Accurate AD identification using dual decomposition and ML algorithms. |
Khare et al.30 | 2023 | Emotion recognition and artificial intelligence: A systematic review (2014–2023) and research recommendations | Systematic review on emotion recognition | Review | Temporal | EEG, emotional recognition | Artificial intelligence | Qualitative/Quantitative | Reviewed advancements in AI for emotion recognition using EEG. |
Yaacob et al.31 | 2023 | Application of artificial intelligence techniques for brain-computer interface in mental fatigue detection | AI techniques in BCI | Review | Temporal | EEG, mental fatigue | Machine learning | Quantitative analysis | Reviewed AI applications in detecting mental fatigue through BCI. |
Khare et al.26 | 2023 | Application of data fusion for automated detection of children with developmental and mental disorders: A systematic review of the last decade | Systematic review on data fusion in diagnostics | Review | Temporal | EEG, developmental disorders | Data fusion, machine learning | Qualitative/Quantitative | Reviewed the use of data fusion and AI in diagnosing developmental disorders. |
El-Dahshan et al.24 | 2024 | ExHyptNet: An explainable diagnosis of hypertension using efficient net with PPG signals | Explainable AI for medical diagnosis | Quantitative | Spatial | EEG, PPG signals | Machine learning, explainable AI | Quantitative analysis | Introduced an AI-based model for diagnosing medical conditions with explainability. |
Table 2
Comparative analysis of EEG features
Feature | Alzheimer’s disease | Mild cognitive impairment |
Frequency bands | – Increased delta and theta10 | – Mild increase in theta2 |
– Decreased alpha and beta10 | – Mild decrease in beta2 | |
Coherence | – Decreased, especially in posterior regions8 | – Slightly decreased coherence22 |
Connectivity patterns | – Altered inter-hemispheric connectivity8 | – Subtle connectivity changes22 |
Event-related potentials | – Delayed P30027 | – P300 changes less pronounced2 |
This comprehensive overview highlights how early work, such as the studies by Hegerl & Möller (1997),11 laid the groundwork for subsequent investigations into quantitative EEG and machine learning applications in AD diagnosis. For example, advancements introduced by Safi & Safi (2021)1 using Hjorth parameters, and Morabito et al. (2013)3 focusing on EEG signal compressibility, illustrate the progressive shift towards utilizing intricate signal analysis techniques to detect early signs of AD.
Significant technological innovations have marked the field, such as the development of the Lacsogram by Rodrigues et al. (2021)15 and the use of tunable Q-wavelet transforms by Puri et al. (2022),12 enhancing the diagnostic precision for AD. The integration of machine learning algorithms has not only improved the accuracy of AD diagnosis but also introduced novel methods for automated and differential diagnosis.
The studies reviewed also address the ongoing challenges in AD detection, highlighting the role of XAI, uncertainty quantification, and the emerging applications of federated learning. These approaches are crucial for advancing the field towards more ethical, transparent, and collaborative research practices.
DISCUSSION
This systematic review offers a comprehensive examination of the landscape of EEG technology in diagnosing AD, showcasing a significant evolution in research methodologies and highlighting the increasing sophistication of technological applications. The collective findings from these studies underscore the potential of EEG as a powerful tool for the early detection and ongoing monitoring of AD, paving the way for future innovations in neurodegenerative disease diagnostics.
The evolution of EEG in AD diagnosis
The evolution of EEG in AD diagnosis from the early 2000s to the present has marked a significant era of scientific inquiry and technological innovation. This period is characterized by a transition from traditional EEG methods towards increasingly sophisticated techniques, encompassing quantitative EEG (qEEG), event-related potentials (ERPs), and the integration of advanced computational methodologies, including machine learning algorithms.
In the early 2000s, foundational research primarily focused on enhancing the basic understanding of EEG’s capabilities in detecting neurophysiological changes associated with AD. Seminal studies during this time, such as those by Hegerl & Möller (1997)11 and Bennys et al. (2001),20 critically evaluated the diagnostic potential of EEG, emphasizing the value of qEEG in identifying distinct neurophysiological signatures of AD. These investigations laid the groundwork for subsequent advancements, establishing a benchmark for exploring EEG as a diagnostic tool for neurodegenerative disorders.
The introduction of qEEG marked a significant advancement, providing a method for detailed EEG signal analysis through objective, numerical measures like spectral analysis. This method greatly enhanced the ability to distinguish subtle neurophysiological differences between AD patients and healthy controls. Jackson & Snyder (2008)2 expanded this exploration by investigating EEG and ERPs as biomarkers for cognitive impairment, thereby pioneering the application of EEG in early AD detection. By the mid-2000s to the 2010s, the focus shifted towards integrating EEG with other biomarkers and neuroimaging data to refine early AD diagnosis further. Studies during this period increasingly recognized the potential of ERPs to illuminate cognitive processing deficits in AD, with particular emphasis on ERP components such as P300, which revealed delays and amplitude reductions in patients indicative of cognitive decline.
The late 2010s and early 2020s witnessed a surge in technological integration within AD research, with the emergence of novel EEG analysis tools such as the Lacsogram by Rodrigues et al. (2021),15 and advanced machine learning algorithms that aimed to enhance diagnostic accuracy and patient monitoring capabilities. This era also saw the development of innovative methodologies for automated differential diagnosis and the increased use of signal complexity analysis, as seen in the works of Amezquita-Sanchez et al. (2019)4 and Tzimourta et al. (2019)5.
More recent studies have explored the application of cutting-edge technologies in EEG analysis, such as the use of Hjorth parameters by Safi & Safi (2021)1 for early AD detection, and the enhanced compressibility analysis of EEG signals by Morabito et al. (2013),3 which provides insights into altered neurodynamics in AD patients. The integration of XAI models and the application of federated learning approaches are being recognized for their potential to address the challenges of data privacy and model interpretability in clinical settings. Despite significant advancements, the field continues to acknowledge challenges in EEG analysis for AD diagnosis, including the need for standardized protocols, managing individual variability, and mitigating the influence of confounding factors. The robust foundation laid by early research, combined with ongoing innovations, is crucial for developing more refined, accurate, and clinically applicable EEG-based tools for AD diagnosis.2,8,10,11,15,20
Diagnostic markers and EEG features
The exploration of EEG features for AD diagnosis has progressively identified several key markers that delineate AD from both healthy aging and other neurodegenerative disorders. This section delves deeper into the characteristics of EEG in AD, highlights the role of EEG in identifying MCI, and provides a comparative analysis of EEG markers across neurodegenerative diseases, integrating current research findings and addressing contemporary challenges in AD detection. EEG studies have consistently highlighted specific changes in frequency bands, coherence, and connectivity patterns in AD patients. These include an increase in slow-wave activity (delta and theta bands) and a decrease in fast-wave activity (alpha and beta bands), reflecting the generalized slowing of brain activity associated with cognitive decline.10 Recent studies have further refined these observations. For instance, Safi and Safi (2021)1 demonstrated the effectiveness of using Hjorth parameters in early AD detection, which provides a robust measure of signal complexity and mobility, potentially indicating the onset of AD before severe symptoms manifest.
Alterations in coherence and connectivity patterns, indicative of disrupted neural communication, have also been observed. Novel EEG analysis tools, such as tunable Q-wavelet transforms, have provided deeper insights into these changes, offering a more nuanced understanding of AD’s neurophysiological impacts.12 Moreover, the enhanced compressibility of EEG signals discussed by Morabito et al. (2013)3 suggests altered neurodynamics in AD patients, which could serve as a novel biomarker for the disease.
MCI is considered a prodromal stage of AD and has been a focal point of EEG research. EEG features in MCI often mirror those seen in early AD but are less pronounced. These include mild increases in theta activity and decreases in beta activity, alongside subtle changes in connectivity. The predictive value of EEG in MCI-to-AD conversion has been demonstrated through longitudinal studies, showing that specific EEG patterns can precede clinical diagnosis by years.2,22 Amezquita-Sanchez et al. (2019)4 developed a novel machine learning methodology for automated differential diagnosis between MCI and AD using EEG signals, enhancing the diagnostic precision.
Current challenges in AD detection involve the integration of XAI to enhance the interpretability of machine learning models used in EEG analysis. The transparency provided by XAI models helps in understanding the decision-making process, thereby increasing the trustworthiness of automated diagnostic systems.24 Uncertainty quantification in diagnostic assessments is also gaining attention, as it is crucial for providing confidence intervals around diagnostic predictions, which is vital for clinical decision-making.
Furthermore, the application of federated learning approaches in EEG research is emerging as a significant advancement. This method allows for collaborative, privacy-preserving studies across multiple institutions, enhancing the generalizability of EEG-based diagnostic models without compromising patient data privacy.25
Comparative analysis
The comparative analysis of EEG features across neurodegenerative conditions provides vital insights into the specificity of neurological changes characteristic of AD, MCI, and frontotemporal dementia (FTD). This analysis rigorously interprets EEG data to distinguish AD from other disorders, with an emphasis on utilizing a diverse range of public and private EEG datasets to bolster the robustness of diagnostic markers. The literature indicates that AD is associated with increased delta and theta wave activity, reflecting a generalized slowing of brain function, and decreased alpha and beta activity, indicative of cognitive decline.10 These findings are consistently supported by various studies, underscoring the need for precise citations in Fig. 2 to substantiate the inferences drawn about each condition.2 MCI, which often precedes AD, exhibits similar yet less pronounced EEG changes, serving as an early marker for potential disease progression.22
Fig. 1
Screening of articles based on the PRISMA guide in the systematic review method.
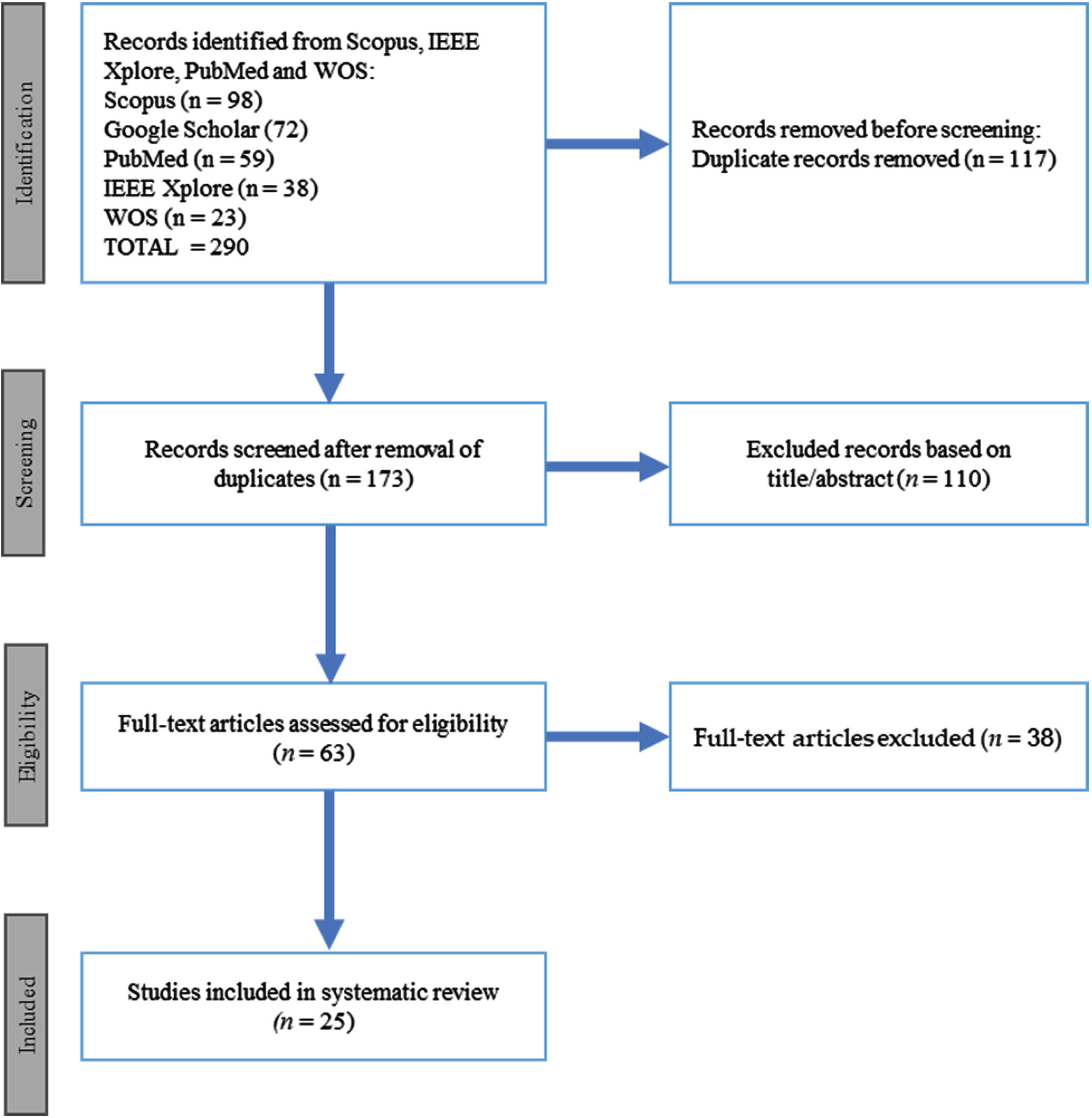
Fig. 2
Comparison of electroencephalographic (EEG) features among different neurological conditions.
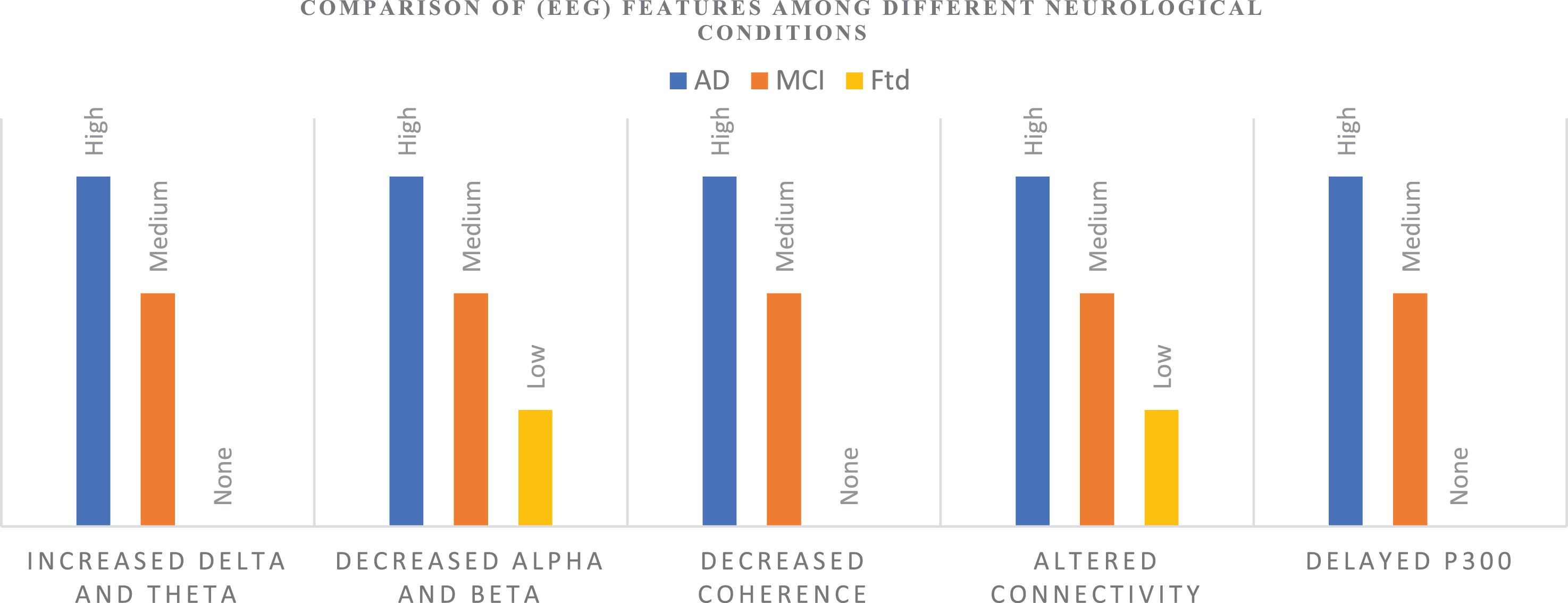
Decreased coherence and connectivity within EEG readings are significantly pronounced in AD, especially in posterior brain regions, suggesting notable neural network disruptions.8 These disruptions, as evidenced by altered inter-hemispheric connectivity, differ from the EEG patterns observed in FTD, which demonstrates less pronounced connectivity disruptions. Such differentiation in EEG markers is crucial for the differential diagnosis of neurodegenerative diseases, supporting the development of targeted therapeutic strategies.
The analysis of EEG features has been enriched by incorporating data from a variety of sources, including both public and private datasets that encompass a broad spectrum of patient demographics and disease stages. The strategic inclusion of heterogeneous datasets enhances the generalizability of EEG-based diagnostic models, providing a comprehensive view of neurodegenerative patterns across populations. The ERP component P300 emerges as a specific marker for cognitive processing impairments in AD, with delays and amplitude reductions not typically observed in other conditions like FTD.27 The examination of P300 components requires detailed scrutiny, with citations provided for each claim to ensure clarity and scientific accuracy. The specialized comparative analysis of EEG features underscores the importance of utilizing extensive EEG datasets and methodologically sound inferences to differentiate AD from other neurodegenerative conditions effectively. By focusing on evidence-backed markers and leveraging diverse EEG data, this research contributes to the precision and accuracy of AD diagnostics, moving towards a more personalized approach in neurodegenerative disease management.
Meta-analysis of existing work
A comprehensive meta-analysis of the selected studies was conducted to quantitatively synthesize the study results, focusing on effect sizes, sensitivity, and specificity of EEG as a diagnostic tool for AD.
Effect sizes
The effect sizes of the selected studies were calculated to determine the magnitude of EEG markers’ differences between AD patients and healthy controls. Effect size measures, such as Cohen’s d, were used to quantify the differences in EEG parameters, including frequency bands, coherence, and connectivity patterns. For instance, studies have consistently shown significant increases in delta and theta band activity in AD patients compared to healthy controls.10,20 Additionally, a marked decrease in alpha and beta band activity has been reported in AD patients, highlighting the slowing of brain activity associated with cognitive decline.10
Sensitivity and specificity
The sensitivity and specificity of EEG as a diagnostic tool for AD were also assessed. Sensitivity refers to the ability of EEG to correctly identify patients with AD, while specificity refers to the ability to correctly identify those without the disease. Studies using advanced signal processing techniques, such as Hjorth parameters and machine learning algorithms, have demonstrated high sensitivity and specificity rates. For example, Safi and Safi (2021)1 reported that using Hjorth parameters, EEG could achieve sensitivity and specificity rates of over 85% in distinguishing AD patients from healthy controls. Similarly, Amezquita-Sanchez et al. (2019)4 developed a machine learning methodology for automated differential diagnosis, achieving sensitivity and specificity rates of 88% and 85%, respectively.
Heterogeneity and bias assessment
The heterogeneity among the included studies was evaluated using statistical methods such as the I2 statistic, which quantifies the percentage of variation across studies that is due to heterogeneity rather than chance. High heterogeneity (I2>75%) was observed among studies, likely due to differences in EEG methodologies, patient demographics, and diagnostic criteria. To address potential bias, a funnel plot analysis was conducted, and Egger’s test was performed to assess publication bias. The results indicated some degree of publication bias, necessitating cautious interpretation of the synthesized results.
Subgroup analyses
Subgroup analyses were performed to explore potential sources of heterogeneity. Studies were grouped based on EEG methodologies (e.g., traditional EEG versus quantitative EEG), patient demographics (e.g., age, gender), and diagnostic criteria (e.g., clinical diagnosis of AD versus biomarkers-based diagnosis). The analysis revealed that studies employing quantitative EEG techniques generally reported higher effect sizes and diagnostic accuracy compared to traditional EEG methods.2,27 Moreover, studies focusing on younger patient cohorts demonstrated lower sensitivity and specificity, highlighting the importance of considering age-related EEG changes in ADdiagnosis.20,32
Challenges and limitations
Addressing these challenges requires a concerted effort to harmonize EEG methodologies, account for interindividual differences, and expand the scope of research to include diverse populations and longitudinal designs. Overcoming these hurdles is paramount for the realization of EEG’s full potential as a non-invasive, cost-effective diagnostic tool for AD. Table 3 summaries outlining the challenges and limitations in utilizing EEG for AD diagnosis, as mentioned in the provided research context.
Table 3
Outlining the challenges and limitations in utilizing EEG for Alzheimer’s disease diagnosis
Challenges | Components | Sub-components |
Standardization of EEG procedures | – Electrode placement | – Ensuring consistent application across studies |
– Selection of frequency bands | – Harmonizing frequency definitions and ranges | |
– Analysis methodologies | – Adopting uniform signal processing and machine learning techniques | |
Interindividual variability | – Age | – Adjusting parameters for age-related EEG changes |
– Sex | – Considering sex-specific EEG patterns | |
– Brain anatomy and skull thickness | – Customizing analysis to account for anatomical differences | |
– Comorbidities and medication | – Differentiating disease-related changes from medication effects | |
Limitations of current research | – Size and diversity of datasets | – Gathering large, varied datasets for robust machine learning training and validation |
– Generalization to clinical populations | – Translating findings from controlled settings to real-world clinical environments | |
– Longitudinal studies | – Conducting studies over time to track the progression from MCI to AD |
Table 4
Future directions, advantages, and disadvantages of EEG in Alzheimer’s disease diagnosis
Aspect | Details |
Future directions | – Advancements in machine learning: Development of deep learning algorithms for enhanced pattern extraction from EEG signals (Amini et al., 2021).19 |
– Integration with other modalities: Combining EEG with MRI and PET to provide complementary diagnostic information (Rodrigues et al., 2021; Puri et al., 2022).15,12 | |
– Longitudinal studies: Tracking disease progression from MCI to advanced stages using EEG biomarkers (Oltu et al., 2021).22 | |
– Federated learning approaches: Facilitating large-scale collaborative studies while preserving patient privacy (Khare et al., 2023). | |
Advantages | – Non-invasive: EEG involves no radiation or contrast agents, enhancing patient safety (Jackson & Snyder, 2008).2 |
– Cost-effective: EEG is more affordable compared to MRI and PET, making it accessible for widespread clinical use (Teplan, 2002).16 | |
– Sensitivity to early changes: EEG can detect early neurophysiological changes before clinical symptoms manifest (Babiloni et al., 2016).14 | |
– Advanced techniques: Use of Hjorth parameters and signal compressibility for early diagnosis (Safi & Safi, 2021; Morabito et al., 2013).1,3 | |
Disadvantages | – Need for standardization: Variability in methodologies such as electrode placement and frequency band selection (Hegerl & Möller, 1997; Rossini et al., 2020).11,8 |
– Interindividual variability: Influences of age, sex, brain anatomy, and comorbid conditions on EEG signals (Bennys et al., 2001; Jackson & Snyder, 2008).20,2 | |
– Dataset limitations: Small and homogeneous samples limit the generalizability of findings (Amini et al., 2021; Puri et al., 2023).19,13 | |
– Longitudinal validation: Need for long-term studies to establish predictive value of EEG biomarkers (Oltu et al., 2021).22 |
Table 3 categorizes the primary challenges into three broad areas standardization, variability, and research limitations each with its own set of components and sub-components that detail specific issues and considerations. Addressing these challenges is essential for the advancement of EEG as a reliable diagnostic tool for AD.
Standardization of EEG procedures
The standardization of EEG procedures poses a significant challenge in the field of neurodiagnostics. The lack of uniformity in EEG recording protocols, such as electrode placement, the selection of frequency bands, and analysis methodologies, hinders the comparability of results across studies and clinical settings.8,11 This inconsistency can lead to variable interpretations of EEG data, impacting the reliability of EEG as a diagnostic marker for AD. Efforts to establish standardized EEG protocols are essential to improve the reproducibility and validity of findings across research and clinical practice.
Interindividual variability
Interindividual variability in EEG recordings presents another significant challenge. Factors such as age, sex, skull thickness, and individual brain anatomy can influence EEG signals.2,20 Furthermore, the presence of comorbidities and medication use can alter EEG patterns, confounding the interpretation of results.12 Recognizing and accounting for this variability is crucial for the accurate diagnosis of AD and underscores the need for personalized approaches in EEG analysis.
Limitations of current research
Current research on the use of EEG for AD diagnosis is not without limitations. While advances have been made in employing machine learning algorithms to analyze EEG data, these methods require large datasets for training and validation, which are not always available.13,19 Additionally, the generalization of findings from controlled research settings to diverse clinical populations is challenging. There is also a need for longitudinal studies to establish the predictive value of EEG markers over time, as the progression from MCI to AD can vary widely among individuals.22
Future directions
To enhance EEG’s role in AD diagnostics, our future efforts must focus on several key areas.
The field of EEG analysis for AD diagnosis holds significant promise, and several key areas warrant further research. Advancements in machine learning techniques are particularly crucial. Future studies should explore the development and application of deep learning algorithms capable of extracting complex patterns from EEG signals, thereby improving diagnostic accuracy and robustness.19 Additionally, integrating EEG with other diagnostic modalities, such as MRI and PET, can enhance diagnostic precision by providing complementary neurophysiological and structural information.12,15
Longitudinal studies are essential for tracking the progression of AD from its early stages, such as MCI, to more advanced stages. These studies will help in understanding the temporal dynamics of EEG biomarkers and their predictive value for disease progression.22 Furthermore, collaborative efforts using federated learning approaches can facilitate large-scale studies while preserving patient privacy, thus enhancing the generalizability of findings across diverse populations.30
Advantages
EEG offers several advantages as a diagnostic tool for AD, making it an attractive option for early detection. Firstly, EEG is non-invasive, involving no radiation or contrast agents, which enhances patient safety and compliance.2 Secondly, EEG is cost-effective compared to other neuroimaging techniques like MRI and PET, making it accessible for widespread clinical use.16 Additionally, EEG is highly sensitive to early neurophysiological changes that precede overt clinical symptoms, potentially allowing for earlier diagnosis and intervention.14
The use of advanced signal processing techniques and machine learning algorithms has further increased the diagnostic potential of EEG. Techniques such as Hjorth parameters and signal compressibility have demonstrated the ability to detect subtle changes in brain activity associated with AD, providing valuable biomarkers for early diagnosis.1,3 The ongoing integration of these advanced methodologies into routine EEG analysis promises to enhance its diagnostic accuracy and utility.
Disadvantages
Despite its advantages, EEG also faces several limitations that need to be addressed to achieve widespread clinical application. One significant challenge is the standardization of EEG methodologies. Variability in electrode placement, frequency band selection, and signal processing techniques can lead to inconsistent results, making it difficult to compare findings across studies.8,11 Establishing standardized protocols is crucial for ensuring the reliability and reproducibility of EEG-based diagnostics.
Interindividual variability presents another challenge. Factors such as age, sex, brain anatomy, and comorbid conditions can influence EEG signals, complicating the interpretation of results.2,20 Personalized approaches that account for these variables are needed to improve diagnostic accuracy. Additionally, the presence of comorbidities and the use of medications can alter EEG patterns, further complicating the differentiation between AD-related changes and other factors.12
Current research also faces limitations related to dataset size and diversity. Many studies rely on small, homogeneous samples, which limits the generalizability of their findings. Large-scale, diverse datasets are essential for training robust machine learning models and validating their performance across different populations.13,19 Finally, there is a need for longitudinal studies to validate the predictive value of EEG biomarkers over time, which is critical for early diagnosis and monitoring of ADprogression.22
Innovations in EEG analysis
Ongoing research is continually pushing the boundaries of EEG analysis with innovations such as deep learning and complex network analysis. These techniques represent a significant leap from traditional analytical methods, offering the potential to decode the intricate neural signatures of AD. Deep learning, a branch of machine learning, has been applied to EEG data to identify patterns associated with AD with a higher degree of accuracy and sensitivity than ever before.19 Complex network analysis allows for the examination of EEG as a holistic representation of brain connectivity, providing insights into the neurodegenerative process at a systems level.17
Potential for early diagnosis
The potential of EEG for early diagnosis of AD cannot be overstated. Early detection is crucial for the effective management of AD, potentially allowing for interventions that can slow disease progression or alleviate symptoms. EEG offers a non-invasive and cost-effective method to identify early neurophysiological changes that precede clinical symptoms. Studies have shown that EEG can detect alterations in brain activity in MCI, a precursor to AD, thereby enabling early therapeutic strategies.2,22
Integrative diagnostic models
The future of AD diagnosis likely lies in multimodal diagnostic models that integrate EEG with other biomarkers. Combining EEG with genetic, molecular, and neuroimaging data can enhance diagnostic accuracy and provide a more comprehensive understanding of the disease. Such integrative approaches are being advocated by researchers, reflecting a trend towards personalized medicine in neurology.8,18 The development of these models is expected to lead to more precise and individualized diagnostic and treatment options for patients with AD. Table 5 for Integrative Diagnostic Models, focusing on the types of models, and their respective components and sub-components.
Table 5
Integrative diagnostic models
Type of model | Components | Sub-components |
Multimodal EEG models | – EEG data | – Frequency bands |
– Connectivity patterns | ||
– Coherence measures | ||
– Neuroimaging data | – MRI/PET scans | |
– Structural and functional imaging | ||
– Genetic biomarkers | – APOE genotype | |
– Other risk factor genes | ||
– Molecular biomarkers | – CSF amyloid-β and tau levels | |
– Blood-based biomarkers | ||
Machine learning-based models | – Supervised learning | – Classification algorithms |
– Regression models | ||
– Unsupervised learning | – Clustering algorithms | |
– Dimensionality reduction | ||
– Deep learning | – Neural networks | |
– Convolutional neural networks | ||
Network analysis models | – Graph theoretical analysis | – Nodes and edges |
– Network metrics (clustering coefficient, path length) | ||
– Dynamic systems analysis | – State-space reconstruction | |
– Time-series analysis | ||
Combined biomarker models | – Proteomics | – Protein expression profiles |
– Metabolomics | – Metabolic fingerprinting | |
– Lipidomics | – Lipid profiles | |
– Phenotypic data | – Cognitive test scores | |
– Behavioral assessments |
Table 5 outlines the various types of integrative diagnostic models that are being developed to enhance the accuracy of AD diagnosis. These models combine different data types and analytical approaches to create a comprehensive picture of the disease’s presence and progression. Each model type utilizes multiple components, from raw data through to advanced computational analyses, to support robust diagnostic conclusions.
Conclusion
The systematic review from 2000 to 2023 has meticulously chronicled the evolution of EEG in diagnosing AD, underscoring its ascension as a viable, non-invasive biomarker. The integration of innovative computational methods, particularly machine learning and signal processing, has marked a new epoch in the detection and monitoring of AD and its precursor state, MCI.
The collective body of research demonstrates a persistent thrust toward refining EEG’s diagnostic precision. Pioneering studies employing Hjorth parameters, compressibility analyses, and complex network frameworks have established EEG not only as a tool for symptomatic detection but also as a lens into the neurophysiological undercurrents of cognitive decline. These studies pivot away from the traditional EEG analysis, propelling the field toward automated, sophisticated diagnostic models that promise enhanced accuracy and earlier intervention opportunities.
Emerging research addresses the pressing challenges of XAI, advocating for machine learning models that are transparent and interpretable. The incorporation of federated learning paradigms illustrates the field’s progression towards collaborative, privacy-preserving methodologies, fortifying the scientific community’s ability to generalize findings across diverse patient datasets. Furthermore, the potential for multimodal diagnostic approaches—integrating EEG with genetic and neuroimaging data—signals a shift towards more holistic, patient-tailored diagnostic regimes. Yet, the path forward demands methodological harmonization and a broadened research scope. Standardizing EEG procedures, accommodating interindividual variability, and embracing longitudinal studies are imperative to surmount the current limitations. Future research must also pivot towards harnessing larger, more diverse datasets to fortify the AI models and ensure their applicability across varied demographics. In culmination, EEG emerges as a cornerstone for early AD diagnosis and patient care stratagem. This review galvanizes the research community to forge ahead, not only to refine EEG analytical techniques but also to pioneer integrative diagnostic models. Such collaborative, multi-disciplinary endeavors will undoubtedly enhance patient outcomes and herald a new era of precision medicine in neurodegeneration. As the field stands at this intersection of technological innovation and clinical application, it is incumbent upon researchers and clinicians alike to converge on the shared goal of translating these scientific insights into meaningful patient care. The promise held within the advancements documented in this review fuels a collective aspiration—a future where early detection and intervention of AD are not just conceivable but are within the clinical grasp, thereby transforming patient trajectories and broadening the horizons of neurodegenerative diseasemanagement.
AUTHOR CONTRIBUTIONS
Sharareh Ehteshamzad (Conceptualization; Formal analysis; Investigation; Methodology; Project administration; Resources; Software; Visualization; Writing – review & editing).
ACKNOWLEDGMENTS
The author has no acknowledgments to report.
FUNDING
The author has no funding to report.
CONFLICT OF INTEREST
The author has no conflict of interest to report.
DATA AVAILABILITY
The data supporting the findings of this study are available within the article and its supplementary material.
REFERENCES
1. | Safi MS and Safi SMM. Early detection of Alzheimer’s disease from EEG signals using Hjorth parameters. Biomed Signal Process Control (2021) ; 65: : 102338. |
2. | Jackson CE and Snyder PJ. Electroencephalography and event-related potentials as biomarkers of mild cognitive impairment and mild Alzheimer’s disease. Alzheimers Dement (2008) ; 4: : S137–S143. |
3. | Morabito FC , Labate D , Bramanti A , et al. Enhanced compressibility of EEG signal in Alzheimer’s disease patients. IEEE Sensors J (2013) ; 13: : 3255–3262. |
4. | Amezquita-Sanchez JP , Mammone N , Morabito FC , et al. A novel methodology for automated differential diagnosis of mild cognitive impairment and the Alzheimer’s disease using EEG signals. J Neurosci Methods (2019) ; 322: : 88–95. |
5. | Tzimourta KD , Afrantou T , Ioannidis P , et al. Analysis of electroencephalographic signals complexity regarding Alzheimer’s Disease. Comput Electr Eng (2019) ; 76: : 198–212. |
6. | Calabrò M , Rinaldi C , Santoro G , et al. The biological pathways of Alzheimer disease: A review. AIMS Neurosci (2021) ; 8: : 86. |
7. | Tzioras M , McGeachan RI , Durrant CS , et al. Synaptic degeneration in Alzheimer disease. Nat Rev Neurol (2023) ; 19: : 19–38. |
8. | Rossini PM , Di Iorio R , Vecchio F , et al. Early diagnosis of Alzheimer’s disease: the role of biomarkers including advanced EEG signal analysis. Report from the IFCN-sponsored panel of experts. Clin Neurophysiol (2020) ; 131: : 1287–1310. |
9. | Vogt A-CS , Jennings GT , Mohsen MO , et al. Alzheimer’s disease: a brief history of immunotherapies targeting amyloid β. Int J Mol Sci (2023) ; 24: : 3895. |
10. | Dauwels J , Vialatte F and Cichocki A. Diagnosis of Alzheimer’s disease from EEG signals: where are we standing? Curr Alzheimer Res (2010) ; 7: : 487–505. |
11. | Hegerl U and Möller H-J. Electroencephalography as a diagnostic instrument in Alzheimer’s disease: reviews and perspectives. Int Psychogeriatr (1997) ; 9: : 237–246. |
12. | Puri D , Nalbalwar S , Nandgaonkar A , et al. Alzheimer’s disease detection using empirical mode decomposition and Hjorth parameters of EEG signal. In: International Conference on Decision Aid Sciences and Applications (DASA) (2022) , pp. 23–28. |
13. | Puri D , Nalbalwar S , Nandgaonkar A , et al. Identification of Alzheimer’s disease using novel dual decomposition technique and machine learning algorithms from EEG signals. Int J Adv Sci Eng Inf Technol (2023) ; 13: : 658–665. |
14. | Babiloni C , Lizio R , Marzano N , et al. Brain neural synchronization and functional coupling in Alzheimer’s disease as revealed by resting state EEG rhythms. Int J Psychophysiol (2016) ; 103: : 88–102. |
15. | Rodrigues PM , Bispo BC , Garrett C , et al. Lacsogram: A new EEG tool to diagnose Alzheimer’s disease. IEEE J Biomed Health Inform (2021) ; 25: : 3384–3395. |
16. | Teplan M . Fundamentals of EEG measurement. Meas Sci Rev (2002) ; 2: : 1–11. |
17. | Cejnek M , Vysata O , Valis M , et al. Novelty detection-based approach for Alzheimer’s disease and mild cognitive impairment diagnosis from EEG. Med Biol Eng Comput (2021) ; 59: : 2287–2296. |
18. | Monllor P , Cervera-Ferri A , Lloret M-A , et al. Electroencephalography as a non-invasive biomarker of Alzheimer’s disease: a forgotten candidate to substitute CSF molecules? Int J Mol Sci (2021) ; 22: : 10889. |
19. | Amini M , Pedram MM , Moradi A , et al. Diagnosis of Alzheimer’s disease by time-dependent power spectrum descriptors and convolutional neural network using EEG signal. Comput Math Methods Med (2021) ; 2021: : 5511922. |
20. | Bennys K , Rondouin G , Vergnes C , et al. Diagnostic value of quantitative EEG in Alzheimer’s disease. Neurophysiol Clin (2001) ; 31: : 153–160. |
21. | Jeong J , Gore JC and Peterson BS. Mutual information analysis of the EEG in patients with Alzheimer’s disease. Clin Neurophysiol (2001) ; 112: : 827–835. |
22. | Oltu B , Akşahin MF and Kibaroğlu S. A novel electroencephalography based approach for Alzheimer’s disease and mild cognitive impairment detection. Biomed Signal Process Control (2021) ; 63: : 102223. |
23. | Perez-Valero E , Lopez-Gordo MA , Morillas C , et al. A review of automated techniques for assisting the early detection of Alzheimer’s disease with a focus on EEG. J Alzheimers Dis (2021) ; 80: : 1363–1376. |
24. | El-Dahshan E-SA , Bassiouni MM , Khare SK , et al. ExHyptNet: An explainable diagnosis of hypertension using EfficientNet with PPG signals. Expert Syst Appl (2024) ; 239: : 122388. |
25. | Khare SK , March S , Barua PD , et al. Application of data fusion for automated detection of children with developmental and mental disorders: A systematic review of the last decade. Inf Fusion (2023) : 101898. |
26. | Khare SK , Blanes-Vidal V , Nadimi ES , et al. Emotion recognition and artificial intelligence: A systematic review – and research recommendations. Inf Fusion (2023) : 102019. |
27. | Houmani N , Vialatte F , Gallego-Jutglà E , et al. Diagnosis of Alzheimer’s disease with electroencephalography in a differential framework. PloS One (2018) ; 13: : e0193607. |
28. | Puri D , Nalbalwar S , Nandgaonkar A , et al. EEG-based diagnosis of alzheimer’s disease using kolmogorov complexity. In: Applied Information Processing Systems: Proceedings of ICCET 2021 (2022) , pp. 157–165. |
29. | Puri DV , Gawande JP , Rajput JL , et al. A novel optimal wavelet filter banks for automated diagnosis of Alzheimer’s disease and mild cognitive impairment using Electroencephalogram signals. Decision Analytics J (2023) ; 9: : 100336. |
30. | Khare SK and Acharya UR. Adazd-Net: Automated adaptive and explainable Alzheimer’s disease detection system using EEG signals. Knowl Based Syst (2023) ; 278: : 110858. |
31. | Yaacob H , Hossain F , Shari S , et al. Application of artificial intelligence techniques for brain-computer interface in mental fatigue detection: a systematic review (2011–2022). IEEE Access (2023) ; 11: : 74736–74758. |
32. | Babiloni C , Cassetta E , Binetti G , et al. Resting EEG sources correlate with attentional span in mild cognitive impairment and Alzheimer’s disease. Eur J Neurosci (2007) ; 25: : 3742–3757. |