Discriminant Potential of the Alzheimer’s Disease Assessment Scale-Cognitive Subscale (ADAS-Cog) in Greek Older Adults with Subjective Cognitive Decline and Mild Cognitive Impairment
Abstract
Background:
Alzheimer’s Disease Assessment Scale Cognitive Subscale (ADAS-Cog) is a widely used screening tool for detecting older adults with Alzheimer’s disease among their cognitively healthy peers. A previous study in Greek population showed that ADAS-Cog-Greek (G) is a valid tool and can identify people with Alzheimer’s disease from older adult control group; however, there is no current data about whether ADAS-Cog can differentiate older adults with mild cognitive impairment (MCI) from those who have subjective cognitive decline (SCD).
Objective:
The current study aimed to examine the discriminant potential of ADAS-Cog-G in Greek older adults who meet the criteria for SCD or MCI.
Methods:
Four hundred eighty-two community-dwelling older adults, visitors of the Greek Alzheimer Association and Related Disorders, were enrolled in the current study. One hundred seventy-six of them met the criteria for SCD and three hundred six had MCI.
Results:
Path analysis applied to the data showed that age, as well as educational level affected ADAS-Cog-G performance. Results showed that the cut-off scores, which better discriminate people with SCD from MCI as well as their sensitivity and specificity values, were extracted in participants with high educational level (13 educational years<) and mainly under the age of 75 years.
Conclusions:
The current study provided evidence concerning the discriminant potential of ADAS-Cog-G to differentiate older adults with SCD from those with MCI in the Greek population, and therefore contributes to the relevant literature on the field.
INTRODUCTION
The need for accurate assessment of general cognitive ability is necessary in older adults’ population, to identify those at greater risk of developing dementia, as well as evaluate the clinical trials implemented in people living with dementia. The Alzheimer’s Disease Assessment Scale (ADAS) calculated by adding the cognitive (ADAS-Cog) and non-cognitive (ADAS-Nocog) subscales is a widely used screening tool to identify older adults with Alzheimer’s disease (AD) and has been adapted in many countries such as Italy, Turkey, Iceland, Spain, Korea, Brazil, Slovakia, Portugal, sub-Saharan Africa, Taiwan, Slovenia, and China [1–11] respectively.
Since, ADAS assesses a plethora of cognitive functioning impairments, which are the core symptoms of dementia and mainly AD, as well as the participants’ functional status [2], it is considered a complete assessment in comparison to other screening batteries [3] and therefore, it is often included in AD pharmacological clinical trials. Specifically, according to Cano et al. [12], ADAS is the widest screening tool used in clinical trials about AD, whereas Paddick et al. [8] support its use in pharmacological and non-pharmacological intervention protocols, especially in high-income countries. In line with this, the Food and Drug Administration (FDA) assumes ADAS-Cog is a useful screening tool to be administered in older adults who participate in clinical trials [13, 14]. Nevertheless, besides its use in identifying patients with AD, it has been also used in studies that involved non-AD populations such as those diagnosed with mild cognitive impairment (MCI) [15], vascular dementia [16], and Parkinson’s disease [17].
As regards the effects of demographic variables on ADAS-Cog, previous studies [6, 18] have found that gender, age, and educational level do not impact its score, whereas Liu and colleagues [19] found that the ADAS-Cog total score was uninfluenced by the demographic variables of gender, age and educational level in a population of cognitively intact older adults, those with questionable dementia as well as those with AD. The only case in which the participants’ performance was affected was in those with low educational level (0–6 years of schooling). Taking into account that ADAS-Cog includes some literacy-dependent tasks, such as the recall and drawing tasks, it is quite significant to validate this screening tool, despite the fact that it is culturally free [20, 21]. On the other side, Rockwood et al. [22] provide evidence that ADAS-Cog declines with age in patients with mild and moderate AD, consequently, it seems that data regarding the effects of demographics on ADAS-Cog are controversial.
Regarding the Greek version, namely the ADAS-Cog-Greek (ADAS-Cog-G) [23], ADAS-Cog-G is very sensitive even in detecting impairment during the later stages of AD, and therefore its use is highly recommended for clinicians and researchers who implement pharmaceutical protocols. Given that ADAS-Cog can be administered in all different stages of AD, from the mild to the more severe ones [12], it can be considered a valuable screening tool to test the efficacy of the therapeutic interventions, both pharmacological and non-pharmacological ones.
Validation studies for the ADAS and ADAS-Cog, particularly in the MCI population, are limited because it has been primarily used to detect patients with mild dementia for clinical or research purposes. Therefore, it is of most importance to examine whether the test can discriminate healthy older adults from those with MCI (multiple domains). Additionally, Sano and colleagues [24] found that ADAS-Cog, and specifically the Delayed Recall task, can effectively identify those with MCI, but has limited capacity to detect those with mild AD. Noteworthy, Llano et al. [25] found that the ADAS 13-item could predict the conversion of MCI to dementia, in comparison with biomarker profiles extracted from volumetric magnetic resonance imaging (MRI), fluorodeoxyglucose-positron emission tomography (FDG-PET), or cerebrospinal fluid (CSF) records, whereas Fleisher et al. [26] support the prediction model from amnestic MCI to AD, which consists of the delayed 10-word recall, the New York University Paragraph Recall Test (NYU) delayed paragraph as well as the ADAS-Cog total score. However, adding volumetric MRI analysis into this model, the prediction indexes were not increased. Therefore, the previous studies claim that cognitive assessment can equally predict the conversion from MCI to AD compared to imaging biomarkers, which highlights the need to calculate the discriminant potential of these tests.
To sum up, given that previous studies [1] support the use of ADAS-Cog in predicting the conversion to AD in MCI population, and highlight its clinical utility in detecting those with MCI [27–29], current diagnostic processes should be enriched with newly developed studies which focus on the use of screening tools in specific clinical populations. The present study attempts to identify the diagnostic accuracy and discriminative capacity of the ADAS-Cog-G regarding MCI and subjective cognitive decline (SCD), which means providing cut-off scores, sensitivity, specificity, area under the curve (AUC), positive predictive value, and negative predictive value as well as concurrent validity of the test.
Purposes of the study
The purposes of the current study were:
a) To identify whether participants’ performance on the ADAS-Cog-G is influenced by the demographic variables of gender, age, and educational level;
b) To examine psychometric properties of ADAS-Cog-G, in specific, concurrent validity and discriminant validity as measured by the cut-off scores;
c) To differentiate people with MCI from those with SCD.
METHODS
Design
In the current study, which was a database one, we selected queries from the electronic database of the Day Care Centers (DCCs) of the Greek Association of Alzheimer’s Disease and Related Disorders (GAADRD). In more detail, the sample consisted of two different groups: a) community-dwelling older adults with SCD, and b) people with MCI (amnestic and non-amnestic of multiple domains) who had initially come to the DCCs, to undergo the neuropsychological and neurological evaluation due to memory complaints, during the period 2020 to 2022. All the study’s participants read the information sheet and signed the informed consent during the initial clinical visit, stating that the research group of the DCCs had permission to use for research purposes, their demographic data such as gender, age, and educational level, as well as data related to their performance in the neuropsychological tests. After signing the informed consent, the official neuropsychological assessment was administered along with the ADAS-Cog-G by neuropsychologists who constitute the expert group. The final diagnosis was set by the neurologists of the research team. It is noteworthy that the ADAS-Cog-G was not taken into account in the diagnostic process. The study was approved by the Scientific and Ethics Committee of the Greek Association of Alzheimer’s Disease and Related Disorders (Scientific Committee Approved Meeting Number: 58-5/27-05-2020, and was aligned with the principles outlined in the Helsinki Declaration [30].
Participants
From the initial sample of 538 people who underwent the neuropsychological examination from 2021 to 2022, 482 met the eligibility criteria and they were also randomized, and therefore were included in the current study. Additionally, Power Analysis was performed to select at least the minimum number of participants in line with the design of the study.
Therefore, four hundred and eighty-two (482) older adults (142 men and 340 women) with a mean age of 68.8 (SD 7.15) years and a mean educational level of 12.27 (SD 3.9) years of schooling, who were Greek native speakers, took part in the study: (a) people with SCD, (n = 176, 36 men and 140 women, age range: 50 to 85 years, M = 66.24, SD = 6.37, educational level range: 2 to 21 years, M = 13.33, SD = 3.58), and (b) people with MCI, (n = 306, 106 men and 200 women, age range: 51 to 87 years, M = 70.33, SD = 7.12, educational level range: 2 to 24 years, M = 11.68, SD = 4.06). The sample was split into three age groups (age by decades), that is 55–64 years old, 65–74 years old, 75 years old and above, and into three education levels, as measured by years of schooling, that is primary education or low educational level (≤to 6 years), secondary education or middle educational level (7–12 years) and high educational level (13 years or more). However, exact years of schooling and exact years of age were also used as predictor variables in an initial path model aiming at examining the demographic differences between diagnostic groups as well as at revealing the complex role of demographics in the ADAS-Cog-G total score. This model was considered important to precede the classic MANOVA, to formulate an initial, holistic “picture” of the role of group demographics in the total score of ADAS-Cog-G.
The study’s sample characteristics are presented in Tables 1 and 2.
Table 1
Descriptive statistics for the sample’s groups
Characteristics | SCD (n = 176) | MCI (n = 306) | p |
Mean (SD) | |||
Age | 66.24 (6.37) | 70.33 (7.12) | 0.001 |
Gender (Male/Female) | 36/140 | 106/200 | < 0.001 |
Years of education | 12.33 (3.58) | 11.68 (4.06) | < 0.001 |
M, median score; SD, standard deviation; SCD, subjective cognitive decline; MCI, mild cognitive impairment.
Table 2
Mean and Standard Deviations of the ADAS-Cog in the two groups
Characteristics | SCD (n = 176) | MCI (n = 306) | p |
Mean (SD) | p | ||
ADAS-Cog | |||
11 item total score | 8.51 (4.00) | 14.05 (3.23) | < 0.001 |
Memory | |||
Word recall (0-10) | 3.05 (2.58) | 4.40 (1.15) | < 0.001 |
Word recognition (0-12) | 0.89 (0.76) | 1.57 (1.04) | < 0.001 |
Orientation (0-8) | 0.02 (0.18) | 0.09 (0.31) | 0.007 |
Language | |||
Naming (0-5) | 0.05 (0.23) | 0.22 (0.47) | < 0.001 |
Word-finding difficulty (0-5) | 0.13 (0.33) | ||
Praxis | |||
Ideational (0-5) | 0.12 (0.37) | 0.13 (0.40) | |
Constructional (0-5) | 0.23 (0.45) | 0.40 (0.61) | 0.001 |
Delayed recall (0-10) | 2.71 (1.72) | 4.94 (1.67) | < 0.001 |
Number cancellation (0-5) | 1.25 (.82) | 1.81 (.99) | < 0.001 |
M, median score; SD, standard deviation; SCD, subjective cognitive decline; MCI, mild cognitive impairment; ADAS-Cog, Alzheimer’s Disease Assessment Scale – Cognitive Subscale.
Data collection and procedure
The two groups of the sample underwent the standard international diagnostic criteria to be evaluated as regards their neurocognitive status. In more detail, they completed the neurological examination, neuropsychological and neuropsychiatric assessment, neuroimaging, such as computed tomography, and MRI, and blood tests before the final decision on whether they should be recruited in the current study. The aforementioned assessments were evaluated by a consensus of a specialized neurologist as well as two neuropsychologists, considered experts in neurocognitive disorders.
The SCD group consisted of older adults who had initially come to the GAADRD in order to pass the diagnostic process reporting subjective memory complaints. Since, no cognitive impairment was found, therefore they were regarded as cognitively intact. However, according to the literature [31, 32], self-reported complaints could be a predictor of objective cognitive decline in the future, whereas a study by Poptsi and colleagues [33] supports that the group of SCD can be discriminated from healthy older adults and those with MCI.
The inclusion criteria for the SCD [34] were as follows: a) feelings of deteriorated memory performance not associated with existing depressive symptomatology, b) lack of objective cognitive impairment, according to the neuropsychological evaluation, and c) completing the criteria for stage 2 of the disease according to the Global Deterioration Scale (GDS) [35]. The inclusion criteria for MCI were: a) diagnosis of MCI according to Petersen [36] and Diagnostic and Statistical Manual of Mental Disorders (DSM-5) [37], b) Mini-Mental State Examination (MMSE) total score≥26, c) stage 3 of the disease according to the GDS [35], d) 1.5 standard deviation (SD) below the normal mean according to age and education, in at least one cognitive domain according to the utilized neuropsychological tests.
The exclusion criteria in both groups are the following: a) history of psychiatric illness such as Schizophrenia or affective disorder (Major Depression-General Anxiety Disorder), b) substance abuse or alcoholism, c) history of traumatic brain injury, d) neurological disorders such as hydrocephalus, Parkinson’s disease, encephalitis, brain tumor, epilepsy, and stroke history, e) thyroid, diabetes, g) drug treatment with opioids, B12, folate, and h) severe sensory deficits. It is worth noting that older adults with mild cardiovascular problems, such as mild hypertension were not excluded from the groups of the sample.
Instruments
The neuropsychological evaluation lasted for two hours, divided into two different face-to-face sessions to obtain the participants’ best performance reducing the possibility of getting tired. In the beginning the following neuropsychological tests, adapted to Greek population, were administered: MMSE (Greek cut-off scores from Fountoulakis et al. [38]), and Montreal Cognitive Assessment (MoCA; Greek cut-off scores from Poptsi et al. [39]), were initially administered because they are screening tools. Afterward, Rey’s Verbal Learning Test (RAVLT; Greek cut-off scores from Messinis et al. [40]) and the Extended Rivermead Behavioral Memory Test (RBMT; Greek cut-off score from Efklides and colleagues [41]) were given to assess participants’ verbal learning as well as episodic memory capacity. Visuospatial skills were measured by the Rey Complex Figure Test (RCFT) adapted for Greek population by Tsatali et al. [42, 43]. Verbal fluency was measured by the verbal fluency test (Greek cut-off score from Kosmidis et al. [44]). Executive functions were measured by the Stroop Neuropsychological Screening Test (SNST; Greek cut-off scores from Zalonis et al. [45]), as well as the Trail Making Test-part B (TMT-B; Greek cut-off scores from Zalonis et al. [46]). Finally, the Wechsler Adult Intelligence Scale (WAIS-FSIQ; Digit span and Digit symbol, Greek cut-off score from Tsatali et al. [47]) subtests were also administered to measure working memory performance. Additionally, functional capacity was measured by the Instrumental Activities of Daily Living (IADL; Greek cut-off scores from Theotoka et al. [48]), and the FUnctional Cognitive Assessment Scale (FUCAS; Greek cut-off scores from Kounti et al. [49]). Moreover, Clinical Dementia Rating (CDR) [50], a global scale developed to identify AD dementia as well as its severity stage, was also used. CDR constituted semi-structured interviews completed by the examiner to rate the participants’ performance in the following domains: memory, orientation, judgment and problem-solving, community affairs, home and hobbies, and personal care. Finally, the Geriatric Depression Scale (GDS-15; Greek cut-off scores from Fountoulakis and colleagues, validity measures were the following: Sensitivity = 92.23 and Specificity = 95.24, Cronbach’s alpha value = 0.94; whereas factor analysis revealed 4 factors [51]), the Short Anxiety Screening Test (SAST; Greek Cronbach’s alpha value was found to be 0.763 and ICC was 0.763; factor analysis revealed three factors with eigenvalues > 1.0 accounting for 60% of variance [52]), and the Perceived Stress Scale (PSS; Greek Cronbach’s alpha value was found to be 0.82 (for the full scale), whereas the correlation coefficients between Greek PSS with DASS-21 subscales scores were the following; correlation with stress (r = 0.64), depression (r = 0.61), and anxiety (r = 0.54) [53], were used to measure depressive and anxiety symptoms.
ADAS-Cog description
Rosen et al. [54] eveloped in 1984 a new screening neuropsychological tool that measures a large variety of cognitive functions as well as motor ability and perception. ADAS includes 21 items assessing the primary symptoms of AD. The Greek translation includes two parts; the first measures cognitive function through cognitive tasks administration; it includes 13 items with scores up to 70; whereas the second evaluates behavioral patterns and signs of psychopathology through the clinical interview. The first part is called ADAS-Cog; it includes 9 cognitive subtests as well as a brief interview that aims to assess various spontaneous language features (e.g., fluency in speech, comprehension, understanding, and quality of speech). The 9 cognitive subtests are the following: immediate word recall, comprehension of commands, constructional praxis, object and finger naming, ideational praxis, orientation, delayed recall, number cancellation, and word recognition [24]. Higher scores reflect poorer performance or greater cognitive, behavioral, and functionality impairment [55]. The ADAS max score is 110, including ADAS-Cog and ADAS-Noncog subtests.
Statistical analysis
Power Analysis was initially performed, to determine the required number of participants needed. The results showed that a sample size of at least 280 participants was required to observe a small between-groups effect size (Cohen’s f = 0.25) with α level of 0.05 and power of 0.95.
Initially, descriptive statistics were calculated. Path analysis followed, to describe group differences due to demographics and their potentially complex (direct and indirect effects) role in the formulation of the total score of ADAS-Cog-G. Path analysis was conducted in EQS (version 6.4) statistical software [56]. A maximum likelihood estimation procedure was performed. Two variables (Gender and Diagnostic group) were dichotomous (0, 1, see Bentler [56] for details regarding MIMIC modeling). Regarding the confirmation of a path model, a non-significant level of the Goodness of Fit index χ2, namely p > 0.05, is indicative of a good fit of the model to the data. When the value of the root mean square error of approximation index (RMSEA) is < 0.05, it is an indication of the good fit of the model to the data. RMSEA values ranging from 0.06 to 0.08 indicate a reasonable and, therefore, acceptable approximation error. Additional support for the fit of the solution is evidenced by a 90% confidence interval range of the RMSEA, whose upper limit is below the cut-off values of 0.08–0.10. However, the RMSEA value is relatively expanded in many cases, and this is reflected in the confidence interval range. In such a case, RMSEA should be considered as a model fit index, but with caution and taking into account other indices as well. The SRMR index is an absolute measure of fit and is defined as the standardized difference between the observed correlation and the predicted correlation. Because the SRMR is an absolute measure of fit, a value of zero indicates perfect fit. A value less than 0.08 is generally considered a good fit.
Conversely, the Comparative Fit Index (CFI) examines whether the data fit a hypothesized path model compared to the basic model. Values greater than 0.90 indicate an adequate fit of the model to the data, whereas values close to 1.00 indicate a good fit. To improve model fit, we examined the modification indices, namely the Wald and the Lagrange tests, which represent frequently used statistics to identify focal areas of a misfit in a path analysis solution [57]. Subsequently, Multivariate Analysis of Variance (MANOVA) was performed to test for demographic (gender, age-group, educational level) and/or diagnostic effects or their interactions on ADAS-Cog-G performance. The Bonferroni test was also used for post hoc multiple comparisons, to test for all the significant interactions. Additionally, Pearson’s correlation coefficients were conducted to explore the concurrent validity to identify the diagnostic accuracy of the ADAS-Cog-G to discriminate SCD from MCI population. In detail, the receiver operating characteristics (ROC) curve was calculated wherein larger AUC indicate better diagnostic accuracy. Moreover, the optimal cut-off points were also determined. For each cut-off point the sensitivity (the probability for subjects with disease to have a positive test), and specificity (the probability for subjects without disease to have a negative test) levels were conducted as well as their positive predictive values (PPV; the probability of disease in participants who have a positive test), and negative predictive values (NPV; probability of the classification “without disease” in participants who have a negative test). The AUC metric was used for identifying whether the ADAS-Cog-G discriminant potential is poor (0.51–0.69), acceptable (0.7–0.79), good (0.8–0.89), excellent (0.9–0.99) or perfect (1.0), according to the relative literature [58].
The statistical analyses were performed by the SPSS software version 27 (IBM Corp, Armonk, New York), whereas an alpha value of 0.05 (two-tailed) was used. The baseline sample was not randomly selected, since the participants were visitors of the DCCs, to be tested for preventive purposes. However, because of the unequal group number of participants (people with SCD and MCI) the sample was randomized through the respective command of the SPSS (in 30%). Therefore, via this method, a random sample of cases was generated.
RESULTS
The path model, which was almost perfectly confirmed, χ2 (2, 482) = 3.807, p < 0.05, CFI = 0.99, SRMR = 0.02, RMSEA = 0.04 (90% CI: 0.00 – 0.10), shows that the group of SCD is constituted of more women than men, and is significantly younger and with more years of schooling compared to the MCI group. Given that the MCI group has a significantly higher score in ADAS-Cog-G than the SCD group, gender, exact age, and education as years of schooling appear to indirectly affect -via their directional relationships with diagnostic group- ADAS-Cog-G total score. However, besides these associations, age and education appear to directly affect ADAS-Cog-G total score as well. This means that besides diagnosis, more years of age and fewer years of schooling can play a significant role in the performance of ADAS-Cog. It is very interesting that according to the model (see Fig. 1), 46.3% of the sample’s performance can be explained by diagnosis, age, and education.
Fig. 1
The directional relationships between demographic factors, diagnostic group, and ADAS-COG total score. *All paths are significant at p < 0.05; E, measurement error.
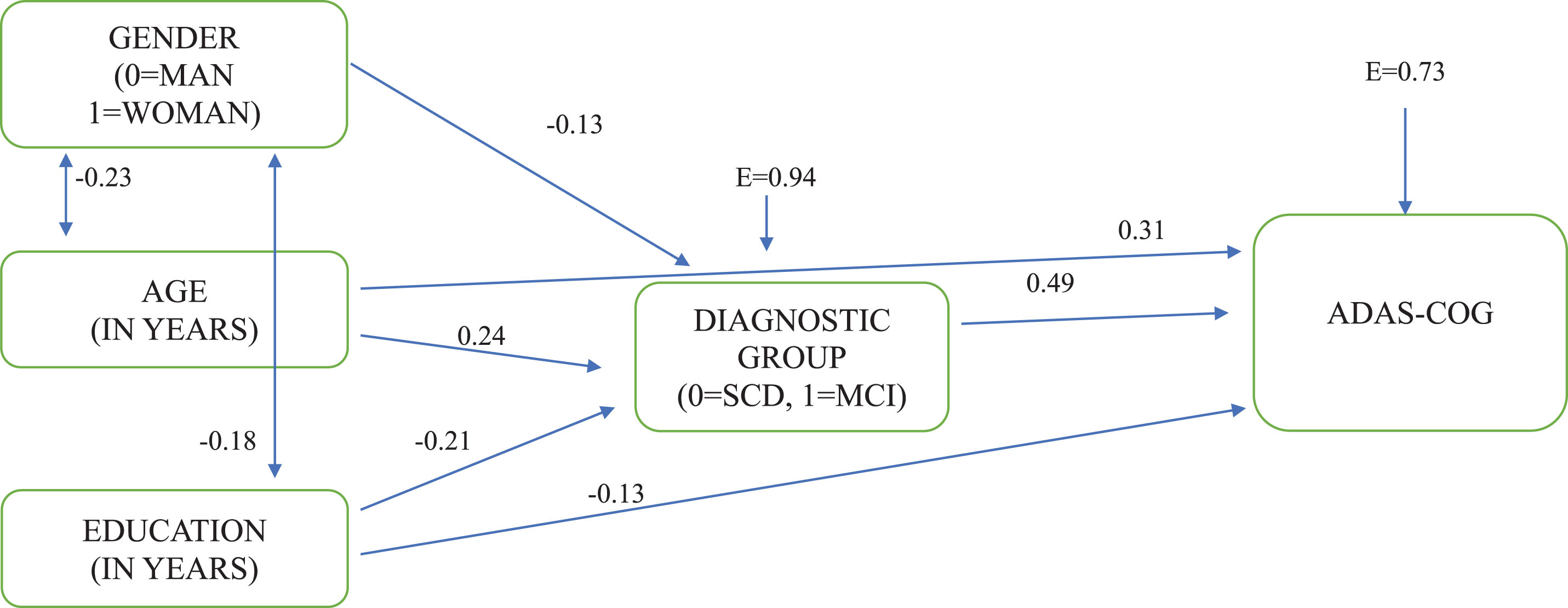
Given the complex role of demographics as shown in the path model, we considered that it would be useful to proceed with multivariate analysis of variance (MANOVA), in order to examine demographic effects (age group: 55–64 years = 1st age group; 65–74 years = 2nd age group; 75 years and older = 3rd age group), gender (men, women), and educational level (≤6 years of schooling = low educational level LEL; 7–12 years = middle educational level MEL; 13 years and more = high educational level HEL), as well as diagnostic group effects on ADAS-Cog-G scores in a more normative way. Results showed that there was no statistically significant effect of gender (p = 0.625). However, age group (p < 0.001), educational level (p < 0.001), and diagnostic group (p < 0.001) had statistically significant effects on ADAS-Cog-G sum score.
Psychometric properties
To explore concurrent validity, Pearson correlation coefficients were computed for ADAS-Cog-G, MMSE, MoCA, and CDR. The results were statistically significant and negative between ADAS-Cog-G and MMSE (r=–0.32; p < 0.001), and MoCA (r=–0.54; p < 0.001); whereas they were positive between ADAS-Cog-G and CDR (r = 0.37; p < 0.001). All correlations are placed in Table 3 below.
Table 3
Correlation between ADAS-Cog and various screening tests in the groups of SCD and MCI
Screening tests | 1 | 2 | 3 | 4 |
1. ADAS Cog | – | –0.319** | –0.534** | 0.365** |
2. MMSE | – | 0.429** | –0.371** | |
3. MoCA | – | –0.443** | ||
4. CDR | – |
ADAS-Cog, Alzheimer’s Disease Assessment Scale Cognitive Subscale; MMSE, Mini-Mental State Examination; MoCA, Montreal Cognitive Assessment; CDR, Clinical Dementia Rating.
ADAS Cog-G sum score’s discriminant potential for SCD and MCI groups
To identify the level of discriminant potential of ADAS-Cog-G, the AUC of the ROC curve was calculated. Therefore, according to the AUC, the ADAS Cog-G sum score discriminant potential regarding SCD and MCI was at a mean score acceptable to perfect.
Cut-off scores, AUC, Sensitivity and Specificity levels as well as PPV and NPV are placed in Table 4.
Table 4
Diagnostic ADAS-Cog classification between the groups of SCD and MCI
ADAS-Cog | AUC | Sensitivity | Specificity | PPV | NPV | p | |
Cutoff | |||||||
SCD-MCI | |||||||
LEL | |||||||
55–64 | 9.65 | 0.947 | 89.5 | 100.0 | 0.85 | 1.00 | 0.015 |
65–74 | 13.40 | 0.750 | 60.0 | 77.8 | 0.63 | 0.92 | 0.009 |
75< | ns* | 0.175 | |||||
MEL | |||||||
55–64 | 9.13 | 0.893 | 75.0 | 83.3 | 0.81 | 0.97 | < 0.001 |
65–74 | 10.65 | 0.892 | 96.0 | 67.0 | 0.81 | 0.97 | < 0.001 |
75< | 12.30 | 0.986 | 91.3 | 83.3 | 0.94 | 1.00 | < 0.001 |
HEL | |||||||
55–64 | 8.35 | 0.906 | 91.3 | 79.0 | 0.83 | 0.98 | < 0.001 |
65–74 | 9.95 | 0.932 | 90.0 | 86.4 | 0.85 | 1.00 | < 0.001 |
75< | 12.10 | 0.877 | 89.1 | 71.4 | 0.74 | 1.00 | 0.001 |
SCD, subjective cognitive decline; MCI, mild cognitive impairment; ns, non significant; LEL, low educational level; MEL, middle educational level; HEL, high educational level; AUC, area under the curve; PPV, positive predictive value; NPV, negative predictive value. Note: Sensitivity and Specificity values are expressed in percentage.
DISCUSSION
In the current study, we used the ADAS-Cog-G, which has been initially translated and adapted in Greek older adult population by Tsolaki et al. [23]. However, in their study they used the ADAS sum score, which was found to perfectly discriminate patients with AD dementia from their healthy counterparts at the score level of 13/14 and/or 14/15. Because in the current study we used ADAS Cog-G sum score, the results between the two studies are not comparable. Up to now, there are still no studies identifying its diagnostic utility to differentiate older adults who are healthy but express memory complaints from those with MCI in Greece, although it is widely used in clinical trials [59] including MCI population, as mentioned above.
According to the aforementioned results, age and educational level significantly affect ADAS-Cog-G sum score, which is in agreement with previous findings across literature, whereas gender was found to have no effect on ADAS-Cog-G total score. In specific, the sum score of ADAS-Cog-G is strongly affected by low educational level and more than 75 years of age. In more detail, the discriminant potential of the ADAS-Cog-G to differentiate older adults with SCD from MCI population is excellent for the age group of people between 55–64 years old, which highlights that ADAS-Cog-G is strongly affected by age. Furthermore, according to our results, ADAS-Cog-G sum score cannot discriminate older adults older than 75 years old with SCD from those with MCI. Hence, it can be assumed that it can be preferably used for younger seniors. As regards educational level, results showed slightly better AUC values for those participants who had more than 13 years of education, whereas sensitivity and specificity indexes were also higher compared to the low and medium educational levels. This is also in line with the study of Liu et al. [19] who found that highly educated older adults with dementia may overlap with low educated cognitively healthy seniors. The cut-off scores in the case of low educational level had satisfactory sensitivity and specificity only in those who belong to the youngest age group.
A wealth of data has focused on the clinical utility of ADAS-Cog in identifying patients with other than dementia clinical conditions. Results from Brazil [5], show that the cut-off scores for people with MCI and AD dementia were respectively 10.9 and 22.9 for 0–4 schooling years, 7.8 and 22.4 for 5–11 schooling years, and 6.2 and 15.4 for 12 years of schooling and more. Hence, it seems that in accordance with our results, Brazilian participants’ ADAS-Cog performance was strongly affected by educational level; however, no age effect has been reported. Furthermore, data from Singapore [29] concerning the ADAS-Cog potential to discriminate MCI and AD dementia showed that cut-off score of≤4 could significantly differentiate participants with MCI from HC (with ADAS-Cog scale range = 0–70), whereas cut-off score of≤12 could differentiate mild AD dementia population from their MCI counterparts. Similarly, Monllau et al. [60] provide evidence that the Colombian ADAS-Cog cut-off point has been determined to be≤5 for MCI population, and≤12 (AUC = 0.94; sensitivity value = 0.89; specificity value = 0.88) for people with dementia. However, according to their study, the cut-off point for MCI detection had poor sensitivity (0.73) and specificity (0.69) levels, which seems it might be even lower taking into account cross-cultural differences. Finally, the cut-off point of 12 found to differentiate MCI from mild AD dementia had good sensitivity (0.86) and specificity (0.89) levels, which is in accordance with the majority of relevant studies. As regards Chinese ADAS-Cog performance, Liu et al. [19] extracted cut-off scores only stratified by educational level providing the median score of 11.9 for Chinese illiterates (for participants with < 4 years of schooling), which is partially in agreement to our study, because we found the cut-off points of 9.65 and 13.40 for those with 55–64 years old and 65–74 years old respectively. Similarly to Chinese results, the Arabic [61] and Slovenian versions of ADAS-Cog scores [9] were found to be 10 and 11, as well as 10/11 points for MCI (having good sensitivity and specificity scores), and 11/12 for those with AD dementia (having excellent to perfect sensitivity and specificity indexes) respectively. However, those cut-off scores were not stratified by demographic variables. Therefore no clear comparison can be made to our study. Concerning the conversion rates from Italian participants with amnestic MCI to AD dementia, Rozzini et al. [1] found that the best cut-off score adjusted for education to predict the conversion rate from amnestic MCI to AD dementia after one-year follow-up was the 9.5 (AUC)=0.67, though having poor to acceptable sensitivity (0.62) and specificity (0.73) values. The Italian cut-off scores are also in line with the Portuguese study of Nogueira and colleagues [7]. They found that the optimal cut-off point of≤9 to detect older adults with MCI in a Portuguese cohort has poor sensitivity index (AUC = 0.835; sensitivity = 58% and specificity = 91%). In accordance with previous studies, the Portuguese cut-off scores were not stratified by age and education. Finally, Paddick et al. [8] reported a median score of 12.8 for healthy controls as well as the medium score of 28.75 for those with mild/moderate dementia diagnosis in the rural sub-Saharan Africa population. These results are in agreement with ours, because the 12-point was found to discriminate healthy older adults from those with MCI who are older than 75 years old and have more than 7 years of schooling. Finally, in the Turkish version [11] ADAS validation scores were included instead of cut-off scores, and therefore, our results are not comparable to this study.
The aforementioned Greek cut-off scores are higher than that expected for people with normal cognition according to the various studies. This is probably the result of participants’ differential socio-economic status, different educational levels as well as cross-cultural differences. A significant factor that could explain this controversy is the fact that we computed different cut-off scores for separate age groups and educational levels, because these variables strongly affect the ADAS-Cog-G sum scores, whereas other studies like the one from Graham et al. [62], did not stratify ADAS-Cog cut-off scores by educational level. Moreover, another reason that could probably explain the controversial results between our study to previous ones, is that in this study participants who had 11–12 years of schooling were considered the ‘medium’, whereas previous studies reported as mean score approximately the 15 years of schooling, which could probably explain better ADAS-Cog scores due to increased educational level. In specific, Youn et al. [4] used the cut-off score of 15/16 to detect those with AD dementia. However, the control group of their study had lower educational level than AD participants. Furthermore, of foremost importance is that in previous studies like those from Ben Jemaa et al. [61], Graham et al. [62], and Kolibáš et al. [6], the ADAS-Cog sum score includes seven out of nine cognitive ADAS-Cog tasks, whereas the number cancellation as well as delayed recall subtasks are missing, plus the four non cognitive subtests which are the following; Spoken language ability, Comprehensive of spoken language, Commands, and Recall of test instructions. Additionally, studies like the one from Graham et al. [62] support that the delayed recall sub test should be assumed as a separate test from the sum of tests used to calculate the ADAS-Cog sum score. On the other hand, the total ADAS-Cog score in this study was calculated from the nine cognitive subtests, mentioned in the Methods section, which probably explains why our cut-off scores are increased compared to those studies. Finally, the number of participants can be an explanative factor to detect controversies across studies regarding differential cut-off scores. For example, the study from Arabic population recruited 25 people with AD dementia and 33 non-AD [61], whereas the same distribution was made in the Slovenian study [9] which recruited 61 healthy controls, 32 people with MCI and 35 people with AD dementia.
Considering concurrent validity examination, ADAS-Cog-G has very satisfactory concurrent validity indexes, because it is highly correlated with screening tools, specifically MMSE, MoCA, and CDR which measure general cognitive functioning, something that is also in line with previous studies [8, 9, 61].
Given that screening tools constitute a significant cornerstone for the diagnosis of clinical neurological conditions, and mainly MCI as well as dementia, it is quite essential to provide neuropsychologists in Greece with sensitive and specified tools in their clinical practice during diagnosis and follow-up assessment, as well as research protocols’ implementation. What is also of foremost importance is to identify which neuropsychological tests can accurately measure the efficacy of non-pharmacological interventions delivered in people living with MCI as well as dementia [63] and therefore, provide their clinical utility in daily practice. Additionally, according to Kueper et al. [64], ADAS-Cog can be assumed as a very satisfactory screening tool to assess the efficacy of treatment in dementia. Taking this significant need into account, creating validation studies to adapt these tools in the different cultural frames, it is quite important for testing whether clinicians can take significant information about their stakeholders either patients or people with SCD and MCI.
Conclusively, the results proved the capacity of ADAS-Cog-G to differentiate participants of a Greek MCI cohort from their healthy SCD counterparts. However, it is not sensitive and specified enough for those with low educational level and older age.
Limitations
In this study, we did not calculate reliability indexes. Future endeavors should focus on extracting internal as well as test-retest reliability scores. Another limitation is the fact that we did not calculate a cut-off score between MCI and AD dementia, which is also crucial to draw safer conclusions. Another significant limitation is that, Ben Jemaa et al. [61] did principal component analysis that extracted three ADAS-Cog-G factors; memory, language and praxis, but no relevant analysis has been made in this study. Finally, due to MCI heterogeneity, future research should do relevant studies separating amnestic and non-amnestic populations with MCI and therefore calculating different cut-off scores for these groups.
AUTHOR CONTRIBUTIONS
Marianna Tsatali (Conceptualization; Formal analysis; Investigation; Methodology; Project administration; Resources; Software; Writing – original draft); Despina Moraitou, Ph.D (Formal analysis; Project administration; Supervision; Writing – review & editing); Moses Gialaouzidis, BSc (Data curation; Resources); Evaggelia Bakoglidou, BSc (Data curation); Vasilis Psaltis (Data curation); Natalia Bertzes (Data curation); Hany Ibrahim Hassanin, Ph.D (Supervision; Visualization); Eudokia Emmanouilidou (Data curation); Michael Totonidis (Data curation); Nikoleta Frantzi (Data curation); Konstantina Avdikou, MSc (Data curation); Andromachi Gavra, MSc (Data curation); Alexandra Diamantidou, BSc (Data curation); Nikolina Kapsali, MSc (Data curation); Eleni Kouroundi, BSc (Data curation); Magda Tsolaki, Ph.D (Conceptualization; Supervision; Writing – review & editing).
ACKNOWLEDGMENTS
The authors have no acknowledgments to report.
FUNDING
The authors have no funding to report.
CONFLICT OF INTEREST
The authors have no conflict of interest to report.
DATA AVAILABILITY
The data supporting the findings of this study are not available due to privacy or ethical restrictions.
REFERENCES
[1] | Rozzini L , Chilovi BC , Bertoletti E , Conti M , Delrio I , Trabucchi M , Padovani A ((2008) ) The importance of Alzheimer disease assessment scale-cognitive part in predicting progress for amnestic mild cognitive impairment to Alzheimer disease. J Geriatr Psychiatry Neurol 21: , 261–267. |
[2] | Hannesdottir K , Snaedal J ((2002) ) A study of the Alzheimer’s Disease Assessment Scale-Cognitive (ADAS-Cog) in an Icelandic elderly population. Nord J Psychiatry 56: , 201–206. |
[3] | Peña-Casanova J , Aguilar M , Santacruz P , Bertran-Serra I , Hernández G , Sol JM , Pujol A , Blesa R ((1997) ) Adaptation andnormalization of the Alzheimer’s disease Assessment Scale for Spain(NORMACODEM) (II). Neurologia 12: , 69–77. |
[4] | Youn JC , Lee DY , Kim KW , Lee JH , Jhoo JH , Lee KU , Ha JI , Woo JI ((2002) ) Development of the Korean version of Alzheimer’s disease assessment scale (ADAS-K). Int J Geriatr Psychiatry 17: , 797–803. |
[5] | Schultz RR , Siviero MO , Bertolucci PH ((2001) ) The cognitive subscale of the “Alzheimer’s disease assessment scale” in a Brazilian sample. Braz J Med Biol Res 34: , 1295–302. |
[6] | Kolibáš E , Kořínková V , Novotný V , Hunáková D , Vajdičková K ((2000) ) P03. 443 ADAS-COG(Alzheimer’s Disease Assessment Scale-Cognitive subscale)-validationof the Slovak version. Eur Psychiatry 15: , 443. |
[7] | Nogueira J , Freitas S , Duro D , Almeida J , Santana I ((2018) ) Validation study of the Alzheimer’s disease assessment scale–cognitive subscale (ADAS-Cog) for the Portuguese patients with mild cognitive impairment and Alzheimer’s disease. Clin Neuropsychol 32: , 46–59. |
[8] | Paddick SM , Kisoli A , Mkenda S , Mbowe G , Gray WK , Dotchin C , Ogunniyi A , Kisima J , Olakehinde O , Mushi D , Walker RW ((2017) ) Adaptation and validation of the Alzheimer’s Disease Assessment Scale–Cognitive (ADAS-Cog) in a low-literacy setting in sub-Saharan Africa. Acta Neuropsychiatr 29: , 244–251. |
[9] | Ulbl J , Rakusa M ((2022) ) Validation of Slovenian version of ADAS-Cog for patients with mild cognitive impairment and Alzheimer’s disease. Acta Neurol Belg 122: , 695–702. |
[10] | Jiang Y , Yang H , Zhao J , Wu Y , Zhou X , Cheng Z ((2020) ) Reliability and concurrent validity of Alzheimer’s disease assessment scale-cognitive subscale, Chinese version (ADAS-Cog-C) among Chinese community-dwelling older people population. Clin Neuropsychol 34: , 43–53. |
[11] | Mavioglu H , Gedizlioglu M , Akyel S , Aslaner T , Eser E ((2006) ) The validity and reliability of the Turkish version of Alzheimer’s Disease Assessment Scale-Cognitive Subscale (ADAS-Cog) in patients with mild and moderate Alzheimer’s disease and normal subjects. Int J Geriatr Psychiatry 21: , 259–265. |
[12] | Cano SJ , Posner HB , Moline ML , Hurt SW , Swartz J , Hsu T , Hobart JC ((2010) ) The ADAS-cog in Alzheimer’s disease clinical trials: Psychometric evaluation of the sum and its parts. J Neurol Neurosurg Psychiatry 81: , 1363–1368. |
[13] | Schneider LS , Sano M ((2009) ) Current Alzheimer’s disease clinical trials: Methods and placebo outcomes. Alzheimers Dement 5: , 388–397. |
[14] | Skinner J , Carvalho J , Potter GC , Thames A , Zelinski E , Crane PK , Gibbons LE ((2012) ) The Alzheimer’s disease assessment scale-cognitive- plus (ADAS-Cog-Plus): An expansion of the ADAS-Cog to improve responsiveness in MCI. Brain Imaging Behav 6: , 489–501. |
[15] | Farlow M , He Y , Tekin S , Xu J , Lane R , Charles HC ((2004) ) Impact of APOE in mild cognitive impairment. Neurology 63: , 1898–1901. |
[16] | Malouf R , Birks J ((2004) ) Donepezil for vascular cognitive impairment. Cochrane Database Syst Rev, CD00439. |
[17] | Emre M , Aarsland D , Albanese A , Byrne EJ , Deuschl G , De Deyn PP , Durif F , Kulisevsky J , van Laar T , Lees A , Poewe W , Robillard A , Rosa MM , Wolters E , Quarg P , Tekin S , Lane R ((2004) ) Rivastigmine for dementia associated with Parkinson’s disease. N Engl J Med 351: , 2509–2518. |
[18] | Weyer G , Erzigkeit H , Kanowski S , Ihl R , Hadler D ((1997) ) Alzheimer’s Disease Assessment Scale: Reliability and validity in a multicenter clinical trial. Int Psychogeriatr 9: , 123–138. |
[19] | Liu HC , Teng EL , Chuang YY , Lin KN , Fuh JL , Wang PN ((2002) ) The Alzheimer’s Disease Assessment Scale: Findings from a low-education population. Dement Geriatr Cogn Disord 13: , 21–26. |
[20] | Paddick SM , Longdon AR , Kisoli A , Dotchin C , Gray WK , Dewhurst F , Chaote P , Kalaria R , Jusabani AM , Walker R ((2013) ) Dementia prevalence estimates in sub-Saharan Africa: Comparison of two diagnostic criteria. Glob Health Action 6: , 19646. |
[21] | Gray WK , Paddick SM , Kisoli A , Dotchin CL , Longdon AR , Chaote P , Samuel M , Jusabani AM , Walker RW ((2014) ) Development and validation of the identification and intervention for dementia in elderly Africans (IDEA) study dementia screening instrument. J Geriatr Psychiatry Neurol 27: , 110–118. |
[22] | Rockwood K , Dai D , Mitnitski A ((2008) ) Patterns of decline and evidence of subgroups in patients with Alzheimer’s disease taking galantamine for up to 48 months. Int J Geriatr Psychiatry 23: , 207–14. |
[23] | Tsolaki M , Fountoulakis K , Nakopoulou E , Kazis A , Mohs RC ((1997) ) Alzheimer’s Disease Assessment Scale: The validation of the scale in Greece in elderly demented patients and normal subjects. Dement Geriatr Cogn Disord 8: , 273–280. |
[24] | Sano M , Raman R , Emond J , Thomas RG , Petersen R , Schneider LS , Aisen PS ((2011) ) Adding delayed recall to the Alzheimer disease assessment scale is useful in studies of mild cognitive impairment but not Alzheimer disease. Alzheimer Dis Assoc Disord 25: , 122–127. |
[25] | Llano DA , Laforet G , Devanarayan V ((2011) ) Derivation of a new ADAS-cog composite using tree-based multivariate analysis: Prediction of conversion from mild cognitive impairment to Alzheimer disease. Alzheimer Dis Assoc Disord 25: , 73–84. |
[26] | Fleisher AS , Sun S , Taylor C , Ward CP , Gamst AC , Petersen RC , Jack CR , Aisen PS , Thal LJ , For the Alzheimer’s Disease Cooperative Study ((2008) ) Volumetric MRI vs clinical predictors of Alzheimer disease in mild cognitive impairment. Neurology 70: , 191–199. |
[27] | Raghavan N , Samtani MN , Farnum M , Yang E , Novak G , Grundman M , Narayan V , DiBernardo A , Alzheimer’s Disease Neuroimaging Initiative ((2013) ) The ADAS-Cog revisited: Novel composite scales based on ADAS-Cog to improve efficiency in MCI and early AD trials. Alzheimers Dement 9: , 21–31. |
[28] | Pyo G , Elble RJ , Ala T , Markwell SJ ((2006) ) The characteristics of patients with uncertain/ mild cognitive impairment on the Alzheimer disease assessment scale-cognitive subscale. Alzheimer Dis Assoc Disord 20: , 16–22. |
[29] | Zainal NH , Silva E , Lim LLH , Kandiah N ((2016) ) Psychometric properties of Alzheimer’s disease assessment scale-cognitive subscale for mild cognitive impairment and mild Alzheimer’s disease patients in an Asian context. Ann Acad Med Singap 45: , 273–283. |
[30] | World Medical Association ((1997) ) Declaration of Helsinki. Recommendations guiding physicians in biomedical research involving human subjects. J Am Med Assoc 277: , 925–926. |
[31] | Dufouil C , Fuhrer R , Alpérovitch A ((2005) ) Subjective cognitivecomplaints and cognitive decline: Consequence or predictor? Theepidemiology of vascular aging study. J Am Geriatr Soc 53: , 616–621. |
[32] | Hohman TJ , Beason-Held LL , Lamar M , Resnick SM ((2011) ) Subjective cognitive complaints and longitudinal changes in memory and brain function. Neuropsychology 25: , 125. |
[33] | Poptsi E , Moraitou D , Tsardoulias E , Symeonidis AL , Tsolaki M ((2020) ) Is the discrimination of subjective cognitive decline from cognitively healthy adulthood and mild cognitive impairment possible? A pilot study utilizing the R4Alz Battery. J Alzheimers Dis 77: , 715–732. |
[34] | Jessen F , Amariglio RE , Van Boxtel M , Breteler M , Ceccaldi M , Chételat G , Dubois B , Dufouil C , Ellis KA , van der Flier WM , Glodzik L , van Harten AC , de Leon MJ , McHugh P , Mielke MM , Molinuevo JL , Mosconi L , Osorio RS , Perrotin A , Petersen RC , Rabin LA , Rami L , Reisberg B , Rentz DM , Sachdev PS , de la Sayette V , Saykin AJ , Scheltens P , Shulman MB , Slavin MJ , Sperling RA , Stewart R , Uspenskaya O , Vellas B , Visser PJ , Wagner M , Subjective Cognitive Decline Initiative ((2014) ) A conceptual framework for research onsubjective cognitive decline in preclinical Alzheimer’s disease. Alzheimers Dement 10: , 844–852. |
[35] | Reisberg B , Ferris SH , de Leon MJ , Crook T ((1982) ) The Global Deterioration Scale for assessment of primary degenerative dementia. Am J Psychiatry 139: , 1136–1139. |
[36] | Petersen RC ((2016) ) Mild cognitive impairment.(2 Dementia). Continuum (Minneap Minn) 22: , 404–418. |
[37] | Roehr B ((2013) ) American Psychiatric Association explains DSM-5. BMJ 346: , f3591. |
[38] | Fountoulakis KN , Tsolaki M , Chantzi H , Kazis A ((2000) ) Mini Mental State Examination (MMSE): A validation study in Greece. Am J Alzheimers Dis Other Demen 15: , 342–345. |
[39] | Poptsi E , Moraitou D , Eleftheriou M , Kounti-Zafeiropoulou F , Papasozomenou C , Agogiatou C , Bakoglidou E , Batsila G , Liapi D , Markou N , Nikolaidou E , Ouzouni F , Soumpourou A , Vasiloglou M , Tsolaki M ((2019) ) Normative data for the Montreal Cognitive Assessment in Greek older adults with subjective cognitive decline, mild cognitive impairment and dementia. J Geriatr Psychiatry Neurol 32: , 265–274. |
[40] | Messinis L , Tsakona I , Malefaki S , Papathanasopoulos P ((2007) ) Normative data and discriminant validity of Rey’s Verbal Learning Test for the Greek adult population. Arch Clin Neuropsychol 22: , 739–752. |
[41] | Efklides A , Yiultsi E , Kangellidou T , Kounti F , Dina F , Tsolaki M ((2002) ) Wechsler Memory Scale, Rivermead Behavioral Memory Test, and Everyday Memory Questionnaire in healthy adults and Alzheimer’s patients. Eur J Psychol Assess 18: , 63. |
[42] | Tsatali M , Avdikou K , Gialaouzidis M , Minopoulou D , Emmanouel A , Kouroundi E , Tsolaki M ((2022) ) The discriminant validity of Rey Complex Figure Test (RCFT) in subjective cognitive decline, mild cognitive impairment (multiple domain) and Alzheimer’s disease dementia (ADD; mild stage) in Greek older adults. Appl Neuropsychol Adult, doi: 10.1080/23279095.2022.2037089. |
[43] | Tsatali M , Emmanouel A , Gialaouzidis M , Avdikou K , Stefanatos C , Diamantidou A , Kouroundi E , Messini C , Tsolaki M ((2022) ) Rey complex figure test (RCFT): Norms for the Greek older adult population. Appl Neuropsychol Adult 29: , 958–966. |
[44] | Kosmidis MH , Vlahou CH , Panagiotaki P , Kiosseoglou G ((2004) ) The verbal fluency task in the Greek population: Normative data, and clustering and switching strategies. J Int Neuropsychol Soc 10: , 164–172. |
[45] | Zalonis I , Christidi F , Bonakis A , Kararizou E , Triantafyllou NI , Paraskevas G , Kapaki E , Vasilopoulos D ((2009) ) The stroop effect in Greek healthy population: Normative data for the Stroop Neuropsychological Screening Test. Arch Clin Neuropsychol 24: , 81–88. |
[46] | Zalonis I , Kararizou E , Triantafyllou NI , Kapaki E , Papageorgiou S , Sgouropoulos PEEA , Vassilopoulos D ((2008) ) A normative study of the trail making test A and B in Greek adults. Clin Neuropsychol 22: , 842–850. |
[47] | Tsatali M , Poptsi E , Moraitou D , Agogiatou C , Bakoglidou E , Gialaouzidis M , Papasozomenou C , Soumpourou A , Tsolaki M ((2021) ) Discriminant validity of the WAIS-R digit symbol substitution test in subjective cognitive decline, mild cognitive impairment (amnestic subtype) and Alzheimer’s disease dementia (ADD) in Greece. Brain Sci 11: , 881. |
[48] | Theotoka I , Kapaki E , Vagenas V , Ilias I , Paraskevas GP , Liappas I ((2007) ) Preliminary report of a validation study of Instrumental Activities of Daily Living in a Greek sample. Percept Mot Skills 104: , 958–960. |
[49] | Kounti F , Tsolaki M , Kiosseoglou G ((2006) ) Functional cognitive assessment scale (FUCAS): A new scale to assess executive cognitive function in daily life activities in patients with dementia and mild cognitive impairment. Hum Psychopharmacol 21: , 305–311. |
[50] | Morris JC ((1993) ) The Clinical Dementia Rating (CDR): Current version and scoring rules. Neurology 43: , 2412–2414. |
[51] | Fountoulakis KN , Tsolaki M , Iacovides A , Yesavage J , O’hara R , Kazis A , Ierodiakonou C ((1999) ) The validation of the short form of the Geriatric Depression Scale (GDS) in Greece. Aging Clin Exp Res 11: , 367–372. |
[52] | Grammatikopoulos IA , Sinoff G , Alegakis A , Kounalakis D , Antonopoulou M , Lionis C ((2010) ) The short anxiety screening test in Greek: Translation and validation. Ann Gen Psychiatry 9: , 1. |
[53] | Andreou E , Alexopoulos EC , Lionis C , Varvogli L , Gnardellis C , Chrousos GP , Darviri C ((2011) ) Perceived stress scale: Reliability and validity study in Greece. Int J Environ Res Public Health 8: , 3287–3298. |
[54] | Rosen WG , Mohs RC , Davis KL ((1984) ) A new rating scale for Alzheimer’s disease. Am J Psychiatry 141: , 1356–1364. |
[55] | Lezak MD , Howieson DB , Loring DW ((2004) ) The behavioral geography of the brain. Neuropsychol Assess 4: , 39–85. |
[56] | Bendler PM ((2005) ) EQS 6 Structural Equation Program Manual. Multivariate Software, Inc., Encino, CA. |
[57] | Brown TA ((2006) ), Confirmatory Factor Analysis for Applied Research. Guilford Press, New York. |
[58] | Carter JV , Pan J , Rai SN , Galandiuk S ((2016) ) ROC-ing along: Evaluation and interpretation of receiver operating characteristic curves. Surgery 159: , 1638–1645. |
[59] | Huang LK , Kuan YC , Lin HW , Hu CJ ((2023) ) Clinical trials of new drugs for Alzheimer disease: A 2020–2023 update. J Biomed Sci 30: , 83. |
[60] | Monllau A , Pena-Casanova J , Blesa R , Aguilar M , Bohm P , Sol JM , Hernandez G ((2007) ) Diagnostic value and functional correlations of the ADAS-Cog scale in Alzheimer’s disease: Data on NORMACODEM project. Neurologica 22: , 493–501. |
[61] | Ben Jemaa S , Attia Romdhane N , Bahri-Mrabet A , Jendli A , Le Gall D , Bellaj T ((2017) ) An Arabic version of the cognitive subscale of the Alzheimer’s Disease Assessment Scale (ADAS-cog): Reliability, validity, and normative data. J Alzheimers Dis 60: , 11–21. |
[62] | Graham DP , Cully JA , Snow AL , Massman P , Doody R ((2004) ) The Alzheimer’s Disease Assessment Scale-Cognitive subscale: Normative data for older adult controls. Alzheimer Dis Assoc Disord 18: , 236–240. |
[63] | Strauss E , Sherman EM , Spreen O ((2006) ), A compendium of neuropsychological tests: Administration, norms, and commentary. American Chemical Society. |
[64] | Kueper JK , Speechley M , Montero-Odasso M ((2018) ) The Alzheimer’s disease assessment scale-cognitive subscale (ADAS-Cog): Modifcations and responsiveness in pre-dementia populations. A narrative review. J Alzheimers Dis 63: , 423–444. |