Association of Serum Liver Enzymes with Brain Amyloidopathy and Cognitive Performance
Abstract
Background:
Alzheimer’s disease (AD) is characterized by amyloid-β (Aβ) plaque accumulation and neurofibrillary tangles in the brain. Emerging evidence has suggested potential interactions between the brain and periphery, particularly the liver, in regulating Aβ homeostasis.
Objective:
This study aimed to investigate the association of serum liver enzymes with brain amyloidopathy and cognitive performance in patients with complaints of cognitive decline.
Methods:
A total of 1,036 patients (mean age 74 years, 66.2% female) with subjective cognitive decline, mild cognitive impairment, AD dementia, and other neurodegenerative diseases were included using the Smart Clinical Data Warehouse. Amyloid positron emission tomography (PET) imaging, comprehensive neuropsychological evaluations, and measurements of liver enzymes, including aspartate aminotransferase (AST), alanine aminotransferase (ALT), alkaline phosphatase, total bilirubin, and albumin, were assessed. After propensity score matching, logistic and linear regression analyses were used to investigate the associations between liver enzymes, amyloid status, and cognitive performance. Additionally, a machine learning approach was used to assess the classification performance of liver enzymes in predicting amyloid PET positivity.
Results:
Lower ALT levels and higher AST-to-ALT ratios were significantly associated with amyloid PET positivity and AD diagnosis. The AST-to-ALT ratio was also significantly associated with poor memory function. Machine learning analysis revealed that the classification performance of amyloid status (AUC = 0.642) for age, sex, and apolipoprotein E ɛ4 carrier status significantly improved by 6.2% by integrating the AST-to-ALT ratio.
Conclusions:
These findings highlight the potential association of liver function on AD and its potential as a diagnostic and therapeutic implications.
INTRODUCTION
Alzheimer’s disease (AD) is the predominant cause of dementia, accounting for a substantial proportion of dementia cases worldwide [1]. Pathologically, AD is characterized by the extracellular accumulation of amyloid-β (Aβ) in the form of diffuse and neuritic plaques, as well as the presence of intracellular neurofibrillary tangles comprised of aggregated hyperphosphorylated tau protein in the brain [2]. Although the etiology of AD remains complex and multifactorial, recent evidence has shed light on the potential interactions between the brain and peripheral organs, particularly the liver, in regulating Aβ homeostasis [3–6].
The term of liver function tests is commonly used to refer to a combination of liver biochemical tests, including aminotransferases and alkaline phosphatase (ALP) for markers of hepatocellular injury, total bilirubin for tests of liver metabolism, and albumin for tests of liver synthetic function [7]. Intriguingly, investigations on the potential link between liver enzymes and AD have yielded notable findings. Nho et al. reported a significant association between a higher aspartate aminotransferase (AST) to alanine aminotransferase (ALT) ratio and lower blood ALT levels with AD diagnosis, poor cognitive performance, and increased Aβ deposition [8]. Likewise, other studies have found that lower AST and ALT levels, along with a higher AST to ALT ratio were associated with AD diagnosis and poor cognitive performance [9–12]. However, despite these intriguing associations, there remains a lack of consensus among studies on the precise relationship between blood AST and ALT levels and AD, as evidenced by Kamada et al., who reported significant negative correlations between plasma AST and ALT levels and cognitive decline [13]. Additionally, the potential association of blood ALT levels and the AST-to-ALT ratio with Aβ, a biomarker for AD, remains to be fullyelucidated.
Given the divergence in findings and the crucial need for comprehensive investigations, our study aimed to investigate the potential significant association of serum liver enzymes, including AST, ALT, AST-to-ALT ratio, ALP, total bilirubin, and albumin, with brain amyloidopathy and cognitive performance using the Smart Clinical Data Warehouse (CDW). Moreover, we employed a machine learning approach to assess the performance of these liver enzymes in the classification of brain amyloidopathy.
MATERIALS AND METHODS
Study subjects
We retrospectively investigated clinical data using the clinical big data analytic solution Smart CDW from the Hallym Sacred Heart Hospital, Dongtan Sacred Heart Hospital, Kangnam Sacred Heart Hospital, and Chuncheon Sacred Heart Hospital between November 2015 and June 2023. Smart CDW is based on the QlikView Elite Solution (Qlik, King of Prussia, PA, USA), and offers electronic medical record text data analysis and an integrated analysis of fixed data. Thus, using Smart CDW, we collected the clinical data of patients aged 50 years and older who visited because of complaints of cognitive decline. All participants underwent comprehensive evaluations, including physical and neurological examinations, laboratory tests, cognitive performance assessment, brain magnetic resonance imaging, and amyloid positron emission tomography (PET) imaging. Participants who had not undergone liver function tests before and after one year of the amyloid PET imaging date were excluded from the study. Additionally, individuals with other medical conditions, such as hepatocellular carcinoma and liver cirrhosis, which may significantly impair liver function, were excluded. Patients with outliers in liver function parameters exceeding three times the interquartile range were excluded from the study. Participants who had not undergone neuropsychological assessments before and after one year of the amyloid PET imaging date were further excluded from the study when investigating the association between liver enzymes and cognitive performance.
The study included a total of 288 patients with probable AD dementia [14], 514 with mild cognitive impairment (MCI) based on the National Institute on Aging–Alzheimer’s Association criteria [15], 77 with subjective cognitive decline (SCD) according to the guideline established by Jessen et al. [16], and 157 with other neurodegenerative diseases (OND) including frontotemporal dementia [17, 18], corticobasal syndrome [19], Parkinson’s disease dementia [20], progressive nuclear palsy [21], and individuals with postponed diagnoses.
The protocol for the study has been approved by the Clinical Research Ethics Committee of Chuncheon Sacred Heart Hospital, Hallym University (IRB No. 2023-07-009) and conforms to the provisions of the Declaration of Helsinki.
Amyloid status
The amyloid status was assessed using amyloid PET imaging with [18F] florbetaben (n = 777) and [18F] flutemetamol (n = 259) ligands. The amyloid PET status was categorized as positive (abnormal) or negative (normal) based on visual ratings by one nuclear medicine physician and one neurologist, both experienced and trained in the field. The raters were blinded to the participants’ clinical information but were made aware of the PET tracer used for each image. In case of discordance, the raters discussed the results and reached a consensus.
Measurements of serum liver enzymes
Venous blood samples were obtained from all participants after a 12-h overnight fast to measure serum AST (normal range < 40 U/L [22]), ALT (normal range < 40 U/L [22]), total bilirubin (normal range 0.2–1.0 mg/dL [23]), ALP (normal range 30–115 U/L [24]), and albumin (normal range 3.5–5.0 g/dL [25]) levels. The blood samples were analyzed using a blood auto-analyzer (Beckman Coulter AU5800, Beckman Coulter Inc., Brea CA, USA) at the Department of Laboratory Medicine in each participating hospital (Hallym Sacred Heart Hospital, Dongtan Sacred Heart Hospital, Kangnam Sacred Heart Hospital, and Chuncheon Sacred Heart Hospital).
Comprehensive neuropsychological assessment
Comprehensive neuropsychological assessments were conducted to evaluate four cognitive domains: language, visuospatial, memory, and executive functions. Raw scores on individual cognitive tests were converted to standardized z-scores and adjusted for age, sex, and educational norms [26]. The z-scores for each cognitive domain were then calculated from the individual test z-scores as follows: the Korean Boston Naming Test [27] for language function, Seoul Verbal Learning Test (20-min delayed recall test) for memory function, Rey Complex Figure Test [28] for visuospatial function, and mean z-scores from the Controlled Oral Word Association Test [29] and Korean Color–Word Stroop test [30] for executive function.
Statistical analysis
We compared the baseline characteristics of the amyloid-negative and amyloid-positive groups using chi-square tests for categorical variables and Mann–Whitney U tests for continuous variables, as appropriate.
We conducted a propensity score matching (PSM) analysis with aim to balance potential confounding factors, such as age, sex, and apolipoprotein E (APOE) ɛ4 carrier status, between the amyloid-negative and amyloid positive groups. The PSM analysis can reduce selection bias and enhance the robustness of our analysis based on the propensity score for each case [31]. Using the propensity scores, we matched the amyloid-negative and amyloid-positive groups in a 1 : 1 ratio through the nearest neighbor method [31]. The R package MatchIt was used for the PSM analysis [31].
Logistic regression analysis was performed to evaluate the association of each liver enzyme with amyloid PET positivity and AD diagnostic status. Linear regression analysis was used to investigate the association between liver enzyme levels and cognitive performance. Covariates in the logistic regression models were included the following: prevalence of hypertension, diabetes mellitus, dyslipidemia, and statin use. Covariates in the linear regression models were included the following: years of education and prevalence of hypertension, diabetes mellitus, dyslipidemia, and statin use. Education was assessed as the total number of years of formal education completed by each participant.
To investigate the classification performance of liver enzymes in differentiating amyloid status, we used a machine learning approach with penalized logistic regression using the STREAMLINE tool [32]. This approach proved advantageous in reducing the effect of multicollinearity on feature selection [33]. The data were randomly divided into 70% for training and 30% for testing the model using a 30-fold cross-validation procedure. We evaluated the classification performance of six different models using the receiver operating characteristic (ROC) curve and the area under the ROC curve (AUC). Training features in Model 1 were age, sex, and APOE ɛ4 carrier status; in Model 2 were ALT levels; in Model 3 were AST-to-ALT ratio; in Model 4 were age, sex, APOE ɛ4 carrier status, and ALT levels; and in Model 5 were age, sex, APOE ɛ4 carrier status, and AST-to-ALT ratio. Paired t-tests [34] were used to compare the AUC of the five models.
All statistical analyses were performed using R version 4.2.0, and statistical significance was set at a p-value of 0.05 after adjusting for multiple comparisons. For multiple testing adjustments, a false discovery rate correction with the Benjamini–Hochberg procedure [35] was used. The workflow of all analysis steps used in this study is illustrated in Fig. 1.
Fig. 1
Schematic overview of the workflow in our study. LFTs, liver function tests; PET, positron emission tomography; PSM, propensity score matching.
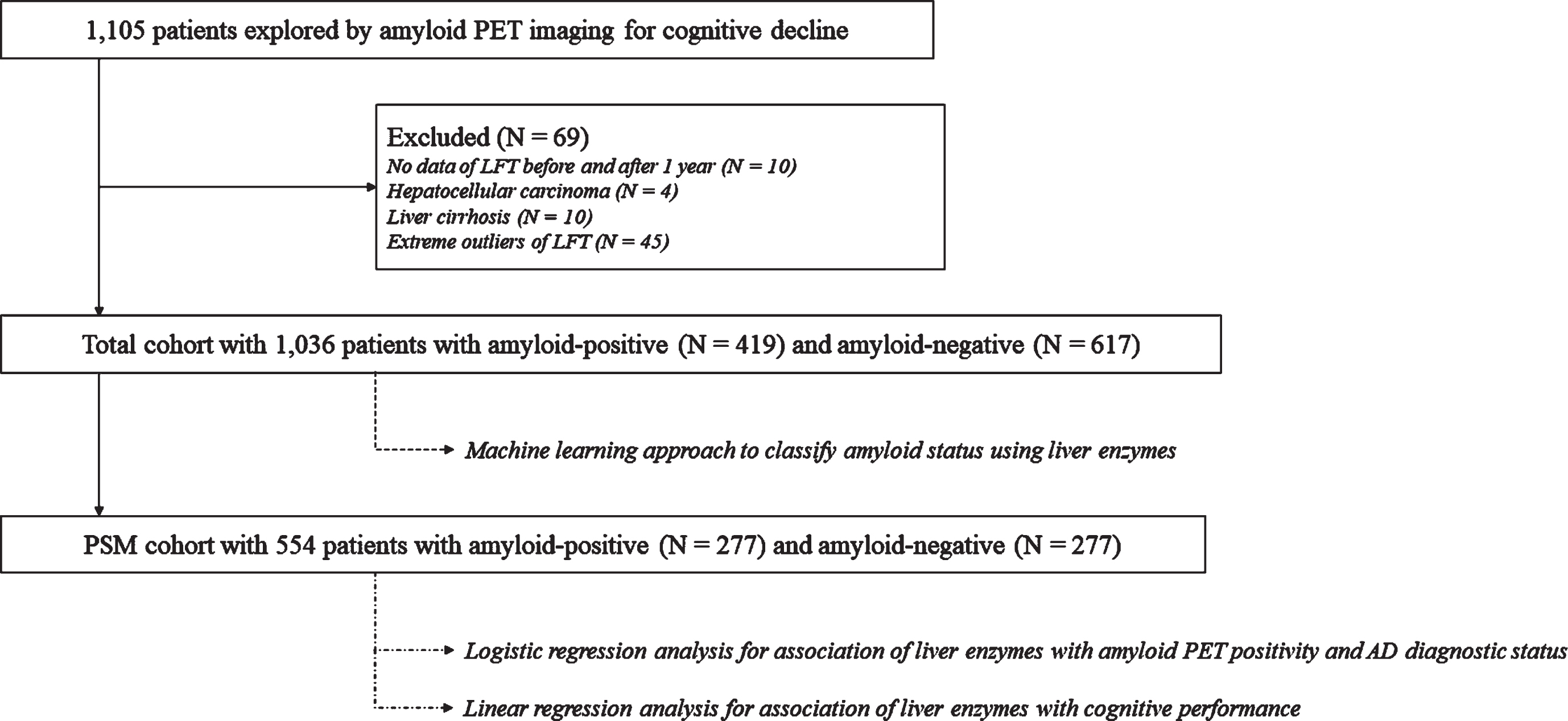
RESULTS
Demographics and clinical characteristics
A total of 1,036 participants with a median age of 74 years were included in the total cohort. Of them, 686 (66.2%) were female. The cohort comprised 419 amyloid-positive (40.4%) and 617 amyloid-negative patients. After PSM, 277 patients in the amyloid-negative group were matched 1 : 1 with patients in the amyloid-positive group. The amyloid-negative group in the PSM cohort included a total of 30 patients with probable AD dementia and 161 with MCI, 20 with SCD, and 66 with OND, while the amyloid-positive group in the PSM cohort included a total of 158 patients with probable AD dementia and 99 with MCI, 17 with SCD, and 3 with OND. The PSM cohort had no significant differences in age, sex, years of education, prevalence of hypertension, diabetes mellitus, dyslipidemia, statin use, or APOE ɛ4 carrier status between the two groups. However, the amyloid-positive group showed lower Mini-Mental State Examination scores, indicative of poor general cognitive performance. Table 1 compares the baseline characteristics of the studyparticipants.
Table 1
Demographic and clinical characteristics, and laboratory results between amyloid-negative and amyloid-positive groups in total and PSM cohorts
Total cohort | PSM cohort | |||||
Amyloid-negative | Amyloid-positive | pa | Amyloid-negative | Amyloid-positive | pa | |
(n = 617) | (n = 419) | (n = 277) | (n = 277) | |||
Female | 401 (65.0) | 285 (68.0) | 0.345 | 198 (71.5) | 190 (68.6) | 0.458 |
Age, median (IQR), y | 74.0 (66.0–80.0) | 74.0 (67.0–79.0) | 0.411 | 74.0 (66.0–80.0) | 75.0 (67.0–80.0) | 0.158 |
Education, median (IQR), y | 9.0 (6.0–12.0) | 9.0 (6.0–12.0) | 0.020 | 8.0 (4.0–12.0) | 9.0 (6.0–12.0) | 0.060 |
Hypertension | 160 (25.9) | 90 (21.5) | 0.117 | 66 (23.8) | 62 (22.4) | 0.687 |
Diabetes mellitus | 123 (19.9) | 51 (12.2) | 0.001 | 45 (16.2) | 39 (14.1) | 0.477 |
Dyslipidemia | 107 (17.3) | 68 (16.2) | 0.700 | 48 (17.3) | 45 (16.2) | 0.733 |
Statin use | 150 (24.3) | 93 (22.2) | 0.475 | 60 (21.7) | 64 (23.1) | 0.684 |
Diagnosis | ||||||
SCD/MCI/ADD/ONDb | 49/357/60/151 | 28/157/228/6 | 20/161/30/66 | 17/99/158/3 | ||
APOE ɛ4 carrier | 98 (19.0) | 183 (50.3) | <0.001c | 96 (34.7) | 96 (34.7) | 1.000 |
MMSE, median (IQR) | 24.0 (19.0–27.0) | 22.0 (18.0–25.0) | <0.001 | 24.0 (20.0–26.0) | 22.0 (18.0–25.0) | <0.001 |
AST, median (IQR), U/L | 21.0 (18.0–26.0) | 22.0 (19.0–27.0) | 0.006 | 22.0 (18.0–26.0) | 22.0 (19.0–27.0) | 0.210 |
ALT, median (IQR), U/L | 17.0 (12.0–23.0) | 15.0 (11.5–20.0) | 0.006 | 17.0 (12.0–22.0) | 15.0 (11.0–20.0) | 0.014 |
AST to ALT ratio, median (IQR) | 1.29 (1.00–1.61) | 1.48 (1.22–1.79) | <0.001 | 1.31 (1.08–1.58) | 1.50 (1.24–1.80) | <0.001 |
ALP, median (IQR), U/L | 73.0 (59.0–86.0) | 69.0 (57.0–83.0) | 0.007 | 75.0 (61.0–87.0) | 71.0 (58.0–85.0) | 0.046 |
Total bilirubin, median (IQR), mg/dL | 0.50 (0.40–0.66) | 0.50 (0.39–0.68) | 0.712 | 0.50 (0.40–0.67) | 0.50 (0.40–0.64) | 0.793 |
Albumin, median (IQR), g/dL | 4.40 (4.20–4.60) | 4.40 (4.10–4.60) | 0.128 | 4.40 (4.20–4.60) | 4.40 (4.20–4.60) | 0.099 |
Values are n (%), unless indicated otherwise. aThe Mann-Whitney U test or chi-square test was used to determine the P value for comparisons between amyloid-negative and amyloid-positive groups, as appropriate. bOND includes FTD, CBS, PDD, PSP, and individuals with postponed diagnosis. cThere were 100 missing values among 617 amyloid-negative subjects and 55 missing values among 419 amyloid-positive subjects. AD, Alzheimer’s disease; ALT, alanine aminotransferase; ALP, alkaline phosphatase; APOE, apolipoprotein E; AST, aspartate aminotransferase; CBS, corticobasal syndrome; FTD, frontotemporal dementia; IQR, interquartile range; MCI, mild cognitive impairment; MMSE, Mini-Mental State Examination; OND, other neurodegenerative disease; PDD, Parkinson’s disease dementia; PSM, propensity score matching; PSP, progressive supranuclear palsy; SCD, subjective cognitive decline.
Association analysis of liver enzymes with amyloid PET positivity, AD diagnostic status, and cognition
In the PSM cohort, ALT levels (ALT; odds ratio (OR), 0.974; 95% confidence interval (CI), 0.953–0.994; p = 0.043) were significantly lower in the amyloid-positive group than in the amyloid-negative group, whereas the AST-to-ALT ratio (AST-to-ALT ratio; OR, 1.564; 95% CI, 1.162–2.162; p = 0.026) was significantly higher in the amyloid-positive group than in the amyloid-negative group after adjusting for prevalence of hypertension, diabetes mellitus, dyslipidemia, and statin use (Table 2). Regarding diagnostic status, ALT levels (ALT; OR, 0.942; 95% CI, 0.906–0.977; p = 0.009) were significantly lower, while the AST-to-ALT ratio (AST-to-ALT ratio; OR, 2.539; 95% CI, 1.253–5.546; p = 0.028) was significantly higher in AD compared with SCD in the PSM cohort. However, no significant associations were found between amyloid PET positivity, AD diagnosis, and levels of AST, ALP, total bilirubin, or albumin.
Table 2
Results of the association analysis of liver enzymes with amyloid PET positivity and AD diagnostic status in the PSM cohort
Amyloid PET positivity | AD diagnostic status | |||||
OR | 95% CI | pa | OR | 95% CI | pa | |
AST, U/L | 1.011 | 0.986–1.038 | 0.458 | 0.992 | 0.942–1.048 | 0.779 |
ALT, U/L | 0.974 | 0.953–0.994 | 0.043 | 0.942 | 0.906–0.977 | 0.009 |
AST-to-ALT ratio | 1.564 | 1.162–2.162 | 0.029 | 2.539 | 1.253–5.546 | 0.028 |
ALP, U/L | 0.992 | 0.985–1.000 | 0.134 | 1.013 | 0.995–1.032 | 0.278 |
Total bilirubin, mg/dL | 1.217 | 0.594–2.508 | 0.592 | 1.798 | 0.363–10.412 | 0.589 |
Albumin, g/dL | 0.726 | 0.445–1.177 | 0.293 | 0.275 | 0.083–0.860 | 0.060 |
All analyses were adjusted for prevalence of hypertension, diabetes mellitus, dyslipidemia, and statin use. aAdjusted p value for FDR. AD, Alzheimer’s disease; ALP, alkaline phosphatase; ALT, alanine aminotransferase; AST, aspartate aminotransferase; CI, confidence interval; FDR, false discovery rate; OR, odds ratio; PSM, propensity score matching.
In terms of cognitive performance, a higher AST-to-ALT ratio (β [standard error (SE)], –0.190 [0.020]; p = 0.02) was significantly associated with poor memory function after adjusting for years of education and prevalence of hypertension, diabetes mellitus, dyslipidemia, and statin use in the PSM cohort (Table 3). However, other liver enzymes were not significantly associated with cognitive performance.
Table 3
Results of the association analysis of liver enzymes with cognition in the PSM cohort
Language | Visuospatial | Memory | Executive | |||||
β (SE) | pa | β (SE) | pa | β (SE) | pa | β (SE) | pa | |
AST, U/L | –0.005 (0.012) | 0.66 | 0.014 (0.018) | 0.44 | –0.004 (0.009) | 0.63 | –0.002 (0.008) | 0.81 |
ALT, U/L | –0.004 (0.010) | 0.67 | 0.014 (0.015) | 0.34 | 0.011 (0.007) | 0.10 | 0.002 (0.007) | 0.80 |
AST-to-ALT ratio | 0.023 (0.126) | 0.86 | –0.072 (0.189) | 0.70 | –0.190 (0.020) | 0.02 | –0.045 (0.083) | 0.58 |
ALP, U/L | –0.003 (0.004) | 0.50 | –0.003 (0.006) | 0.66 | –0.002 (0.003) | 0.50 | 0.002 (0.002) | 0.53 |
Total bilirubin, mg/dL | 0.304 (0.343) | 0.38 | –0.080 (0.512) | 0.88 | –0.260 (0.238) | 0.27 | –0.232 (0.223) | 0.30 |
Albumin, g/dL | –0.052 (0.235) | 0.83 | 0.496 (0.354) | 0.16 | 0.311 (0.168) | 0.06 | 0.110 (0.154) | 0.48 |
All analyses were adjusted for years of education and prevalence of hypertension, diabetes mellitus, dyslipidemia, and statin use. aAdjusted p value for FDR. ALP, alkaline phosphatase; ALT, alanine aminotransferase; APOE, apolipoprotein E; AST, aspartate aminotransferase; FDR, false discovery rate; SE, standard error.
We performed a sensitivity analysis to evaluate the effect of OND on our findings and observed similar or slightly more significant results (Supplementary Tables 1 and 2). Notably, among the six liver function markers, the AST-to-ALT ratio showed a significant correlation with amyloid PET positivity and AD diagnosis, as well as a negative association with memory function.
Machine learning analysis for amyloid PET positivity
A machine learning approach using penalized logistic regression was employed to classify the amyloid status, and six different classification models were evaluated (Table 4). The results of the 30-fold cross-validation are presented in Fig. 2. Model 1 achieved a mean AUC value of 0.642 with a standard deviation (SD) of 0.087 (Fig. 2A). Model 5 (mean AUC value of 0.704 with SD = 0.082), obtained by adding the AST-to-ALT ratio to Model 1, demonstrated a significantly improved classification performance compared with Model 1 (p < 0.001) (Fig. 2E), which was comparable with that of Model 6, obtained by adding all six liver function markers to Model 1 (Fig. 2F). The mean AUC values of Model 2, including only ALT levels; Model 3, including only the AST-to-ALT ratio; and Model 4, obtained by adding ALT levels to Model 1, were 0.533, 0.611, and 0.650, respectively, with SDs of 0.106, 0.107, and 0.090, respectively (Fig. 2B–D).
Table 4
Mean AUC and standard deviation of machine learning models using penalized logistic regression
Model | Training features | Mean AUC | Standard deviation of AUC | pa |
1 | Age + Sex + APOE ɛ4 carrier status | 0.642 | 0.087 | |
2 | ALT levels | 0.533 | 0.106 | <0.001 |
3 | AST-to-ALT ratio | 0.611 | 0.107 | 0.201 |
4 | Age + Sex + APOE ɛ4 carrier status + ALT levels | 0.650 | 0.090 | 0.459 |
5 | Age + Sex + APOE ɛ4 carrier status + AST-to-ALT ratio | 0.704 | 0.082 | <0.001 |
6 | Age + Sex + APOE ɛ4 carrier status + All six liver function markersb | 0.702 | 0.107 | 0.019 |
30-fold cross validation was used to investigate and compare the classification performance of six different machine learning models for differentiating amyloid status. The machine learning model, mean AUC, and standard deviation of the AUC are presented. ap value for the paired t-test to compare with the AUC measured in model 1. bSix liver function markers include the levels of AST, ALT, ALP, total bilirubin, and albumin, and the ratio of AST-to-ALT. ALP, alkaline phosphatase; ALT, alanine aminotransferase; APOE, apolipoprotein E; AST, aspartate aminotransferase; AUC, area under the curve; ROC, receiver operating characteristic.
Fig. 2
The ROC curves and mean AUC of machine learning approach using penalized logistic regression. Sensitivity is on the y-axis and 1-specificity is on the x-axis. 30-fold cross validation was used to investigate and compare the classification performance of six different classification models for differentiating amyloid status. The gray zone around the mean ROC curve represents±1 standard deviation. Six different classification models are shown per following training features. (A) training features include age, sex, and APOE ɛ4 carrier status (B) training features include ALT levels (C) training features include the AST-to-ALT ratio (D) training features include age, sex, APOE ɛ4 carrier status and ALT levels (E) training features include age, sex, APOE ɛ4 carrier status, and the AST-to-ALT ratio. (F) training features include age, sex, APOE ɛ4 carrier status, and all six liver function markers including the levels of AST, ALT, ALP, total bilirubin, and albumin, and the ratio of AST-to-ALT. ALP, alkaline phosphatase; ALT, alanine aminotransferase; APOE, apolipoprotein E; AST, aspartate aminotransferase; AUC, area under the curve; ROC, receiver operating characteristic.
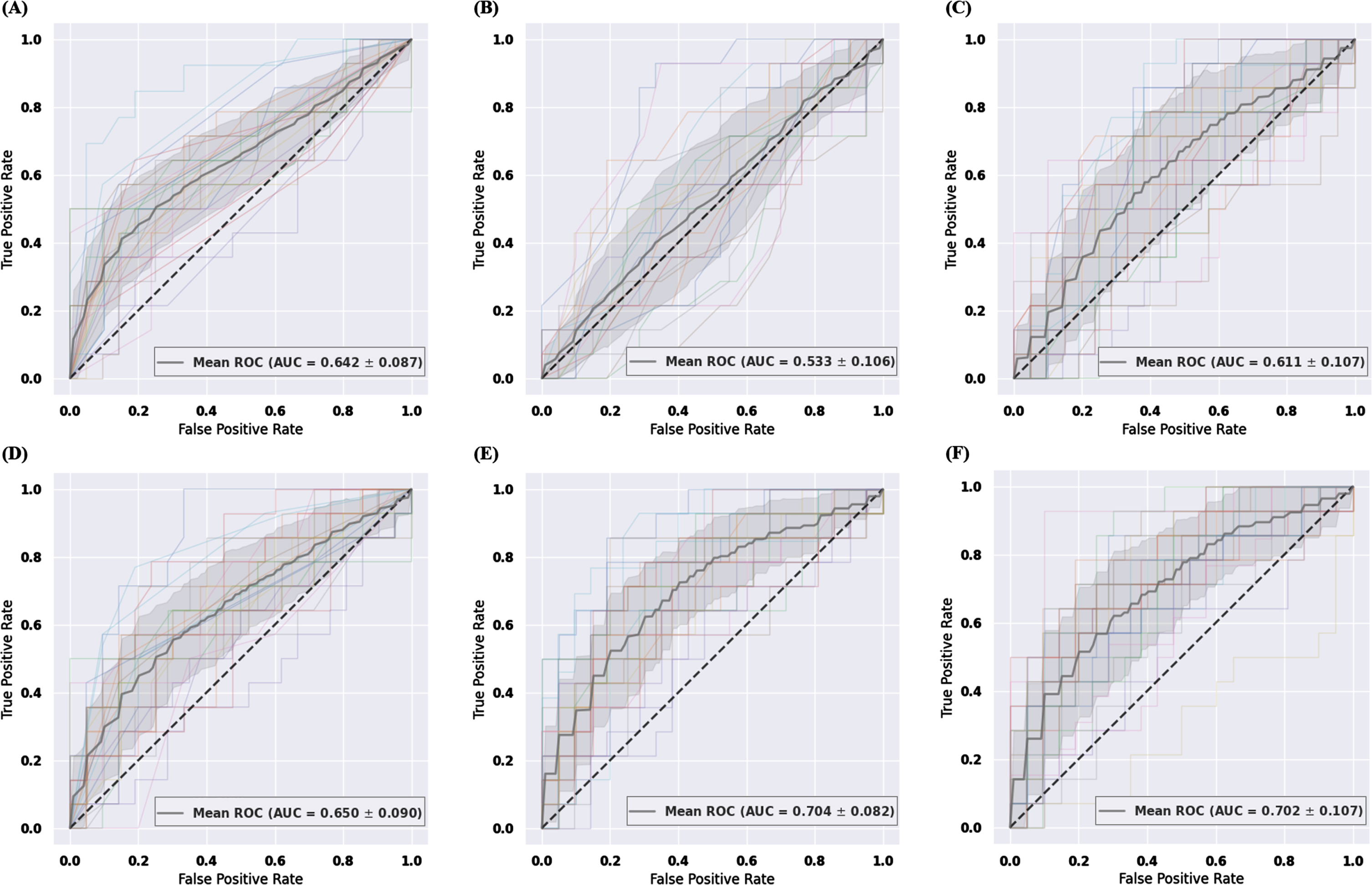
DISCUSSION
In this study, we investigated the association between liver enzymes and amyloid status in a heterogeneous population of individuals with AD, MCI, SCD, and OND, using Smart CDW. Our findings revealed that ALT levels and the AST-to-ALT ratio were significantly associated with amyloid PET positivity and AD diagnosis, with the AST-to-ALT ratio being the strongest and associated with amyloid PET positivity, AD diagnosis, and memory function, suggesting potential associations of liver function in the pathogenesis of AD. Additionally, machine learning analysis demonstrated that the integration of liver enzymes, particularly the AST-to-ALT ratio, with age, sex, and APOE ɛ4 carrier status significantly improved the classification performance of amyloid status by 6.2% compared with using age, sex, and APOE ɛ4 carrier status alone (AUC = 0.642).
While an increase in serum AST and ALT levels often holds clinical significance as hepatocyte membrane damage can release these enzymes into the bloodstream [7], previous studies have reported that lower AST and ALT levels within their normal ranges and a higher AST-to-ALT ratio were associated with AD diagnosis, poor cognitive performance, and increased Aβ deposition [8–12]. Our results are generally consistent with these earlier findings. However, it is important to note that the association with amyloid status in the brain was evident for ALT levels and the AST-to-ALT ratio, but not for AST levels. This observation aligns with the notion that ALT, which is primarily hepatic in origin, serves as a more specific marker of liver function than AST [7, 36], suggesting an underlying relationship between liver function and brain amyloidosis. However, the significance of these results requires further investigation.
Hepatic aging is a possible explanation for the association between low ALT levels and brain amyloidopathy. Hepatic aging is characterized by a reduced liver size, altered blood flow, and histological changes, presumably due to chronic oxidative stress [36, 37]. Consequently, greater hepatic cell apoptosis caused by chronic oxidative stress may lead to a decrease in the total number of hepatocytes, which in turn reduces the production and release of ALT [36, 37]. This reduction in hepatocyte number could impact the hepatic clearance of Aβ from plasma because these processes are partially mediated by low-density lipoprotein receptor-related protein (LRP-1) present on the plasma membrane in hepatocytes [38]. Impaired hepatic Aβ degradation may potentially contribute to increased Aβ accumulation in the brain [38, 39]. However, the precise role of reduced ALT levels in AD pathogenesis remains unclear. Subsequent investigations could explore this potential influence and elucidate the complex interplay. In particular, serum AST-to-ALT ratio is commonly used in the clinic as a marker of liver fibrosis in non-alcoholic fatty liver disease [7, 40]. Liver fibrosis is associated with early AD pathology, poor cognition, and lower hippocampal and total brain volumes [41, 42].
The improved classification performance achieved by integrating liver enzymes with clinical information is a novel finding that has not been reported in previous studies, and provides intriguing insights into potential therapeutic targets for AD. For instance, silymarin, a hepatoprotective agent extracted from the seeds of Silybum marianum, has shown promise as a therapeutic intervention for AD because of its inhibitory effects on Aβ fibril and oligomer formation as well as its positive impact on cholinergic energy, oxidative stress protection, and neuroinflammation inhibition [43–45]. Additionally, decreased levels of transthyretin (TTR), a transporter protein primarily synthesized in the liver and secreted into the blood, observed in AD, might have implications for AD pathogenesis [46]. TTR, with its crucial role in reducing brain and plasma Aβ by binding to Aβ peptide in the plasma and increasing Aβ internalization in hepatocyte cells, might provide relevant information for the development of therapeutic strategies for AD [46, 47]. Lastly, lifestyle modification such as a Mediterranean diet, physical activity and exercise can serve as a primary therapeutic approach for AD by effectively managing non-alcoholic fatty liver diseases [48].
Despite these intriguing findings, our study has several limitations. Firstly, we were unable to analyze the association between liver enzymes and quantitative measures of amyloid PET because of the lack of imaging data, which limited the depth of our analysis. Nevertheless, the use of the visual rating of amyloid PET as a routine reading process remains valuable in clinical practice. Secondly, the cross-sectional design of our study may have introduced an inherent bias. Therefore, well-designed prospective studies should be conducted to deepen the understanding of the roles of liver enzymes in AD pathogenesis. Thirdly, the broad normal ranges for AST and ALT, varying by sex and instruments, may introduce variability. Fourthly, although liver function tests complement amyloid PET imaging, they should not be considered as stand-alone measures. Fifthly, serum Aβ tests were not performed in this study. To recognize the importance of investigating correlations with liver function tests, future studies should consider incorporating serum Aβ tests to provide a more comprehensive understanding of the observed associations. Lastly, the lack of data on body mass index, an important covariate associated with ALT levels [36, 37], and history of cerebrovascular disease or stroke might have influenced our results.
Conclusions
In summary, our study provides evidence for the association of lower ALT levels and a higher AST-to-ALT ratio with amyloid PET positivity and AD. Furthermore, the integration of liver enzymes, particularly the AST-to-ALT ratio, with clinical information significantly improved the classification performance of amyloid status in the brain. These findings shed light on potential therapeutic targets for AD; however, further investigations are necessary to unravel the underlying mechanisms and validate the significance of these findings in larger and well-controlled studies.
ACKNOWLEDGMENTS
The authors have no acknowledgments to report.
FUNDING
This research was supported by the Bio&Medical Technology Development Program of the National Research Foundation (NRF) funded by the Korean government (MSIT) (No. RS-2023-00223501) and Hallym University Medical Center Research Fund.
CONFLICT OF INTEREST
The authors have no conflict of interest to report.
DATA AVAILABILITY
The datasets used and/or analyzed during the current study are available from the corresponding author on reasonable request.
SUPPLEMENTARY MATERIAL
[1] The supplementary material is available in the electronic version of this article: https://dx.doi.org/10.3233/ADR-230148.
REFERENCES
[1] | Gaugler J , James B , Johnson T , Reimer J , Solis M , Weuve J , Buckley RF , Hohman TJ ((2022) ) 2022 Alzheimer’s disease facts and figures. Alzheimers Dement 18: , 700–789. |
[2] | Long JM , Holtzman DM ((2019) ) Alzheimer disease: an update on pathobiology and treatment strategies. Cell 179: , 312–339. |
[3] | Bassendine MF , Taylor-Robinson SD , Fertleman M , Khan M , Neely D ((2020) ) Is Alzheimer’s disease a liver disease of the brain? J Alzheimers Dis 75: , 1–14. |
[4] | Estrada LD , Ahumada P , Cabrera D , Arab JP ((2019) ) Liver dysfunction as a novel player in Alzheimer’s progression: looking outside the brain. Front Aging Neurosci 11: , 174. |
[5] | Cheng Y , Tian D-Y , Wang Y-J ((2020) ) Peripheral clearance of brain-derived Aβ in Alzheimer’s disease: pathophysiology and therapeutic perspectives. Transl Neurodegener 9: , 16. |
[6] | Huang Z , Lin HW , Zhang Q , Zong X ((2022) ) Targeting Alzheimer’s disease: the critical crosstalk between the liver and brain. Nutrients 14: , 4298. |
[7] | Woreta TA , Alqahtani SA ((2014) ) Evaluation of abnormal liver tests. Med Clin North Am 98: , 1–16. |
[8] | Nho K , Kueider-Paisley A , Ahmad S , MahmoudianDehkordi S , Arnold M , Risacher SL , Louie G , Blach C , Baillie R , Han X ((2019) ) Association of altered liver enzymes with Alzheimer disease diagnosis, cognition, neuroimaging measures, and cerebrospinal fluid biomarkers. JAMA Netw Open 2: , e197978. |
[9] | Han S-W , Park YH , Jang ES , Nho K , Kim S ((2022) ) Implications of liver enzymes in the pathogenesis of Alzheimer’s disease. J Alzheimers Dis 88: , 1371–1376. |
[10] | Li W , Yue L , Sun L , Xiao S ((2022) ) An increased aspartate to alanine aminotransferase ratio is associated with a higher risk of cognitive impairment. Front Med 9: , 780174. |
[11] | Lu Y , Pike JR , Selvin E , Mosley T , Palta P , Sharrett AR , Thomas A , Loehr L , Sidney Barritt A , Hoogeveen RC ((2021) ) Low liver enzymes and risk of dementia: the atherosclerosis risk in communities (ARIC) study. J Alzheimers Dis 79: , 1775–1784. |
[12] | Ferri E , Rossi PD , Scichilone M , Lucchi TA , Arosio B ((2022) ) Liver enzymes in a cohort of community-dwelling olderpersons: focus on sex contribution. Nutrients 14: , 4973. |
[13] | Kamada Y , Hashimoto R , Yamamori H , Yasuda Y , Takehara T , Fujita Y , Hashimoto K , Miyoshi E ((2016) ) Impact of plasma transaminase levels on the peripheral blood glutamate levels and memory functions in healthy subjects. BBA Clin 5: , 101–107. |
[14] | McKhann GM , Knopman DS , Chertkow H , Hyman BT , Jack CR Jr , Kawas CH , Klunk WE , Koroshetz WJ , Manly JJ , Mayeux R ((2011) ) The diagnosis of dementia due to Alzheimer’s disease: Recommendations from the National Institute on Aging-Alzheimer’s Association workgroups on diagnostic guidelines for Alzheimer’s disease. Alzheimers Dement 7: , 263–269. |
[15] | Albert MS , DeKosky ST , Dickson D , Dubois B , Feldman HH , Fox NC , Gamst A , Holtzman DM , Jagust WJ , Petersen RC ((2011) ) The diagnosis of mild cognitive impairment due to Alzheimer’s disease: recommendations from the National Institute on Aging-Alzheimer’s Association workgroups on diagnostic guidelines for Alzheimer’s disease. Alzheimers Dement 7: , 270–279. |
[16] | Jessen F , Amariglio RE , Van Boxtel M , Breteler M , Ceccaldi M , Chételat G , Dubois B , Dufouil C , Ellis KA , Van Der Flier WM ((2014) ) A conceptual framework for research on subjective cognitive decline in preclinical Alzheimer’s disease. Alzheimers Dement 10: , 844–852. |
[17] | Rascovsky K , Hodges JR , Knopman D , Mendez MF , Kramer JH , Neuhaus J , Van Swieten JC , Seelaar H , Dopper EG , Onyike CU ((2011) ) Sensitivity of revised diagnostic criteria for the behavioural variant of frontotemporal dementia. Brain 134: , 2456–2477. |
[18] | Gorno-Tempini ML , Hillis AE , Weintraub S , Kertesz A , Mendez M , Cappa SF , Ogar JM , Rohrer JD , Black S , Boeve BF ((2011) ) Classification of primary progressive aphasia and its variants. Neurology 76: , 1006–1014. |
[19] | Armstrong MJ , Litvan I , Lang AE , Bak TH , Bhatia KP , Borroni B , Boxer AL , Dickson DW , Grossman M , Hallett M ((2013) ) Criteria for the diagnosis of corticobasal degeneration. Neurology 80: , 496–503. |
[20] | Dubois B , Burn D , Goetz C , Aarsland D , Brown RG , Broe GA , Dickson D , Duyckaerts C , Cummings J , Gauthier S ((2007) ) Diagnostic procedures for Parkinson’s disease dementia: recommendations from the movement disorder society task force. Mov Disord 22: , 2314–2324. |
[21] | Litvan I , Agid Y , Calne D , Campbell G , Dubois B , Duvoisin R , Goetz C , Golbe LI , Grafman J , Growdon J ((1996) ) Clinical research criteria for the diagnosis of progressive supranuclear palsy (Steele-Richardson-Olszewski syndrome): report of the NINDS-SPSP international workshop. Neurology 47: , 1–9. |
[22] | Neuschwander-Tetri BA , Ünalp A , Creer MH , Network NSCR ((2008) ) Influence of local reference populations on upper limits of normal for serum alanine aminotransferase levels. Arch Intern Med 168: , 663–666. |
[23] | Franchini M , Targher G , Lippi G ((2010) ) Serum bilirubin levels and cardiovascular disease risk: a Janus Bifrons? Adv Clin Chem 50: , 47–63. |
[24] | Lum G ((1995) ) Significance of low serum alkaline phosphatase activity in a predominantly adult male population. Clin Chem 41: , 515–518. |
[25] | Doweiko JP , Nompleggi DJ ((1991) ) Reviews: role of albumin in human physiology and pathophysiology. J Parenter Enteral Nutr 15: , 207–211. |
[26] | Kang Y , Na D , Hahn S ((2003) ) Seoul Neuropsychological Screening Battery. Human Brain Research & Consulting Co., Incheon. |
[27] | Kim H , Na DL ((1999) ) Normative data on the Korean version of the Boston naming test. J Clin Exp Neuropsychol 21: , 127–133. |
[28] | Meyers J , Meyers KR ((1995) ) Rey complex figure test and recognition trial. Psychological Assessment Resources, Incorporated. |
[29] | Kang Y , Chin J-H , Na D , Lee J , Park J ((2000) ) A normative study of the Korean version of Controlled Oral Word Association Test (COWAT) in the elderly. Korean J Clin Psychol 19: , 385–392. |
[30] | Lee J , Kang Y , Na D ((2000) ) Efficiencies of Stroop interference indexes in healthy older adults and dementia patients. Korean J Clin Psychol 19: , 807–818. |
[31] | Randolph JJ , Falbe K ((2014) ) A step-by-step guide to propensity score matching in R. Pract Assess Res Eval 19: , 18. |
[32] | Urbanowicz R , Zhang R , Cui Y , Suri P ((2023) ) STREAMLINE: A simple, transparent, end-to-end automated machine learning pipeline facilitating data analysis and algorithm comparison. In Genetic Programming Theory and Practice XIX, Springer, pp. 201–231. |
[33] | Teipel SJ , Grothe MJ , Metzger CD , Grimmer T , Sorg C , Ewers M , Franzmeier N , Meisenzahl E , Klöppel S , Borchardt V ((2017) ) Robust detection of impaired resting state functional connectivity networks in Alzheimer’s disease using elastic net regularized regression. Front Aging Neurosci 8: , 318. |
[34] | Bouckaert RR , Frank E ((2004) ) Evaluating the replicability of significance tests for comparing learning algorithms. In Advances in Knowledge Discovery and Data Mining. PAKDD 2004. Lecture Notes in Computer Science, vol 3056, Dai H, Srikant R, Zhang C, eds. Springer, Berlin, Heidelberg, pp. 3–12. |
[35] | Benjamini Y , Hochberg Y ((1995) ) Controlling the false discovery rate: a practical and powerful approach to multiple testing. J R Stat Soc B Methodol 57: , 289–300. |
[36] | Ndrepepa G , Kastrati A ((2019) ) Alanine aminotransferase—a marker of cardiovascular risk at high and low activity levels. J Lab Precis Med 4: , 29. |
[37] | Elinav E , Ackerman Z , Maaravi Y , Ben-Dov IZ , Ein-Mor E , Stessman J ((2006) ) Low alanine aminotransferase activity in older people is associated with greater long-term mortality. J Am Geriatr Soc 54: , 1719–1724. |
[38] | Tamaki C , Ohtsuki S , Terasaki T ((2007) ) Insulin facilitates the hepatic clearance of plasma amyloid β-peptide (1–40) by intracellular translocation of low-density lipoprotein receptor-related protein 1 (LRP-1) to the plasma membrane in hepatocytes. Mol Pharmacol 72: , 850–855. |
[39] | Maarouf CL , Walker JE , Sue LI , Dugger BN , Beach TG , Serrano GE ((2018) ) Impaired hepatic amyloid-beta degradation in Alzheimer’s disease. PLoS One 13: , e0203659. |
[40] | Allwright M , Mundell HD , McCorkindale AN , Lindley RI , Austin PJ , Guennewig B , Sutherland GT ((2023) ) Ranking the risk factors for Alzheimer’s disease; findings from the UK Biobank study. Aging Brain 3: , 100081. |
[41] | Parikh NS , Kamel H , Zhang C , Gupta A , Cohen DE , de Leon MJ , Gottesman RF , Iadecola C ((2023) ) Association of liver fibrosis with cognitive test performance and brain imaging parameters in the UK Biobank study. Alzheimers Dement 19: , 1518–1528. |
[42] | Weinstein G , O’Donnell A , Davis-Plourde K , Zelber-Sagi S , Ghosh S , DeCarli CS , Thibault EG , Sperling RA , Johnson KA , Beiser AS ((2022) ) Non-alcoholic fatty liver disease, liver fibrosis, and regional amyloid-β and tau pathology in middle-aged adults: The Framingham study. J Alzheimers Dis 86: , 1371–1383. |
[43] | Haddadi R , Shahidi Z , Eyvari-Brooshghalan S ((2020) ) Silymarin and neurodegenerative diseases: Therapeutic potential and basic molecular mechanisms. Phytomedicine 79: , 153320. |
[44] | Guo H , Cao H , Cui X , Zheng W , Wang S , Yu J , Chen Z ((2019) ) Silymarin’s inhibition and treatment effects for Alzheimer’s disease. Molecules 24: , 1748. |
[45] | Murata N , Murakami K , Ozawa Y , Kinoshita N , Irie K , Shirasawa T , Shimizu T ((2010) ) Silymarin attenuated the amyloid β plaque burden and improved behavioral abnormalities in an Alzheimer’s disease mouse model. Biosci Biotechnol Biochem 74: , 2299–2306. |
[46] | Alemi M , Gaiteiro C , Ribeiro CA , Santos LM , Gomes JR , Oliveira SM , Couraud P-O , Weksler B , Romero I , Saraiva MJ ((2016) ) Transthyretin participates in beta-amyloid transport from the brain to the liver-involvement of the low-density lipoprotein receptor-related protein 1? Sci Rep 6: , 20164. |
[47] | Alemi M , Silva SC , Santana I , Cardoso I ((2017) ) Transthyretin stability is critical in assisting beta amyloid clearance–Relevance of transthyretin stabilization in Alzheimer’s disease. CNS Neurosci Ther 23: , 605–619. |
[48] | Romero-Gómez M , Zelber-Sagi S , Trenell M ((2017) ) Treatment of NAFLD with diet, physical activity and exercise. J Hepatol 67: , 829–846. |