Identification of molecular biomarkers associated with non-small-cell lung carcinoma (NSCLC) using whole-exome sequencing
Abstract
OBJECTIVES:
Significant progress has been made in the treatment of patients with pulmonary adenocarcinoma (ADCA) based on molecular profiling. However, no such molecular target exists for squamous cell carcinoma (SQCC). An exome sequence may provide new markers for personalized medicine for lung cancer patients of all subtypes. The current study aims to discover new genetic markers that can be used as universal biomarkers for non-small cell lung cancer (NSCLC).
METHODS:
WES of 19 advanced NSCLC patients (10 ADCA and 9 SQCC) was performed using Illumina HiSeq 2000. Variant calling was performed using GATK HaplotypeCaller and then the impacts of variants on protein structure or function were predicted using SnpEff and ANNOVAR. The clinical impact of somatic variants in cancer was assessed using cancer archives. Somatic variants were further prioritized using a knowledge-driven variant interpretation approach. Sanger sequencing was used to validate functionally important variants.
RESULTS:
We identified 24 rare single-nucleotide variants (SNVs) including 17 non-synonymous SNVs, and 7 INDELs in 18 genes possibly linked to lung carcinoma. Variants were classified as known somatic (
CONCLUSIONS:
Our bioinformatics analysis identified KCNJ18, GPRIN2, TEKT4, HRNR, FOLR3, ESSRA, CTBP2, MPRIP, TBP, and FBXO6 may contribute to progression in NSCLC and could be used as new biomarkers for the treatment. The mechanism by which GPRIN2, KCNJ12, and TEKT4 contribute to tumorigenesis is unclear, but our results suggest they may play an important role in NSCLC and it is worth investigating in future.
1.Introduction
Various studies have proven NSCLC to be a histologically and molecularly heterogeneous group of cancer. The two main histological NSCLC subtypes are adenocarcinoma (ADCA) and squamous cell carcinoma (SQCC). Although the incidence of ADCA is on the rise, SQCC is currently the second most frequent histologic subtype. Distinct subtypes of NSCLC are driven by a specific genetic alteration, the molecular mechanisms of which remains to be fully elucidated. The Cancer Genome Atlas (TCGA) has conducted comprehensive genome studies of NSCLC, displaying a great diversity of molecular variations. Some of the mutated genes were common in both the histology subtypes, and some were group specific. ADCA shows more complex and heterogeneous molecular patterns than SQCC, with a greater number of associated genomic aberrations [1, 2]. Tumor genotype analysis has identified driver alterations in 50–80% of NSCLC patients according to demographics, and particularly ethnicity. Asian people have unique clinical characteristics, tumor histology and show different prevalence of oncogenic mutations [3].
Significant advancement has been made in the treatment of patients with pulmonary ADCA because of the molecular profile. The discovery of EGFR mutations and ALK rearrangement has opened a new era of targeted therapy in ADCA. However, no such molecular target exists for squamous cell carcinoma (SQCC). Whole exome sequencing (WES) has been in wide use for the discovery of new genetic markers which may offer more information for the development of personalized medicine for all subtypes of lung cancer [4]. WES has been widely used in clinical research for the discovery of new genetic markers. This study aims to identify novel genetic markers for NSCLC that can be used as universal biomarkers for the treatment. Additionally, this study identifies and compares the genomic alterations of ADCA subtypes and SQCC subtypes.
2.Materials and methods
2.1Sample collection and diagnosis
A total of 19 NSCLC cases (EGFR, ALK and ROS1 negative) with available clinical follow-up were retrieved from the Department of Pathology, AIIMS, New Delhi. The haematoxylin and eosin-stained slides were analysed and histological type of the tumour was determined according to World Health Organization (WHO) 2021 classification of thoracic tumours. Blocks showing more than 80% tumour component in their respective sections were used for WES. Treatment and follow up details were retrieved from case record files from the Department of Medical Oncology, AIIMS, New Delhi.
2.2Formalin fixed paraffin embedded (FFPE) DNA isolation and repair
DNA extraction was performed using FFPE DNA tissue extraction kit (A2352, Promega, USA) according to the manufacturer’s instructions. FFPE DNA was repaired and purified using Gene JET FFPE DNA Purification Kit (K0881, Thermo Scientific, USA) according to the manufacturer’s instructions. Quantity and purity of gDNA were assessed by Qubit
2.3Sample sequencing
Sequencing libraries were prepared using the SureSelect All Human Exon V5 Kit (USA) according to the manufacturer’s instructions. The final enriched pooled were sequenced on Illumina HiSeq 2000 platform (Illumina Inc., USA) generating 2
2.4Variant calling and quality control
Quality of raw reads (FASTQ files) was examined using FastQC [5]. Adaptor and low-quality sequences were trimmed using Trimmomatic software [6]. Paired clean reads with longer than 50 bases were aligned against the Genome Reference Consortium Human Build 38 patch release 7 (GRCh38.p7) using BWA-MEM algorithm of Burrows-Wheeler Aligner (BWA 0.6.1) [7]. SAM/BAM post-processing steps including SAM to BAM conversion, sorting, adding read group information, mark duplicates, and base quality score recalibration were performed using the Genome Analysis Toolkit (GATK 4.0.6.0) [8, 9]. The quality of the recalibrated BAM files was checked with QualiMap v2.0.2 [10]. Finally, a genomic variation, including single-nucleotide polymorphisms (SNPs) and small INDELs (insertion and deletion) were detected for each sample individually using GATK HaplotypeCaller in GVCF mode (-ERC GVCF), and the results were combined using GenotypeGVCFs. Raw variant calls were soft filtered using GATK VariantFiltration based on the following parameters: LowCoverage (DP
2.5Variant annotations
The impacts of variants on protein structure or function were predicted using SnpEff [11] and ANNOVAR [12]. It compiles prediction scores from multiple algorithms including PhyloP, SIFT, LRT, SiPhy, Polyphen-2, GERP++, MutationAssessor, Fathmm, MutationTaster, CADD, and MetaSVM. In addition to these tools, variants were re-annotated using the germline/population databases (dbSNP, 1000 Genomes and ExAC) [13, 14], and cancer/somatic databases (COSMIC, TCGA and ICGC) [15, 16]. ClinVar [17] and My Cancer Genome (http://www.mycancergenome.org) were used to determine the clinical significance of each variant, while drug databases (PharmGKB, OncoKB) [18, 19] were utilized to gain information about the treatment implications of specific cancer gene mutations and how these mutations affect treatment response.
2.6Additional filters to reduce false positives somatic variants
A high-confidence somatic variant for tumor samples without a matched normal control was selected based on the following criteria: 1) mutations were considered true positives if they have a) QUAL
2.7Enrichment analysis and candidate gene prioritization
Known or predicted variants to be involved in the lung or in related cancers were predicted using DisGenNET [25]. The Human Gene Damage Index server (http://lab.rockefeller.edu/casanova/GDI) was used to predict LoF-intolerant genes. The gene product physically interacts with a protein encoded by a known disease gene was explored using NetworkAnalyst [26]. The DAVID tool was used to perform KEGG pathway and GO functional-enrichment analyses of DEGs. Gene product in a pathway associated with the disease and gene expressed in the tissue or organ of interest was retrieved from the literature.
2.8Survival and expression analysis
A web-based tool, GEPIA (Gene Expression Profiling Interactive Analysis; http://gepia.cancer-pku.cn), was used in the study of lung cancer patients to assess gene expression between tumor and normal data from the Cancer Genome Atlas (TCGA) and Genotype-Tissue Expression (GTEx). During the expression analysis, the threshold included an expression fold change of 1.5 between cancer and normal tissues, as well as a p value of 0.05. This relationship was then visualized with a boxplot. A survival analysis as well as a correlation analysis between two genes were also conducted using GEPIA.
2.9Sanger sequencing
PCR was carried out on ABI Palm thermal cycler (Applied Biosystem, California), using both forward and reverse primers for greater accuracy and the results were analysed using SeqMan II software (DNASTAR 5.07). The mutations with both base counts more than 10% and QV (quality value) more than 20 were considered to be trusted mutations.
3.Results
3.1Patient characteristics and sequencing statistics
A case study of 19 patients was included in this study, where 10 were ADCA (EGFR, ALK and ROS1 wild type), and nine were SQCC histological subtypes of NSCLC. Patients were early onset with the average diagnostic age of 56 years, where male: female ratio was 5.6: 1. Among, three patients were the non-smoker, whereas one case was with unknown smoking history. Nearly 75% of patients were present with co-morbidities, where all patients were either in stage III or IV. A total of 13.48 GB raw data and 11.98 GB processed data were generated per exome for the tumor sample of ADCA, whereas on an average 13.76 GB raw data and 12.24 GB processed data were obtained for the tumor sample of SQCC using WES. A higher percentage of reads were aligned to the human reference genome (GRCh38) in the tumors of ADCA patients (98.87%; range 96.48–99.95%) compared to tumors of SQCC patients (97.40%; range 85.03–99.51%), indicating that the generated dataset was highly relevant with the reference genome. The average GC content (49.74%) in the tumors of ADCA patients ranged from 42.10 to 64.63%, whereas average GC content (48.50%) in the tumors of SQCC patients ranged from 41.81 to 52.28%. Clinical characteristics and sequencing summary of lung cancer patient’s participants in this study are listed in Table S1 in Supplemental File 1.
3.2Detection and characterization of SNVs
After initial variant filtering (as described in methods), a total of 1,157,921 (single-allelic 1,157,792 and multi-allelic 129) variants were retained in the tumors of ADCA patients (
Table 1
Summary of high-confidence somatic SNVs and indels observed in lung cancer patients
Gene | Nucleotide mutation | GRCh38 location | Mutation type | Amino acid alteration | dbSNP ID |
---|---|---|---|---|---|
TEKT4 | G | Chr2: 94876674 | SNV/nonsynonymous | NM_144705:exon6:c.G1213A:p.A405T | rs75603622 |
HRNR | G | Chr1:152216579 | SNV/nonsynonymous | NM_001009931:exon3:c.C5050G:p.R1684G | rs4845749 |
KCNJ18 | C | Chr17:21703417 Chr17:21703571 Chr17:21703568 | SNV/nonsynonymous | NM_001194958:exon3:c.C631T:p.L211F NM_001194958:exon3:c.T785G:p.I262S NM_001194958:exon3:c.G782A:p.R261H | rs1435776313 rs1450551937 rs1291886575 |
ESRRA | G | Chr11:64315821 Chr11:64315823 | SNV/nonsynonymous Deletion/nonframeshift | NM_001282450:exon7:c.G1127T:p.R376L NM_001282450:exon7:c.1130_1132del:p.377_378del | rs201971362 rs759464632 |
CTBP2 | C | Chr10:124994577 Chr10:124994505 Chr10:124994563 Chr10:124994542 | SNV/nonsynonymous | NM_022802:exon5:c.G2292T:p.Q764H NM_022802:exon5:c.T2364A:p.H788Q NM_022802:———-exon5:c.T2306A:p.L769Q NM_022802:exon5:c.G2327A:p.S776N | rs80025996 rs937366751 rs150320719 rs78155918 |
MPRIP | CCAGCAG | Chr17:17136247 | Deletion/nonframeshift | NM_015134:exon6:c.537_539del:p.179_180del | rs779205841 |
TBP | A | Chr6:170561958 | Insertion /nonframeshift | NM_003194:exon3:c.222_223insCAG:p.Q74delinsQQ | rs775224229 |
FBXO6 | A | Chr1:11668809 | SNV/nonsynonymous | exon2:c.A151G:p.M51V | rs138203471 |
FOLR3 | CTA | Chr11:72139110 | Deletion/nonframeshift | exon3:c.46_47del:p.Y16fs | rs1278032834 |
GPRIN2 | C | Chr10:46550016 | SNV/nonsynonymous | NM_014696:exon3:c.G721A:p.V241M | rs9422022 |
LILRA2 | G | Chr19:54574903 | SNV/stopgain | NM_001290270:exon3:c.G489A:p.W163X | rs1455280111 |
MTRNR2L8 | T | Chr11:10508153 | Insertion/frameshift | NM_001190702:exon1:c.73_74insGACAC:p.X25delinsX |
|
UMPS | CT | Chr3:124730565 | Deletion/nonframeshift | NM_000373:exon1:c.95delT:p.L32fs |
|
FDFT1 | GTCCCAC | Chr8:11808709 | Deletion/nonframeshift | exon1:c.193_198del:p.65_66del | rs71711801 |
KRT18 | G | Chr12:52949285 | SNV/stopgain | NM_000224:exon1:c.G112T:p.G38C | rs77999286 |
NYX | G | ChrX:41473563 | SNV/nonsynonymous | NYX:NM_022567:exon2:c.G110A:p.C37Y |
|
LRP2 | C | Chr2:169256124 | SNV/nonsynonymous | LRP2:NM_004525:exon19:c.G2752A:p.G918R |
|
MIR3689F | CGGGATCACACCTCCCAG GAAAGCACGGGATCAGA CCTCCCAGGGAGCACGG GATCACACCTCCCAGCGA GTGT | Chr9:134850674 | SNV/nonsynonymous |
|
|
Figure 1.
Nonsynonymous somatic SNVs and INDELs identified in lung cancer patients by whole-exome sequencing. The outer-coloured ring and number indicate chromosome number and partition; the middle green ring and letters represent genes with non-synonymous SNVs and their corresponding chromosomes; the inner violet ring shows non-synonymous INDELs and their corresponding chromosomes. The star symbol signifies that gene associated with more than one mutation.
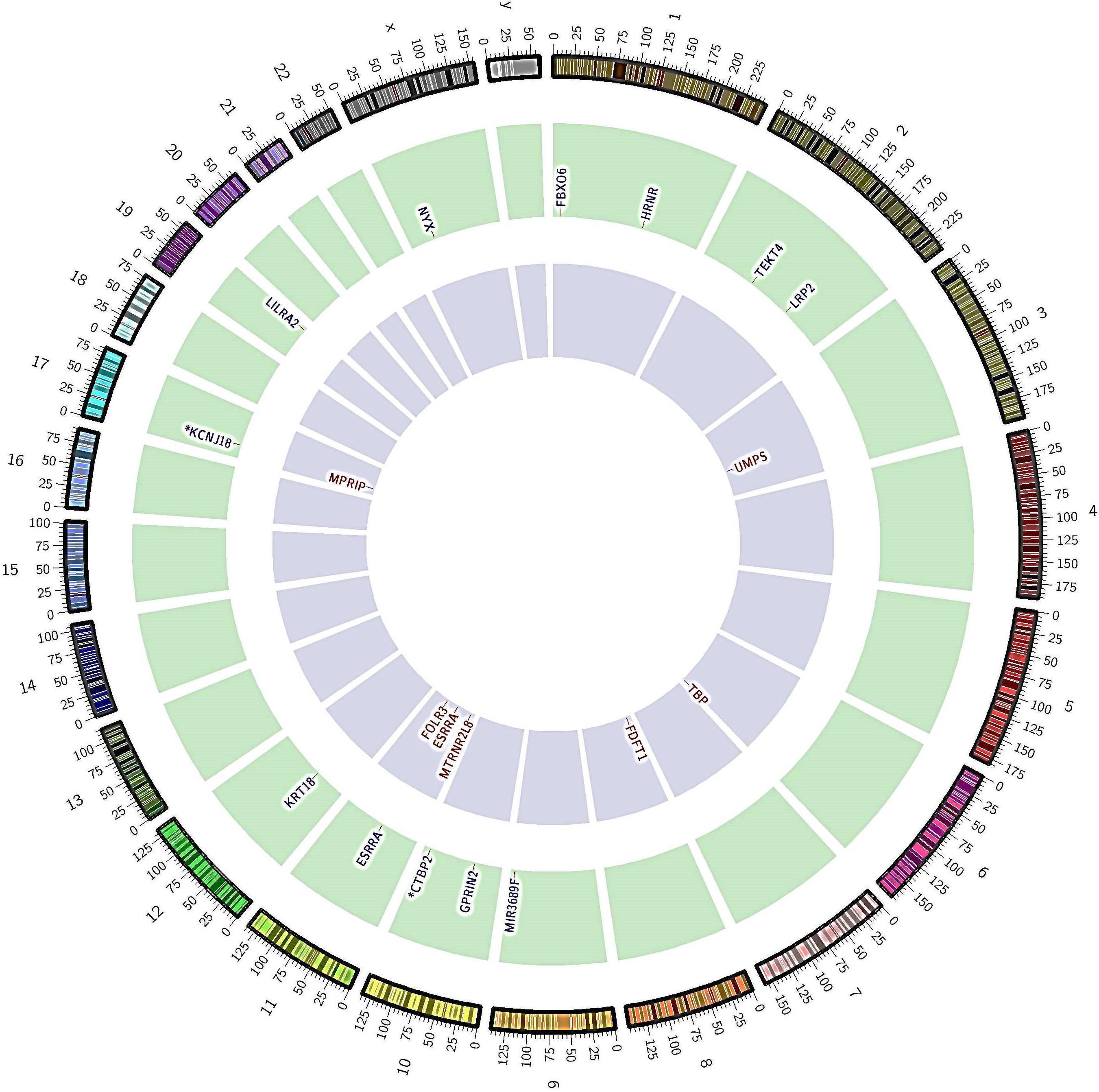
Figure 2.
Oncoplot for the somatic variants in non-small cell lung cancer (NSCLC). The graph depicting top 18 mutated genes ordered by decreasing frequency. The right barplot shows overall frequency in ADCA and SQCC-subtype. The colour box represents the type of mutations including SNV/nonsynonymous (violate), SNV/ stop gain (light blue), deletion/nonframeshift (dark blue), insertion/frameshift (light green) and insertion nonframeshift (red). The top stacked barplot shows a number of somatic mutations per sample.
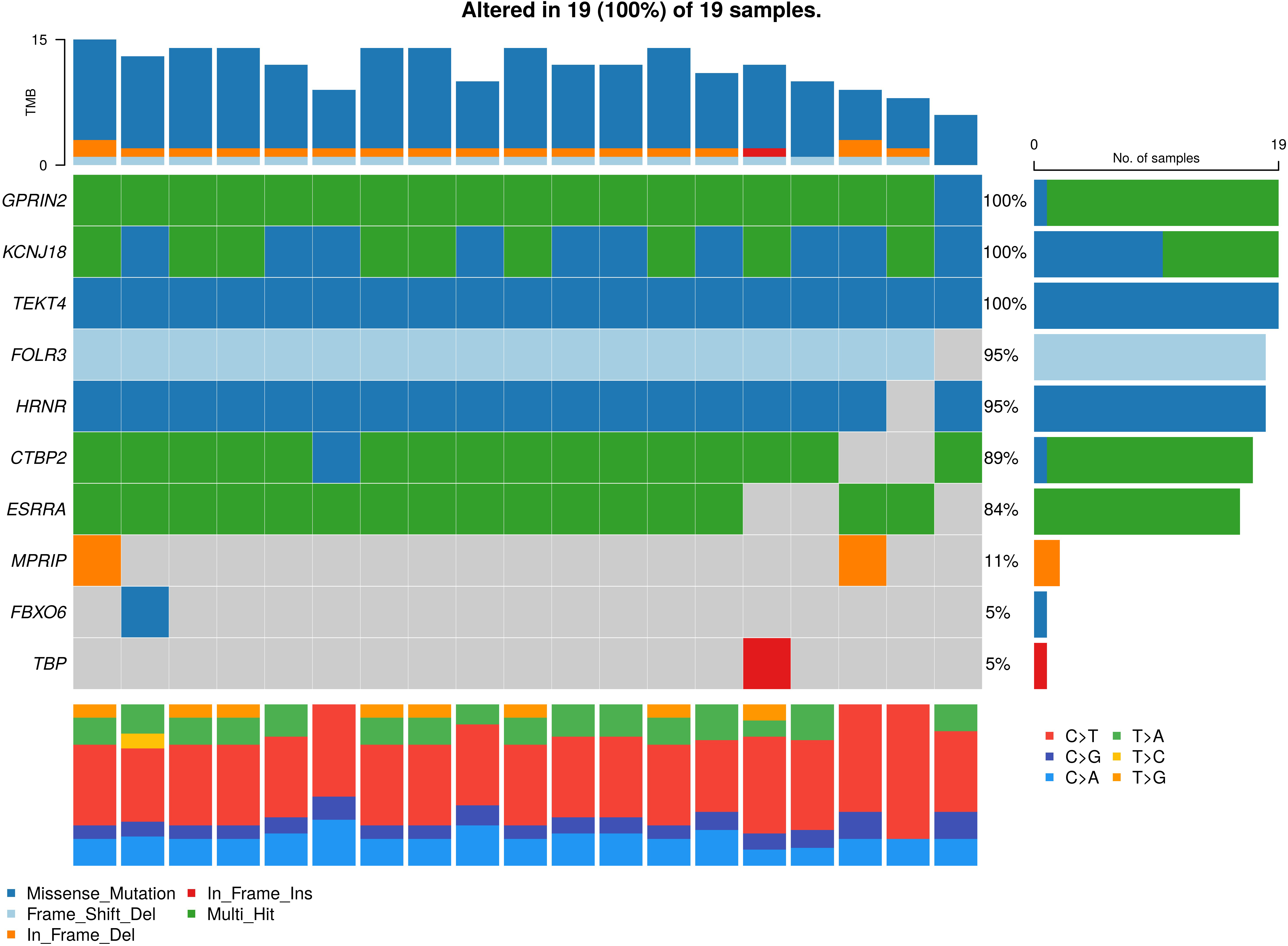
3.3Detection of somatic variants in tumor only samples
To detect somatic SNV, the present study focused on missense variants in the exonic regions or splice sites. The downstream filtering by genomic location revealed a total of 6,712 and 8,000 exonic variants in ADCA and SQCC subtype, respectively. Among exonic SNVs (ADCA subtype), 2,113 were synonymous, 1,985 were nonsynonymous, 28 were frame-shift indels, 51 were nonframe-shift indels, 15 were stop-gain, 2 were stop-loss, and 2,518 were non-coding SNVs. In SQCC-subtype, 3,249 were synonymous, 2,656 were nonsynonymous, 52 were frame-shift indels, 59 were nonframe-shift indels, 36 splicing-variant, 1 was gene fusion, and 1,947 were non-coding SNVs. Exonic missense, nonsense, stop-loss, frameshift and splice site variants all have potential to affect protein function. Therefore, we excluded the synonymous variants that have no functional impact and retained 4,599 and 4,751 variants from ADCA and SQCC subtype, respectively. As rarity [27] is the key criterion to have a functional effect on the encoded protein, the filtered variants were used to eliminate the common germline mutations (minor allele frequency below 5% in population/germline databases) and as an outcome 1,642 and 2,141 variants were retained in ADCA and SQCC subtype, respectively. After excluding false positive mutations (based on additional filter criteria’s 1a-given in methodology), 500 and 734 variants in ADCA and SQCC subtype, respectively was observed. To exclude deemed polymorphic/benign variants, high-quality rare variants (MAF
Table 2
Characteristics of candidate variants from public resources and published literature
Gene | Lung carcinoma | Other carcinoma | Hub genes | Protein overexpression in lung cancer | Pathway associated with lung cancer | Ongoing drug trials | LoF-intolerant genes |
CTBP2 |
|
|
| P16 |
|
| |
ESRRA |
|
|
|
| VEGF |
| |
FBXO6 |
|
|
| ERDA |
|
| |
FDFT1 |
|
|
|
| Mevalonate, WNT |
| |
FOLR3 |
|
|
| ||||
GPRIN2 | Glutamate |
| |||||
HRNR |
|
|
| AKT |
| ||
KCNJ18 |
| P53 | |||||
LILRA2 |
|
|
| ||||
LRP2 |
|
| MAPK, JNK, Headong |
| |||
MIR3689F | |||||||
MPRIP |
|
|
|
| Fusion |
|
|
MTRNR2L8 |
| ||||||
NYX |
| ||||||
TBP |
|
|
| RAS |
| ||
TEKT4 |
| P13K/AKT |
| ||||
UMPS |
|
|
|
| Nucleotide metabolism |
| |
KRT18 |
|
|
|
|
|
Figure 3.
Hub genes in significant network modules. The hub genes with high degree and high betweenness were denoted with red colour.
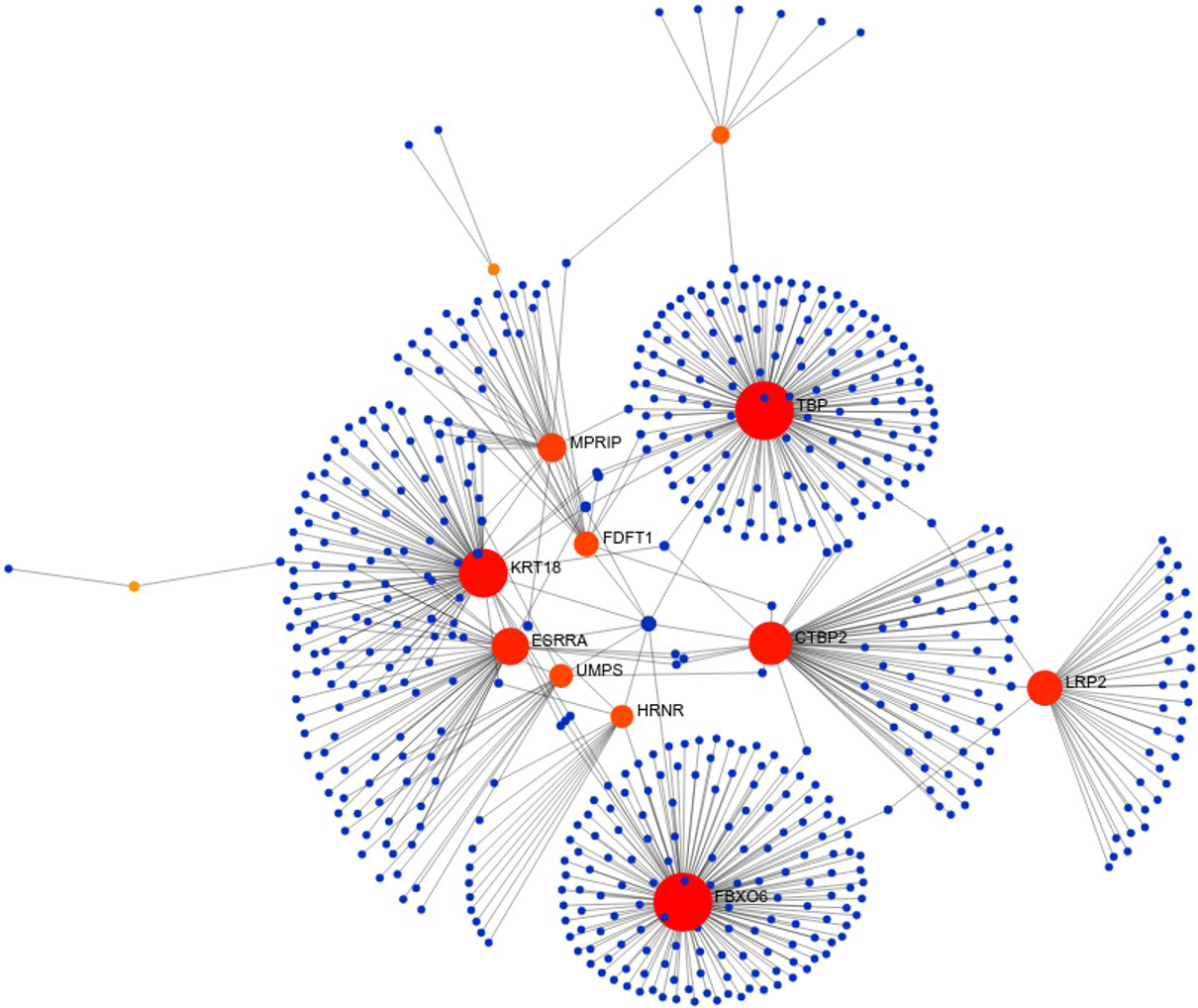
Figure 4.
Scatter plot illustrating enriched KEGG pathways and gene ontology. Top 40 KEGG pathways are depicted in the Fig. 3A. The rich factor was determined by dividing the number of genes enriched in a pathway by the total number of genes annotated in that pathway. Figure 3B, C, and D show the top 10 Biological Processes (BP), Molecular Functions (MF), and Cellular Components (CC), respectively. The colour and size of the dots denote the range of the -log P-value and the number of genes in the shown pathways, respectively. The scatter plot was made using R software v4.0.3.
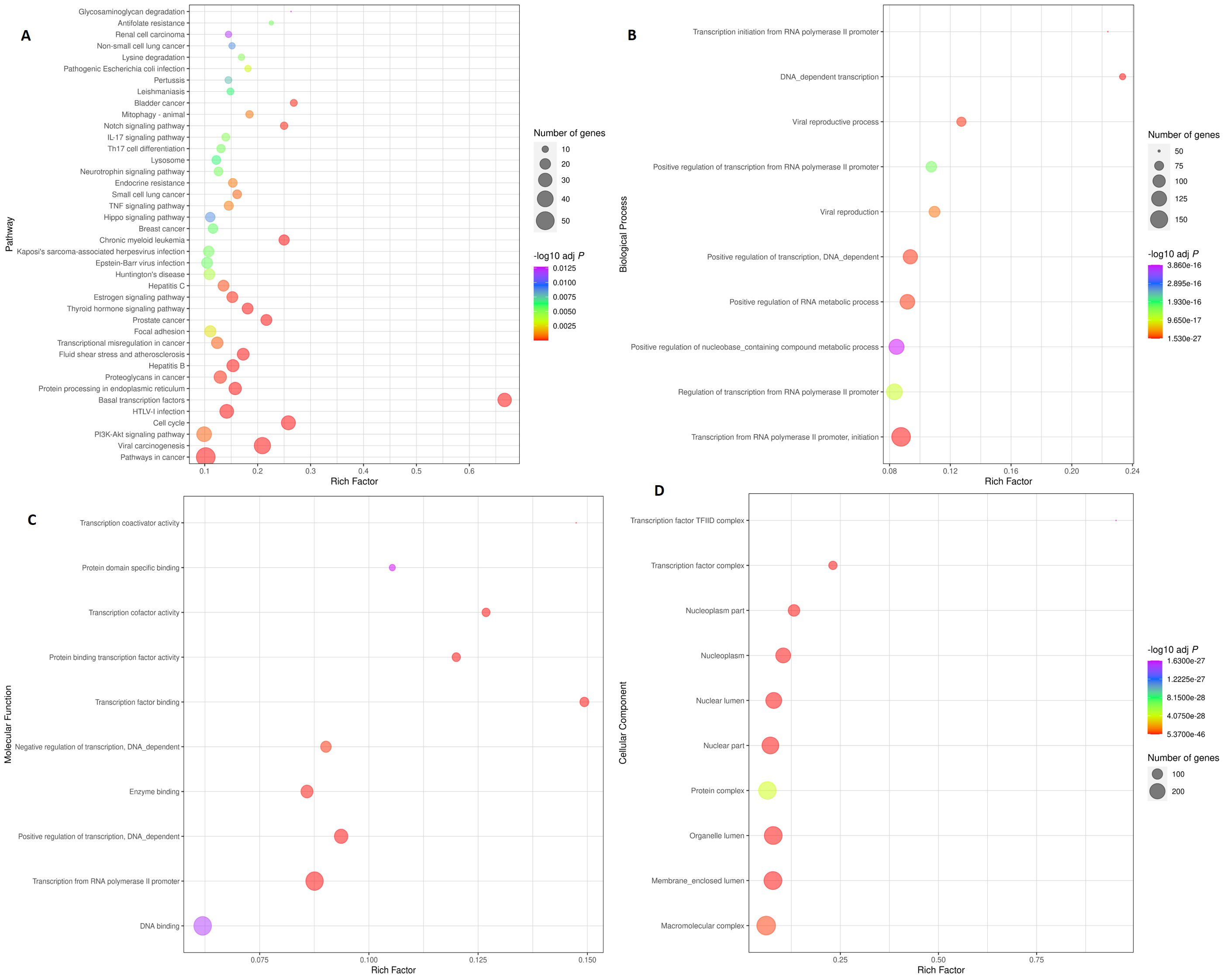
3.4Knowledge-driven variant prioritization
Figure 5.
Prognostic roles of potential key genes in the NSCLC patients. Survival curves are plotted for NSCLC cancer patients. (A) Overall survival: CTBP2, GPRIN2, KRT18, LILRA2, LRP2, UPMS; (B) Disease free survival: CTBP2, KRT18, LRP2.
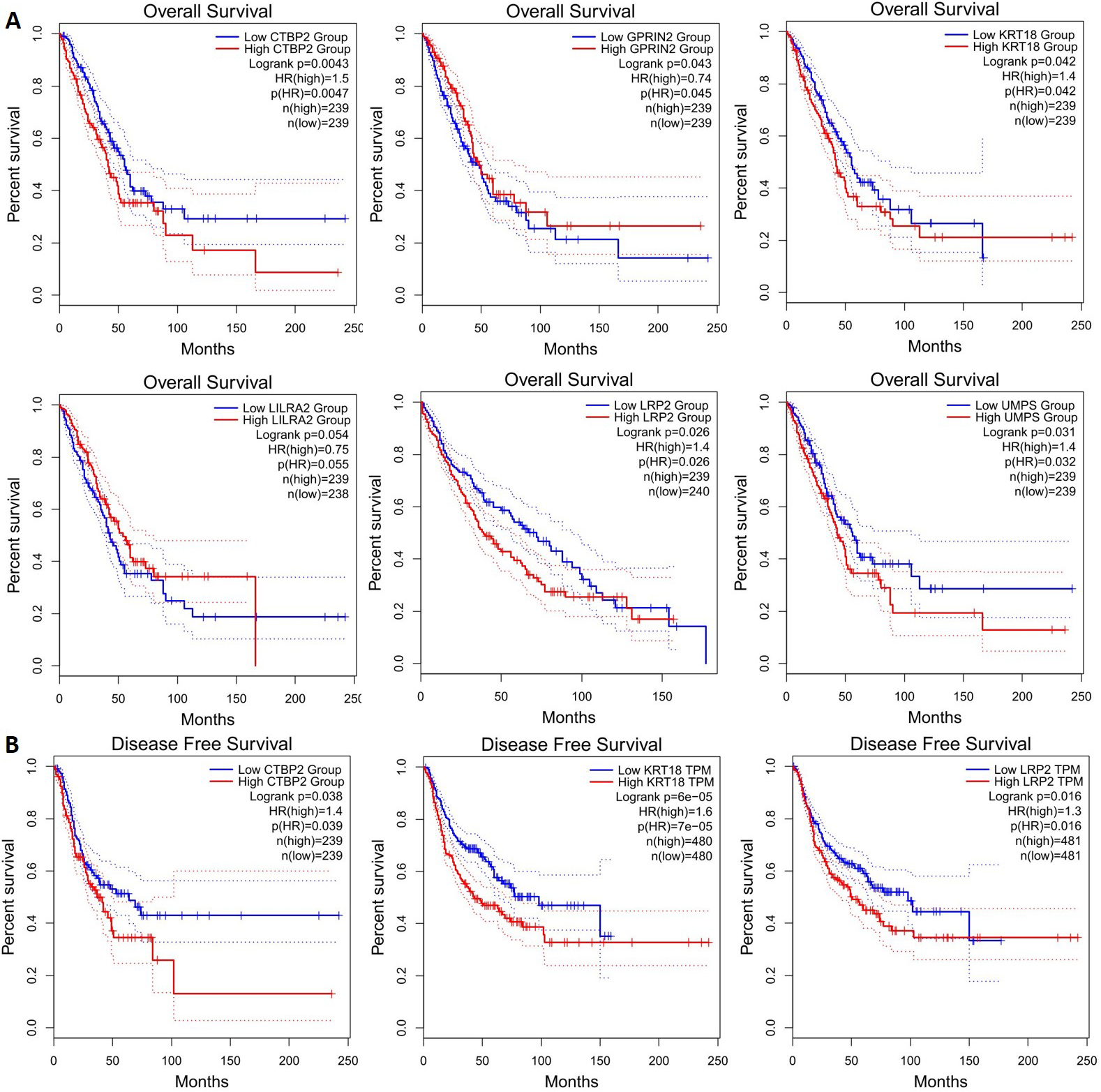
Figure 6.
Analysis of potential key genes expression level in NSCLC patients. The red and gray boxes represent cancer and normal tissues, respectively. (A) LRP2; (B) GPRIN2; (C) FOLR3; (D) MPRIP; (E) KRT18 and (F) LILRA2; LUAD: Lung adenocarcinoma; LUSC: Lung squamous cell carcinomas.
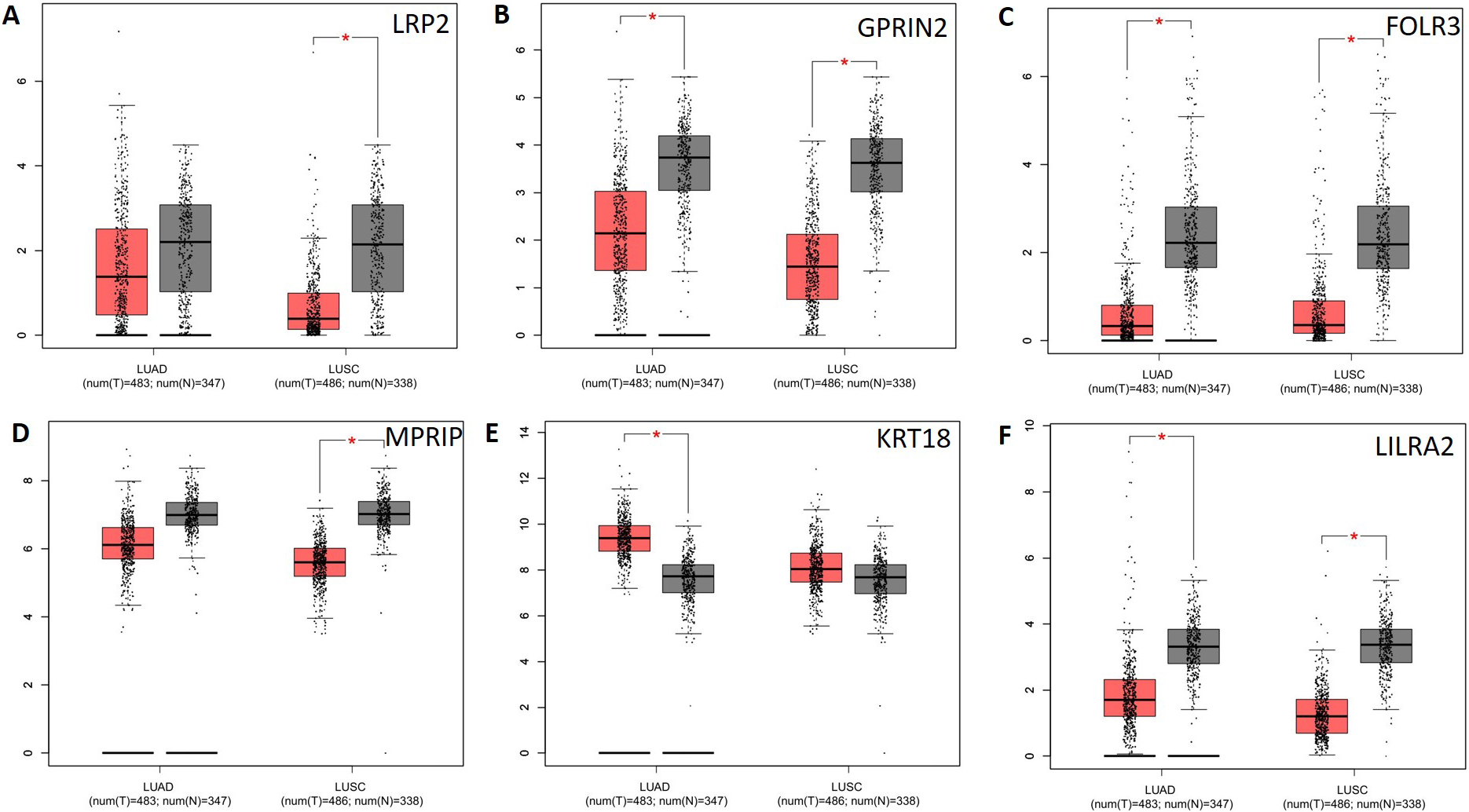
Though, we followed the guidelines suggested for experimental design and variant filtering, yet we obtained more candidates with likely functional effects than can be verified experimentally. In this study, high priority candidates were selected based on the biological hypothesis. The analysis of known or predicted variants to be involved in the lung or in a related cancer revealed nine genes (CTBP2, ESRRA, FBXO6, FDFT1, KRT18, MPRIP, TBP, LILRA2 and UMPS) associated with the lung carcinoma. In addition, four genes i.e., LRP2 (bone, breast, colorectal, pancreatic, prostate, and renal cell carcinoma), HRNR (breast, liver and pancreatic carcinoma), FOLR3 (breast and ovarian carcinoma) and TEKT4 (breast and thyroid carcinoma) were involved in other’s cancer types. The genes namely MIR3689F, MTRNR2L8, NYX, GPRIN2 and KCNJ18 were observed to be not associated with any type of cancer and considered as low priority genes for the validation. The encoded proteins of 16 genes, have loss-of-function (LoF) variants that damage or eliminate them. The LoF-intolerant genes (CTBP2, GPRIN2, HRNR and TEKT4) were classified as extremely loss of function intolerant (pLI
Figure 7.
Correlation analysis of potential key genes in NSLCL. TCGA and/or GTEx expression data show significant correlations between LRP2, FOLR3, LILRA2, and GPRIN2.
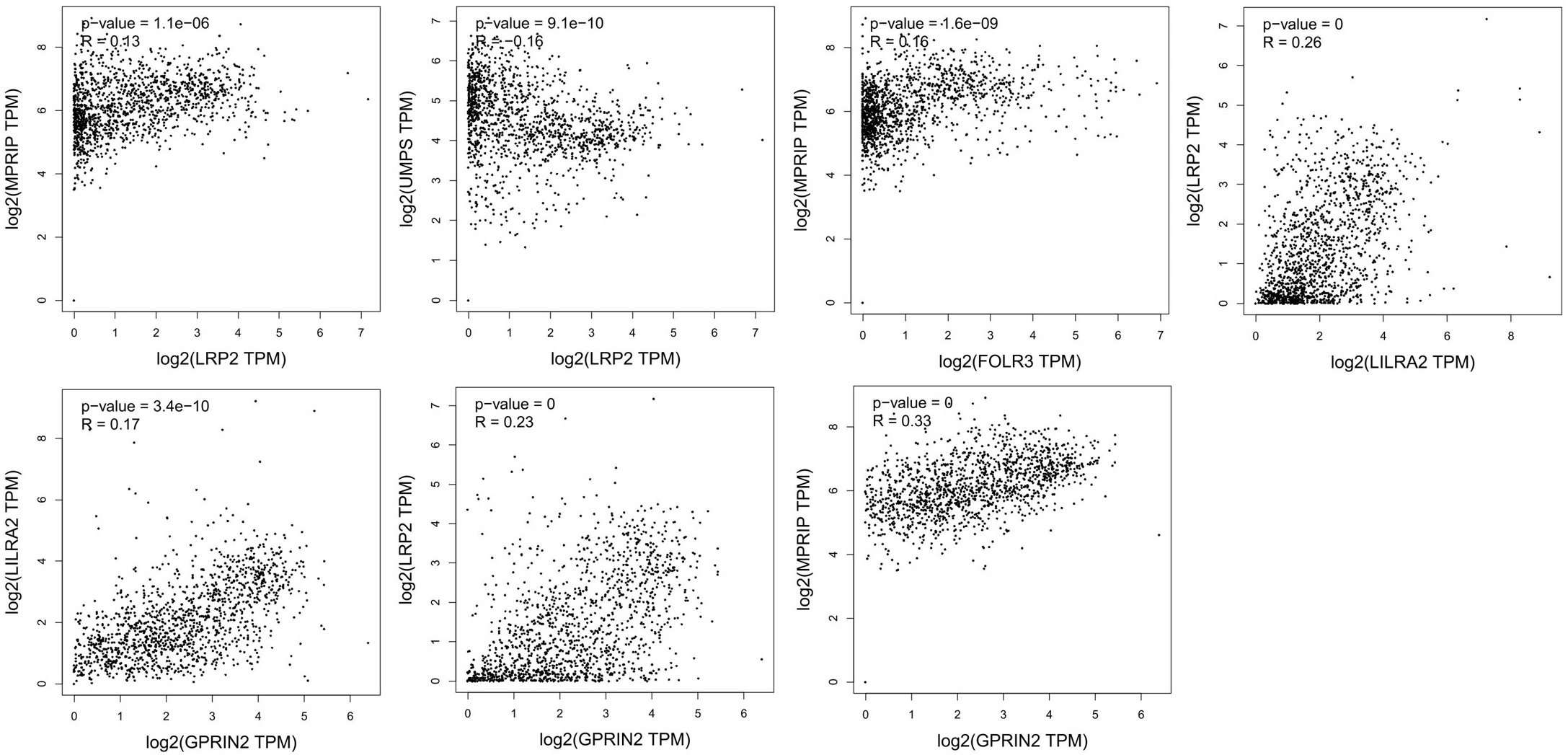
Table 3
Candidate variants identified through whole-exome sequencing which passed in the validation practice by Sanger sequencing
T.S. | KCNJ18 | GPRIN2 | TEKT4 | HRNR | FOLR3 | ESRRA | CTBP2 | MPRIP | TBP | FBXO6 |
---|---|---|---|---|---|---|---|---|---|---|
ADCA subtype | ||||||||||
ADCA01 | B | B | B | B | B | B | B |
|
|
|
ADCA02 | B | B | B | B | B | B | B |
|
|
|
ADCA03 | B | B | B | B | B | W | B |
|
|
|
ADCA04 | B | B | B | B | B | B | W |
| B |
|
ADCA05 | B | B | B | B | B | W | B |
|
|
|
ADCA06 | B | W | B | B | B | B | B |
|
|
|
ADCA07 | B | B | B | B | B | B | W |
|
|
|
ADCA08 | B | B | B | B |
| B | B |
|
|
|
ADCA09 | B | B | B | B | B | B | B | B |
|
|
ADCA10 | B | W | B | B | B | W | B | B |
|
|
SQCC subtype | ||||||||||
SQCC11 | B | B | B | B | B | W | W |
|
| B |
SQCC12 | B | B | B | B | B | B | B |
|
|
|
SQCC13 | B | W | B | B | B | B | B |
|
|
|
SQCC14 | B | B | B | B | B | W | W |
|
|
|
SQCC15 | B | B | B | B | B | B | B |
|
|
|
SQCC16 | B | B | B | B | B | W | B |
|
|
|
SQCC17 | B | B | B |
| B | B | B |
|
|
|
SQCC18 | B | B | B | B | B | W | B |
|
|
|
SQCC19 | B | B | B | B | B | B | B |
|
|
|
B: candidate variants confirmed by both WES and Sanger sequencing; W: candidate variants confirmed by WES only and missed by Sanger sequencing possibly due to low sensitivity;
3.5Expression analysis of potential biomarkers for NSCLC
CTBP2, GPRIN2, KRT18, LILRA2, LRP2, and UMPS mutations are associated with lower overall survival (Fig. 5a), and CTBP2, KRT18, and LRP2 mutations are associated with lower disease-free survival (Fig. 5b). It is therefore possible to identify these genes as potential biomarkers for NSCLC. Next, we applied GEPIA to check gene expression levels in NSCLC tissues and in normal tissues. The expression levels of the 5 genes (LRP2, GPRIN2, FOLR3, MPRIP, LILRA2) decreased significantly, but the expression level of one gene (KRT18) increased in comparison with normal tissues. It was observed that LRP2 and MPRIP gene expression was significantly decreased in the LUSD, whereas KRT18 gene expression increased in the LUAD (Fig. 6). These genes appear to be promising therapeutic targets. Additionally, we examined the correlation between mRNA expression of LRP2 and prognosis in patients with MPRIP (
3.6Somatic variants validation by Sanger sequencing
To eliminate false-positive rates of the identified somatic mutations from WES data, we selected 10 genes for Sanger sequencing validations. Interestingly we observed that seven genes were mutated in more than 60% samples and three genes were mutated in either one or two samples. Further, Sanger sequencing results showed 100% concordance in seven genes and the remaining three genes concordances were found only in 80% cases. The mutations observed along with WES and Sanger sequencing data have been depicted in the Table 3 and Fig. S1 in Supplemental File 1.
The gene-wise results of Sanger sequencing are given below:
• TEKT1, GPRIN2 and KCNJ18 point mutation: These three genes were found mutated in all the samples by WES. On further validation by Sanger sequencing, we also found TEKT1 (exon6: c.G1213A:p.A405T) were positive in 18 cases, GPRIN2 (exon3:c.G721A:p.V241M) in 16 cases and KCNJ18 (c.C631T:p.L211F) point mutations in all samples.
• Hornerin (HRNR) and FOLR3 mutation: These two genes were found mutated in 18 samples by WES. Validation by Sanger sequencing revealed 100% concordance. The Hornerin gene was present with the point mutation in exon 3 (c.C5050G:p.R1684G) and FOLR3 gene with deletion in exon 3 (c.46_47del:p.Y16fs).
• ESSRA and CTBP2 mutation: WES revealed ESSRA gene was mutated in 16 cases and CTBP2 in 17 cases. Sanger sequencing revealed ESRRA gene exon 7 point mutation (c.G1127T:p.R376L) and deletion (c.1130_1132del:p.377_378del) in 12 and 5 cases whereas CTBP2 (exon5:c.G2292T:p. Q764H) point mutations in 15 cases.
• MPRIP, TBP and FBXO6 mutation: Exon 6 deletion of MPRIP gene (c.537_539del:p.179_180del) was found in 2 samples whereas TBP gene (exon3:c.222_223insCAG:p.Q74delinsQQ) deletion and FBXO6 gene (exon2:c.A151G:p.M51V) point mutation were found in one case each by WES and Sanger sequencing.
4.Discussion
This study used whole-exome sequencing to predict genomic alterations in ADCA and SQCC histological subtypes of NSCLC. Overall, we detected 24 somatic variants (ADCA
4.1Mutated genes present in all samples of ADCA and SQCC subtype
Of interest, the three genes, KCNJ18, TEKT4, and GRIPN2 are mutated in all NSCLC samples and can serve as common diagnostic markers for both subtypes.
4.1.1Potassium inwardly rectifying channel subfamily J member 18 (KCNJ18)
Gene encodes a member of the inwardly rectifying potassium channel family and plays a role in resting membrane potential maintenance [28]. The potassium channel involvement in tumour cell proliferation has been studied previously in colorectal carcinoma cell line DLD-1 and human prostate cancer cell line LNCaP by modulating calcium influx [29, 30]. The E139K (rs76265595), G145S (rs75029097) and A185V (rs73979896) mutations in KCNJ12/KCNJ18 gene were identified in esophageal SQCC [31]. Mutations were found in KCNJ18 gene in all the NSCLC patients studied, but the amino acid variations (c.C631T, p.L211F) were different from those reported earlier. So, it can be postulated that KCNJ18 might be involved in p53 pathway, and it may be investigated in larger cohort of patients.
4.1.2G protein-regulated inducer of neurite outgrowth 2 (GPRIN2)
Gene is located on chromosome 16 and encodes glutamate NMDA receptor [32]. Variations in this gene have been found in malignant as well as non-malignant diseases [31, 33]. Rare damaging novel mutations in GPRIN2 genes has been found in 33% melanoma patients (somatic) [34], familial human esophageal SQCC (germline/somatic) [31] as well as 501-Mel melanoma cell line [34]. Mutated GPRIN2 might play a major role in tumorigenesis via glutamate pathway where excess release of glutamate showed more aggressive growth [35, 36]. In the present study we found p.V241M (c.G721A) mutation in all the NSCLC cases, although mutation observed was different from those reported in the literature (p.A233S, rs11204659). The role of this mutation in tumorigenesis is unclear; however, high frequency observed in our study hints that it may be explored in other studies.
4.1.3Tektin 4 gene (TEKT4)
Is present on chromosome 2, encodes tektin4, a constitutive protein of microtubules in cilia, flagella, basal bodies, and centrioles [37]. The biological function of TEKT4 has not been well explained in cancer initiation and development. Variations in the TEKT4 gene play an important role in papillary thyroid cancer progression. TEKT4 knockdown in papillary thyroid cancer cell lines inhibits tumorigenesis by impairing cell proliferation, colony formation, migration, and invasion via blocking the activity of PI3K/AKT pathway [38]. We found TEKT4 gene mutations in all 19 cases studied. However, mutations (c.G1213A, A405T) were different from those reported in papillary thyroid cancer (c.1276_1279delinsACCC). Mutated TEKT4 is associated with increased paclitaxel resistance and poor prognosis in breast cancer patients [39]. This mutation might play a vital role in the pathogenesis of lung cancer however, the role of TEKT4 gene in PI3K/AKT pathway signalling and treatment resistance require further investigations.
4.2Mutated genes present in 80% samples of ADCA and SQCC subtype
In addition to the three aforementioned genes, HRNR, FOLR3, CTBP2 and ESSRA are significantly mutated in more than 80% of the NSCLC samples. All these mutated genes are directly or indirectly play a role in tumorigenesis and can additionally serve as common pathogenetic link for subtypes of NSCLC.
4.2.1C-terminal-binding protein 2 (CTBP2)
Is a member of the CTBP family protein located in the human chromosome 10. CTBP2 is an evolutionary conserved transcriptional co-regulator that interacts with DNA binding transcription factors and chromatin remodelers. CTBP2 represses a number of tumour suppressor genes (E-cadherin, PTEN, and INK4), induces the epithelial-to-mesenchymal transition and functions as an apoptosis antagonist. Aberrant expression of CTBP2 has been found to be associated with tumorigenesis, cancer progression, and poor prognosis [40, 41]. Accumulating evidences indicated that CTBP2 expression is elevated in several types of malignancies which include gastric cancer, melanoma, breast cancer, esophageal SQCC, prostate cancer, hepatocellular carcinoma, and ovarian cancer. High expression of CTBP2 results in progression of esophageal SQCC through negatively regulating p16 (INK4A). CTBP2 is considered as a co-factor of TGF-
4.2.2Estrogen related receptor alpha (ESRRA)
Is evolutionary related to estrogen receptor and can efficiently bind to estrogen receptor that are commonly shared by many target genes. Over-expression of ESRRA has been found in carcinoma of the thyroid, ovary, breast, prostate, colon and endometrium [44, 45]. It is correlated with the poor prognosis. ESRRA suggested being a molecular target for treatment of endometrial cancer. Other investigators reported ESRRA as one of the negative prognostic factors in human prostate cancer. ESRRA is also over-expressed in lung cancer patients and cell line A549 while some studies report low or undetectable [46], estrogen receptor expression in NSCLC cells. ESRRA is up-regulated in NSCLC tissues and promotes the progression, proliferation and invasion via NF-
4.2.3Hornerin gene (HRNR)
Is clustered on the chromosome region 1q21 and it is a member of the S100 protein family. The function of HRNR is poorly clarified in the development of human tumours. Altered expression of HRNR was reported to be involved in cancer development, malignant transformation and invasion. Elevated HRNR has been found in many tumours viz lung SQCC, hepatocellular carcinoma, colorectal cancer, prostate cancer, glioblastoma and cell lines, breast carcinoma and cell linesand acute myeloid leukemia [48]. HRNR has been found to contribute to hepatocellular carcinoma progression via the regulation of the AKT pathway [49]. In the lung SQCC and colorectal carcinoma, altered HRNR expression has been associated with disease recurrence [50]. In the current study we have also found recurrence occurred in nine patients all of whom were mutated with the HRNR gene.
4.2.4Folate receptor gamma (FOLR3)
Gene is located on chromosome 11 and consists of five exons. The FOLR3 receptor is a constitutively secreted form of the folate receptor. FOLR3 is one of the key genes involved in the pemetrexed pathway. Variation in FOLR3 gene affects pemetrexed uptake, metabolism, treatment tolerability, response and survival [51]. In NSCLC and mesothelioma patients, variation in the FOLR3 gene has been reported. FOLR3 germline mutation (rs61734430, c.292C
4.3Histological subtypes specific mutated genes
In the current study 3 histology specific genes were emerged from WES analysis. TBP and MPRIP genes were solely associated with the ADCA subtype whereas FBOX6 was found in one case of SQCC.
4.3.1Myosin Phosphatase Rho Interacting Protein (MPRIP)
Is involved in actin cytoskeleton regulation and has been implicated in a gene fusion (NTRK gene) in lung cancer. However, NTRK fusions are rare in lung cancer. Vaishnavi et al. [53], detected NTRK1-MPRIP gene fusion in NSCLC ADCA subtype (3.3%) that did not contain other common oncogenic alterations. Whereas Peifer (2012) [54] detected mutated MPRIP gene and MPRIP-TP53 gene fusion in small cell lung cancer. They predicted loss-of-function of the mutated MPRIP gene putatively caused early termination of TP53 [54]. We found two patients, of ADCA histology, mutated with MPRIP gene (in-frame deletion, c.537_539del, p.179_180del) which corroborates the fact that it is more common in ADCA subtype of NSCLC. Clinical trials targeting NTRK1-MPRIP fusion in lung cancer are undergoing [55, 56], hence, detecting MPRIP mutations in the lung ADCA is clinically useful.
4.3.2TATA-box binding protein (TBP)
Gene encodes the TATA-binding protein present on chromosome 6. A distinctive feature of TBP is the presence of a long string of glutamines in the N-terminus. This region modulates the DNA binding activity and affects the rate of transcription complex formation and initiation of transcription. It has been reported that alterations in cellular TBP concentrations play an important role in cellular differentiation [57, 58]. RAS oncogenic signalling pathways up-regulate TBP expression. The two key studies [59] have reported enhanced TBP expression induces VEGFA expression and enhances cell migration and tumour vascularization in human colorectal cancers (ADCA subtype). It has been suggested that dysregulation of TBP expression is an early event in tumour development. Given the strong correlation between VEGFA and TBP expression in colon cancer, TBP expression represents a novel biomarker and function as an oncogene. In our small sample size only one ADCA patient was mutated (c.222_223insCAG, p.Q74delinsQQ) with TBP gene.
4.3.3F-box protein 6 (FBXO6)
A member of F-box proteins, component of the evolutionarily conserved ubiquitin-protein ligase complex SCF and known to interact with cancer hallmark pathways [60]. There was one case of SQCC mutated with FBXO6 gene (c.A151G, p.M51V), the same mutation has also been studied in Merkel cell carcinoma and rectal carcinoma previously [61]. Impaired FBXO6 expression induces ubiquitin-mediated degradation of target molecules thereby promoting the therapeutic resistance of human cancer cells [62, 63]. FBXO6 promotes growth and proliferation in gastric cancer [64, 65]. On the contrary, studies in NSCLC (cell lines and tumours) found inhibitory effects of FBXO6 along with positive correlation with early TNM stage and favourable survival [66]. Cisplatin is one of the most commonly used platinum-based chemotherapy for the SQCC subtype of NSCLC [67, 68]. FBXO6 is known to inhibit the phosphorylation of checkpoint kinase 1 (Chk1), an important component of DNA repair pathway. This effect, in turn, promotes the sensitivity of cisplatin. Studies have proposed that any defect in FBXO6 gene leads to early development of cisplatin resistance and treatment failure [69]. FBXO6 may be a useful therapeutic target to overcome chemoresistance of cisplatin-based chemotherapy agents [66]. Thus, FBXO6 can serve as a potential biomarker in SQCC of lung cancer for predicting anticancer drugs responsiveness.
5.Conclusions
In this study, novel somatic mutations and subtype-specific mutations were found with WES and subsequently confirmed by Sanger sequencing. Mutated TBP and MPRIP genes were exclusively associated with ADCA subtype, whereas FBOX6 was associated with SQCC. In addition, mutations in the GPRIN2, KCNJ18, and TEKT4 genes were detected in all patients [70, 71, 72, 73, 74]. Although the mechanisms of GPRIN2, KCNJ12 and TEKT4 in tumorigenesis are unclear, our results suggest that these genes may play important roles in NSCLC, and they are worth investigating in the future. The target genes identified in our study can be used as biomarkers for detection and diagnosis of NSCLC. This study shows that WES can be applied to samples from clinical settings to find or validate biomarkers in cancer research.
Abbreviations
NSCLC: non-small-cell lung carcinoma; ADCA: pulmonary adenocarcinoma; SNVs: single-nucleotide variants; FFPE: formalin fixed paraffin embedded; BWA: burrows-wheeler aligner; GRCh: genome reference consortium human; COSMIC: the catalogue of somatic mutations in cancer; ICGC: the international cancer genome consortium; TCGA: the cancer genome atlas; VAF: variant allele frequency; PCR: polymerase chain reaction.
Data availability
Raw data files have been submitted to the Sequence Read Archive (SRA) of National Center for Biotechnology Information (NCBI; https://www.ncbi.nlm.nih.gov/ sra) under BioProject accession number PRJNA734015.
Author contributions
Conception: Jain Deepali.
Sample collection: Mohan Anant, Malik Prabhat, Kumar Sunil.
Bioinformatic analysis: Katiyar Amit.
Validation: Singh Varsha, Jain Deepali.
Interpretation or analysis of data: Katiyar Amit, Singh Varsha.
Figure construction: Katiyar Amit, Singh Varsha.
Manuscript preparation: Singh Varsha, Katiyar Amit.
Review and Supervise: Jain Deepali, Singh Harpreet.
Ethical clearance
The study on 19 NSCLC patients retrieved from the Department of Pathology, A.I.I.M.S., New Delhi was conducted in accordance with the ethical guidelines and regulations of the AIIMS and after obtaining approval from the AIIMS ethics committee. The ethical approval number is IECPG No. 480/29.08.2016. Study participants were enrolled following their voluntary written informed consent.
Supplementary data
The supplementary files are available to download from http://dx.doi.org/10.3233/CBM-220211.
Acknowledgments
We gratefully acknowledge the financial support from the Lady Tata Memorial Trust (N-1611). We thank the ICMR-AIIMS Genomics Centre and CCRF: Bioinformatics Facility at All India Institute of Medical Sciences (A.I.I.M.S.), New Delhi for providing their facility for the data analysis.
Conflict of interest
All the authors declare that there is no conflict of interest related to this study.
References
[1] | P. Hammerman, D. Voet, M. Lawrence, D. Voet, R. Jing, K. Cibulskis, et al., Comprehensive genomic characterization of squamous cell lung cancers, Nature 489: ((2026) ), 519–525. |
[2] | D.H. Hwang, L.M. Sholl, V. Rojas-Rudilla, D.L. Hall, P. Shivdasani, E.P. Garcia et al., KRAS and NKX2-1 Mutations in Invasive Mucinous Adenocarcinoma of the Lung, J Thorac Oncol 11: ((2016) ), 496–503. |
[3] | S. Dearden, J. Stevens, Y.L. Wu and D. Blowers, Mutation incidence and coincidence in non small-cell lung cancer: meta-analyses by ethnicity and histology (mutMap), Ann Oncol Off J Eur Soc Med Oncol 24: ((2013) ), 2371–2376. |
[4] | E. Maggi, N.E. Patterson and C. Montagna, Technological advances in precision medicine and drug Development, Expert Rev Precis Med Drug Dev 1: ((2016) ), 331–343. |
[5] | S. Andrews, Babraham, Bioinformatics FastQC A Quality Control tool for High Throughput Sequence Data, Soil 5: ((2020) ). |
[6] | A.M. Bolger, M. Lohse and B. Usadel, Trimmomatic: a flexible trimmer for Illumina sequence data, Bioinformatics 30: ((2014) ), 2114–2120. |
[7] | H. Li and R. Durbin, Fast and accurate short read alignment with Burrows-Wheeler transform, Bioinformatics 25: ((2009) ), 1754–1760. |
[8] | A. McKenna, M. Hanna, E. Banks, A. Sivachenko, K. Cibulskis, A. Kernytsky, et al., The Genome Analysis Toolkit: a MapReduce framework for analyzing next-generation DNA sequencing data, Genome Res 20: ((2010) ), 1297–1303. |
[9] | G.A. Van der Auwera, M.O. Carneiro, C. Hartl, R. Poplin, G. Del Angel, A. Levy-Moonshine, et al., From FastQ data to high confidence variant calls: the Genome Analysis Toolkit best practices Pipeline, Curr Protoc Bioinformatics 43: ((2013) ), 1110.1–11.10.33. |
[10] | K. Okonechnikov, A. Conesa and F. García-Alcalde, Qualimap 2: advanced multi-sample quality control for high-throughput sequencing data, Bioinformatics32 ((2016) ), 292–294. |
[11] | P. Cingolani, A. Platts, L. Wang le, M. Coon, T. Nguyen, L. Wang et al., A program for annotating and predicting the effects of single nucleotide polymorphisms, SnpEff: SNPs in the genome of Drosophila melanogaster strain w1118; iso-2; iso-3, Fly (Austin) 6: ((2012) ), 80–92. |
[12] | K. Wang, M. Li and H. Hakonarson, ANNOVAR: functional annotation of genetic variants from high-throughput sequencing data, Nucleic Acids Res 38: ((2010) ), e164. |
[13] | K.J. Karczewski, B. Weisburd, B. Thomas, M. Solomonson, D.M. Ruderfer, D. Kavanagh, et al., The Exome Aggregation Consortium, Daly MJ, MacArthur DG. The ExAC browser: displaying reference data information from over 60000 exomes, Nucleic Acids Res 45: ((2017) ): D840–D845. |
[14] | Genomes Project Consortium, G.R. Abecasis, A. Auton, L.D. Brooks, M.A. DePristo, R.M. Durbin, et al., An integrated map of genetic variation from 1,092 human genomes, Nature 491: ((2012) ), 56–65. |
[15] | S.A. Forbes, G. Bhamra, S. Bamford, E. Dawson, C. Kok, J. Clements, et al., The Catalogue of Somatic Mutations in Cancer (COSMIC), Curr Protoc Hum Genet 10: ((2008) ). |
[16] | T.J. Hudson, W. Anderson, A. Artez, A.D. Barker, C. Bell et al., International network of cancer genome projects. International network of cancer genome projects, Nature 464: ((2010) ), 993–998. |
[17] | M.J. Landrum, J.M. Lee, G.R. Riley, W. Jang, W.S. Rubinstein, D.M. Church, et al., ClinVar: public archive of relationships among sequence variation and human phenotype, Nucleic Acids Res 42: ((2014) ), D980–D985. |
[18] | M. Whirl-Carrillo, E.M. McDonagh, J.M. Hebert, L. Gong, K. Sangkuhl, C.F. Thorn, et al., Pharmacogenomics knowledge for personalized medicine, Clin Pharmacol Ther 92: ((2012) ), 414–417. |
[19] | D. Chakravarty, J. Gao, S.M. Phillips, R. Kundra, H. Zhang, J. Wang, et al., Onco KB: A Precision Oncology Knowledge Base, JCO Precis Oncol PO PO.17.00011: ((2017) ), doi: 10.1200/PO.17.00011. |
[20] | M.A. Laginestra, L. Cascione, G. Motta, F. Fuligni, C. Agostinelli, M. Rossi, et al., Whole exome sequencing reveals mutations in FAT1 tumor suppressor gene clinically impacting on peripheral T-cell lymphoma not otherwise specified, Mod Pathol 33: ((2020) ), 179–187. |
[21] | J.G. Tate, S. Bamford, H.C. Jubb, Z. Sondka, D.M. Beare, N. Bindal, et al., COSMIC: the Catalogue Of Somatic Mutations In Cancer, Nucleic Acids Res 47: ((2019) ), D941–D947. |
[22] | D.C. Koboldt, Best practices for variant calling in clinical sequencing, Genome Med 12: ((2020) ): 91. |
[23] | C. Kandoth, M.D. McLellan, F. Vandin, K. Ye, B. Niu, C. Lu, et al., Mutational landscape and significance across 12 major cancer types, Nature 502: ((2013) ), 333–339. |
[24] | V. Heinrich, J. Stange, T. Dickhaus, P. Imkeller, U. Krüger, S. Bauer, et al., The allele distribution in next-generation sequencing data sets is accurately described as the result of a stochastic branching process, Nucleic Acids Res 40: ((2012) ), 2426–2431. |
[25] | J. Piñero, N. Queralt-Rosinach, À. Bravo, J. Deu-Pons, A. Bauer-Mehren, M. Baron, et al., DisGeNET: a discovery platform for the dynamical exploration of human diseases and their genes, Database (Oxford) 2015:bav028: ((2015) ), doi: 10.1093/database/bav028. |
[26] | G. Zhou, O. Soufan, J. Ewald, R.E.W. Hancock, N. Basu and J. Xia, NetworkAnalyst 3.0: a visual analytics platform for comprehensive gene expression profiling and meta-analysis, Nucleic Acids Res 47: ((2019) ), W234–W241. |
[27] | L. Ding, M.H. Bailey, E. Porta-Pardo, V. Thorsson, A. Colaprico, D. Bertrand, et al., Perspective on Oncogenic Processes at the End of the Beginning of Cancer Genomics, Cell 173: ((2018) ), 305–320. |
[28] | J.P. Hugnot, F. Pedeutour, C. Le Calvez, J. Grosgeorge, E. Passage, M. Fontes, et al., The human inward rectifying K+ channel Kir 2.2 (KCNJ12) gene: gene structure, assignment to chromosome 17p11.1, and identification of a simple tandem repeat polymorphism, Genomics 39: ((1997) ), 113–116. |
[29] | R.N. Skryma, N.B. Prevarskaya, L. Dufy-Barbe, M.F. Odessa, J. Audin and B. Dufy, Potassium conductance in the androgen-sensitive prostate cancer cell line, LNCaP: involvement in cell proliferation, Prostate 33: ((1997) ), 112–122. |
[30] | Y. Xiaoqiang and H.Y. Kwan, Activity of voltage-gated K+ channels is associated with cell proliferaton and Ca2+ influx in carcinoma cells of colon cancer, Life Sci 65: ((1999) ), 55–62. |
[31] | N. Khalilipour, A. Baranova, A. Jebelli, A. Heravi-Moussavi, S. Bruskin and M.R. Abbaszadegan, Familial Esophageal Squamous Cell Carcinoma with damaging rare/germline mutations in KCNJ12/KCNJ18 and GPRIN2 genes, Cancer Genet 221: ((2018) ), 46–52. |
[32] | J.W. Johnson and P. Ascher, Glycine potentiates the NMDA response in cultured mouse brain Neurons, Nature 325: ((1987) ), 529–531. |
[33] | N. Iida and T. Kozasa, Identification and biochemical analysis of GRIN1 and GRIN2, Methods Enzymol 390: ((2004) ), 475–483. |
[34] | X. Wei, V. Walia, J.C. Lin, J.K. Teer, T.D. Prickett, J. Gartner, et al., Exome sequencing identifies GRIN2A as frequently mutated in melanoma, Nat Genet 43: ((2011) ), 442–446. |
[35] | T. Takano, J.H. Lin, G. Arcuino, Q. Gao, J. Yang and M. Nedergaard, Glutamate release promotes growth of malignant gliomas, Nat Med 7: ((2001) ), 1010–1115. |
[36] | M.B. Dalva, M.A. Takasu, M.Z. Lin, S.M. Shamah, L. Hu, N.W. Gale, et al., EphB receptors interact with NMDA receptors and regulate excitatory synapse formation, Cell 103: ((2000) ), 945–956. |
[37] | L.A. Amos, The tektin family of microtubule-stabilizing proteins, Genome Biol 9: ((2008) ), 229. |
[38] | Z. Zheng, X. Zhou, Y. Cai, E. Chen, X. Zhang, O. Wang, et al., TEKT4 Promotes Papillary Thyroid Cancer Cell Proliferation, Colony Formation, and Metastasis through Activating PI3K/Akt Pathway, Endocr Pathol 29: ((2018) ), 310–316. |
[39] | Y.Z. Jiang, K.D. Yu, W.T. Peng, G.H. Di, J. Wu, G.Y. Liu, et al., Enriched variations in TEKT4 and breast cancer resistance to paclitaxel, Nat Commun 5: ((2014) ), 3802. |
[40] | C. Zhang, C. Gao, Y. Xu and Z. Zhang, CtBP2 could promote prostate cancer cell proliferation through c-Myc signaling, Gene 546: ((2014) ), 73–79. |
[41] | F. Dai, Y. Xuan, J.J. Jin, S. Yu, Z.W. Long, H. Cai, et al., CtBP2 overexpression promotes tumor cell proliferation and invasion in gastric cancer and is associated with poor prognosis, Oncotarget 8: ((2017) ), 28736–28749. |
[42] | M.W. Straza, S. Paliwal, R.C. Kovi, B. Rajeshkumar, P. Trenh, D. Parker, et al., Therapeutic targeting of C-terminal binding protein in human cancer, Cell Cycle 9: ((2010) ), 3740–3750. |
[43] | D.P. Wang, L.L. Gu, Q. Xue, H. Chen and G.X. Mao, CtBP2 promotes proliferation and reduces drug sensitivity in non-small cell lung cancer via the Wnt/β-catenin pathway, Neoplasma 65: ((2018) ), 888–897. |
[44] | H. Luo, G.O. Rankin, L. Liu, M.K. Daddysman, B.H. Jiang and Y.C. Chen, Kaempferol inhibits angiogenesis and VEGF expression through both HIF dependent and independent pathways in human ovarian cancer cells, Nutr Cancer 61: ((2009) ), 554–563. |
[45] | P. Sun, J. Sehouli, C. Denkert, A. Mustea, D. Könsgen, I. Koch, et al., Expression of estrogen receptor-related receptors, a subfamily of orphan nuclear receptors, as new tumor biomarkers in ovarian cancer cells, J Mol Med 83: ((2005) ), 457–467. |
[46] | J.C. Lai, Y.W. Cheng, H.L. Chiou, M.F. Wu, C.Y. Chen and H. Lee, Gender difference in estrogen receptor alpha promoter hypermethylation and its prognostic value in non-small cell lung cancer, Int J Cancer 117: ((2005) ), 974–980. |
[47] | J. Zhang, X. Guan, N. Liang and S. Li, Estrogen-related receptor alpha triggers the proliferation and migration of human non-small cell lung cancer via interleukin-6, Cell Biochem Funct 36: ((2018) ), 255–262. |
[48] | J.H. Cho, J. Sun, S. Lee, J.S. Ahn, K. Park, K.U. Park, et al., OA10.05 An Open-Label, Multicenter, Phase II Single Arm Trial of Osimertinib in NSCLC Patients with Uncommon EGFR Mutation(KCSG-LU15-09), J Thorac Oncol 13: ((2018) ), S344. |
[49] | S.J. Fu, S.L. Shen, S.Q. Li, Y.P. Hua, W.J. Hu, B.C. Guo, et al., Hornerin promotes tumor progression and is associated with poor prognosis in hepatocellular carcinoma, BMC Cancer 18: ((2018) ), 4719–4725. |
[50] | H. Zhang, J. Liu, D. Yue, L. Gao, D. Wang, H. Zhang, et al., Clinical significance of E-cadherin, β-catenin, vimentin and S100A4 expression in completely resected squamous cell lung carcinoma, J Clin Pathol 66: ((2013) ), 937–945. |
[51] | A. Corrigan, J.L. Walker, S. Wickramasinghe, M.A. Hernandez, S.J. Newhouse, A.A. Folarin, et al., Pharmacogenetics of pemetrexed combination therapy in lung cancer: Pathway analysis reveals novel toxicity associations, Pharmacogenomics J 14: ((2014) ), 411–417. |
[52] | G.V. Scagliotti, P. Parikh, J. Von Pawel, B. Biesma, J. Vansteenkiste, C. Manegold et al., Phase III study comparing cisplatin plus gemcitabine with cisplatin plus pemetrexed in chemotherapy-naïve patients with advanced-stage non-small-cell lung cancer, J Clin Oncol 26: ((2008) ), 3543–3551. |
[53] | A. Vaishnavi, M. Capelletti, A.T. Le, S. Kako, M. Butaney, D. Ercan, et al., Oncogenic and drug-sensitive NTRK1 rearrangements in lung cancer, Nat Med 19: ((2013) ), 1469–1472. |
[54] | M. Peifer, L. Fernández-Cuesta, M.L. Sos, J. George, D. Seidel, L.H. Kasper, et al., Integrative genome analyses identify key somatic driver mutations of small-cell lung cancer, Nat Genet 44: ((2012) ), 1104–1110. |
[55] | A. Drilon, G. Li, S. DOgan, M. GOunder, R. Shen, M. Arcila, et al., What Hides Behind the MASC: Clinical Response and Acquired Resistance to Entrectinib After ETV6-NTRK3 Identification in a Mammary Analogue Secretory Carcinoma (MASC), Ann Oncol 27: ((2016) ), 920–926. |
[56] | M. Russo, S. Misale, G. Wei, G. Siravegna, G. Crisafulli, L. Lazzari, et al., Acquired resistance to the TRK inhibitor entrectinib in colorectal cancer, Cancer Discov 6: ((2016) ), 36–44. |
[57] | S. Zhong, J. Fromm and D.L. Johnson, TBP Is Differentially Regulated by c-Jun N-Terminal Kinase 1 (JNK1) and JNK2 through Elk-1, Controlling c-Jun Expression and Cell Proliferation, Mol Cell Biol 27: ((2007) ), 54–64. |
[58] | M.J. Xu, D.E. Johnson and J.R. Grandis, EGFR-targeted therapies in the post-genomic era, Cancer Metastasis Rev 36: ((2017) ), 463–473. |
[59] | H.L. Goel and A.M. Mercurio, VEGF targets the tumour cell, Nat Rev Cancer 13: ((2013) ): 871–882. |
[60] | S.J. Randle and H. Laman, F-box protein interactions with the hallmark pathways in cancer, Semin Cancer Biol 36: ((2016) ), 3–17. |
[61] | P.W. Harms, P. Vats, M.E. Verhaegen, D.R. Robinson, Y.M. Wu, S.M. Dhanasekaran, et al., The Distinctive Mutational Spectra of Polyomavirus-Negative Merkel Cell Carcinoma, Cancer Res 75: ((2015) ), 3720–3727. |
[62] | X. Hong, H. Huang, X. Qiu, Z. Ding, X. Feng, Y. Zhu, et al., Targeting posttranslational modifications of RIOK1 inhibits the progression of colorectal and gastric cancers, Elife 7: ((2018) ), e29511. |
[63] | H.Z. Xu, Z.Q. Wang, H.Z. Shan, L. Zhou, L. Yang, H. Lei, et al., Overexpression of Fbxo6 inactivates spindle checkpoint by interacting with Mad2 and BubR1, Cell Cycle 17: ((2018) ), 2779–2789. |
[64] | J. Gong, J. Cao, G. Liu and J.R. Huo, Function and mechanism of F-box proteins in gastric cancer (Review), Int J Oncol 47: ((2015) ), 43–50. |
[65] | Y. Zhao, J. Liu, X. Cai, Z. Pan, J. Liu, W. Yin, et al., Efficacy and safety of first line treatments for patients with advanced epidermal growth factor receptor mutated, non-small cell lung cancer: Systematic review and network meta-analysis, BMJ 367: ((2019) ), 5460. |
[66] | L. Cai, J. Li, J. Zhao, Y. Guo, M. Xie, X. Zhang, et al., Fbxo6 confers drug-sensitization to cisplatin via inhibiting the activation of Chk1 in non-small cell lung cancer, FEBS Lett 593: ((2019) ), 1827–1836. |
[67] | J.H. Schiller, D. Harrington, C.P. Belani, C. Langer, A. Sandler, J. Krook, et al., Comparison of four chemotherapy regimens for advanced non-small-cell lung cancer, N Engl J Med 346: ((2002) ), 92–98. |
[68] | R. Arriagada, B. Bergman, A. Dunant, T. Le Chevalier, J.P. Pignon and J. Vansteenkiste, Cisplatin-Based Adjuvant Chemotherapy in Patients with Completely Resected Non-Small-Cell Lung Cancer, N Engl J Med 350: ((2004) ), 351–360. |
[69] | J. Gong, Y. Zhou, D. Liu and J. Huo, F-box proteins involved in cancer-associated drug resistance, Oncol Lett 15: ((2018) ), 8891–8900. |
[70] | V. Singh, A. Katiyar, P. Malik, A. Mohan, H. Singh and D. Jain, P1. 14-48 Whole Exome Sequencing (WES) in Non-Small Cell Lung Carcinoma (NSCLC): Identification of Novel Biomarkers, Journal of Thoracic Oncology 14: ((2019) ), S574. |
[71] | Y.S. Chang, S.J. Tu, Y.C. Chen, et al., Mutation profile of non-small cell lung cancer revealed by next generation sequencing, Respir Res 22: ((2021) ), 3. |
[72] | L. Ruihan, G.E. Chuang, X. Xiao, S. Jing, M. Shiqi and T. Yongyao, Identification of genetic variations associated with drug resistance in non-small cell lung cancer patients undergoing systemic treatment, Brief Bioinform, 22: ((2021) ): bbab187. doi: 10.1093/bib/bbab187. |
[73] | J.B. Mitchem, A. Miller, Y. Manjunath, M. Barbirou, M. Raju and Y. Shen, Somatic mutation variant analysis in rural, resectable non-small cell lung carcinoma patients, Cancer Genet. ((2022) ), 268-269: 75-82. doi: 10.1016/j.cancergen.2022.09.008. |
[74] | L.M. Hess, P.M. Krein, D. Haldane, Y. Han and A.N. Sireci, Biomarker Testing for Patients With Advanced/Metastatic Nonsquamous NSCLC in the United States of America, 2015 to 2021. Journal of Thoracic Oncology 3: ((2022) ). |