The perceived usability of the learning management system during the COVID-19 pandemic: Integrating system usability scale, technology acceptance model, and task-technology fit
Abstract
BACKGROUND:
The education system has shifted from traditional to online during the COVID-19 pandemic. Thus, the Learning Management System (LMS) is one of the most important and comprehensive learning platforms that support and facilitate online learning during this pandemic.
OBJECTIVE:
This study explored the perceived system usability of LMS during the COVID-19 pandemic by utilizing Technology Acceptance Model (TAM), Task-Technology Fit (TTF), and System Usability Scale (SUS).
METHODS:
An online survey was utilized to collect data from 502 Filipino students from different academic institutions and different areas of study.
RESULTS:
Structural Equation Modeling (SEM) indicated that students’ perceived ease of use of LMS had a significant effect on perceived usefulness which subsequently and positively led to students’ perceived satisfaction of LMS. In addition, LMS technology characteristics was found to have a significant effect on LMS task-technology fit which subsequently led to perceived usefulness and perceived satisfaction. Interestingly, perceived satisfaction was found to have a significant effect on students’ perceived system usability of LMS which was calculated using a System Usability Scale (SUS).
CONCLUSION:
The findings have implications within the education system globally, particularly in recognizing the relevance of LMS moving forward. Furthermore, since this is the first study that integrated SUS, TAM, and TTF, the conceptual framework can be utilized to evaluate the perceived usability of LMS worldwide.
1Introduction
During the COVID-19 pandemic, the education system has shifted from traditional to online [1–3]. Many countries have adopted the online delivery system to sustain learning despite the closure of schools and academic institutions due to physical distancing restrictions and other health protocols [2, 4]. In the Philippines, the Commission on Higher Education (CHED) has promoted and established guidelines for online learning [5].
Online learning is not new. It particularly pertains to any form of learning conducted and delivered via the internet [6]. While it has not been a formal and primary mode of learning, it has become popular over the past 10 years with the success of massive open online courses (MOOCs) [7]. Online learning is supported by modern technologies such as internet access and information and communication technologies (ICTs) that facilitate content delivery and other educational needs [8, 9]. The integration of ICTs and the need to strengthen teaching and learning channels have led to the development of Learning Management Systems (LMS) [6].
LMS is an educational software system or an application or a website that being utilized to deliver courses online and digitally [10]. Priss [11] describes LMS as a complex software system with many features that match today’s content delivery needs. Ndegeya [12] also mentioned that LMS has become a platform capable of delivering various educational content and information, such as school announcements and lectures and executing tasks such as exams, discussion boards, online assignments, and course registration [12]. Additionally, Mehrolia et al. [6] emphasize the importance and advantage of some LMSs having features for monitoring, feedback, data analytics, and reporting. Given the number of tasks that can be supported by the variety of features available in an LMS, Priss [11] states the importance of obtaining an in-depth understanding of the user preferences, usability, and other underlying concepts that support the learning process in such software system.
Even before the COVID-19 pandemic, studies on LMS have already been conducted and they have a number of gaps in research. These studies have taken different approaches. The majority of them have used specific factors such as users’ attitudes, satisfaction, and usability to understand and evaluate how effective LMS is for learning and how it is accepted and adopted by users. Nguyen and Mehloria et al. [6, 10] recommend future studies on LMS to consider and integrate more factors and theories to further the scientific understanding of LMS. For instance, Pal and Vanijja [13] suggest the integration of the system usability scale (SUS) with more constructs in different contexts to provide newer insights on and develop stronger standards for evaluating online learning platforms.
The development of online learning platforms, such as LMS, has been at a rapid pace [10]. Together with their rapid development, more educational institutions are investing in such technologies to sustain academic continuity even during times of crisis, such as the COVID-19 pandemic. Thus, studies exploring different perspectives of LMS and its users could give more insights on how to be well-prepared in emergency situations and still sustain learning integrity [14]. Since the impact of emergencies, like the COVID-19 pandemic could be global in scale, the recommendation of Mehloria et al. [6] to conduct studies on LMS in different and specific educational settings and contexts with different groups of respondents has become more relevant than ever.
This study aimed to evaluate the perceived usability of LMS during the COVID-19 pandemic by utilizing Technology Acceptance Model (TAM), Task-Technology Fit (TTF), and System Usability Scale (SUS). Different factors influencing Perceived Satisfaction of LMS to Perceived System Usability of LMS were considered such as perceived ease of use of LMS, perceived usefulness of LMS, LMS technology characteristics, LMS task-technology fit, and student’s attitudes toward using LMS in online learning during COVID-19. Structural equation modeling (SEM) was utilized to analyze the causal relationship among the different variables.
At a time when the education system has taken a drastic shift from traditional to online [15], this study will give more insights on the effectiveness of LMS in delivering educational and content needs and how it must be deployed and utilized to maximize its function and potential. It will also give insights on how LMS may be improved, and which aspects may be further developed to better suit students’ learning essentials and preferences. Furthermore, since this is the first study that integrated SUS, TAM, and TTF, the conceptual framework can be utilized to evaluate the perceived usability of LMS worldwide.
2Related literature and development of hypotheses
2.1Online learning and Learning Management System (LMS)
Pal and Vanijja [13] stated that studies on the overall experience and usability of online learning platforms are relevant, especially with the present circumstances brought by the COVID-19 pandemic. All over the world, educational and academic institutions have shifted from traditional to online learning. Online learning was a result of the advancement in ICTs and the progress in the delivery methods for higher education [16]. Through online learning, people have the ability to access their learning and educational needs anytime and from anywhere at their own pace [17].
Online learning has been a massive development in educational access and reach. According to studies, alongside the objective to improve educational access, the primary purpose of online learning is to improve the quality of learning with the use of existing technologies and tools and to reduce the cost of delivering educational needs to a wide range of people or the general mass [16, 18]. Even during COVID-19, online learning’s cost- and time-effectiveness have been found as positive aspects. Students specify that online learning saves them the cost and time to travel to and from the university and makes their daily routine more efficient [14, 19]. Aside from convenience and improved participation, safety, due to the health risk posed by COVID-19, are also positive aspects generated from online learning. According to existing literature, while some apprehension toward online learning with respect to motivation and language barrier still exists [19], perceptions of students, in general, are mostly positive [17, 19, 20]. These positive insights keep students and educators interested and invested enough to further dedicate research on online learning and the tools and technologies that support and facilitate it [13].
Tools, technologies, and platforms for online learning have become crucial aspects of the radical change brought by COVID-19 to the education sector [21]. The interest of students in using technologies for online learning has been sustained in order to accommodate different learning styles and needs in different settings and circumstances [13, 22, 23]. A variety of technologies and tools have been evaluated for online learning. Multimedia contents, such as animation, audio, image, video, and 3-D, have long been integrated with online learning. Studies suggest that multimedia technology positively influences learning as it delivers content in several ways that capture the interest of learners [22, 24, 25]. Social media is also an emerging tool used in online learning. Social media functions, such as virtual communication, knowledge formation, and documents exchange, bring positive outcomes to learning [26]. Virtual meeting platforms are also widely used in online learning as they also provide comprehensive features such as audio and video conferencing, chat, email invitations, polls, file sharing, screen sharing, recording, and annotation tools [27]. During the COVID-19 pandemic, virtual meeting platforms have been a crucial tool in upholding learning despite face-to-face restrictions. For instance, nursing students had high levels of satisfaction, student engagement, and academic self-efficacy upon using virtual meeting platforms for their academic requirements during COVID-19 [28]. Virtual meeting platforms are able to meet the students’ learning needs and support a variety of learning activities even during times of crisis, resonating with the fact that these technologies are a great part of the future of education [28–30].
Nguyen [10] states that among the technologies used for online learning today, LMS is regarded as one of the most important with respect to learning management and knowledge acquisition. LMS is the most comprehensive among these technologies as the features and tools present in multimedia technology, social media, and virtual meeting platforms are existent and well-integrated into this software system [12]. Aside from learning management features, learning analytics and reporting tools are also present in LMS [6, 31]. The plethora of features and tools available and integrated with LMS have strengthened its capabilities to successfully deliver courses, achieve knowledge acquisition, and control learning [32]. Convenience and access in communication and coordination, even at a global level, allow LMS administrators and governance boards to create a robust learning environment where user involvement and participation are high [6, 33]. LMS administrators and instructors also play an essential role in boosting the performance of users [34]. A robust learning environment through good governance leads to organizational acceptance. Thus, LMS also plays a crucial role in rapid organizational response to changes in the business and industry, which places the company in a strong competitive stance [33].
The crucial role LMS plays in organizational response is also underscored during COVID-19. In the study by Stickney et al. [35], a group of educators in the medical field and clinicians were able to reengineer surgical care process training for COVID-19 patients through LMS and its flexibility. As a result, team members were able to easily access and learn at their own pace, manage patients safely and prevent exposure in the workplace. While studies have shown the positive outcomes of LMS, the importance of its role in crucial and challenging times, and the ways in which it is evaluated and assessed continue to become complex and intricate due to differences in availability of features and tools in specific LMSs, learning needs, user groups, and areas or fields of study [10, 11]. To analyze effectivity of LMS, different tools such as the System Usability Scale, theories such as Task-Technology Fit and Technology Acceptance Model may be utilized.
2.2System Usability Scale (SUS)
A System Usability Scale (SUS) is an effective, quick, and cost-saving tool used to evaluate the usability of a certain product [36]. SUS has been used in a surplus of studies in different contexts [37, 38]. It was originally developed by John Brooke in 1986 to evaluate the usability of a wide range of products and services, from software products, such as websites and applications, to hardware ones, such as mobile and electronic devices [36, 38]. Its flexibility and popularity have led to studies exploring and proving the validity and reliability of SUS in a variety of fields. The research benefits from using SUS are it can be easily administered among respondents or participants, and it may be used on small sample sizes but still generate reliable results [36, 37, 39]. The SUS questionnaire consists of 10 items, alternating with one having a positive tone and the next item with a negative one [40]. The five statements in a positive tone and the other five negatives are all rated through a 5-point Likert scale: 5 –strongly agree, 4 –agree, 3 –neutral, 2 –disagree and 1 –strongly disagree. Generated SUS scores can range from 0 to 100, where higher scores indicate better usability. SUS has been widely used in information systems research, particularly in evaluating interactive systems [36]. With the rise of learning mediated and supported by tools, technologies, and software systems, SUS has also been used to evaluate online learning platforms with high levels of reliability [13]. Revythi and Tselios [41] considered SUS in evaluating e-learning acceptance while also integrating the Technology Acceptance Model. Their study showed how factors affecting satisfaction showed be explored more in order to highlight indicators affecting student acceptance. The extensive literature on the reliability and validity of SUS, its ease of use and availability have led to the use of SUS to measure the perceived system usability of LMS in this study alongside theories and proposed models from other studies.
2.3Satisfaction and Technology Acceptance Model (TAM)
Satisfaction is considered as one of the key elements in the evaluation and assessment of online learning [17, 42–44]. Satisfaction is a reflection of a student’s learning experience point of view, particularly how a certain technology or system satisfies information and learning needs [17, 45]. Studies show that students’ satisfaction is positively related to online learning effectiveness and establishing a robust learning environment [42, 46]. Consequently, students’ learning satisfaction is often affected by their technology acceptance, user experience, and quality of and support for learning [10, 17, 42, 47, 48].
As online learning is a delivery method mediated and facilitated by technology, Nguyen explored the factors that affect students’ satisfaction with LMS and found that system content, instruction information, interaction, and technology quality are positively related to students’ perceived LMS usefulness, which is students’ opinions on how LMS may be used to improve learning. In turn, perceived usefulness is found to be directly related to students’ perceived satisfaction, which is also evident in other studies [10, 49, 50]. Ease of use and user-friendliness encompass instruction information in Nguyen’s model [10]. Ease of use is described as the time spent finding any information or material on LMS, while user-friendliness pertains to how well the interface supports students in accomplishing tasks on LMS [10, 51]. Perceived usefulness and perceived ease of use are major factors in the Technology Acceptance Model (TAM). TAM was developed by Fred Davis, Richard Bagozzi and Paul Warshaw in 1989 and has become one of the most useful means to predict the use and acceptance of various forms of technologies [52]. With the favorable results of Nguyen’s study [10] and how some of its model’s elements are parallel to TAM, the students’ perceived ease of use, perceived usefulness, and perceived satisfaction constructs were adopted in this study with the following hypotheses:
H1. Students’ perceived ease of use of LMS positively influences students’ perceived usefulness of LMS.
H2. Students’ perceived usefulness of LMS positively influences student’s perceived satisfaction of LMS.
2.4Task-Technology Fit (TTF)
Task-Technology Fit (TTF) is an Information System (IS) theory developed by Goodhue and Thompson in 1995 and states that system use and performance are linked to system characteristics and how suitable a system is to the tasks that must be performed [22, 50, 51–55]. For instance, Park et al. [22] used TTF to explore the adoption of multimedia technology by students in learning. The study states that the characteristics of multimedia technology positively affect the students’ perceived fit in learning as multimedia technology characteristics capture the interest of students in various interesting ways. TTF has been used increasingly over the past decade due to the rapid rise and development of IS [53]. Education is a field that has seen a rise in the use of various forms of technology and IS. In online learning studies, perceived fit or simply TTF is often related to technology or system acceptance and adoption [50].
Aside from system acceptance and adoption, TTF is also considered a significant predictor of system perceived usefulness in online learning [56]. In addition, some studies state that an online learning system’s perceived fit in learning is associated with students’ satisfaction [50, 54, 56]. When it comes to the application of TTF to LMS studies, they state that TTF strongly influences learning with the use of LMS [54, 57]. Moreover, Al-Maatouk et al. [58] considered the integration of TTF and TAM in exploring the adoption of social media in Academia. It was seen that the overall integrated model showed a great fit in evaluating adoption in academe. Moreover, Vlachogianni and Tselios [59] integrated TTF and SUS for the evaluation of educational technology developers. In their study, they adopted the SUS for the evaluation of perceived usability. Considering the strong evidence of the links between TTF, perceived usefulness, and satisfaction in online learning, this study conceptualized the following hypotheses:
H3. The technology characteristics of LMS positively influence the fit in using LMS for online learning.
H4. The fit in using LMS for online learning positively influences students’ perceived usefulness of LMS.
2.5Theory of Reasoned Action (TRA) and attitudes
Theory of Reasoned Action (TRA) was developed by Fishbein and Ajzen in 1975 to explain the relationship between attitudes, behavioral intentions, and subjective norms. In the existing literature, TRA is found to be a relevant framework in exploring one’s attitude toward their behavior [58]. Fishbein and Ajzen [60] describes attitude as “a person’s general feeling of favorableness or unfavorableness toward some stimulus object”.
Attitudes have been explored in many studies on online learning [14, 61–63]. The strong links between attitudes and student satisfaction have been established in several studies [63–65]. Moreover, Liaw et al. [63] mention that students’ attitudes must be examined when structuring a web-based learning management system. Aligning students’ attitudes with the development of LMS has a high chance of LMS acceptance. As today’s LMSs are also available on mobile devices, studies have also shown that educators’ and students’ attitudes toward the use of mobile devices in learning are relatively positive [58, 61, 62]. Hussein et al.’s study [14] explored students’ attitudes toward online learning during the COVID-19 pandemic and found that students are positive toward online learning’s cost- and time- effectiveness, and safety aspects. Moreover, Jogezai et al. [58] used TRA to conceptualize the connection of COVID-19 with teachers’ attitudes toward the use of social media in online learning.
In TAM, which is also derived from TRA, perceived usefulness contributes to users’ attitudes toward the use of a certain technology [52]. With the significance of attitudes in TRA and TAM, this study adopted a construct on Jogezai’s model [58] that integrated users’ attitudes, COVID-19, and the use of a certain technology in online learning and conceptualized the following hypotheses:
H5. Students’ perceived usefulness of LMS positively influences students’ attitudes toward using LMS in online learning.
H6. Students’ attitudes toward using LSM in online learning positively influence students’ perceived satisfaction with LMS.
2.6Integrated research model
This study utilized SUS to measure the perceived system usability of LMS. Furthermore, the fit in using LMS for online learning, students’ attitudes, perceived usefulness, and perceived satisfaction of LMS are also explored by using TTF and TAM. Harrati et al. [66] explored student satisfaction for different e-learning systems using the SUS. Their study suggested utilizing subjective measurements to enhance the relationship with the metric scale. In accordance, Revythi and Tselios [41] utilized SUS with TAM for e-learning with results showing how stakeholders should focus more on factors affecting satisfaction to enhance effectiveness. As this study determined whether a relationship exists between measures of students’ perceived system usability of LMS and perceived satisfaction, it conceptualized the final hypothesis:
H7. Students’ perceived user satisfaction of LMS positively affects the perceived system usability.
Integrating all the factors discussed in the literature review and the conceptualized hypotheses, Fig. 1 shows the study’s integrated research model. Based on the studies by Revythi and Tselios [41], Al-Maatouk et al. [58], and Vlachogianni and Tselios [59], TAM, TTF, and SUS were integrated and utilized in this study.
Fig. 1
The integrated research model.
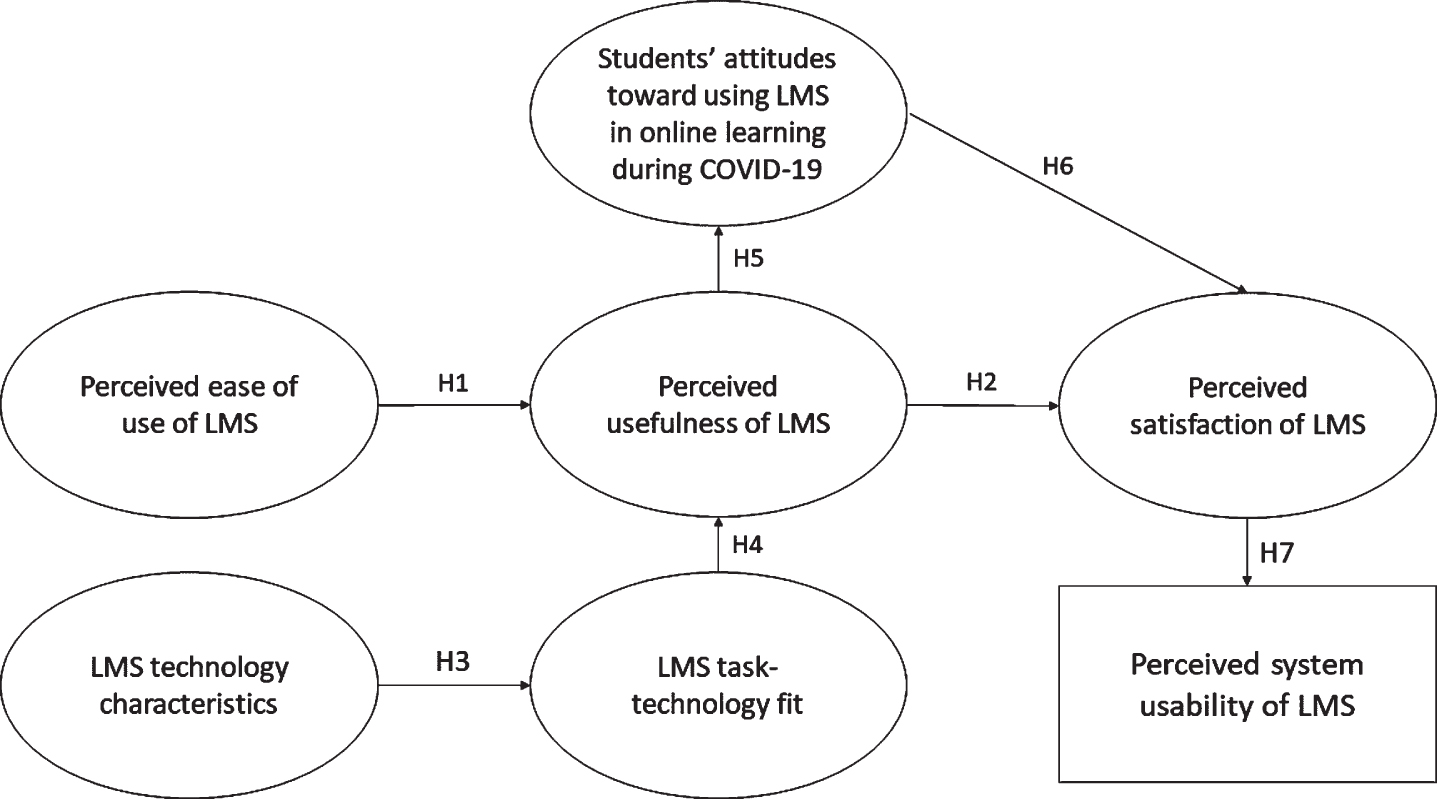
3Methodology
3.1Sample
The sample is composed of Filipino students from tertiary, graduate, and post-graduate levels in various areas and fields of study. Utilizing purposive sampling, a total of 502 valid responses were collected from Filipino students from different schools and universities across the Philippines. Purposive sampling was utilized in this study since this study is specific for students who are utilizing an online learning management system. While a bigger sample size generates more accurate testing, this study applied Nguyen’s sampling concept in his study [10], in which the acceptable sample size must be five times the number of items measured in the instrument. This questionnaire has 37 measured items in total. Thus, the ideal sample size for this study would be a minimum of 185 respondents. Alongside Lombard et al.’s sample size recommendation [68] of 200–300 for reliable results and Nguyen’s sampling concept [10], this study’s sample size of 502 was enough to generate results with high reliability.
The majority (57%) of the respondents were female, twenty-nine percent (29%) were male, ten (2%) of respondents preferred not to disclose their gender, while the non-binary and transgender females had one (0.2%) respondent each. The youngest respondent was 17 years old, while the oldest was 57 years old, providing a mean age of 22 years old (StD = 5.62). A big majority of the respondents (88%) were tertiary students, followed by the master (7%), and lastly, doctoral students (6%). The higher education student population in the Philippines is dominated by students from the tertiary level, which is reflected in the sample of this study [69].
Almost half of the respondents (47%) were taking programs under the Science, Technology, Engineering and Mathematics fields, followed by Health Sciences and Medicine (25%), Business and Management (14%), Social Sciences (7%), Law (3%), Education (2%), and lastly, Humanities with only eight respondents (2%).
3.2Quantitative instrument development
This study was approved by School of Industrial Engineering and Engineering Management Mapua University Research Ethics Committees (FM-RC-21-80). An online survey was used to gather data from the respondents. The questionnaire consisted of multiple-choice, checklists, and rating items. Before answering the survey, informed consent was asked among respondents to consider their responses for this study. In addition, the respondents had the option to withdraw at any given time. The online survey had five sections: (1) demographics, (2) attitudes, (3) perceived satisfaction, (4) task-technology fit and (5) system usability. The attitudes, perceived satisfaction, task-technology fit, and system usability sections all contained rating items. For the rating items, a 5-point Likert scale: 5 –strongly agree, 4 –agree, 3 –neutral, 2 –disagree and 1 –strongly disagree was used. Items for the attitudes toward using LMS in the online learning (AT) construct were based on the study by Jogezai et al. [58] that explored teachers’ attitudes toward the use of social media in online learning during COVID-19. Moreover, items for the perceived ease of use of LMS (PE), perceived usefulness of LMS (PU) and perceived satisfaction of LMS (PS) were based on Nguyen’s study [10] on the factors influencing students’ satisfaction toward LMS. On the other hand, LMS technology characteristics (TC) and LMS task-technology fit (TF) constructs’ items were taken from ChongWoo et al.’s study [22] that used TTF for the adoption of multimedia technology in learning and McGill & Klobas’ study [57] on task-technology fit and learning management system. Lastly, items for LMS perceived system usability (SU) measurement were taken from the standard SUS questionnaire [40]. Table 2 shows the summary of the questions included in the quantitative measurement instrument which was approved by the School of Industrial Engineering and Engineering Management Mapua University Research Ethics Committee.
Table 1
Demographic profile of respondents
Variable | Categories | Frequency | % |
Gender | Female | 284 | 56.6 |
Male | 206 | 41.0 | |
Prefer not to say | 10 | 2.0 | |
Non-binary | 1 | 0.2 | |
Transgender female | 1 | 0.2 | |
Age | 16–25 years old | 457 | 91.0 |
26–35 years old | 21 | 4.2 | |
36–45 years old | 16 | 3.2 | |
46–55 years old | 7 | 1.4 | |
56 years old (Over) | 1 | 0.2 | |
Level of study | Tertiary | 439 | 87.5 |
Master’s | 34 | 6.8 | |
Doctorate | 29 | 5.8 | |
Area of study | Sciences, Technology, Engineering, Mathematics | 235 | 46.8 |
Health Sciences, Medicine | 126 | 25.1 | |
Business, Management | 71 | 14.1 | |
Social Sciences (Anthropology, Economics, Geography, Political Science, Psychology) | 37 | 7.4 | |
Law | 13 | 2.6 | |
Education | 12 | 2.4 | |
Humanities (Performing Arts, Visual Arts, Communication, Languages and literature, Philosophy) | 8 | 1.6 |
Table 2
Quantitative measurement instrument
Construct | Item | Question | Reference |
Perceived ease of use of LMS | PE1 | It only takes me a little time to get adept with the system/software. | [10] |
PE2 | Instruction information is detailed and clear. | [10] | |
PE3 | I could get quick support whenever I encounter any problem or issue with LMS. | [10] | |
PE4 | I could easily use LMS even during my first time. | [10] | |
PE5 | All guidelines are designed in an understandable and convenient structure. | [10] | |
PE6 | All functions are running smoothly. | [10] | |
Perceived usefulness of LMS | PU1 | LMS is extremely useful to my studies. | [10] |
PU2 | LMS largely affects my study results and outcomes. | [10] | |
PU3 | LMS helps me get informed of school announcements and updates. | [10] | |
PU4 | LMS effectively assists me in taking exams and other assessments. | [10] | |
Perceived satisfaction of LMS | PS1 | I feel that using LMS increases my learning. | [57] |
PS2 | I feel that using LMS well serves my crucial studying requirement. | [10] | |
PS3 | I feel satisfied using LMS. | [10] | |
PS4 | I think there is NOTHING to improve with LMS. | [10] | |
LMS technology characteristics | TC1 | The content of the lecture and learning materials is relevant. | [22] |
TC2 | The content of the lecture and learning materials is accurate. | [22] | |
TC3 | The content of the lecture and learning materials is concise and straight to the point. | [22] | |
TC4 | The content of the lecture and learning materials is comprehensible. | [22] | |
TC5 | Overall, the lecture and learning materials are of good quality. | [22] | |
LMS task-technology fit | TF1 | I believe that using LMS supports my learning well in online and hybrid arrangements. | [22] |
TF2 | I believe that using LMS helps me complete my learning tasks. | [56] | |
TF3 | I believe that using LMS is appropriate for learning in online and hybrid arrangements. | [22] | |
TF4 | I believe that using LMS matches the content delivery in online and hybrid arrangements. | [22] | |
Attitudes toward using LMS in online learning during COVID19 | AT1 | The use of LMS for online learning during COVID-19 is good for me. | [57] |
AT2 | To me, using LMS for online learning during COVID-19 is important. | [57] | |
AT3 | With the use of LMS in online learning, my skills and knowledge will improve. | [57] | |
AT4 | My skills and knowledge development are important. | [57] | |
Perceived system usability of LMS | SU1 | I think I would like to use LMS frequently. | |
SU2 | I found LMS unnecessarily complex. | ||
SU3 | I thought LMS was easy to use. | ||
SU4 | I think I need the support of a technical person to be able to use LMS. | ||
SU5 | I found the various functions of LMS well integrated. | ||
SU6 | I thought there was too much inconsistency in this application. | ||
SU7 | I would imagine that most people would learn to use LMS quickly. | ||
SU8 | I found LMS tricky to use. | ||
SU9 | I felt confident using LMS. | ||
SU10 | I needed to learn a lot of things before I could get going with LMS. |
3.3Procedure
The survey was distributed and delivered online from June 27— July 8, 2021, through Google Forms, an online web-based survey administration software. The survey questionnaire provided an introduction to the purpose of the study and prior to each section. A pilot survey was initially conducted with two students for further and possible improvement of the questionnaire and other content of the survey. The two students who participated in the pilot survey were no longer part of the actual survey. The online link to the survey was personally sent to some respondents, shared in online classes, and posted on various social media sites, such as Facebook and LinkedIn, to gather more respondents. Statistical software packages, such as Google Sheets, Microsoft Excel, and Statistical Package for the Social Sciences software acquired by the International Business Machine Corporation (IBM SPSS), were used to analyze, group, and manage the quantitative survey results and SUS scores. Additionally, IBM SPSS Analysis of Moment Structures (AMOS) was used to construct, run and analyze the structural equation model of this study.
3.4Statistical analysis
Structural equation modeling (SEM) using IBM SPSS AMOS, a software program used to fit and analyze structural equation models, was chosen to analyze the proposed hypotheses and integrated research model of this study. AMOS analyzes and measures complex cause-effect relationship models with and among latent variables, constructs, and indicators through estimation.
For the perceived system usability of LMS, a single indicator (SU) represented it and was correlated with the PS construct. The overall SUS score was the measurement used for the SU indicator, which was considered as an observed variable (represented by a square from Fig. 1). Primarily, the SUS score contribution for individual items ranges from 0 to 4. Items 1, 3, 5, 7, and 9 were items with a positive tone, so each of these item’s score contribution is the scale position subtracted by 1. On the other hand, for the negatively worded items (2, 4, 6, 8, and 10), the score contribution is 5 subtracted by the scale position. Finally, for the overall SUS score, each item’s score contribution is multiplied by 2.5 and summed up. As a result, generated overall SUS scores range from 0 to 100.
SUS scores were interpreted through Sauro and Lewis’s Curved Grading Scale (CGS) [70]. The CGS approach has been extensively used in SUS studies. The CGS grading method provides a robust way of empirically interpreting overall SUS scores. Table 3 shows the CGS grading scheme.
Table 3
The curved grading scale for SUS scores
SUS score range | Grading | Percentile range |
84.1–100 | A+ | 96–100 |
80.8–84.0 | A | 90–95 |
78.9–80.7 | A– | 85–89 |
77.2–78.8 | B+ | 80–84 |
74.1–77.1 | B | 70–79 |
72.6–74.0 | B– | 65–69 |
71.1–72.5 | C+ | 60–64 |
65.0–71.0 | C | 41–59 |
62.7–64.9 | C– | 35–40 |
51.7–62.6 | D | 15–34 |
0.0–51.6 | F | 0–14 |
This result was then utilized as a dependent observed variable to measure the system overall usability. The other figures presented are considered unobserved variable, represented by the ovals. These are influenced by different factors coming from the construct adapted from related studies to measure each factor such as LMS technology characteristics, perceived ease of use of LMS, Student’s attitude towards using LMS in online learning during COVID-19, perceived usefulness of LMS, LMS task-technology fit, and perceived satisfaction of LMS.
4Results and discussion
4.1LMS perceived system usability
The students’ perceived system usability of LMS was measured using SUS. This study considered the usability of LMS in general and not specific LMS software systems or applications. Table 4 shows the SUS score analytic values of LMS.
Table 4
LMS SUS overall score
Indicator | Min Score | MaxScore | Mean Score | StD | Min Grade | Max Grade | Mean Grade |
SU | 22.50 | 97.50 | 59.30 | 10.97 | F | A+ | D |
Note 1. SU = LMS perceived system usability.
With a mean score of 59.30 and an equivalent grade of D within the CGS grading scheme, the perceived system usability of LMS was low. This result signifies Filipino students have low regard for the system usability of LMS in online learning. At this point, LMS is not seen as an effective or usable platform to use in online learning. It should be noted, however, that respondents rated different LMS software systems, depending on which they use, or which is available in their respective academic institutions. The variety of LMS software may be a factor for the low perceived system usability score, which presents a drawback of this study. While LMS becomes more relevant in the education system, the ways in which it is assessed still present challenges due to the differences of specific LMS software systems and their complexity [10, 11]. In line with the results from Prasetyo et al. [71], LMS should consider factors such as perceived ease of use, user interface, system, and information quality which would lead to students’ acceptability. In this regard, similar results were found and are included in the discussion.
4.2Latent construct
The relevant analytics values of the measurement model of the research, particularly of the latent constructs, are shown in Table 5.
Table 5
The relevant analytic values of the measurement model of the research
Constructs | Item | Mean | StD | Loadings* |
Perceived ease of use of LMS | PE1 | 3.93 | 0.797 | 0.708 |
PE2 | 3.46 | 1.043 | 0.719 | |
PE3 | 3.82 | 0.912 | 0.624 | |
PE4 | 3.69 | 0.965 | 0.779 | |
PE5 | 3.94 | 0.797 | 0.803 | |
PE6 | 4.00 | 0.814 | 0.648 | |
Perceived usefulness of LMS | PU2 | 4.07 | 0.944 | 0.712 |
PU3 | 4.09 | 0.815 | 0.710 | |
PU4 | 3.69 | 0.964 | 0.716 | |
Perceived satisfaction of LMS | PS1 | 3.48 | 1.051 | 0.828 |
PS3 | 3.45 | 1.007 | 0.747 | |
LMS technology characteristics | TC1 | 4.28 | 0.722 | 0.707 |
TC2 | 4.12 | 0.742 | 0.810 | |
TC3 | 3.93 | 0.901 | 0.831 | |
TC4 | 4.00 | 0.829 | 0.867 | |
TC5 | 3.98 | 0.866 | 0.883 | |
LMS task-technology fit | TF1 | 3.88 | 0.926 | 0.833 |
TF2 | 4.04 | 0.803 | 0.832 | |
TF3 | 3.90 | 0.922 | 0.787 | |
Attitudes toward using LMS in online learning during COVID-19 | AT1 | 3.58 | 1.075 | 0.810 |
AT2 | 4.18 | 0.792 | 0.655 | |
AT3 | 3.52 | 1.083 | 0.813 |
Note 1. Items (AT4, PU1, PS2, PS4 and TF4) were removed from the instrument due to their lower values of factor loading. Note 2. StD = Standard Deviation, AT = Attitudes toward using LMS in online learning, PE = Perceived ease of use of LMS, PU = Perceived usefulness of LMS, PS = Perceived satisfaction of LMS, TC = LMS technology characteristics, TF = LMS task-technology fit. Note 3. *p < 0.001.
4.2.1Perceived ease of use LMS
Items PE1–PE6 showed the Filipino students’ perceived ease of use of LMS. Overall, the composite mean score for this construct was 3.81. This result signifies that most Filipino students agree that LMS is easy to use, particularly in finding information or any relevant material on the software and in executing tasks in a quick and timely manner. As item PE6 (All functions are running smoothly) had the highest mean score, it shows how the LMS interface contributes to perceived ease of use [10, 52]. Similar to the results found by Ong et al. [72], students prefer ease of use as one of the important attributes during online learning. Consequently, this factor was seen to be the highest significant effect before [73] and even during the COVID-19 pandemic [71]. It could be deferred that students consider the ease of use of an LMS for their perception in its usability.
4.2.2Perceived usefulness of LMS
Filipino students’ perceived usefulness of LMS was represented through items PU2–PU4. This construct had a composite mean score of 3.95. The mean score indicates that Filipino students have positive opinions on how LMS may be used to improve their learning. Students widely consider LMS crucial in attaining study outcomes and essential in getting informed of school activities as items PU2 (LMS largely affects my study results and outcomes.) and PU4 (LMS helps me get informed of school announcements and updates.) scored 4.07 and 4.09, respectively. During the COVID-19 pandemic, LMS was seen to be the most useful tool for delivering education off-site. Ibrahim et al. [74] and Mailizar et al. [75] discussed how perceived usefulness was seen to be one of the highest significant factors affecting students’ satisfaction. It could be deduced that even if LMS is useful during the COVID-19, students still suffer a great deal of challenges due to the sudden shift in the mode of learning.
4.2.3Perceived satisfaction of LMS
Items PS1 and PS3 represented Filipino students’ perceived satisfaction with LMS. With a composite mean score of 3.47, Filipino students are neutral but leaning toward agreement when it comes to their perceived satisfaction with LMS. This result still positively means students are quite satisfied with LMS and how it increases their learning. Baharin et al. [42] explained that student satisfaction progresses as they utilize such tools and technologies for online learning in the long run. Additionally, among the latent constructs, PS had the lowest mean score, which contributed to the low LMS SUS score (59.30).
4.2.4LMS technology characteristics
The technology characteristics of LMS for online learning were represented in the TC construct. Items TC1–TC5 had a composite mean score of 4.06, the highest among the latent constructs. The high mean score pertains to Filipino students’ strong positive views on the content and information materials available within and through LMS. The relevance of LMS content and information materials contributes greatly to this construct as item TC1 (The content of the lecture and learning materials is relevant.) generated the highest mean for this construct with 4.28. Ong et al. [72] showed how the contents are part of the preferences of students during the online learning. Moreover, Prasetyo et al. [73] explained how interactivity also plays a significant role in students’ acceptance of an LMS.
4.2.5LMS task-technology fit
Items TF1–TF3 showed the fit in using LMS for online learning. This construct represents how suitable LMS is for online learning. The construct’s composite mean score of 3.94 indicates that Filipino students highly consider LMS a suitable platform for online learning. Task-technology fit is often considered to have a strong influence on perceived usefulness [22, 56]. This finding was reflected in the proximity of the composite mean scores of this and the PU constructs with 3.94 and 3.95, respectively. Being the main learning platform during COVID-29, LMS proves to be essential in accomplishing learning tasks as students gave item TF2 (I believe that using LMS helps me complete my learning tasks.) the highest mean score (4.04) for this construct.
4.2.6Attitudes toward using LMS in online learning
The attitudes of Filipino students toward using LMS in online learning was reflected in items AT1–AT3. With a composite mean score of 3.76 for this construct, Filipino students are positively inclined to use and accept LMS for online learning, especially during the COVID-19 pandemic. This attitude is highly evident in item AT2 (To me, using LMS for online learning during COVID-19 is important.) as students gave it a mean score of 4.18. This finding emphasizes and strengthens the role of technology, particularly LMS, in sustaining learning during emergency situations, such as the COVID-19 [14, 58].
4.3The structural research model analysis and hypothesis testing
As shown in Fig. 2, the structural research model and its direct effects were examined through path coefficients and their significance levels by running the bootstrapping method. To which, the insignificant relationship (p > 0.050) of student attitude towards using LMS in online learning during the COVID-19 pandemic on satisfaction was removed [71]. In addition, the nonsignificant factors (<0.50) were also removed. The model was run to present the final SEM as represented by Fig. 3. In addition, the validity and reliability of the constructs were examined. As seen in Table 6, the Cronbach’s alpha and Composite reliability are greater than the threshold of 0.7 [71–73], while the Average Variance Extracted (AVE) has values greater than 0.50. This indicates that the constructs and latent considered are acceptable [76, 77].
Fig. 2
Analysis of direct effects in the structural research model.
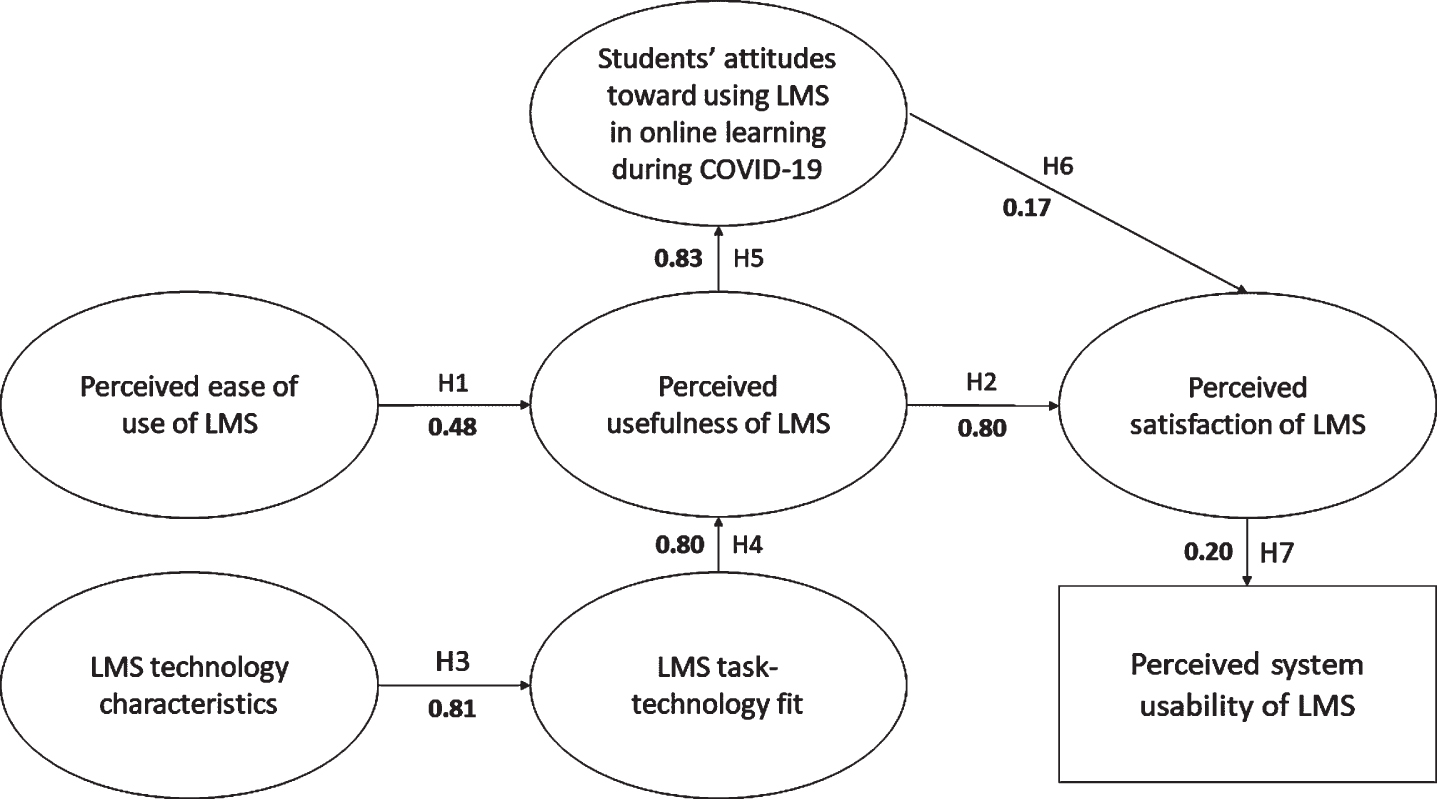
Fig. 3
The final SEM for factors affecting perceived usability of Learning Management System.
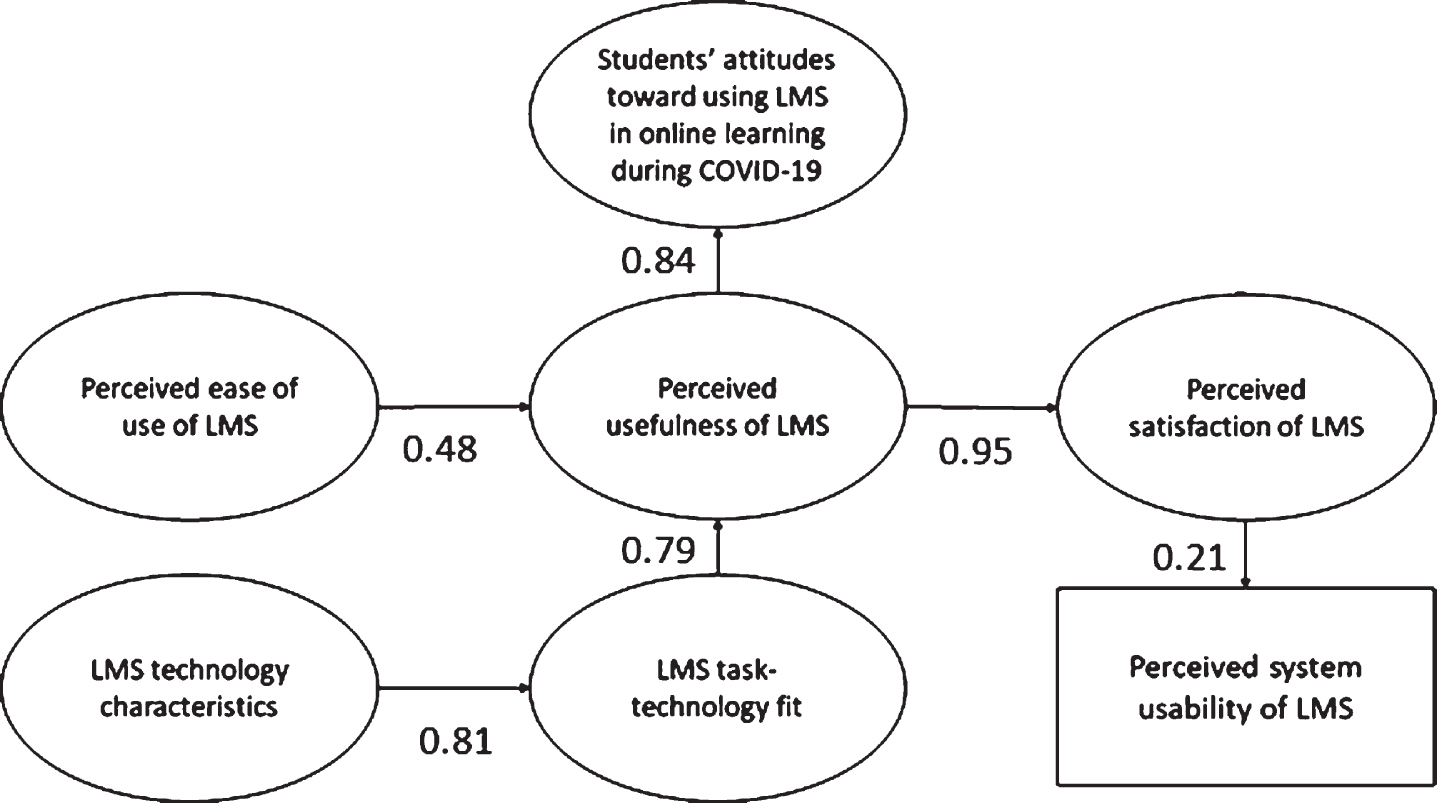
Table 6
Composite reliability and validity
Factor | Cronbach’s α | Composite Reliability (CR) | Average Variance Extracted (AVE) |
Perceived ease of use | 0.856 | 0.863 | 0.513 |
Perceived usefulness | 0.779 | 0.756 | 0.508 |
Perceived satisfaction | 0.823 | 0.766 | 0.622 |
LMS technology characteristics | 0.914 | 0.912 | 0.676 |
LMS task – technology fit | 0.856 | 0.858 | 0.668 |
Attitude towards using LMS | 0.714 | 0.805 | 0.582 |
Six of seven paths in the model were significant, supporting H1 (β= 0.48, t = 8.496, p < 0.05), H2 (β= 0.95, t = 7.045, p < 0.05), H3 (β= 0.81, t = 16.469, p < 0.05), H4 (β= 0.79, t = 10.650, p < 0.05), H5 (β= 0.84, t = 10.412, p < 0.05) and H7 (β= 0.21, t = 4.244, p < 0.05). On the other hand, H6 (β= 0.17, t = 1.789, p > 0.05) was insignificant and therefore not supported. Table 7 summarizes the hypothesis test results. Moreover, Table 8 reflects the direct, indirect, and total effects of the model.
Table 7
Path coefficient and hypothesis testing
Hypothesis | Path | Path coefficient (β) | T-Value | P-Value | Decision |
H1 | PE ->PU | 0.48 | 8.496 | 0.001 | Supported |
H2 | PU ->PS | 0.95 | 7.045 | 0.001 | Supported |
H3 | TC ->TF | 0.81 | 16.469 | 0.001 | Supported |
H4 | TF ->PU | 0.79 | 10.650 | 0.001 | Supported |
H5 | PU ->AT | 0.84 | 10.412 | 0.001 | Supported |
H6 | AT ->PS | 0.17 | 1.789 | 0.074 | Not supported |
H7 | PS ->SU | 0.21 | 4.244 | 0.001 | Supported |
Table 8
Direct, indirect, and total effects
No | Variable | Direct Effect | P-Value | Indirect Effect | P-Value | Total Effect | P-Value |
1 | TC ⟶ TF | 0.813 | 0.001 | – | – | 0.815 | 0.001 |
2 | TF ⟶ PU | 0.790 | 0.001 | – | – | 0.786 | 0.001 |
3 | PE ⟶ PU | 0.475 | 0.001 | – | – | 0.500 | 0.001 |
4 | PU ⟶ AT | 0.842 | 0.001 | – | – | 0.823 | 0.001 |
5 | PU ⟶ PS | 0.953 | 0.001 | 0.122 | 0.001 | 0.941 | 0.003 |
6 | PS ⟶ SU | 0.208 | 0.001 | – | – | 0.201 | 0.001 |
7 | TC ⟶ PU | – | – | 0.640 | 0.001 | 0.640 | 0.001 |
8 | TC ⟶ AT | – | – | 0.527 | 0.001 | 0.527 | 0.001 |
9 | TC ⟶ PS | – | – | 0.602 | 0.001 | 0.602 | 0.001 |
10 | TC ⟶ SU | – | – | 0.121 | 0.001 | 0.121 | 0.001 |
11 | TF ⟶ AT | – | – | 0.647 | 0.001 | 0.647 | 0.001 |
12 | TF ⟶ PS | – | – | 0.739 | 0.001 | 0.739 | 0.001 |
13 | TF ⟶ SU | – | – | 0.149 | 0.001 | 0.149 | 0.001 |
14 | PE ⟶ AT | – | – | 0.411 | 0.001 | 0.411 | 0.001 |
15 | PE ⟶ PS | – | – | 0.470 | 0.001 | 0.470 | 0.001 |
16 | PE ⟶ SU | – | – | 0.094 | 0.001 | 0.094 | 0.001 |
17 | PU ⟶ SU | – | – | 0.189 | 0.001 | 0.189 | 0.001 |
18 | AT ⟶ SU | – | – | 0.030 | 0.001 | 0.030 | 0.001 |
With a path coefficient of 0.48, H1 was supported, thus students’ perceived ease of use of LMS positively influences the perceived usefulness of LMS. This finding is in line with TAM’s proposition Bagozzi [52] and Nguyen’s study [10]. Perceived ease of use positively contributes to perceived usefulness [10, 22]. Next, the perceived usefulness of LMS strongly and positively influences students’ perceived satisfaction of LMS [10, 49, 50] as H2 generated a path coefficient of 0.80. The link between perceived usefulness and satisfaction LMS is further strengthened by the ease of use aspects, such as LMS instruction information and support. Several studies emphasize how information quality and online support service are strongly related to student satisfaction [50, 78, 79].
Moving over to H3, with a path coefficient of 0.081, LMS technology characteristics is proven to have a positive influence on the fit in using LMS for online learning. This finding is consistent with TTF’s proposition [80]. The characteristics of a certain technology always remain crucial to task-technology fit or how suitable a certain technology is to the tasks the user must perform [22, 50, 53–55]. Furthermore, H4 had a path coefficient of 0.80, which suggests how the fit in using LMS for online learning significantly and positively influences students’ perceived usefulness of LMS [22, 54, 57]. This finding is not surprising as task-technology fit and perceived usefulness are quite similar in their respective postulations.
In addition, LMS technology characteristics also contribute to the relationship between LMS task-technology fit and perceived usefulness [79]. Subsequently, LMS task-technology fit is also a factor in the strong relationship (H2: β= 0.80) between students’ perceived usefulness and perceived satisfaction of LMS as task-technology fit is found to have significant effects on student satisfaction in several studies [50, 54, 56].
The next hypothesis, which establishes the influence of students’ perceived usefulness of LMS on their attitudes toward using LMS in online learning, was supported. With a path coefficient of 0.83, H5 confirms the strong and positive influence of perceived usefulness on the Filipino students’ attitudes, or favorableness in general, toward the use of LMS in online learning. TAM’s proposition [52], and other studies’ findings [50, 61] on the relationship between perceived usefulness, attitudes, and intentions toward the use of a certain technology are reflected in this study. On the other hand, H6 (β= 0.17, p > 0.05) was not supported.
H6 was not considered significant. This finding indicates that the influence and impact of Filipino students’ attitudes toward using LMS in online learning on their perceived satisfaction of LMS are insignificant. This study’s result with this aspect is in contrast with several studies showing the significance of students’ attitudes toward satisfaction their learning satisfaction [63–65]. The constructs indicate that they do not find it good to utilize LMS during the COVID-19 pandemic, they perceived that individual knowledge and skills will not improve, and have difficulty developing their knowledge and skills when utilizing LMS for learning. Ku et al. [65] explained how students perceived learning without groupmates or classmates around is difficult among students. Similarly, both studies of Liaw et al. [63] and Heflin et al. [64] explained that there will be a positive attitude among students when system function, collaboration tasks, learner characteristics, system acceptance, and satisfaction are taken into consideration when implementing LMS. As explained by Heflin et al. [64], it could also be taken into consideration that students have already adopted the set-up and dwell more on the completion of tasks rather than the technology being utilized. Thus, to highlight a positive attitude among students, the factors explained could be taken into consideration to enhance satisfaction when using an LMS.
Finally, with a path coefficient of 0.20, H7 was supported. While not as high as the other five supported and accepted paths, the final hypothesis generated a result significant enough to assert that Filipino students’ perceived satisfaction of LMS positively affects their perceived system usability of LMS. This finding further supports that satisfaction is one of the key elements in evaluating online learning, alongside the tools and technologies that support and facilitate it [17, 45]. After all, student satisfaction plays a significant role in forming an effective and robust learning system [42, 46]. The low perceived system usability score of LMS (mean score = 59.30, mean grade = D) of Filipino students, however, may have been a huge factor in this hypothesis’ path coefficient result just going a little above the significant threshold.
Table 9 shows the fit indices values of the structural research model. Based on the recommended values for a good fit by Gefen and Boudreau [82], the structural research model was accepted and indicated a good fit for the data tested with GFI, AGFI, TLI, CFI, and IFI values greater than 0.80. Moreover, RMSEA with values less than 0.07 was said to be acceptable as indicated by Steiger [83]. The results of the fit indices values of the structural research model signify its reliability.
Table 9
Fit indices result versus recommended values
Goodness of fit measures of SEM | Parameter estimates | Minimum cut-off | Suggested by |
Incremental Fit Index (IFI) | 0.876 | >0.80 | [82] |
Tucker Lewis Index (TLI) | 0.854 | >0.80 | [82] |
Comparative Fit Index (CFI) | 0.876 | >0.80 | [82] |
Goodness of Fit Index (GFI) | 0.848 | >0.80 | [82] |
Adjusted Goodness of Fit Index (AGFI) | 0.806 | >0.80 | [82] |
Root Mean Square Error (RMSEA) | 0.065 | <0.07 | [83] |
5Implications
5.1Theoretical contributions
While studies on online learning have been widely conducted, this study further advances the literature on online learning and the technologies that support and facilitate it, particularly on LMS. First, this study evaluated students’ perceived system usability of LMS using SUS. Filipino students, however, had given a low SUS score on the perceived system usability of LMS. Second, this study developed a model integrating SUS, TAM, and TTF. Specifically, students’ perceived system usability of LMS, alongside their perceived satisfaction of LMS, perceived usefulness, attitudes, and LMS task-technology fit were explored in pursuit to deepen the knowledge on the different underlying factors in the evaluation and assessment of LMS. Furthermore, this study also explored how emergency situations, such as COVID-19, affect students’ use of and perceptions of LMS.
The findings of the study support the notions of how perceived usefulness and the fit in using LMS for online learning positively influence students’ perceived satisfaction with LMS. No significant link, however, was found between students’ attitudes toward using LMS during COVID-19 and their perceived satisfaction as opposed to previous research [63–65]. Most importantly, this study contributes to the knowledge and insights establishing the link between the perceived system usability of LMS and student satisfaction, recognizing the importance of student satisfaction in online learning assessment [17, 45]. The relationship between perceived system usability and student satisfaction, influenced by perceived usefulness and task-technology fit, has not been explored in previous studies. Remarkably, the findings in this study establish a significant relationship between student satisfaction and perceived system usability.
While this study focused on the Filipino educational and academic context, it still offers a step further toward understanding the underlying factors that support learning, especially at the time when the education system has taken a drastic shift from traditional to online delivery. The concept and analysis this study has developed may be used and adopted in further studies on the use and of certain technologies in online learning.
5.2Practical implications
The study holds relevant practical implications, especially during this time when emergency situations, such as the COVID-19 pandemic, have caused major disruptions in the education system globally. The new and relevant findings from this study may prompt educators to put considerable importance on how students’ learning is supported and is carried out through online platforms and various technologies. During the time in which learning arrangements and the learning process must be flexible and resilient enough to keep students engaged, educators, academic professionals, and students should work together to bring out the best learning outcomes even during uncertain circumstances.
The different perceptions of students on the learning process, especially their satisfaction, are key elements in the effectiveness of online learning [17, 45]. For students to be satisfied with the use of LMS, it must be proven useful to the learning tasks they must perform within the software system and, in turn, impactful to their study results and outcomes. LMS developers play a crucial role in this undertaking as majority of the software system’s usefulness, ease of use, and characteristics are rooted from the availability and complexity of LMS features [12]. The availability and capability of LMS features must be supported by a smooth and easy-to-navigate interface as it also contributes highly to the perceived usefulness of such technology. Furthermore, as LMS is a complex software system in nature, exceptional support service strongly contributes to how inclined and satisfied students are with LMS [50, 74, 75].
Aside from LMS features and support service, the quality and relevance of the content and other learning materials within LMS must also be given significant attention and detail by instructors and teachers. Students tend to have more positive insights with the use of LMS if the overall quality of available content is excellent and relevant. Finally, educators, instructors, and LMS developers could make use of the feedback and practical implications to further improve the potential and capabilities of LMS in delivering educational needs even during emergency situations and uncertain circumstances.
6Conclusion
The results reveal that Filipino students’ perceived system usability of LMS, utilizing SUS, is low. This study asserts that students’ perceived satisfaction with LMS has a positive and significant influence on perceived system usability. Specifically, Filipino students’ perceived satisfaction with LMS is positively influenced by their perceived usefulness, while their attitudes toward using it in online learning during COVID-19 do not. Furthermore, perceived ease of use and LMS task-technology fit, which is strongly influenced by LMS technology characteristics, significantly and positively influence students’ perceived usefulness of LMS. Considering the factors integrated with this study with regard to the use of LMS and the results generated thereafter, it is clear that LMS is a major part of sustaining learning within the education system going forward.
6.1Limitations and recommendations
While this study integrated several factors related to the use of LMS, this study has a few limitations and drawbacks. First, it should be noted that this study considers LMS in general and not specific LMS software systems. The variety of LMS software systems rated by the students may be a factor for the low perceived system usability score given by Filipino students According to Nguyen [10] and Priss [11], the variety of features and tools available in different LMS software systems presents a constant challenge on how LMS is evaluated and assessed. Second, this study is conducted among Filipino students from tertiary, graduate and post-graduate levels from local academic institutions. Third, only students’ perceptions are explored in this study. Examining the insights and perceptions of instructors and would enrich the understanding of LMS as they are also major users of the software system.
With the limitations present in this study, recommendations include the application of this study’s concept and analysis in other cultures or in specific academic institutions and student groups to further understand how LMS is perceived in various settings [82, 83]. Allocating more resources and time to gather more data from a larger sample size could also further establish the propositions made in this study. Moreover, modifications in the structural research model to better examine the underlying concepts [87–90] and relationships among latent constructs related to the use of LMS are welcome as LMS becomes more relevant to the future of online learning and education.
Ethical approval
This study was approved by the School of Industrial Engineering and Engineering Management Mapua University Research Ethics Committees (FM-RC-21-80).
Informed consent
Informed consent was obtained from all subjects involved in the study.
Conflict of interest
The authors declare no conflict of interest.
Acknowledgments
The authors would like to extend their deepest gratitude to the respondents of this study despite the COVID-19 pandemic.
Funding
This research was funded by Mapúa University Directed Research for Innovation and Value Enhancement (DRIVE).
References
[1] | Clark AE , Nong H , Zhu H , Zhu R . Compensating for academic loss: Online learning and student performance during the COVID-19 pandemic. China Economic Review. (2021) ;68: :101629. |
[2] | UNESCO. COVID-19 Educational Disruption and Response: UNESCO; 2020 [Available from: https://en.unesco.org/covid19/educationresponse. |
[3] | Zheng F , Khan NA , Hussain S . The COVID 19 pandemic and digital higher education: Exploring the impact of proactive personality on social capital through internet self-efficacy and online interaction quality. Children and Youth Services Review. (2020) ;119: :105694. |
[4] | Organization WH. Q&A on coronaviruses (COVID-19): www.who.int; 2020 [Available from: https://www.who.int/emergencies/diseases/novel-coronavirus-2019/questionand-answers-hub/q-a-detail/q-a-coronaviruses. |
[5] | Hernando-Malipot M , San Juan A Learning in the time of coronavirus: Manila Bulletin; 2020 [Available from: https://mb.com.ph/2020/03/11/learning-in-the-time-of-coronavirus/. |
[6] | Mehrolia S , Alagarsamy S , Sabari MI . Moderating effects of academic involvement in web-based learning management system success: A multigroup analysis. Heliyon. (2021) ;7: (5):e07000. |
[7] | Hidalgo FJP , Abril CAH . MOOCs: Origins, concept and didactic applications: A systematic review of the literature –Technology, Knowledge and Learning (2020) ;25: (4):853–79. |
[8] | Lloyd M , Towards a definition of the integration of ICT in the classroom. AARE’05 Education Research Creative Dissent: Constructive. 2006:1-18. |
[9] | Lew S-L , Lau S-H , Leow M-C . Usability factors predicting continuance of intention to use cloud e-learning application. Heliyon. (2019) ;5: (6):e01788. |
[10] | Nguyen N-T A study on satisfaction of users towards learning management system at International University–Vietnam National University HCMC. Asia Pacific Management Review. 2021. |
[11] | Priss U . A Preliminary Semiotic-Conceptual Analysis of a Learning Management System. Procedia Computer Science. (2020) ;176: :3702–9. |
[12] | Ndegeya RM Comparison of Learning Management Systems. 2019. |
[13] | Pal D , Vanijja V . Perceived usability evaluation of Microsoft Teams as an online learning platform during COVID-19 using system usability scale and technology acceptance model in India. Children and youth services review. (2020) ;119: :105535. |
[14] | Hussein E , Daoud S , Alrabaiah H , Badawi R . Exploring undergraduate students’ attitudes towards emergency online learning during COVID- A case from the UAE. Children and youth services review. (2020) ;119: :105699. |
[15] | Lin SH , Lee H-C , Chang C-T , Fu CJ . Behavioral intention towards mobile learning in Taiwan, China, Indonesia, and Vietnam. Technology in Society.. (2020) ;63: :101387. |
[16] | Dang YM , Zhang YG , Ravindran S , Osmonbekov T . Examining student satisfaction and gender differences in technology-supported, blended learning. Journal of Information Systems Education. (2016) ;27: (2):119. |
[17] | Alqurashi E . Predicting student satisfaction and perceived learning within online learning environments. Distance Education. (2019) ;40: (1):133–48. |
[18] | Hamidi H , Chavoshi A . Analysis of the essential factors for the adoption of mobile learning in higher education: A case study of students of the University of Technology. Telematics and Informatics.. (2018) ;35: (4):1053–70. |
[19] | Fidalgo P , Thormann J , Kulyk O , Lencastre JA . Students’ perceptions on distance education: A multinational study. International Journal of Educational Technology in Higher Education. (2020) ;17: :1–18. |
[20] | Baber H . Modelling the acceptance of e-learning during the pandemic of COVID-19-A study of South Korea. The International Journal of Management Education. (2021) ;19: (2):100503. |
[21] | Abidah A , Hidaayatullaah HN , Simamora RM , Fehabutar D , Mutakinati L . The impact of covid-19 to indonesian education and its relation to the philosophy of “merdeka belajar”. Studies in Philosophy of Science and Education. (2020) ;1: (1):38–49. |
[22] | Park C , Kim D-G , Cho S , Han H-J . Adoption of multimedia technology for learning and gender difference. Computers in Human Behavior. (2019) ;92: :288–96. |
[23] | Tseng H , Walsh EJ Jr . Blended vs. traditional course delivery: Comparing students’ motivation, learning outcomes, and preferences. Quarterly Review of Distance Education. (2016) ;17: (1):1–21. |
[24] | Abdulrahaman M , Faruk N , Oloyede A , Surajudeen-Bakinde N , Olawoyin L , Mejabi O , et al., Multimedia tools in the teaching and learning processes: A systematic review. Heliyon. (2020) ;6: (11):e05312. |
[25] | Gümüs S , Okur MR . Using multimedia objects in online learning environment. Procedia-Social and Behavioral Sciences. (2010) ;2: (2):5157–61. |
[26] | Hosen M , Ogbeibu S , Giridharan B , Cham T-H , Lim WM , Paul J , Individual motivation and social media influence on student knowledge sharing and learning performance: Evidence from an emerging economy. Computers&Education. 2021:104262. |
[27] | Nanos AG , James AE , editors. A virtual meeting system for the new age. 2013 IEEE 10th International Conference on e-Business Engineering; 2013: IEEE. |
[28] | Luo Y , Geng C , Pei X , Chen X , Zou Z , The evaluation of the distance learning combining webinars and virtual simulations for senior nursing students during the COVID-19 period. Clinical Simulation in Nursing. 2021. |
[29] | Bhargava S , Negbenebor N , Sadoughifar R , Ahmad S , Kroumpouzos G , Virtual conferences and e-learning in dermatology duringCOVID-19 pandemic: Results of a webbased, global survey. Clinics in Dermatology. 2021. |
[30] | Giddens J , Curry-Lourenco K , Miles E , Reeder E . Enhancing learning in an online doctoral course through a virtual community platform. Journal of Professional Nursing. (2021) ;37: (1):184–9. |
[31] | Duin AH , Tham J . The current state of analytics: Implications for learning management system (LMS) use in writing pedagogy. Computers and Composition. (2020) ;55: :102544. |
[32] | Petrina S . Sidney Pressey and the automation of education, -Technology and Culture (2004) ;45: (2):305–30. |
[33] | Martin K , Quigley MA , Rogers S . Implementing a learning management system globally: An innovative change management approach. IBM Systems Journal. (2005) ;44: (1):125–45. |
[34] | Elfeky AIM , Masadeh TSY , Elbyaly MYH . Advance organizers in flipped classroom via e-learning management system and the promotion of integrated science process skills. Thinking Skills and Creativity. (2020) ;35: :100622. |
[35] | Stickney I , Schoenbrod T , Cushing H , Grosvenor J , Cushing B . Rapid development and deployment of a learning management system to train an interprofessional team to manage surgery for a COVID-19–positive patient. The Joint Commission Journal on Quality and Patient Safety. (2021) ;47: (5):313–7. |
[36] | Anam H , Sadiq M , Jamil H . Development of system usability scale (SUS) for the urdu language. International Journal of Computer Science and Information Security (IJCSIS). (2020) ;18: (6):73–8. |
[37] | Bangor A , Kortum PT , Miller JT . An empirical evaluation of the system usability scale. Intl Journal of Human–Computer Interaction. (2008) ;24: (6):574–94. |
[38] | Lewis JR . . Usability: Lessons learned. . . and yet to be learned. International Journal of Human-Computer Interaction. (2014) ;30: (9):663–84. |
[39] | Peres SC , Pham T , Phillips R , editors. Validation of the system usability scale (SUS) SUS in the wild. Proceedings of the Human Factors and Ergonomics Society Annual Meeting; (2013) SAGE Publications Sage CA: Los Angeles, CA. |
[40] | Brooke J . SUS-A quick and dirty usability scale. Usability Evaluation in Industry. (1996) ;189: (194):4–7. |
[41] | Revythi A , Tselios N . Extension of technology acceptance model by using system usability scale to assess behavioral intention to use e-learning. Education and Information Technologies. (2019) ;24: (4):2341–55. |
[42] | Baharin AT , Lateh H , mohd Nawawi H , Nathan SS . Evaluation of satisfaction using online learning with interactivity. Procedia-Social and Behavioral Sciences. (2015) ;171: :905–11. |
[43] | Shih H-F , Chen S-HE , Chen S-C , Wey S-C . The relationship among tertiary level EFL students’ personality, online learning motivation and online learning satisfaction. Procedia-Social and Behavioral Sciences. (2013) ;103: :1152–60. |
[44] | Yang L , Bian Y , Zhao X , Liu X , Yao X . Drivers’ acceptance of mobile navigation applications: An extended technology acceptance model considering drivers’ sense of direction, navigation application affinity and distraction perception. International Journal of Human-Computer Studies. (2021) ;145: :102507. |
[45] | Ives B , Olson MH , Baroudi JJ . The measurement of user information satisfaction. Communications of the ACM. (1983) ;26: (10):785–93. |
[46] | Zhan Z , Mei H . Academic self-concept and social presence in face-to-face and online learning: Perceptions and effects on students’ learning achievement and satisfaction across environments. Computers & Education. (2013) ;69: :131–8. |
[47] | Ghazal S , Aldowah H , Umar I , editors. Critical factors to learning management system acceptance and satisfaction in a blended learning environment. International Conference of Reliable Information and Communication Technology; Springer, (2017) . |
[48] | Richardson JC , Maeda Y , Lv J , Caskurlu S . Social presence in relation to students’ satisfaction and learning in the online environment: A meta-analysis. Computers in Human Behavior. (2017) ;71: :402–17. |
[49] | Gao BW , Jiang J , Tang Y . The effect of blended learning platform and engagement on students’ satisfaction— — the case from the tourism management teaching. Journal of Hospitality, Leisure, Sport & Tourism Education. (2020) ;27: :100272. |
[50] | Lin W-S , Wang C-H . Antecedences to continued intentions of adopting e-learning system in blended learning instruction: A contingency framework based on models of information system success and task-technology fit. Computers & Education. (2012) ;58: (1):88–99. |
[51] | Farhan W , Razmak J , Demers S , Laflamme S . E-learning systems versus instructional communication tools: Developing and testing a new e-learning user interface from the perspectives of teachers and students. Technology in Society. (2019) ;59: :101192. |
[52] | Bagozzi RP . The legacy of the technology acceptance model and a proposal for a paradigm shift. Journal of the Association for Information Systems. (2007) ;8: (4):3. |
[53] | Furneaux B . Task-technology fit theory: A survey and synopsis of the literature. Information systems theory: Springer. (2012) )87–106. |
[54] | Isaac O , Aldholay A , Abdullah Z , Ramayah T . Online learning usage within Yemeni higher education: The role of compatibility and task-technology fit as mediating variables in the IS success model. Computers & Education. (2019) ;136: :113–29. |
[55] | Lu H-P , Yang Y-W . Toward an understanding of the behavioral intention to use a social networking site: An extension of task-technology fit to social-technology fit. Computers in Human Behavior. (2014) ;34: :323–32. |
[56] | Lee DY , Lehto MR . User acceptance of YouTube for procedural learning: An extension of the Technology Acceptance Model. Computers & Education. (2013) ;61: :193–208. |
[57] | McGill TJ , Klobas JE . A task–technology fit view of learning management system impact. Computers & Education. (2009) ;52: (2):496–508. |
[58] | Al-Maatouk Q , Othman MS , Aldraiweesh A , Alturki U , Al-Rahmi WM , Aljeraiwi AA . Task-technology fit and technology acceptance model application to structure and evaluate the adoption of social media in Academia. IEEE Access. (2020) ;8: :78427–40. |
[59] | Vlachogianni P , Tselios N , Perceived usability evaluation of educational technology using the system usability scale (SUS): A systematic review. Journal of Research on Technology in Education. 2021;1-18. |
[60] | Jogezai NA , Baloch FA , Jaffar M , Shah T , Khilji GK , Bashir S . Teachers’ attitudes towards social media (SM) use in online learning amid the COVID-19 pandemic: The effects of SM use by teachers and religious scholars during physical distancing. Heliyon. (2021) ;7: (4):e06781. |
[61] | Fishbein M , Ajzen I . Belief, attitude, intention, and behavior: An introduction to theory and research. Philosophy and Rhetoric. (1977) ;10: (2). |
[62] | Al-Emran M , Elsherif HM , Shaalan K . Investigating attitudes towards the use of mobile learning in higher education. Computers in Human Behavior. (2016) ;56: :93–102. |
[63] | Heflin H , Shewmaker J , Nguyen J . Impact of mobile technology on student attitudes, engagement, and learning. Computers & Education. (2017) ;107: :91–9. |
[64] | Liaw S-S , Chen G-D , Huang H-M . Users’ attitudes toward Web-based collaborative learning systems for knowledge management. Computers & Education. (2008) ;50: (3):950–61. |
[65] | Ku H-Y , Tseng HW , Akarasriworn C . Collaboration factors, teamwork satisfaction, and student attitudes toward online collaborative learning. Computers in human Behavior. (2013) ;29: (3):922–9. |
[66] | Yawson DE , Yamoah FA . Understanding satisfaction essentials of E-learning in higher education: A multi-generational cohort perspective. Heliyon. (2020) ;6: (11):e05519. |
[67] | Harrati N , Bouchrika I , Tari A , Ladjailia A . Exploring user satisfaction for e-learning systems via usage-based metrics and system usability scale analysis. Computers in Human Behavior. (2016) ;61: :463–71. |
[68] | Lombard M , Snyder-Duch J , Bracken CC . Content analysis in mass communication: Assessment and reporting of intercoder reliability. Human Communication Research. (2002) ;28: (4):587–604. |
[69] | Higher Education Enrollment by Program Level and Sex: AY 2019-20 Program Level Male Female Total: Commission on Higher Education; 2020 [Available from: https://ched.gov.ph/wp-content/uploads/Higher-Education-Enrollment-by-Program-Level-and-Sex-AY-2019-20.pdf. |
[70] | Sauro J , Lewis JR , Quantifying the user experience: Practical statistics for user research: Morgan Kaufmann; (2016) . |
[71] | Prasetyo YT , Ong AK , Concepcion GK , Navata FM , Robles RA , Tomagos IJ , et al., Determining factors affecting acceptance of e-learning platforms during the COVID-19 pandemic: Integrating Extended Technology Acceptance Model and Delone & McLean is success model. Sustainability. (2021) ;13: (15):8365. |
[72] | Ong AK , Prasetyo YT , Young MN , Diaz JF , Chuenyindee T , Kusonwattana P , et al., Students’ preference analysis on online learning attributes in industrial engineering education during the COVID-19 pandemic: A conjoint analysis approach for sustainable industrial engineers. Sustainability. (2021) ;13: (15):8339. |
[73] | Prasetyo YT , Tumanan SA , Yarte LA , Ogoy MC , Ong AK , Blackboard e-learning system acceptance and satisfaction among Filipino high school students: An extended technology acceptance model (TAM) approach. 2020 IEEE International Conference on Industrial Engineering and Engineering Management (IEEM). 2020. |
[74] | Ibrahim NK , Al Raddadi R , AlDarmasi M , Al Ghamdi A , Gaddoury M , AlBar HM , et al., Medical students’ acceptance and perceptions of e-learning during the covid-19 closure time in King Abdulaziz University, Jeddah. Journal of Infection and Public Health. (2021) ;14: (1):17–23. |
[75] | Mailizar M , Burg D , Maulina S . Examining university students’ behavioural intention to use e-learning during the COVID-19 pandemic: An extended tam model. Education and Information Technologies. (2021) ;26: (6):7057–77. |
[76] | Ong AK , Cleofas MA , Prasetyo YT , Chuenyindee T , Young MN , Diaz JF , et al., Consumer behavior in clothing industry and its relationship with open innovation dynamics during the COVID-19 pandemic. Journal of Open Innovation: Technology, Market, and Complexity. . (2021) ;7: (4):211. |
[77] | Ong AK , Prasetyo YT , Salazar JM , Erfe JJ , Abella AA , Young MN , et al. Investigating the acceptance of the reopening Bataan Nuclear Power Plant: Integrating Protection Motivation Theory and extended theory of planned behavior. Nuclear Engineering and Technology. 2021. |
[78] | Lee J-W . Online support service quality, online learning acceptance, and student satisfaction. The Internet and Higher Education. (2010) ;13: (4):277–83. |
[79] | Lee SJ , Srinivasan S , Trail T , Lewis D , Lopez S . Examining the relationship among student perception of support, course satisfaction, and learning outcomes in online learning. The Internet and Higher Education. (2011) ;14: (3):158–63. |
[80] | Goodhue DL , Thompson RL , Task-technology fit and individual performance. MIS Quarterly. 1995:213-36. |
[81] | Cheng Y-M . Towards an understanding of the factors affecting m-learning acceptance: Roles of technological characteristics and compatibility. Asia Pacific Management Review. (2015) ;20: (3):109–19. |
[82] | Gefen D , Straub D , Boudreau M-C . Structural equation modeling and regression: Guidelines for research practice. Communications of the Association for Information Systems. (2000) ;4: (1):7. |
[83] | Steiger JH . Understanding the limitations of global fit assessment in structural equation modeling. Personality and Individual Differences. (2007) ;42: (5):893–8. |
[84] | Abbasi MS , Ahmed N , Sajjad B , Alshahrani A , Saeed S , Sarfaraz S , et al., E-learning perception and satisfaction among health sciences students amid the COVID-19 pandemic. Work. (2020) ;67: (3):549–56. |
[85] | Shabila NP , Alkhateeb NE , Dauod AS , Al-Dabbagh A . Exploring the perspectives of medical students on application of e-learning in medical education during the COVID-19 pandemic. Work. (2021) ;70: (3):751–62. |
[86] | Naddeo A , Califano R , Fiorillo I . Identifying factors that influenced wellbeing and learning effectiveness during the sudden transition into elearning due to the COVID-19 lockdown. Work. (2021) ;68: (1):45–67. |
[87] | Prasetyo YT , Montenegro LD , Nadlifatin R , Kurata YB , Ong AK , Chuenyindee T . The influence of organizational commitment on the perceived effectiveness of virtual meetings by Filipino professionals during the COVID-19 pandemic: A structural equation modeling approach. Work. (2022) ;71: (1):19–29. |
[88] | Navarro MM , Prasetyo YT , Young MN , Nadlifatin R , Redi AA . The perceived satisfaction in utilizing learning management system among engineering students during the COVID-19 pandemic: Integrating Task Technology Fit and extended technology acceptance model. Sustainability. (2021) ;13: (19):10669. |
[89] | Kishimoto RT , Prasetyo YT , Persada SF , Redi AA . Filipino generation z on mobile legends during COVID- A determination of playtime and satisfaction. International Journal of Information and Education Technology. (2021) ;11: (8):381–6. |
[90] | Ong AK , Prasetyo YT , Pinugu JN , Chuenyindee T , Chin J , Nadlifatin R , Determining factors influencing students’ future intentions to enroll in chemistry-related courses: Integrating self-determination theory and theory of planned behavior. International Journal of Science Education. 2022;1-23. |