Clinical perspectives on serum tumor marker use in predicting prognosis and treatment response in advanced non-small cell lung cancer
Abstract
The optimal positioning and usage of serum tumor markers (STMs) in advanced non-small cell lung cancer (NSCLC) care is still unclear. This review aimed to provide an overview of the potential use and value of STMs in routine advanced NSCLC care for the prediction of prognosis and treatment response. Radiological imaging and clinical symptoms have shown not to capture a patient’s entire disease status in daily clinical practice. Since STM measurements allow for a rapid, minimally invasive, and safe evaluation of the patient’s tumor status in real time, STMs can be used as companion decision-making support tools before start and during treatment. To overcome the limited sensitivity and specificity associated with the use of STMs, tests should only be applied in specific subgroups of patients and different test characteristics should be defined per clinical context in order to answer different clinical questions. The same approach can similarly be relevant when developing clinical applications for other (circulating) biomarkers. Future research should focus on the approaches described in this review to achieve STM test implementation in advanced NSCLC care.
1Introduction
Lung cancer is the leading cause of cancer-related deaths worldwide, since more than 50% of patients are diagnosed at advanced disease stage where curative treatment is no option for most patients [1–4]. Lung cancer has historically been divided into non-small cell lung carcinoma (NSCLC) and small cell lung carcinoma (SCLC), accounting for approximately 85% and 15% of all established lung cancer cases, respectively [3]. For advanced stage NSCLC patients, treatment has revolutionized and significantly improved overall survival in the last decade with the introduction of small molecule inhibitors (SMIs) and immune checkpoint inhibitors (ICIs) [5, 6]. Other new treatment modalities (e.g., antibody-drug conjugates (ADCs), cytokine therapy, chimeric antigen receptor (CAR)-T cell therapy, etc.), are emerging, augmenting the treatment landscape for this subgroup of patients [7].
The arrival of these novel treatment options enables personalized medicine, but also increases the need for treatment guidance for the clinician in order to make the most optimal treatment decisions. To aid the decision-making process, there is a clinical need for tools that enable the prediction of prognosis and treatment response. The prediction of prognosis can be defined as prediction of the probable or expected development of a disease, including whether and how quickly symptoms will improve, worsen, or remain stable over time, regardless of treatment [8] (Fig. 1). Estimating prognosis can be useful for the selection of patients for a certain treatment [8]. Prediction of treatment response on the other hand, will give information about the effect of a therapeutic intervention [8]. From a clinical point of view, the main interest is to predict which patients will not respond to treatment or who will develop treatment resistance during course of treatment since this requires change in therapeutic strategy. Treatment response prediction can be subdivided into primary treatment response and secondary treatment resistance [9]. In case of primary non-response, patients do not respond to the initially given treatment. In secondary treatment resistance, patients relapse after a period of initial response to treatment due to the development of tumor evasion mechanisms [9]. For both scenarios, early identification of resistance is crucial to adjust treatment, avoid the loss of valuable time and occurrence of undesirable adverse events, and reduce unnecessary treatment costs. The definition of early primary treatment response prediction refers to prediction before the first moment of radiological response assessment (T1), which is often after six weeks of treatment [10]. Early identification of secondary treatment resistance on the other hand, refers to identification of treatment resistance before clinical and/or radiological progression is observed after an initial clinical and/or radiological response (Fig. 1). Which biomarker is most suitable to predict prognosis and to identify this primary and/or secondary progression, however, is still an open question.
Fig. 1
Timeline illustrating the definitions of prediction of (a) prognosis, (b) early primary treatment response, and (c) secondary treatment resistance.
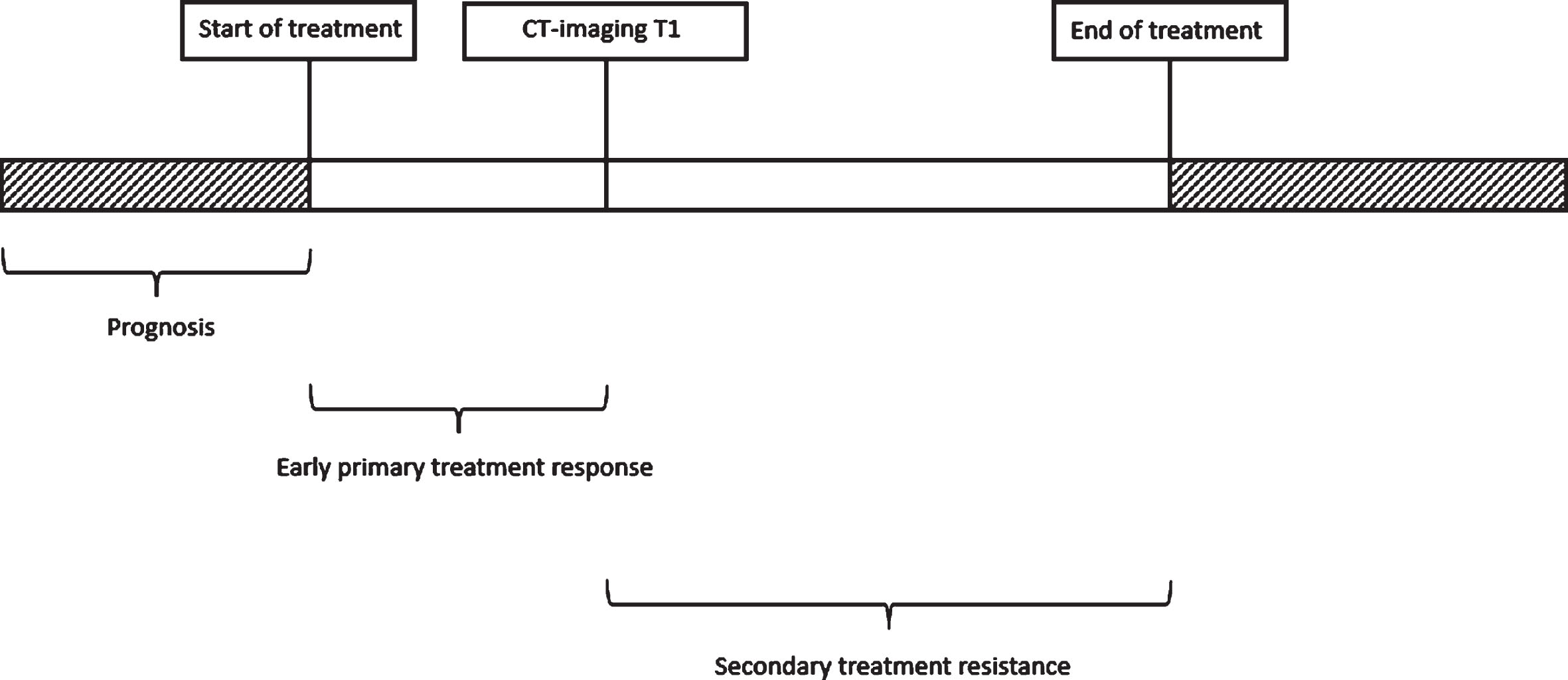
Serum tumor markers (STMs) are circulating protein-based molecules that are produced by tumor cells or other cells of the body in response to cancer or certain benign diseases [11, 12]. Their serum level alterations have shown to reflect tumor mass, making them valuable in predicting prognosis and evaluating response to treatment during follow-up. Results have shown that CA 125 antigen (CA-125), carcinoembryonic antigen (CEA), Cytokeratin 19 fragment (Cyfra 21.1), and Squamous Cell Carcinoma antigen (SCC-Ag) are associated with NSCLC disease [13, 14]. However, the optimal positioning and usage in daily clinical practice is still unclear since studies often investigated small and heterogenous patient populations, included patients with comorbidities that can influence the STM levels, and used different non-harmonized measurement systems and reference values for STM concentrations (unpublished data,15). Furthermore, there is a lack of comparison with other existing prognostic and predictive biomarkers to assess the added value of STM level measurements (unpublished data).
This review aims to highlight the unmet needs in current practice in prediction of (a) prognosis, (b) early treatment response, and (c) secondary treatment resistance in advanced NSCLC patients from a clinician’s perspective. Moreover, we will address STM implementation requirements and challenges. Finally, we will discuss the value of STMs as decision-making support tools in advanced NSCLC patients as available in daily clinical practice.
2Unmet needs in current practice in prediction of prognosis and response to treatment
2.1Prediction of prognosis
Currently, prognosis prediction in NSCLC patients is predominantly based upon TNM stage, histology, gender, age, and performance status [16]. These characteristics, however, have not shown to be ultimate in predicting the outcome for the individual patient due to tumor heterogeneity and immune response heterogeneity of the host [17]. As a consequence, patients may not receive proper treatment and be under- or overtreated for their disease. Undertreatment, which is defined by insufficient treatment of the disease, may result in an increased risk of mortality, deterioration in quality of life, and increased health care costs [18]. Overtreatment on the other hand, which is defined by excessive dosing or too frequent treatment, may additionally cause an increased risk in treatment-related toxicities [19]. Therefore, there is an urgent need to identify biological variables that directly reflect the disease process during course of disease and that can be easily implemented in routine care. The clear advantage of the implementation of such variables would be the facilitation of shared decision-making, patient education and counselling, and the improvement of personalized treatment within subgroups of advanced NSCLC patients.
2.2Prediction of early treatment response
Regardless of the type of treatment, the gold standard for response assessment in advanced NSCLC patients is based on tumor dynamics observed with radiological imaging using the Response Evaluation Criteria in Solid Tumors (RECIST) (version 1.1) [20]. However, these dynamics are reliant on human measurement, which is rather subjective, and can only be observed with a delay of several weeks after initiation of systemic treatment [21]. Furthermore, treatment-specific limitations of early radiological response assessment have also been identified. In patients treated with SMIs, a decrease in tumor density has also shown to reflect response to treatment, even in the absence of a decrease in size of the target lesions [22]. For patients treated with ICIs, inappropriate discontinuation of treatment can be the result of (a) a response after an initial increase in the total tumor burden, also called pseudoprogression, or (b) a response in the presence of new lesions, also called mixed response [20]. These limitations highlight that radiological imaging does not suffice to guide early clinical decision-making and its standalone use in daily clinical practice may result in relatively late and potential inappropriate (dis)continuation of treatment. Therefore, there is a need for a predictive biomarker with rapid kinetics that directly reflects a tumor’s clinical and biological behaviour to be able to determine response early after start of treatment.
2.3Prediction of secondary treatment resistance
In the follow-up of advanced NSCLC treatment, radiological imaging also plays a prominent role in response assessment. However, repeated radiological assessment is time-consuming, costly, and results in a significant increase in radiation exposure for the patient [20, 22]. In literature, a probability of 30% was reported of misclassifying the presence of tumor progression, resulting in ineffective treatment continuation in almost one-third of patients [23]. RECIST 1.1-specific limitations additionally include (a) the issue of non-measurable disease (e.g. due to pleural effusion or bone metastases), (b) the lack of visualization of extra-thoracic disease activity when performing a thoracic CT-scan, and (c) unreliable response assessment at short follow-up intervals (from three to four weeks) [10]. In daily clinical practice, approximately one-third of all advanced NSCLC patients are not evaluable for RECIST 1.1 assessment during follow-up of treatment and can therefore not be monitored accurately (unpublished data of the authors). Furthermore, radiological assessment may not correspond to the clinical benefit experienced by the patient (e.g. patients radiologically categorized as stable disease according to RECIST 1.1, but having progressive symptoms [24–26]). These drawbacks highlight the need for a predictive monitoring biomarker that is able to predict development of progressive disease during treatment, in order to enable an early treatment switch.
3Requirements and challenges to implement STMs as biomarker tests
The biomarker development process is a multiphase process that can be schematically subdivided into pre-analytical validation, analytical validation, clinical validation, demonstration of clinical utility, and regulatory approval [27]. For predictive biomarkers, clinical validity refers to the ability of a predictive assay to reliably divide the patient population into distinct groups with different expected outcomes to a specific treatment. The validation criteria are determined by the question that the biomarker must address and mainly comprise (pre)analytical stability and accuracy, predetermined clinical sensitivity/specificity criteria, reproducibility, and cut-off point on the receiver operating characteristics (ROC) curve of the assay. The optimal cut-off point of the assay is the point on the ROC curve corresponding to a positive (PPV) and negative predictive value (NPV) best suited to the clinical context. This cut-off point should be externally validated in an independent dataset reflecting the intended target population before obtaining regulatory approval. For the individual patient, PPVs and NPVs are the most valuable test characteristics, since these statistics consider the prevalence of a condition and therefore allow the clinician to estimate how likely it is an individual patient has this specific condition investigated by the biomarker [27, 28]. For prognostic biomarkers, it is necessary to evaluate the effect of an interaction between the biomarker and treatment, and use of the biomarker should subsequently result in a significant difference in survival between distinct groups with different expected outcomes to a specific treatment [27]. The clinical utility corresponds to the question whether the results of the biomarker support specific decisions or actions that result in improvement of patient overall survival. This step is carried out under the rationale that the methods used for biomarker assessment are predetermined and that the clinical validation results confirm the prognostic and predictive ability of the marker [27].
An ideal tumor marker should have the following characteristics: (a) it should be highly specific to a given tumor type, (b) it should be highly sensitive to avoid false positive results, (c) it should provide a lead-time over clinical diagnosis, (d) its levels should correlate with tumor burden, (e) it should have a short half-life, (f) the test should be relatively simple and cheap, and (g) specimens should be easily obtained [29]. STMs in advanced NSCLC have shown to approximately meet most of the above-mentioned criteria, since their serum levels may precede tumor dynamics observed by radiological imaging, their concentration tends to reflect the extent of tumor mass or the presence of occult metastatic disease, most of them have a half-life between hours and days, they are easy and inexpensive to analyse, and they can be easily obtained by peripheral blood draw (Fig. 2) [30–33]. However, STMs are not specific for NSCLC disease and may also be elevated in patients with benign comorbidities and in case of treatment-related toxicities [34–36]. Furthermore, limiting sensitivity is a challenge, since STMs have shown to be mainly elevated in a subgroup of patients based on histological type (e.g. adenocarcinoma), tumor extension (e.g. advanced stage disease), and tumor mutational status (e.g. EGFR mutations), reaching exclusively higher sensitivity rates in these specific subgroup populations of advanced NSCLC patients [37]. Due to the limited sensitivity and specificity, the lack of prospective validation in large patient cohorts, and the lack of comparison with other existing prognostic and predictive biomarkers, current guidelines do not offer any consensus recommendations for the use of STMs in prognosis prediction and therapeutic monitoring of lung cancer [13, 38].
Fig. 2
Early assessment of secondary progression.
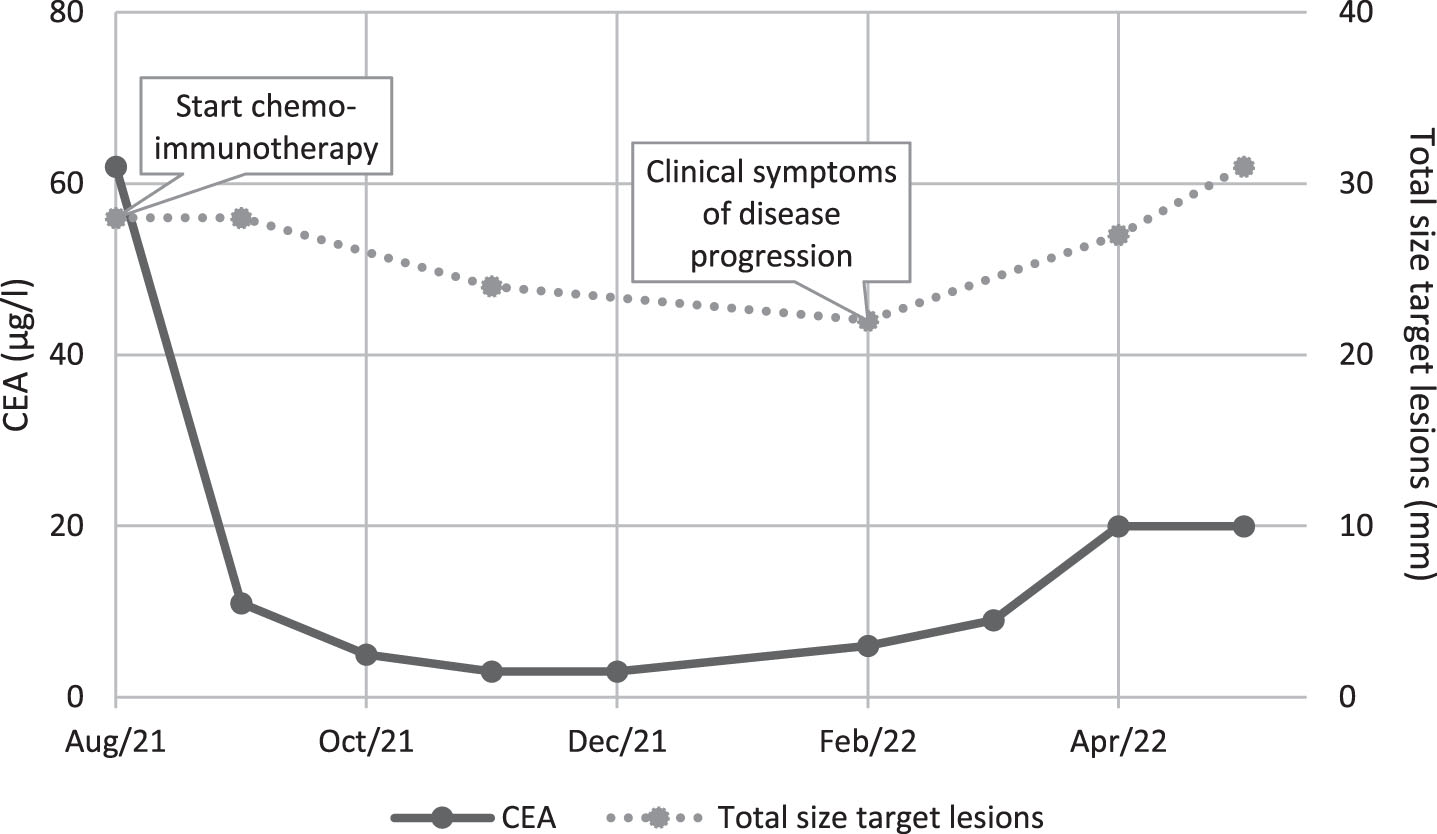
4STMs as decision support tools for advanced NSCLC in daily clinical practice
In order to solve the unmet needs in prediction of prognosis and response to treatment, different test-specific characteristics are requested dependent on which clinical question has to be answered. Here, we describe several clinical scenarios to illustrate which test characteristics are needed and which approaches can be used to obtain these test characteristics.
4.1Prediction of prognosis
Prognostic biomarkers inform the clinician about an increased likelihood of a certain clinical outcome, allowing for the selection of therapy or intensity of monitoring during treatment [8]. To be used as prognostic biomarkers, STMs should be able to differentiate patients based on survival outcome. Additionally, STMs can be used as therapeutic prognostic classifiers if they are able to identify a subset of patients who have a good prognosis when undergoing a certain treatment [39]. In patients diagnosed with stage III disease for example, higher STM levels may identify patients with a significant worse prognosis [40]. These patients could potentially benefit from stage IV treatment (e.g., with SMIs or ICIs) (Fig. 3A). The same approach could be applied for patients achieving radiological complete remission, partial response, or stable disease during treatment (Fig. 3B). Identifying which patients could benefit from additional treatment (e.g., dual ICI therapy, radiotherapy, chemotherapy) due to the presence of micrometastases, should lead to a significantly improved survival rate in each subgroup of patients. To reduce overtreatment on the other hand, STM levels may show which patients treated with ICIs achieving a radiologically assessed partial response have approximately the same prognosis as patients with a complete remission. In these patients, treatment could potentially be discontinued earlier than per protocol [41].
Fig. 3
Flowcharts showing two potential scenarios for the treatment management of (A) stage III and (B) stage IV advanced NSCLC patients. Abbreviations: NSCLC, non-small cell lung cancer; STMs, serum tumor markers; OS, overall survival; SOC, standard of care.
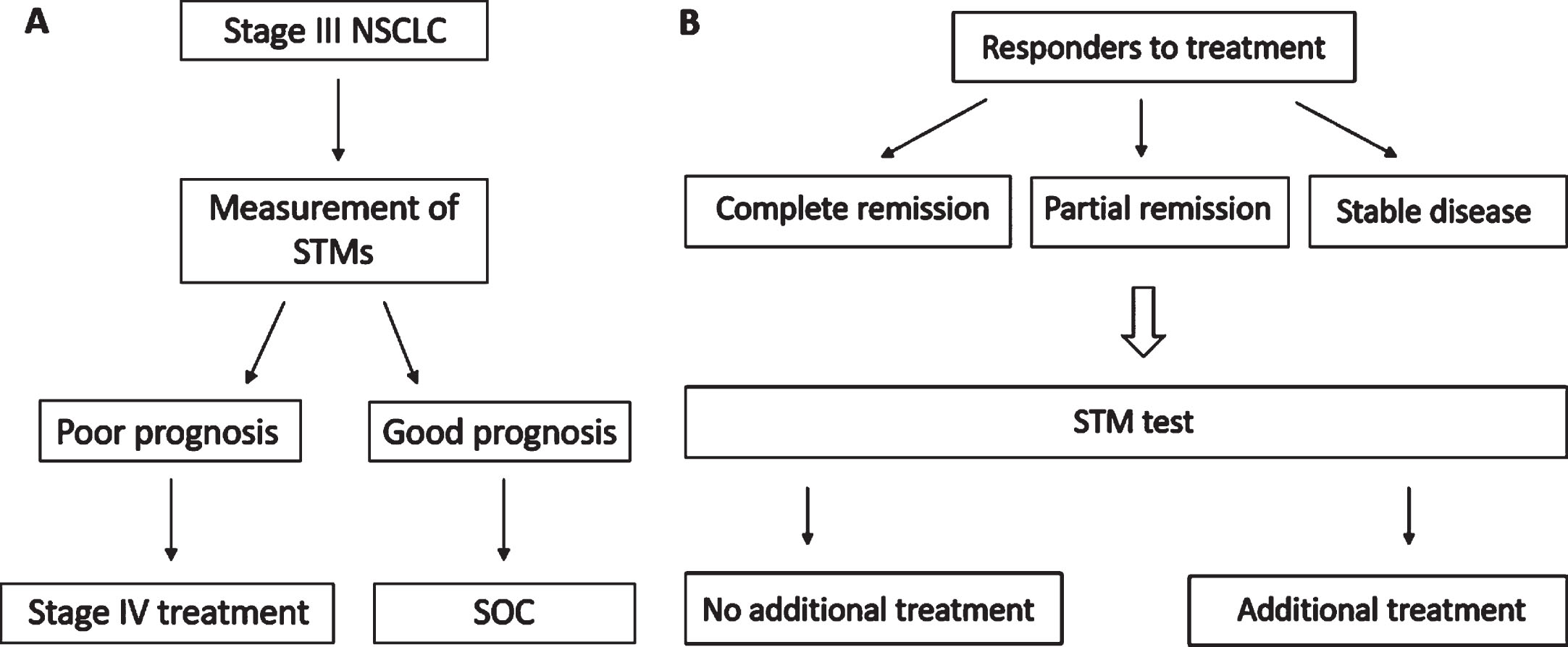
4.2Prediction of early treatment response
For the prediction of early treatment non-response, a high specificity is required to prevent inappropriate discontinuation of treatment in patients who potentially benefit from treatment [15]. Furthermore, the biomarker should be of added value next to currently used standard tests (e.g. radiological response and clinical assessment) [15]. Recently, Schuurbiers et al. showed that in advanced NSCLC patients treated with first-line ICIs, the CT-scan yields a specificity and sensitivity of approximately 96% and 33%, respectively, for prediction of non-response [42]. Previous results obtained by Muller et al. and Van Delft et al. showed that it is possible to obtain a high specificity with a developed STM test that predicts early non-response to ICI treatment [15, 43]. However, sensitivity rates per STM ranged between 5-32%. The combinatorial use of multiple STMs, yielded a sensitivity range between 30–38% [15]. This approach would therefore be preferred compared to the use of single STMs and could complement the radiological assessment. Indeed, STMs have shown to be able to distinguish radiological pseudoprogression from true progression in patients treated with ICIs, resulting in a high specificity of identifying non-responders [44–46]. Combining STM models with RECIST will be the next step towards improving early assessment of the disease status.
The prevalence of response directly influences the certainty with which a clinician can inform patients about their chance of responding to treatment. In subgroups with higher response rates (e.g. in patients treated with targeted therapy like ALK, ROS, EGFR, RET, NTRK, BRAF or MET), the PPV is relatively higher when compared to patient subgroups with lower response rates (e.g. in patients treated with ICIs) when applying a test with the same sensitivity and specificity (Example 1) [47, 48]. In these subgroups, applying a STM test allows the clinician to inform patients more accurately about their chance of response to treatment when compared to patient subgroups with lower response rates. On the other hand, the probability of identifying response in these patients based on radiological assessment is already relatively high. Applying a STM test as a companion biomarker next to radiological assessment in patient subgroups with low prevalence of response rates could therefore be of added value to increase the overall detection rate of response.
Example 1:
In case of ALK-positive advanced NSCLC patients, the response rate to first-line alectinib treatment is approximately 83% [47]. Applying a STM test with a sensitivity of 30% and specificity of 95%, would result in a PPV and NPV of 96% and 22%, respectively (Table 1).
Response to treatment | No response to treatment | Total number of patients | |
+ | 25 | 1 | 26 |
– | 58 | 16 | 74 |
Total number of patients | 83 | 17 |
In case of advanced NSCLC patients treated with second-line nivolumab, the response rate is approximately 19% [48]. Applying a STM test with a sensitivity of 30% and specificity of 95%, would result in a PPV and NPV of 60% and 86%, respectively (Table 2).
Response to treatment | No response to treatment | Total number of patients | |
+ | 6 | 4 | 10 |
– | 13 | 77 | 90 |
Total number of patients | 19 | 81 |
4.3Prediction of secondary treatment resistance
The same criteria for determining specificity and sensitivity minimum cut-off values as described for early treatment response can also be applied in the case of secondary treatment resistance prediction. In the case of secondary treatment resistance however, a lower sensitivity of the STM test could be accepted since repeated measurements will take place. Repeated measurements provide a predictive advantage over single observations as they capture changes in individual patients over time and are less sensitive to measurement errors, thereby increasing the sensitivity rate of a test [49]. Since STMs also reflect extra-thoracic disease activity, we expect that sensitivity of STMs will be higher when compared to CT-imaging during follow-up of treatment. Sensitivity can on the other hand be reduced in the prediction of secondary treatment resistance due to elevated STM levels in patients experiencing treatment-related adverse events or (benign) comorbidities (Fig. 4). A simultaneous increase in STM levels and decrease in total size of the determined target lesions should therefore be interpreted with caution, and always correlated to the clinical symptoms experienced by the patient.
Fig. 4
Serum tumor marker dynamics in immunotherapy-related pneumonitis.
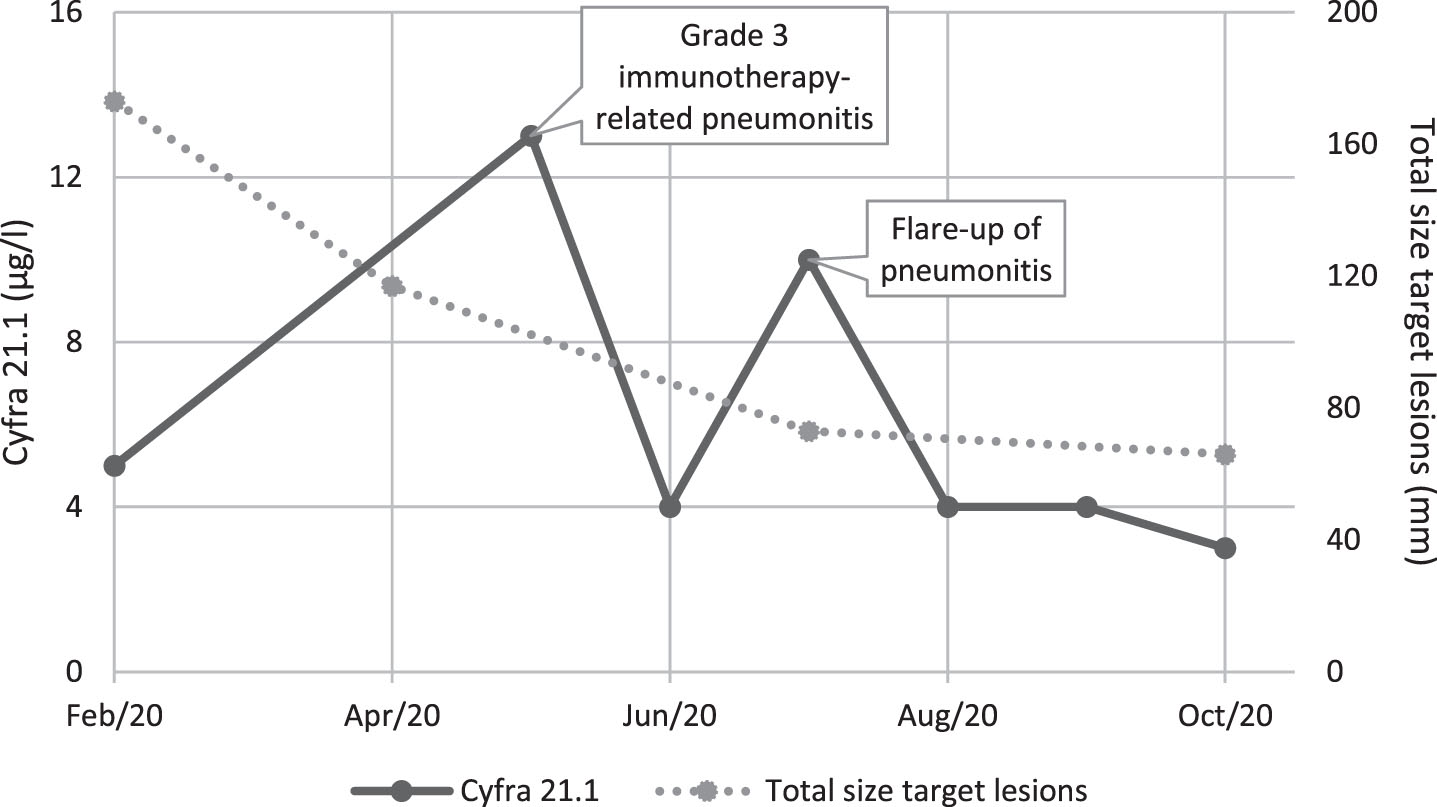
The applicability of STMs for multiple treatment modalities makes them suitable biomarkers to be continuously used for response monitoring during course of subsequent lines of treatment. This can provide both patient and doctor an overview of the disease control, independent of the treatment modality and prevent the necessity to apply a specific follow-up biomarker for each line of treatment (Fig. 5). However, depending on the context and the clinical question asked, different criteria should be applied when using the STM tests.
Fig. 5
Monitoring of multiple treatment modalities.
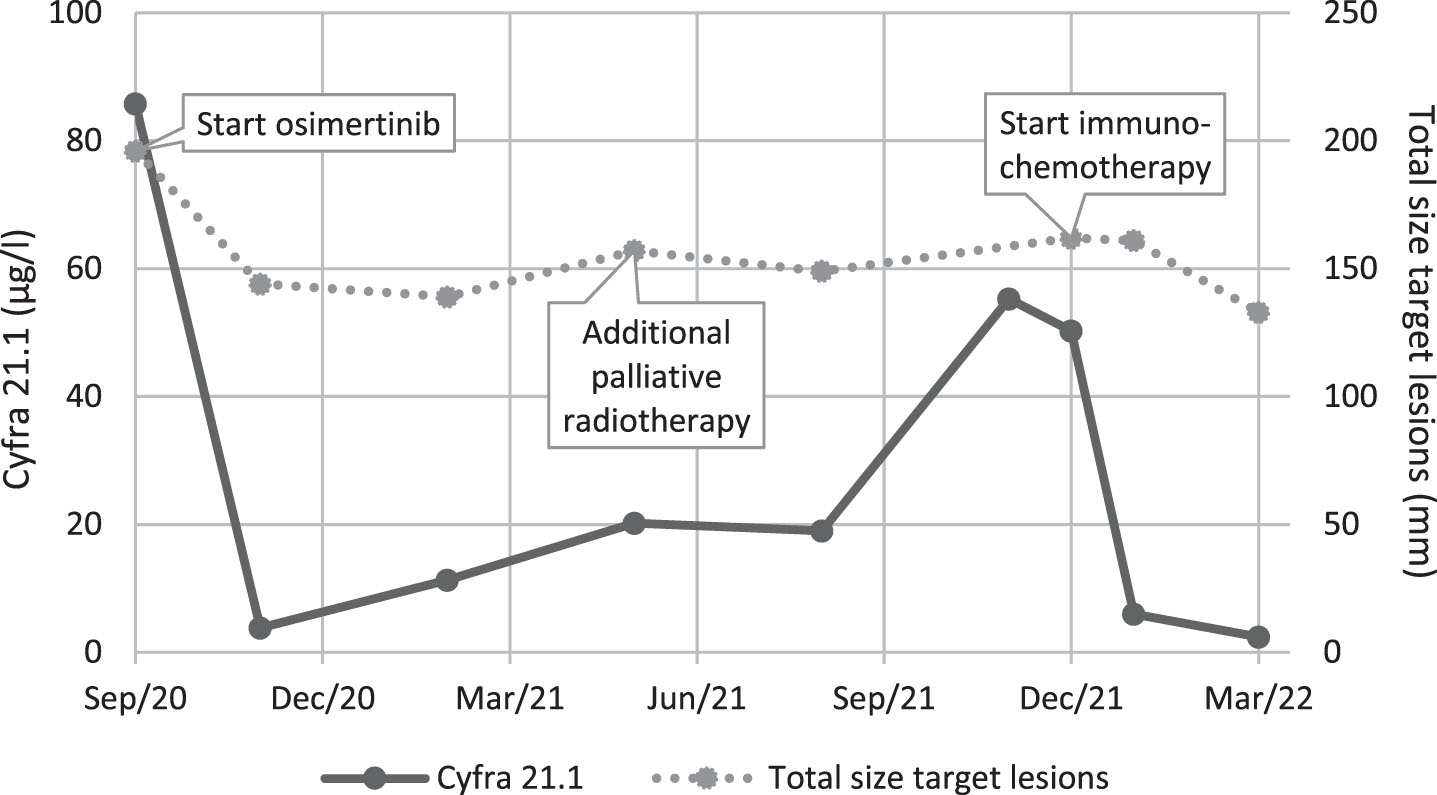
5Conclusion
There is no consensus in current clinical practice on the optimal positioning and usage of STMs. In this review, we attempted to provide an overview of the potential use and value of STMs in advanced NSCLC patients. From a clinician’s point of view, radiological imaging and clinical symptoms are often not sufficient to capture a patient’s disease status and therefore additional tools are necessary. STM measurements allow for a rapid, minimally invasive, and safe evaluation of the patient’s tumor status in real time. From a patient’s point of view, the use of STMs can help giving patients a more tangible way of having insight into their disease process next to radiological imaging. This could help them understand why (dis-)continuation and/or change of treatment is necessary from a doctor’s point of view, thereby facilitating shared decision-making, patient education and counselling. Based on the unmet needs in current practice in prediction of (a) prognosis, (b) early treatment response, and (c) secondary treatment resistance, considering the requirements and challenges to implement STMs in routine care and the value of STMs as decision-making support tools, we believe that the limitations identified during STM biomarker development can be overcome by applying different, specific test characteristics per clinical context. Also, the presented clinical applications can similarly be relevant when developing clinical applications for any other (circulating) biomarker (e.g. cell-free tumor DNA (ctDNA), circulating tumor cells (CTCs)). Future research should apply the approaches described in this review to achieve STM test implementation in advanced NSCLC care.
Acknowledgments
No acknowledgements.
Author contributions
CONCEPTION: Alessandra I.G. Buma, Michel M. van den Heuvel, Milou M.F. Schuurbiers and Huub H. van Rossum
PREPARATION OF THE MANUSCRIPT: Alessandra I.G. Buma
REVISION FOR IMPORTANT INTELLECTUAL CONTENT: Michel M. van den Heuvel, Milou M.F. Schuurbiers and Huub H. van Rossum
SUPERVISION: Michel M. van den Heuvel
Conflict of interest
Alessandra I.G. Buma has no conflict of interest to report.
Milou M.F. Schuurbiers has no conflict of interest to report.
Huub van Rossum is owner and director of Huvaros B.V. and holds stock in SelfSafeSure Blood Collections B.V. Huub van Rossum is a board member of Tumor Biology but had no participation in the peer review process of this paper.
Michel van den Heuvel has received research funding or honoraria from AbbVie, AstraZeneca, Bristol Myers Squibb, Eli Lilly, Janssen Pharmaceuticals, Merck & Co., Inc., Merck Sharp & Dohme, Novartis, PamGene, Pfizer, Roche, and Stichting Treatmeds. Michel van den Heuvel is a guest editor in the special issue “Lung Cancer in Tumor Markers” but had no participation in the peer review process of this paper.
References
[1] | Bassanelli M , Sioletic S , Martini M , Giacinti S , Viterbo A , Staddon A , et al. Heterogeneity of PD-L1 expression and relationship with biology of NSCLC. Anticancer Res (2018) ;38: (7):3789–9610. doi: 10.21873/anticanres.12662. |
[2] | Gridelli C , Ardizzoni A , Barberis M , Cappuzzo F , Casaluce F , Danesi R , et al. Predictive biomarkers of immunotherapy for non-small cell lung cancer: results from an experts panel meeting of the Italian Association of Thoracic Oncology. Transl Lung Cancer Res. (2017) ;6: (3):373–86. doi: 10.21037/tlcr.2017.05.09. |
[3] | Kort S , Tiggeloven MM , Brusse-Keizer M , Gerritsen JW , Schouwink JH , Citgez E , et al. Multi-centre prospective study on diagnosing subtypes of lung cancer by exhaled-breath analysis. Lung Cancer. (2018) ;125: :223–9. doi: 10.1016/j.lungcan.2018.09.022. |
[4] | Postmus PE , Kerr KM , Oudkerk M , Senan S , Waller DA , Vansteenkiste J , et al. Early and locally advanced non-small-cell lung cancer (NSCLC): ESMO clinical practice guidelines for diagnosis, treatment and follow-uup. Ann Oncol. (2017) ;28: (suppl_4);iv1–iv21. doi: 10.1093/annonc/mdx222. |
[5] | Duma N , Santana-Davila R , Molina JR , Non-small cell lung cancer: Epidemiology screening, diagnosis, and treatment. Mayo Clin Proc. (2019) ;94: (8):1623–40. doi: 10.1016/j.mayoc2019.01.013. |
[6] | American Cancer Society [homepage on the Internet]. Treatment choices for non-small cell lung cancer, by stage [updated 2022 March 14]. Available from: https://www.cancer.org/cancer/lung-cancer/treating-non-small-cell/by-stage.html. |
[7] | Chen R , Manochakian R , James L , Azzouqa A , Shi H , Zhang Y , et al. Emerging therapeutic agents for advanced non-small cell lung cancer. J Hematol Oncol. (2020) ;13: (1):58. doi: 10.1186/s13045-020-00881-7. |
[8] | Ballman KV . Biomarker: Predictive or Prognostic? J Clin Oncol. (2015) ;33: (33)3968–71. doi: 10.1200/JCO.2015.63.3651. |
[9] | George B , Chowdhury SM , Hart A , Sircar A , Singh SK , Nath UK , et al. Ibrutinib resistance mechanisms and treatment strategies for B-cell lymphomas. Cancers (Basel). (2020) ;12: (5):1328. doi: 10.3390/cancers12051328. |
[10] | Yaung SJ , Woestmann C , Ju C , Ma XM , Gattam S , Zhou Y , et al. Early assessment of chemotherapy response in advanced non-small cell lung cancer with circulating tumor DNA. Cancers (Basel). (2022) ;14: (10):2479. doi: 10.3390/cancers14102479. |
[11] | The Free Dictionary [homepage on the Internet]. Tumor Markers; (2010) . Available from: https://medical-dictionary.thefreedictionary.com/Tumor+Markers. |
[12] | Wahed A , Dasgupta A . Chapter 12 – Pitfalls in testing for common tumor markers. Accurate Results in the Clinical Laboratory (Second Edition). (2019) ;191–211. |
[13] | Vos D , Rao S , Pierce JD , Smith DA , Tirumani SH , Yoest JM , et al. The Past, Present, and Future (Liquid Biopsy) of Serum Tumor Markers in Lung Cancer: A Primer for the Radiologist. J Comput Assist Tomogr. (2021) ;45: :950–8. doi: 10.1097/RCT.0000000000001204. |
[14] | Wang L , Wang D , Zheng G , Yang Y , Du L , Dong Z , et al. Clinical evaluation and therapeutic monitoring value of serum tumor markers in lung cancer. Int J Biol Markers. (2016) ;31: (1)e80–7. doi: 10.5301/jbm.5000177. |
[15] | Muller M , Hoogendoorn R , Moritz RJG , van der Noort V , Lanfermeijer M , Korse CM , et al. Validation of a clinical blood-based decision aid to guide immunotherapy treatment in patients with non-small cell lung cancer. Tumour Biol. (2021) ;43: (1):115–27. doi: 10.3233/TUB-211504. |
[16] | Woodard GA , Jones KD , Jablons DM . Lung Cancer Staging and Prognosis. Cancer Treatment and Research book series. (2016) ;170: :47–75. doi: 10.1007/978-3-319-40389-2_3. |
[17] | Han K , Qian K , Zhao T , Liu XS , Zhang Y . Prediction of prognosis of patients with lung cancer in combination with the immune score. Biosci Rep. (2021) ;41: (5):BSR20203431. doi: 10.1042/BSR20203431. |
[18] | Pham J , Conron M , Wright G , Mitchell P , Ball D , Philip J , et al. Excess mortality and undertreatment in elderly lung cancer patients: treatment nihilism in the modern era? ERJ Open Res. (2021) ;7: (2):00393–2020. doi: 10.1183/23120541.00393-2020. |
[19] | ten Haaf K , van der Aalst CM , de Koning HJ . Clinically detected non-aggressive lung cancers: implications for overdiagnosis and overtreatment in lung cancer screening. Thorax. (2018) ;73: (5):407–8. doi: 10.1136/thoraxjnl-2017-211149. |
[20] | Nishino M . Tumor Response Assessment for Precision Cancer Therapy: Response Evaluation Criteria in Solid Tumors and Beyond. American Society of Clinical Oncology Educational Book. (2018) ;38: :1019–29. doi: 10.1200/EDBK_201441. |
[21] | Beer L , Hochmair M , Prosch H . Pitfalls in the radiological response assessment of immunotherapy. Memo. (2018) ;11: (2):138–43. doi: 10.1007/s12254-018-0389-x. |
[22] | Villaruz LC , Socinski MA . The Clinical Viewpoint: Definitions, Limitations of RECIST, Practical Considerations of Measurement. Clin Cancer Res. (2013) ;19: (10):2629–36. doi: 10.1158/1078-0432.CCR-12-2935. |
[23] | Nishino M , Hatabu H , Johnson BE , McLoud TC . State of the Art: Response Assessment in Lung Cancer in the Era of Genomic Medicine. Radiology. (2014) ;271: (1):6–27. doi: 10.1148/radiol.14122524. |
[24] | Hoang T , Schiller JH . The Benefits of Achieving Stable Disease in Advanced Lung Cancer. Oncology (Williston Park). (2003) ;17: (7):957–63. |
[25] | McCarrier KP , Atkinson TM , DeBusk KPA , Liepa AM , Scanlon M , Coons SJ , et al. Qualitative development and content validity of the non-small cell lung cancer symptom assessment questionnaire (NSCLC-SAQ), a patient-reported outcome instrument. Clin Ther. (2016) ;38: (4):794–810. doi: 10.1016/j.clinthera.2016.03.012. |
[26] | Bushnell DM , Atkinson TM , McCarrier KP , Liepa AM , DeBusk KP , Coons SJ , et al. Non-small cell lung cancer symptom assessment questionnaire: psychometric performance and regulatory qualification of a novel patient-reported symptom measure. Curr Ther Res Clin Exp. (2021) ;95: :100642. doi: 10.1016/j.curtheres.2021.100642. |
[27] | Dobbin KK , Cesano A , Alvarez J , Hawtin R , Janetzki S , Kirsch I , et al. Validation of biomarkers to predict response to immunotherapy in cancer: Volume II – clinical validation and regulatory considerations. J Immunother Cancer. (2016) ;4: :77. doi: 10.1186/s40425-016-0179-0. |
[28] | Parikh R , Mathai A , Parikh S , Sekhar GC , Thomas R . Understanding and using sensitivity, specificity and predictive values. Indian J Opthalmol. (2008) ;56: (1):45–50. doi: 10.4103/0301-4738.37595. |
[29] | Sharma S . Tumor markers in clinical practice: General principles and guidelines. Indian J Med Paediatr Oncol. (2009) ;30: (1):1–8. doi: 10.4103/0971-5851.56328. |
[30] | Duffy MJ . Role of tumor markers in patients with solid cancers: A critical review. Eur J Intern Med. (2007) ;18: (3):175–84. doi: 10.1016/j.ejim.2006.12.001. |
[31] | Yoshimasu T , Maebeya S , Suzuma T , Bessho T , Tanino H , Arimoto J , et al. Disappearance curves for tumor markers after resection of intrathoracic malignancies. Int J Biol Markers. (1999) ;14: (2):99–105. doi: 10.1177/172460089901400207. |
[32] | Cristofori R , Aimo G , Mengozzi G , Oliaro A , Revello F , Rapellino M . Tumor markers kinetic in malignant lung neoplasms. J Cardiovasc Surg (Torino). (1999) ;40: (2):299–305. |
[33] | Massacesi C , Rochhi MBL , Marcucci F , Pilone A , Galeazzi M , Bonsignori M . Serum tumor markers may precede instrumental response to chemotherapy in patients with metastatic cancer. Int J Biol Markers. (2003) ;18: (4):295–300. doi: 10.5301/jbm.2008.3624. |
[34] | Li X et al. Pulmonary sequestration associated with increased serum tumor markers and elevated standard uptake value level in PET/CT: A case report and literature review. Medicine (Baltimore). (2018) ;97: (31):e41171. doi: 10.1097/MD.0000000000011714. |
[35] | Yang Y , Xu M , Huang H , Jiang X , Gong K , Liu Y , et al. Serum carcinoembryonic antigen elevation in benign lung diseases. Sci Rep. (2021) ;11: (1):1904. doi: https://doi.org/10.1038/s41598-021-98513-8. |
[36] | Trapé J , Filella X , Alsina-Donadeu M , Juan-Pereira L , Bosch-Ferrer Á , Rigo-Bonnin R . Increased plasma concentrations of tumour markers in the absence of neoplasia. Clin Chem Lab Med. (2011) ;49: (10):1605–20. doi: 10.1515/CCLM.2011.694. |
[37] | Wen L , Wang S , Xu W , Xu X , Li M , Zhang Y , et al. Value of serum tumor markers for predicting EGFR mutations in non-small cell lung cancer patients. Ann Diagn Pathol. (2020) ;49: :151633. doi: 10.1016/j.anndiagpath.2020.151633. |
[38] | M.D./alert [homepage on the Internet]. New ESMO metastatic NSCLC clinical practice guidelines; 2019. Available from: https://www.mdalert.com/ms/calung/article/new-esmo-nsclc-clinical-practice-guidelines. |
[39] | Simon R . Clinical trial designs for evaluating the medical utility of prognostic and predictive biomarkers in oncology. Per Med. (2010) ;7: (1):33–47. doi: 10.2217/pme.09.49. |
[40] | Evison M ., AstraZeneca UK Limited. The current treatment landscape in the UK for stage III NSCLC. Br J Cancer. (2020) ;123: :3–9. doi: 10.1038/s41416-020-01069-z. |
[41] | Bilger G , Girard N , Doubre H , Levra MG , Giroux-Leprieur E , Giraud F , et al. Discontinuation of immune checkpoint inhibitor (ICI) above 18 months of treatment in real-life patients with advanced non-small cell lung cancer (NSCLC): INTEPI, a multicentric retrospective study. Cancer Immunol Immunother. (2022) ;71: (7):19–31. 10.1007/s00262-021-03114-z. |
[42] | Schuurbiers MMF , van Delft FA , Koffijberg H , IJzerman MJ , van Rossum HH , van den Heuvel MM . Validated blood-based model combined with RECIST predicts no durable benefit to immunotherapy in metastasized non-small cell lung cancer. Available from: https://oncologypro.esmo.org/meeting-resources/esmo-congress. |
[43] | van Delft FA , Schuurbiers M , Muller M , Burgers SA , van Rossum HH , IJzerman MJ , et al. Modeling strategies to analyse longitudinal biomarker data: An illustration on predicting immunotherapy non-response in non-small cell lung cancer. Heliyon. (2022) ;8: (10):e10932. doi: 10.1016/j.heliyon.2022.e10932. |
[44] | Dong Q , Sun X , Zhou Y , Diao Y , Ran J , Zhang J . Dynamic monitoring of serum specific tumor markers predicts the response to PD-L1 blockade and prognosis of patients with malignant tumors. Transl Cancer Res. (2021) ;10: (2):779–89. 10.21037/tcr-20-2560. |
[45] | Yoshimura A , Takumi C , Tsuji T , Hamashima R , Shiotsu S , Yuba T , et al. Pulmonary pleomorphic carcinoma with pseudoprogression during nivolumab therapy and the usefulness of tumor markers: A case report. Clin Case Rep. (2018) ;6: (7):1338–41. doi: 10.1002/ccr3.1627. |
[46] | Tanizaki J , Hayashi H , Kimura M , Tanaka K , Takeda M , Shimizu S , et al. Report of two cases of pseudoprogression in patients with non-small cell lung cancer treated with nivolumab-including histological analysis of one case after tumor regression. Lung Cancer. (2016) ;102: :44–8. doi: 10.1016/j.lungcan.2016.10.014. |
[47] | Peters S , Camidge R , Shaw AT , Gadgeel S , Ahn JS , Kim D , et al. Alectinib versus crizotinib in untreated ALK-positive non-small cell lung cancer. N Engl J Med. (2017) ;377: :829–38. doi: 10.1056/NEJMoa1704795. |
[48] | Borghaei H , Paz-Ares L , Horn L , Spigel DR , Steins M , Ready NE , et al. Nivolumab versus docetaxel in advanced nonsquamous non-small-cell lung cancer. N Engl J Med. (2015) ;373: :1627–39. doi: 10.1056/NEJMoa1507643. |
[49] | Bull LM , Lunt M , Martin GP , Hyrich K , Sergeant JC . Harnessing repeated measurements of predictor variables for clinical risk prediction: a review of existing methods. Diagn Progn Res. (2020) ;4: :9. doi: 10.1186/s41512-020-00078-z. |