The inhibition mechanism of epilepsy disease in a computational model
Abstract
BACKGROUND:
The mechanism of prevention and treatment of epilepsy is a hot issue in theoretical research.
OBJECTIVE:
In this paper, we studied the control mechanism of the generalized spike-and-wave discharges (GSWD) by different types of external electrical stimulation acting on the subthalamic nucleus (STN) in a computational model.
METHODS:
Firstly, we analyzed the pathological mechanism of seizures, which were induced by different parameters in the thalamocortical (TC) circuit. Then, a voltage V was exerted in the STN. At last, we used the sine wave and square wave current stimulation in the STN.
RESULTS:
We found that seizures can be inhibited by tuning stimulus intensity into suitable range, and the direction of adjustment depended on the size of the parameter. We observed that the seizure can also be inhibited by tuning different parameters in current.
CONCLUSIONS:
Different inhibition mechanisms can be explained in this model, which may provide theoretical evidences for selecting the optimal treatment scheme in the clinical.
1.Introduction
Absence epilepsy (AE) is the most common type of primary generalized epilepsy in children [1]. The clinical features of AE are convulsive unconsciousness attacks, and the generalized spike-and-wave discharges (GSWD) (2–4 Hz) appear in the background of normal electroencephalogram (EEG) [2]. Recently, many studies based on the functional MRI localization (fMRI) of EEG synchronous have found that the thalamus structure and cerebral cortex closely relate to the onset of the GSWD [3, 4], and the theoretical mechanism of which has been explored in many dynamic models [5, 6]. Amiri et al. found that disruption of the signaling function of astrocytes may induce seizure activities in a modified TC model [5]. Haghighi et al. studied the transition mechanism between ictal and interictal states based on dynamic analysis in a TC network [6]. Shojaee et al. observed that the change of coupling strength in TC network can be taken as detector to predict epilepsy seizures [7]. These results may provide reference for the prevention of seizures.
Antiepileptic drug therapy is a common method to relieve seizures [8], drugs control the regulation mechanism inside the body. However, the treatment and mitigation of many intractable seizures must resort to surgical brain stimulation technique, such as the deep brain stimulation (DBS). The DBS has been proved to be very effective in relieving epilepsy symptoms and the subthalamic nucleus (STN) is a common stimulus area [9, 10, 11]. Handforth et al. found that subthalamic DBS as an adjunct treatment for refractory epilepsy can partly control seizures [11]. Usui et al. shown that unilateral STN DBS has significant effect in inhibiting limbic seizures [12]. Ke et al. pointed out that electrical stimulation on dorsal STN can control melanocortinergic signaling in astrocytes [13]. Kase et al. indicated that the STN is involved in the rhythm maintenance and STN DBS can inhibit the GSWD in AE by tuning frequency [14]. Wille et al. shown that the STN may be an effective stimulus target for progressive myoclonic epilepsy, especially for less impaired patients [15]. Prabhu et al. pointed out that STN DBS could be used as an experimental therapy for motor epilepsy [16]. Recently, Wang et al. reported a favorable outcome of STN DBS for focal myoclonic seizures [17]. And, the STN DBS has been proved to be successful in treating many other nervous system diseases, such as Parkinson’s disease [18, 19] and dystonia [20]. The effect of the DBS for controlling drug-resistant seizures in childhood can refer to a systematic review in the reference [21]. However, the mechanism of the DBS is still unclear and theoretical research evidences for epilepsy are rare now.
In this paper, we used a computational model [22, 23] to explore the inhibition mechanism of the GSWD by different electrical stimulation acting in the STN. The results may provide theoretical evidences for experimental studies in inhibiting of AE, and can inspire control mechanism research in other types of epilepsy.
2.Network model and computing method
2.1Mathematical mean-field model
The network connection structure is shown in Fig. 1 [22, 23]. Different nerve nuclei are abbreviated as follows:
Figure 1.
![The connection structure diagram of the basal ganglia-thalamocortical model (BGTC) [22, 23].](https://ip.ios.semcs.net:443/media/thc/2022/30-S1/thc-30-S1-thc228015/thc-30-thc228015-g001.jpg)
The coupling relation of the model is described by the following first-order mean-field differential equations, the specific biological significance of which has been discussed in previous research [22, 23, 24, 25, 26]:
2.2Numerical simulation technology and data
All simulations were obtained by the Runge-Kutta algorithm and bifurcation analysis [22, 26]. The default data were derived from previous studies [22, 23, 24, 25, 26, 27, 28, 29], which were listed as follows: The unit in mV s:
Figure 2.
(a): A state bifurcation process induced by
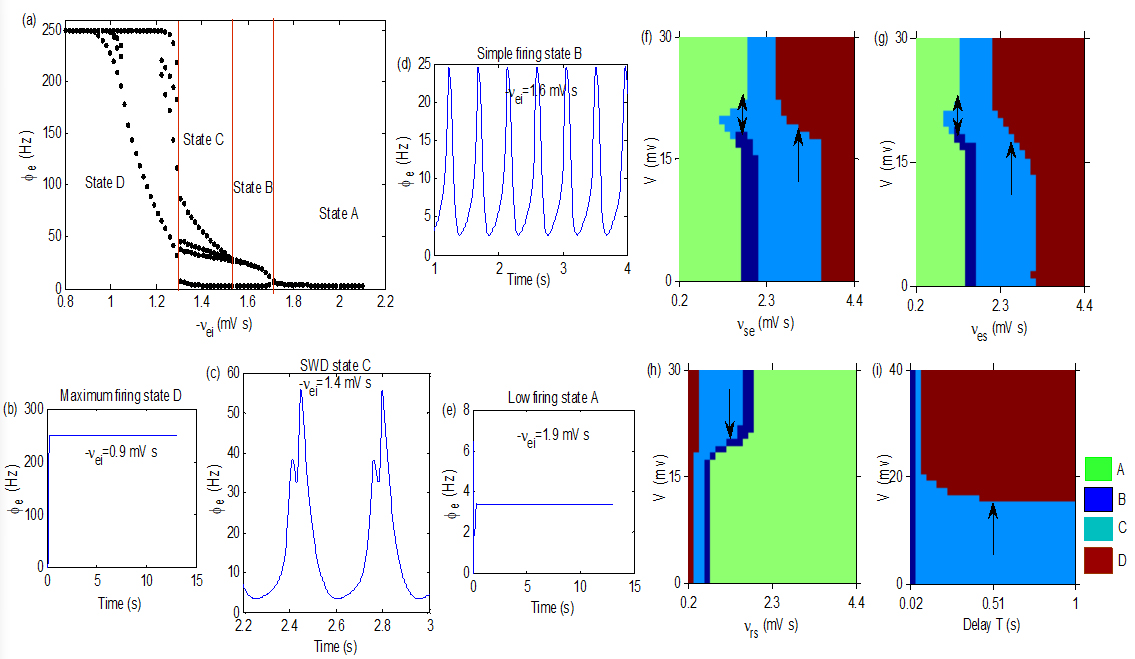
3.Main results
Coupling weights are main parameters in the model, which represent connection strengths between different populations and the change of them is easy to induce state transition in the TC network. Figure 2a is a typical state bifurcation process, which was induced by
Figure 2f–i show four control analysis diagrams in (
Figure 3.
(a): The sine wave current. Here, we set the parameter P in (1) to 30 s. (b): The control effect when the current Isin acts on STN, with P
![(a): The sine wave current. Here, we set the parameter P in (1) to 30 s. (b): The control effect when the current Isin acts on STN, with P = 30. (c): The square wave current. Here, we set the parameter P in (2) to be 3 s and D = 2 s. (d): The control effect diagram obtained by adjusting the D. Here, we set A = 50, T = 40. (e): The state transition diagram obtained by tuning the T. Here, we set simulation calculation points in the interval [0.0375, 3] for T is 80, A = 40, D = 0.0006 and -νsr= 1.5. We take the resistance R=1Ω.](https://ip.ios.semcs.net:443/media/thc/2022/30-S1/thc-30-S1-thc228015/thc-30-thc228015-g003.jpg)
In addition to uniform voltage stimulation, other types of variable – frequency currents, such as sine wave (1) and square wave (2) [31, 32] stimulation, are often used in clinical trials. Where A represents the amplitude, P is a parameter associated with the period of the current, and D is the effective duration of current in a period. Figure 3a describes the sine wave current. Figure 3b is the inhibition effect of the seizures by employing the sine wave current on the STN. Indicated by arrows, state C may be relieved via tuning the current amplitude A into suitable values. However, the control effect depends on
(1)
(2)
4.Conclusion
In this paper, we used a basal ganglia – thalamocortical computational model to study the control mechanism of absence epileptiform activities by different electrical stimulation acting in the STN. Firstly, we analyzed the pathological mechanism of seizures, which were caused by the coupling weight
Acknowledgments
This research was supported by the National Science Foundation of China (No. 11602092); the Natural Science Foundation of Hubei Province (No. 2018CFB628); and the China Postdoctoral Science Foundation (No. 2018M632184).
Conflict of interest
None to report.
References
[1] | Pavone P, Bianchini R, Trifiletti RR, et al. Neuropsychological assessment in children with absence epilepsy. Neurology. (2001) ; 56: (8): 1047-1051. |
[2] | Hedström A, Olsson I. Epidemiology of absence epilepsy: EEG findings and their predictive value. Pediatr. Neurol. (1991) ; 7: (2): 100-104. |
[3] | Kim JB, Suh S, Seo WK, et al. Altered thalamocortical functional connectivity in idiopathic generalized epilepsy. Epilepsia. (2014) ; 55: (4): 592-600. |
[4] | Jiang S, Luo C, Gong J, et al. Aberrant thalamocortical connectivity in juvenile myoclonic epilepsy. Int.J. Neural.Syst. (2018) ; 28: (01): 1750034. |
[5] | Amiri M, Bahrami F, Janahmadi M. Modified thalamocortical model: A step towards more understanding of the functional contribution of astrocytes to epilepsy. J.Comput. Neurosci. (2012) ; 33: (2): 285-299. |
[6] | Haghighi HS, Markazi AHD. A new description of epileptic seizures based on dynamic analysis of a thalamocortical model. Sci. Rep. (2017) ; 7: (1): 1-10. |
[7] | Shojaee M, Soltanpour MR. Effects of coupling parameter in thalamocortical model on seizure prediction for temporal lobe epilepsy. J. Clin.Eng. (2018) ; 43: (2): 83-91. |
[8] | Glauser TA, Holland K, O’Brien VP, et al. Pharmacogenetics of antiepileptic drug efficacy in childhood absence epilepsy. Ann. Neurol. (2017) ; 81: (3): 444-453. |
[9] | Charbades S, Kahane P, Minotti L, et al. Deep brain stimulation in epilepsy with particular reference to the subthalamic nucleus. Epileptic Disord. (2002) ; 4: : S83-S93. |
[10] | Lee KJ, Jang KS, Shon YM. Chronic deep brain stimulation of subthalamic and anterior thalamic nuclei for controlling refractory partial epilepsy//Advances in functional and reparative neurosurgery. Springer, Vienna, (2006) ; 87-91. |
[11] | Handforth A, DeSalles AAF, Krahl SE. Deep brain stimulation of the subthalamic nucleus as adjunct treatment for refractory epilepsy. Epilepsia. (2006) ; 47: (7): 1239-1241. |
[12] | Usui N, Maesawa S, Kajita Y, et al. Suppression of secondary generalization of limbic seizures by stimulation of subthalamic nucleus in rats. J. Neurosurg. (2005) ; 102: (6): 1122-1129. |
[13] | Ke B, Liu TT, Liu C, et al. Dorsal subthalamic nucleus electrical stimulation for drug/treatment-refractory epilepsy may modulate melanocortinergic signaling in astrocytes. Epilepsy Behav. (2014) ; 36: : 6-8. |
[14] | Kase D, Inoue T, Imoto K. Roles of the subthalamic nucleus and subthalamic HCN channels in absence seizures. J. Neurophysiol. (2012) ; 107: (1): 393-406. |
[15] | Wille C, Steinhoff BJ, Altenmüller DM, et al. Chronic high-frequency deep-brain stimulation in progressive myoclonic epilepsy in adulthood – Report of five cases. Epilepsia. (2011) ; 52: (3): 489-496. |
[16] | Prabhu S, Chabardès S, Sherdil A, et al. Effect of subthalamic nucleus stimulation on penicillin induced focal motor seizures in primate. Brain Stimul. (2015) ; 8: (2): 177-184. |
[17] | Wang X, Du J, Wang D, et al. Long-term outcome of unilateral deep brain stimulation of the subthalamic nucleus for a patient with drug-resistant focal myoclonic seizure. Ann. Transl. Med. (2020) ; 8: (1): 18. |
[18] | Campos ACP, Kikuchi DS, Paschoa AFN, et al. Unraveling the Role of Astrocytes in Subthalamic Nucleus Deep Brain Stimulation in a Parkinson’s Disease Rat Model. Cell. Mol. Neurobiol. (2020) ; 40939-954. |
[19] | Gorecka-Mazur A, Furgala A, Krygowska-Wajs A, et al. Activities of daily living and their relationship to health-related quality of life in patients with parkinson disease after subthalamic nucleus deep brain stimulation. World Neurosurg. (2019) ; 125: : e552-e562. |
[20] | Tsuboi T, Wong JK, Almeida L, et al. A pooled meta-analysis of GPi and STN deep brain stimulation outcomes for cervical dystonia. J. Neurol. (2020) ; 2671278: -1290. |
[21] | Yan H, Toyota E, Anderson M, et al. A systematic review of deep brain stimulation for the treatment of drug-resistant epilepsy in childhood. J.Neurosurg-Pediatr. (2018) ; 23: (3): 274-284. |
[22] | Chen MM, Guo DQ, Wang TB, et al. Bidirectional control of absence seizures by the basal ganglia: A computational evidence. PLoS Comput. Biol. (2014) ; 10: (3): e1003495. |
[23] | Hu B, Guo D, Wang Q. Control of absence seizures induced by the pathways connected to SRN in corticothalamic system. Cogn Neurodynamics. (2015) ; 9: (3): 279-289. |
[24] | van Albada SJ, Robinson PA. Mean-field modeling of the basal ganglia thalamocortical system. I Firing rates in healthy and parkinsonian states. J. Theor. Biol. (2009) ; 257: (4): 642-663. |
[25] | van Albada SJ, Gray RT, Drysdale PM, et al. Mean-field modeling of the basal ganglia-thalamocortical system. II: Dynamics of parkinsonian oscillations. J. Theor. Biol. (2009) ; 257: (4): 664-688. |
[26] | Chen M, Guo D, Li M, et al. Critical roles of the direct GABAergic pallido-cortical pathway in controlling absence seizures. PLoS Comput. Biol. (2015) ; 11: (10): e1004539. |
[27] | Roberts JA, Robinson PA. Modeling absence seizure dynamics: Implications for basic mechanisms and measurement of thalamocortical and corticothalamic latencies. J. Theor. Biol. (2008) ; 253: (1): 189-201. |
[28] | Hu B, Wang D, Shi Q. The effect of external voltage stimulation on absence seizures. Technol Health Care. (2020) ; 28: : 245-251. |
[29] | Roberts JA, Robinson PA. Modeling absence seizure dynamics: Implications for basic mechanisms and measurement of thalamocortical and corticothalamic latencies. J. Theor. Biol. (2008) ; 253: (1): 189-201. |
[30] | Marten F, Rodrigues S, Benjamin O, et al. Onset of polyspike complexes in a mean-field model of human electroencephalography and its application to absence epilepsy. Philos. Trans. R. Soc., A. (2009) ; 367: (1891): 1145-1161. |
[31] | Hu B, Chen S, Chi H, et al. Controlling absence seizures by tuning activation level of the thalamus and striatum. Chaos, Solitons Fractals, (2017) ; 95: : 65-76. |
[32] | Rubin JE, Terman D. High frequency stimulation of the subthalamic nucleus eliminates pathological thalamic rhythmicity in a computational model. J.Comput. Neurosci. (2004) ; 16: (3): 211-235. |