Risk assessment of type 2 diabetes in northern China based on the logistic regression model
Abstract
BACKGROUND:
Type 2 diabetes mellitus (T2DM) is a complex disease with high incidence and serious harm associated with polygenic determination. This study aimed to develop a predictive model so as to assess the risk of T2DM and apply it to health care and disease prevention in northern China.
OBJECTIVE:
Based on genotyping results, a risk warning model for type 2 diabetes was established.
METHODS:
Blood samples of 1042 patients with T2DM in northern China were collected. Multiplex polymerase chain reaction and high-throughput sequencing (NGS) techniques were used to design the amplification-based targeted sequencing panel to sequence the 21 T2DM susceptibility genes.
RESULT:
The related key gene KQT-like subfamily member 1 played an important role in the T2DM risk model, and single-nucleotide polymorphism rs2237892 was highly significant, with a
CONCLUSIONS:
Susceptibility genes in different populations were examined, and a model was developed to assess the risk-based genetic analysis. The performance of the model reached 92.8%.
1.Introduction
The prevalence rate of diabetes in China has increased rapidly, and the increase in medical expenses has brought a heavy burden to families and society in the last two decades. At present, the prevalence rate of diabetes in China is 9.1%. China has more than 100 million patients and 400 million potential patients [1]. Hence, diabetes has become a prominent public health and social problem. Type 2 diabetes mellitus (T2DM) occurs mainly in adults and is the main type of diabetes, accounting for more than 95% of the total patients with diabetes. Recent genome-wide association studies (GWAS) and improved single-nucleotide polymorphism (SNP) analyses have revealed hundreds of common genetic mutations closely related to T2DM. However, the relationship between gene polymorphism and the occurrence of T2DM, as well as its underlying mechanism, is still unclear [2, 3, 4, 5]. Consequently, the etiology and pathogenesis of T2DM have not been fully elucidated. Further, differences exist in the genetic background, living environment, and behavioral patterns. The T2DM susceptibility gene mapping of different regions and nationalities is different. Also, no accurate detection and evaluation model is available to screen populations at high risk of diabetes. Therefore, the occurrence of T2DM and its correlation with the SNP of the T2DM susceptibility gene have become a hot spot in recent years [6, 7, 8].
In 2007, a study entitled “risk assessment method of diabetes in adults in China” published in the Chinese Journal of Health Management proposed the first DM risk assessment model in China [9]. The model was not based on population data, but was a composite model estimated by literature and expert experience. However, it was an effective method in the absence of prospective cohort studies in China. Unfortunately, the model had no data validation results. In 2009, Chien [10] established a prediction model for DM in the Taiwan community population. This model was the first individual risk score model of DM in a Chinese population based on Framingham cardiovascular prediction model. The cohort data of people aged more than 35 years were tracked for 10 years using the Cox proportional hazards model. The risk assessment score system was established using the Framingham risk score equation published by Sullivan [11] in 2004. The indicators included age, fasting blood glucose level, BMI, TG, i-idl-c, and blood leukocyte count. After the establishment of the model, the statistical methods of net reclamation improvement and integrated discrimination improvement were used for training. The AUC reached 0.702, which was better than that of the classic models such as San Antonio, Framingham, PROCAM, and Cambridge. To a large extent, the model covered the risk assessment of DM in China. However, the application of the model was limited due to the close relationship between leukocyte count and infection.
This study was performed to develop a predictive model so as to assess the risk of T2DM and apply it to health care and disease prevention in northern China.
2.Materials and method
2.1Screening of susceptibility genes
Data resources from the following databases were used: OMIM online [12], GWAS Catalog [13], GeneCards [14], and HGMD [15]. More than 350 susceptibility genes related to T2DM were statistically analyzed using the aforementioned databases. Also, the distribution of different populations was different. The susceptibility genes reported in European populations were TCF7L2, CDKAL1, SLC30A8, FTO, CDKN2B, and so forth, while the susceptibility gene reported in East Asian populations was KQT-like subfamily member 1 (KCNQ1) [16, 17, 18, 19, 20, 21, 22, 23]. The frequency of the report is shown in Fig. 1. Furthermore, 21 of the 16 susceptibility genes were selected in combination with the reported frequency of the susceptibility genes or loci on the basis of the analysis of population differences, IPA gene, and mind pathway, as shown in Table 1.
Table 1
Screening susceptibility genes and locus as well as the function of genes
Gene name | Mutation locus | Gene function |
---|---|---|
KCNQ1 | rs2237892 rs2237895 rs2237897 | Potassium voltage-gated channel, KQT-like subfamily member 1 (KCNQ1), is an |
C2CD4A | rs7172432 | C2 calcium-dependent domain containing 4A (C2CD4A/C2CD4B) is a protein dependent on C2 structural domain and contains calcium ion. Research group of Grarup found that the gene of C2CD4A/C2CD4B causes diabetes by impairing the function of islet |
TCF7L2 | rsl2243326 rs7903146 | The TCF7L2 gene is located in the long arm 25 region of human chromosome 10; it has 2159 base pairs and contains 17 exons. The expression of this gene is related to the secretory function, proliferation, and apoptosis of islet |
SLC30A8 | rs13266634 | The rs13266634 locus of the SLC30A8 gene is associated with an increase in waist circumference, the insulin sensitivity of the population carrying the allele is reduced, and the insulin secretion stimulated by intravenous glucose tolerance is reduced in this population. |
CDKAL1 | rs712523 | The cyclin-dependent kinase 5 regulatory subunit-associated protein 1-like l (CDKAL1) gene is one of the most important genes for type 2 diabetes (T2DM). The CDKAL1 gene is located at 6p22.3 and is 37 kb in length; it encodes a protein containing 579 amino acid residues. |
CDKN2A/ 2B | rs10811661 | The cyclin-dependent kinase inhibitor (CDKN2A/2B) gene can inhibit a key regulator of pancreatic 13 cell replication CDK4, which causes islet hypoplasia and diabetes. |
KCNJ11 | rs5219G/A | Potassium inwardly-rectifying channel, subfamily J, member ll (KCNJ11) is a Kir6.2 protein, which is a K |
PPAR | rsl801282 | It is involved in the regulation of adipocyte differentiation. It is the target of thiazolidinedione hypoglycemic drugs. The PPAR |
IGF2BP2 | rs4402960 | The insulin-like growth factor 2 mRNA-binding protein 2 (IGF2BP2) is an analog of IGF2BP1 that binds to the 5’-untranslated region of insulin-like growth factor 2 (IGF-II) mRNA. It regulates translational H of IGF-II and is involved in pancreas development, growth, and insulin secretion. The SNP rs4402960 with the strongest correlation is located in the 50-kb region of intron 2, correlating with insulin sensitivity and blood glucose levels. |
FTO | rs8050136 and rs9939609 | The fat mass and obesity associated (FTO) is a gene expressed in the hypothalamus to regulate appetite. The effect of FTO on the association of T2DM is through the action of certain components that affect BMI or metabolic syndrome. Both rs8050136 and rs9939609 are located in the first intron of the FTO gene, which is associated with BMI, obesity, and metabolic syndrome components in the general population. rs9930506 is significantly associated with BMI, hip circumference, and body weight. G mutant homozygotes have 1.3 unit BMI more than that of the wild-type ones. |
.JAZFl | rs864745 | Juxtaposed with another zinc finger gene 1 (JAZFl) belonging to the nuclear receptor subfamily 2, group C, member 2 is an orphan nuclear receptor for three C2-H2-like zinc finger proteins. Mice lacking the NR2C2 gene showed growth retardation, low IGF-I levels, and perinatal and postnatal hypoglycemia. Rs864745 is located in the first intron of JAZFI, and its allelic mutation is significantly associated with T2DM. |
Table 1, continued | ||
---|---|---|
Gene name | Mutation locus | Gene function |
GRK5 | rs10886471 | Protein-coupled receptor kinase 5 (GRK5) rs10886471 belongs to the family of protein kinases and plays a key role in the phosphorylation of various GPCR and non-GPCR matrices, such as the glucagon receptor, the Hsp70-interacting protein, and the nuclear factor kB1/p105, which regulate blood sugar levels. |
RASGRP1 | rs7403531 | This site is located in the second intron of the RASGRP1 gene. The RASGRP1 gene is highly expressed in lymphocytes. The loss of function of this gene in beta-cells leads to islet inflammation and impairs the function of beta cells. These factors are associated with T2DM. |
HHEX | rs1111875 | Hematopoietic stem cell expression homeobox gene (HHEX) rs1111875G/A polymorphism is associated with GDM; G allele may be its risk allele, and HHEX gene may be one of the GDM (gestational diabetes) susceptibility genes in the region. |
PTPRD | rs17584499 | The PTPRD gene is widely expressed in muscle and pancreatic tissues and is highly expressed in the brain. Mice lacking the PTPRD gene also have memory and cognitive disorders. PTPRD belongs to the protein tyrosine phosphorylase subfamily receptor type IIA (R2A), and R2A consists of LAR, PTPRS, and PTPRD. |
Figure 1.
Reported frequency of susceptibility genes among Asians (left) and Europeans (right).
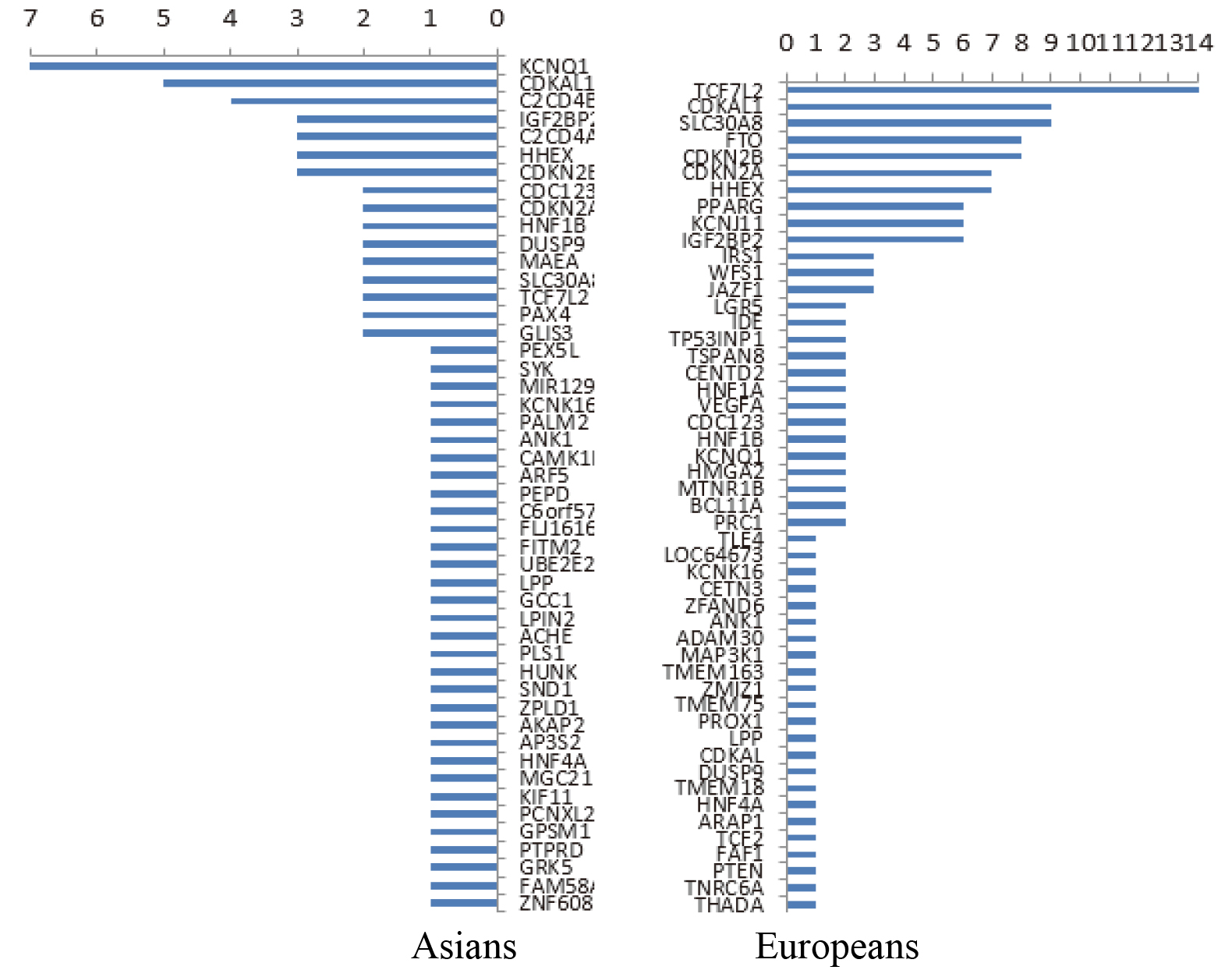
2.2Sample collection
In 2014 and 2015, 1042 peripheral blood DNA samples of patients diagnosed with T2DM in Hebei Yiling Hospital were selected, which met the 1999 World Health Organization diagnostic criteria for diabetes. This study was approved by the ethics committee of each hospital, and all participants signed informed consent. Each individual sample should be no less than 5 mL to meet the requirements of subsequent DNA extraction and gene mutation identification. Vacuum containers with EDTA anticoagulant were collected. The samples are stored at
3.Results and analysis
3.1Statistical analysis of sequencing results
High-quality sequencing data were obtained through second-generation sequencing. The software analyzed the mutation frequency of 21 SNP sites in the samples of 1042 patients, as shown in Table 2, in accordance to a previous study [24]. A comparison of the genotypes of the SNP sites showed that the distribution frequency of the genotypes was as follows: PPARG rs1801282, IGF2BP2 rs4402960, HHEX rs1111875, HNF1 rs4430796, and WFS1 rs10010131 with no significant difference (T2D
Table 2
Statistics of mutation frequency of 21 SNP loci
CHR | SNP | A2 | A1 case freq | A1 control freq | OR | L95 | U95 | |
---|---|---|---|---|---|---|---|---|
3 | rs1801282 | C | 0.2932 | 0.03223 | 6.05E-64 | 12.46 | 8.696 | 17.84 |
3 | rs4402960 | G | 0.4488 | 0.2529 | 4.23E-26 | 2.405 | 2.039 | 2.836 |
6 | rs1800629 | G | 0.05455 | 0.05762 | 0.7255 | 0.9438 | 0.6832 | 1.304 |
7 | rs1800795 | G | 0.102 | 0.003906 | 5.67E-24 | 28.96 | 10.74 | 78.1 |
7 | rs864745 | T | 0.473 | 0.2314 | 1.68E-38 | 2.98 | 2.518 | 3.527 |
8 | rs13266634 | C | 0.4862 | 0.458 | 0.1378 | 1.12 | 0.9643 | 1.301 |
9 | rs17584499 | C | 0.4948 | 0.1445 | 5.22E-80 | 5.797 | 4.775 | 7.038 |
9 | rs10811661 | T | 0.4995 | 0.4365 | 0.00093 | 1.288 | 1.109 | 1.497 |
10 | rs1111875 | T | 0.4763 | 0.2871 | 6.69E-24 | 2.258 | 1.924 | 2.65 |
10 | rs7903146 | C | 0.02277 | 0.02246 | 0.9565 | 1.014 | 0.6134 | 1.677 |
10 | rs12243326 | T | 0.08776 | 0.01367 | 1.54E-15 | 6.94 | 4.01 | 12.01 |
10 | rs10886471 | C | 0.305 | 0.2275 | 5.84E-06 | 1.49 | 1.253 | 1.771 |
11 | rs2237892 | C | 0.2751 | 0.3516 | 1.20E-05 | 0.7001 | 0.5966 | 0.8216 |
11 | rs2237895 | A | 0.4307 | 0.333 | 1.61E-07 | 1.516 | 1.297 | 1.771 |
11 | rs2237897 | C | 0.2372 | 0.3496 | 3.65E-11 | 0.5785 | 0.4915 | 0.6809 |
11 | rs5219 | C | 0.2898 | 0.3389 | 0.005225 | 0.7963 | 0.6786 | 0.9345 |
15 | rs7403531 | C | 0.4972 | 0.3486 | 4.52E-15 | 1.847 | 1.583 | 2.155 |
15 | rs7172432 | A | 0.4891 | 0.3721 | 6.66E-10 | 1.616 | 1.387 | 1.882 |
16 | rs8050136 | C | 0.4094 | 0.1699 | 1.05E-40 | 3.386 | 2.815 | 4.073 |
16 | rs9939609 | T | 0.3961 | 0.1729 | 4.94E-36 | 3.139 | 2.611 | 3.773 |
19 | rs10425678 | T | 0.2761 | 0.2109 | 8.66E-05 | 1.427 | 1.194 | 1.705 |
3.2T2DM risk prediction model
The logistic regression model was used to predict the risk of T2DM. The criteria for T2DM were used in the
construction of the analytical model. The classification variance was assumed as
A total of 1563 people were statistically analyzed based on the susceptibility typing results of 21 loci. After constructing the data set, the logistic regression analysis was used to establish the classification prediction model.
In the aforementioned model,
In practical application, the overall evaluation could be judged based on the calculation. When
Refinement evaluation: A
4.Discussion and conclusion
Genetic testing was performed on 1042 patients with diabetes. A total of 1028 confirmed cases of T2DM were detected through model verification. The detection accuracy was 97.5%. Further, 512 Asian health population data were acquired from the 1000G project public database. Based on the analysis results, the aforementioned formula was used for calculation. Of these, 451 individuals had no T2DM. The detection accuracy was 88.1%. The overall detection accuracy was 92.8%.
Previous studies found that early interventions (diet, exercise, medications, and so forth) could slow or even reverse the T2D development because the early onset of T2D was mild, and the current diagnostic criteria failed to detect and diagnose diabetes in most patients as early as possible. This not only delayed prevention and treatment but even worsened the disease. The pathogenesis of T2D is related to not only environmental factors such as high sugar intake and lack of exercise but also genetic factors. It is a complex genetic disease caused by multiple genetic mutations. Many SNPs related to the pathogenesis of T2D have been found with the development of GWAS and meta-analyses. The genes for these loci are located in the cells of the pancreas. They affect cell function by acting on different physiological and pathological processes. Screening for these genetic variants to assess the risk of diabetes is a hot topic in genetic diagnosis. Sequencing and genotyping statistical analysis of genomic DNA extraction in patients with type 2 diabetes with normal blood control group Blood examination and clinical biochemical examination specimen collection to screen susceptible gene SNP site design primers [25, 26, 27, 28].
This study further detected the T2D-related loci in northern China so as to establish the early genetic screening model of Chinese people. A total of 1563 people participated in the study. The T2D case group (Han population in northern China) and the normal control group (1000 Genomics) comprised 1042 and 512 cases. A risk warning model was established for T2DM. The risks of rs10425678, rs10811661, rs10886471, rs1111875, rs12243326, rs13266634, rs17584499, rs1800629, rs1800795, rs1801282, and T2D were closely related. Also, rs2237892, rs2237895, rs2237897, rs4402960, rs5219, rs7172432, rs7403531, rs7903146, rs8050136, rs864745, and rs9939609 were included. First, this study verified that the SNP locus rs2237892 of the KCNQ1 gene was at the start of the T2D risk gene in the T2D population of Han nationality in China. The logistic regression analysis model results were more accurate compared with other models. Moreover, a software tool was developed for diabetes risk assessment in northern China, thus laying the foundation for the development of subsequent gene detection products.
However, a limitation of this study was the insufficient sample size or different frequencies of different susceptible genes in different populations [29, 30, 31, 32]. Therefore, further verification and improvement are needed in this regard. In this study, the SNP locus rs5219 of KCNJ11 in the Chinese population was the same as that reported in the European population, and therefore it was believed that this locus has not been repeated in more samples.
Acknowledgments
This study was financially supported by the Beijing Science and Technology Project (No. Z181100001918015) and the Beijing Municipal Financial Project (No. PXM2019_178305_000019).
Conflict of interest
None to report.
References
[1] | Hu C, Jia W. Diabetes in China: epidemiology and genetic risk factors and their clinical utility in personalized medication. Diabetes. (2018) ; 67: (1): 3–11. |
[2] | Smith CJ, Saftlas AF, Spracklen CN, et al. Genetic risk score for essential hypertension and risk of preeclampsia. Am J Hypertens. (2016) ; 29: (1): 17–24. |
[3] | Masoodi TA, Banaganapalli B, Vaidyanathan V, et al. Computational Analysis of Breast Cancer GWAS Loci Identifies the Putative Deleterious Effect of STXBP4 and ZNF404 Gene Variants. J Cell Biochem. (2017) . |
[4] | Khan SH, Sarwar U. Newer molecular insights into type-2 diabetes mellitus. Journal of the Pakistan Medical Association. (2020) ; 70: (6): 1072–1075. |
[5] | Lau W, Andrew T, Maniatis N. High-resolution genetic maps identify multiple type 2 diabetes loci at regulatory hotspots in african americans and europeans. Am J Hum Genet. (2017) ; 100: (5): 803–816. |
[6] | Song F, Han G, Bai Z, et al. Alzheimer’s disease: genomics and beyond. Int Rev Neurobiol. (2015) ; 121: : 1–24. |
[7] | Selvaraju V, Joshi M, Suresh S, et al. Diabetes, oxidative stress, molecular mechanism, and cardiovascular disease – an overview. Toxicol Mech Methods. (2012) ; 22: (5): 330–335. |
[8] | Manolio TA, Collins FS, Cox NJ, et al. Finding the missing heritability of complex diseases. Nature. (2009) ; 461: (7265): 747–753. |
[9] | Wu H, Pan P, He Y, et al. A risk model for prediction of diabetes in Chinese adults. Chinese Journal of Health Management. (2007) ; 1: (2): 95–98. |
[10] | Chien K, Cai T, Hsu H, et al. A prediction model for type2 diabetes risk among Chinese people. Diabetologia. (2009) ; 52: (3): 443–450. |
[11] | Sullivan LM, Massaro JM, et al. Presentation of multivariate data for clinical use: the framingham study risk score functions. StatMed. (2004) ; 23: : 1631–1660. |
[12] | Amberger JS, Hamosh A. Searching online mendelian inheritance in man (OMIM): a knowledgebase of human genes and genetic phenotypes. Curr Protoc Bioinformatics. (2017) ; 58: : 1.2.1–1.2.12. Published 2017 Jun 27. doi: 10.1002/cpbi.27. |
[13] | MacArthur J, Bowler E, Cerezo M, et al. The new NHGRI-EBI Catalog of published genome-wide association studies (GWAS Catalog). Nucleic Acids Res. (2017) ; 45: (D1): D896–D901. doi: 10.1093/nar/gkw1133. |
[14] | Fishilevich S, Zimmerman S, Kohn A, et al. Genic insights from integrated human proteomics in GeneCards. Database (Oxford). (2016) ; 2016: baw030. Published 2016 Apr 5. doi: 10.1093/database/baw030. |
[15] | Stenson PD, Mort M, Ball EV, et al. The Human Gene Mutation Database: towards a comprehensive repository of inherited mutation data for medical research, genetic diagnosis and next-generation sequencing studies. Hum Genet. (2017) ; 136: (6): 665–677. doi: 10.1007/s00439-017-1779-6. |
[16] | Voight BF, Scott LJ, Steinthorsdottir V, et al. Twelve type 2 diabetes susceptibility loci identified through large-scale association analysis. Nat Genet. (2010) ; 42: (7): 579–589. |
[17] | Sladek R, Rocheleau G, Rung J, et al. A genome-wide association study identifies novel risk loci for type 2 diabetes. Nature. (2007) ; 445: (7130): 881–885. |
[18] | Steinthorsdottir V, Thorleifsson G, Reynisdottir I, et al. A variant in CDKAL1 influences insulin response and risk of type 2 diabetes. Nat Genet. (2007) ; 39: (6): 770–775. |
[19] | Unoki H, Takahashi A, Kawaguchi T, et al. SNPs in KCNQ1 are associated with susceptibility to type 2 diabetes in East Asian and European populations. Nat Genet. (2008) ; 40: (9): 1098–1102. |
[20] | Zeggini E, Scott LJ, Saxena R, et al. Meta-analysis of genome-wide association data and large-scale replication identifies additional susceptibility loci for type 2 diabetes. Nat Genet. (2008) ; 40: (5): 638–645. |
[21] | Rung J, Cauchi S, Albrechtsen A, et al. Genetic variant near IRS1 is associated with type 2 diabetes, insulin resistance and hyperinsulinemia. Nat Genet. (2009) ; 41: (10): 1110–1115. |
[22] | Shu XO, Long J, Cai Q, et al. Identification of new genetic risk variants for type 2 diabetes. PLoS Genet. (2010) ; 6: (9): e1001127. |
[23] | Tsai FJ, Yang CF, Chen C, et al. A genome-wide association study identifies susceptibility variants for type 2 diabetes in Han Chinese. PLoS Genet. (2010) ; 6: (2): e1000847. |
[24] | Li C, Yuhua X, Zhiyong P, et al. NGS-based gene targeting panel design of type 2 diabetes susceptible genes and its clinical application. Chinese Journal of Diabetes. (2019) . |
[25] | Hara K, Fujita H, Johnson TA, et al. Genome-wide association study identifies three novel loci for type 2 diabetes. Hum Mol Genet. (2014) ; 23: (1): 239–46. |
[26] | Mansoori Y, Daraei A, Naghizadeh M, et al. Significance of a common variant in the CDKAL1 gene with susceptibility to type 2 diabetes mellitus in Iranian population. Adv Biomed Res. (2015) ; 4: : 45. |
[27] | Ren Q, Han X, Tang Y, et al. Search for genetic determinants of sulfonylurea efficacy in type 2 diabetic patients from China. Diabetologia. (2014) ; 57: (4): 746–53. |
[28] | Yasuda K, Miyake K, Horikawa Y, et al. Variants in KCNQ1 are associated with susceptibility to type 2 diabetes mellitus. Nat Genet. (2008) ; 40: (9): 1092–7. |
[29] | Rastegari A, Rabbani M, Sadeghi HM, et al. Association of KCNJ11 (E23K) gene polymorphism with susceptibility to type 2 diabetes in Iranian patients. Adv Biomed Res. (2015) ; 4: : 1. |
[30] | Sun X, Sui W, Wang X, et al. Whole-genome re-sequencing for the identification of high contribution susceptibility gene variants in patients with type 2 diabetes. Mol Med Rep. (2016) ; 13: (5): 3735–46. |
[31] | Cui B, Zhu X, Xu M, et al. A genome-wide association study confirms previously reported loci for type 2 diabetes in Han Chinese. PLoS One. (2011) ; 6: (7): e22353. |
[32] | Lu J, Luo Y, Wang J, et al. Association of type 2 diabetes susceptibility loci with peripheral nerve function in a Chinese population with diabetes. J Diabetes Investig. (2016) . |