Effect of potassium channel noise on nerve discharge based on the Chay model
Abstract
BACKGROUND:
The nervous system senses and transmits information through the firing behavior of neurons, and this process is affected by various noises. However, in the previous study of the influence of noise on nerve discharge, the channel of some noise effects is not clear, and the difference from other noises was not examined.
OBJECTIVE:
To construct ion channel noise which is more biologically significant, and to clarify the basic characteristics of the random firing rhythm of neurons generated by different types of noise acting on ion channels.
Method:
Based on the dynamics of the ion channel, we constructed ion channel noise. We simulated the nerve discharge based on the Chay model of potassium ion channel noise, and used the nonlinear time series analysis method to measure the certainty and randomness of nerve discharge.
RESULTS:
In the Chay model with potassium ion noise, the chaotic rhythm defined by the original model could be effectively unified with the random rhythm simulated by the previous random Chay model into a periodic bifurcation process.
CONCLUSION:
This method clarified the influence of ion channel noise on nerve discharge, better understood the randomness of nerve discharge and provided a more reasonable explanation for the mechanism of nerve discharge.
1.Introduction
Neural discharge is the basis of the neuron coding mechanism. The nervous system senses and transmits information through the discharge behavior of neurons [1, 2, 3, 4, 5]. The basis of discharge is the charged ion flow formed by selective ion channels on the membrane of neurons that allow different ions to enter and exit cells [6, 7]. At first, the essence of neuron discharge rhythm was studied based on ionic current modeling. Neural discharge rhythm was studied under the deterministic model. The deterministic model could simulate multiple periodic and chaotic rhythms of experimental nerve starting points, and simulate the transition modes among the various discharge rhythms [8, 9, 10].
K
Because of the complex non-linear structure of neurons and the random factors within the nervous system, the discharge has a complex discharge rhythm. Neurons are affected by various noises in the biological process of transmitting information, such as the randomness of the opening and closing of ion channels, the randomness of neurotransmitters released by chemical synapses, the randomness of the movement of surrounding molecules, and the randomness of input signals of other neurons [15, 16]. Hence it is very important to study the effect of noise on the nervous system. Previous concepts have suggested that noise is harmful to some discharge behaviors, but noise is necessary for the occurrence of some important dynamic processes in non-linear systems.
Previous studies on the influence of noise on nerve discharge have achieved rich results. The synergistic effect of noise and system is beneficial to the output of system signals, which is different from people’s intuitive understanding of noise [17, 18]. Many scholars have studied the effects of white noise, additive noise, phase noise, conductance-based noise and non-Gaussian noise on the random discharge of neurons, suggesting that noise has an important influence on the discharge activity of neurons, so it may participate in the information processing of nervous system, which is helpful to explore the function of randomness in nerve stimulation [19, 20, 21, 22, 23, 24]. Studying the rhythm of nerve discharge and the role of noise in nervous system movement is helpful to discover the general law of nerve movement and the existence and significance of noise in life activities.
Although many achievements have been made in the past on the effect of noise on neuronal discharge, there are still some problems in the study of noise-related neuronal random discharge rhythm, which have not been well solved or reasonably explained. These include: 1. The channel of some noise effects was not clear. Most previous studies used the neuron model to explain the characteristics of nerve discharge and used white Gaussian noise as simple additive noise, which was added to the formula of neuron membrane potential, thus ignoring the effect of specific noise on which channel. 2. Some studies on the effect of adding noise to an ion channel item have not examined the difference from other noises.
In view of the existing problems, this paper focused on the construction of ion channel noise with more realistic and biologically meaningful neuron discharge models, and studied the discharge rhythm of neurons. We aimed to achieve the following objectives: 1. To clarify the basic characteristics of the random discharge rhythm of neurons generated by different types of noise acting on ion channels, and to elaborate the reasonable mechanism of the generation of the rhythm; 2. To clarify the difference between the ion channel noise and the overall additive noise in the generation of neuron discharge.
2.Materials and method
2.1The Chay model
The Chay model (CM) is an ion channel model that describes the complex rhythm of nerve discharge. It can simulate many kinds of periodic and chaotic rhythms of experimental nerve starting points, and the transitional patterns among various rhythms. It can be described as follows:
(1)
(2)
(3)
where
The CM could only simulate some periodic discharge and chaotic discharge, and the random rhythm of periodic alternation could be simulated by adding noise to the CM. However, the channel of noise effect was not clear. Most previous studies used neuron models to explain the characteristics of nerve discharge used white Gaussian noise as simple additive noise, which was added to the formula of neuron membrane potential, thus ignoring the effect of specific noise on which channel. This paper focused on the study of the effect of ion channel noise on nerve discharge.
The sources of ion channel noise included many aspects. Here we could make some assumptions according to the dynamic behavior of ion channel. We believed that the random transition of the opening and closing of the ion channel led to the noise of the ion channel, which was the internal noise of the neuron, thus increasing the random fluctuation of the ionic conductance and eventually leading to the generation of complex discharge rhythm, as shown below.
The two parameters represent the transition probability from one state to another. This stochastic transition process was considered as a Markov process, and the corresponding polynomial of ion channel noise can be referred to as follows [25]:
(4)
where
In order to characterize more realistic and biologically meaningful neuron discharge models, the effect of channel noise on neuron discharge was studied. The Gauss white noise of potassium channel was added to the Eq. (2) of the CM system, Eqs (1), (3), (5) constituted a random CM based on Gauss white noise of fast potassium channel.
(5)
(6)
2.2Time series analysis methods
Whether for model simulation data or biological experimental data, we aimed to identify its basic properties through a series of reasonable index analysis, such as the basic dynamic properties to identify whether the rhythm is deterministic or random.
In this paper, the time interval between two adjacent discharge peaks was defined as interspike intervals (ISI). Then the one-dimensional non-linear time series of ISI was analyzed by means of surrogate data (SD), first return map (FRM), autocorrelation coefficient (ACC), nonlinear prediction (NPE) and other methods [22], so as to characterize the stochastic and deterministic characteristics of discharge rhythm and to carry out its signal identification of the source. We intend to analyze the discharge rhythm on the premise of the same long-term dynamic bifurcation background, combined with the non-linear time series analysis method and other traditional statistical methods. The reason for choosing the same bifurcation background is that even though some non-linear indices are the same or similar, the same rhythm cannot be easily determined because they are in different bifurcation backgrounds, such as one between discharge and rest, one between cycle 1 discharge and cycle 2 discharge. The combination of non-linearity and other indicators is due to the misjudgment of the conclusions obtained by relying solely on non-linearity indicators, and the union of other methods often leads to unexpected results, such as the combination of nonlinear prediction and FRM with SD, which can effectively judge the non-linearity determination mechanism of the original data.
3.Results
In the CM, the Gauss white noise and the Gauss white noise of the potassium channel were added respectively. Then, the simulation was carried out when
Figure 2.
PAB in the CM (
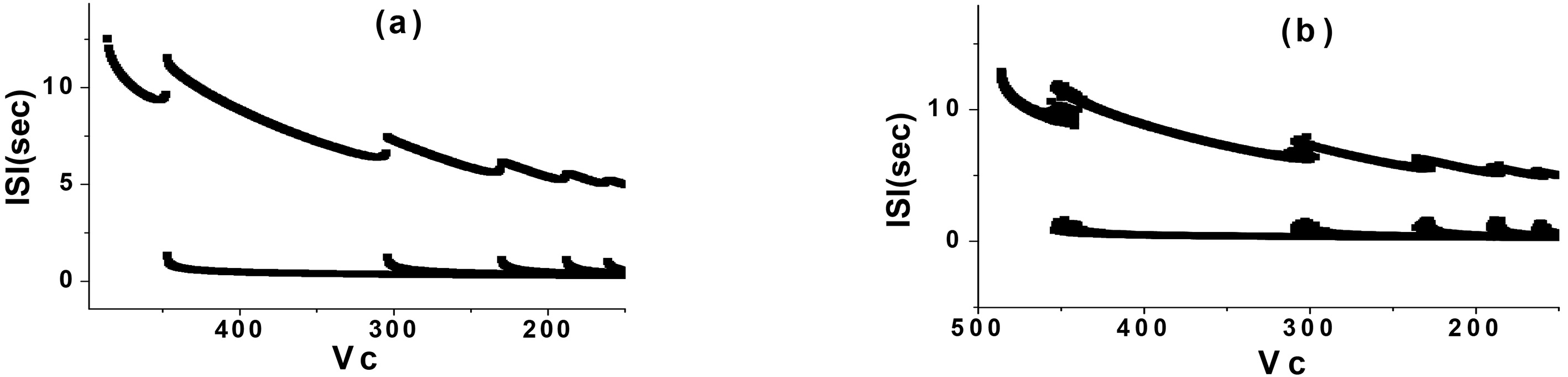
By comparison, it was found that when Gauss white noise was added to the CM with the above parameters, the discharge rhythm was directly from one periodic bursting to another periodic bursting near each bifurcation in the process of PAB, and no other type of rhythm appeared. However, a PAB mode with random rhythm could be generated when the Gauss white noise of potassium channel was added to the same parameters. The random rhythm was located near the bifurcation point of two adjacent periodic bursting, which occurred alternately by two periodic bursting, as shown in Fig. 2b. It was obviously different from the bifurcation process of CM with Gauss white noise in this parameter interval.
The random rhythm near the bifurcation point of period 2 and 3 bursting (Fig. 2b) was selected for analysis. When
Figure 3.
Stochastic firing trajectory diagram with period 2 and 3 bursting (
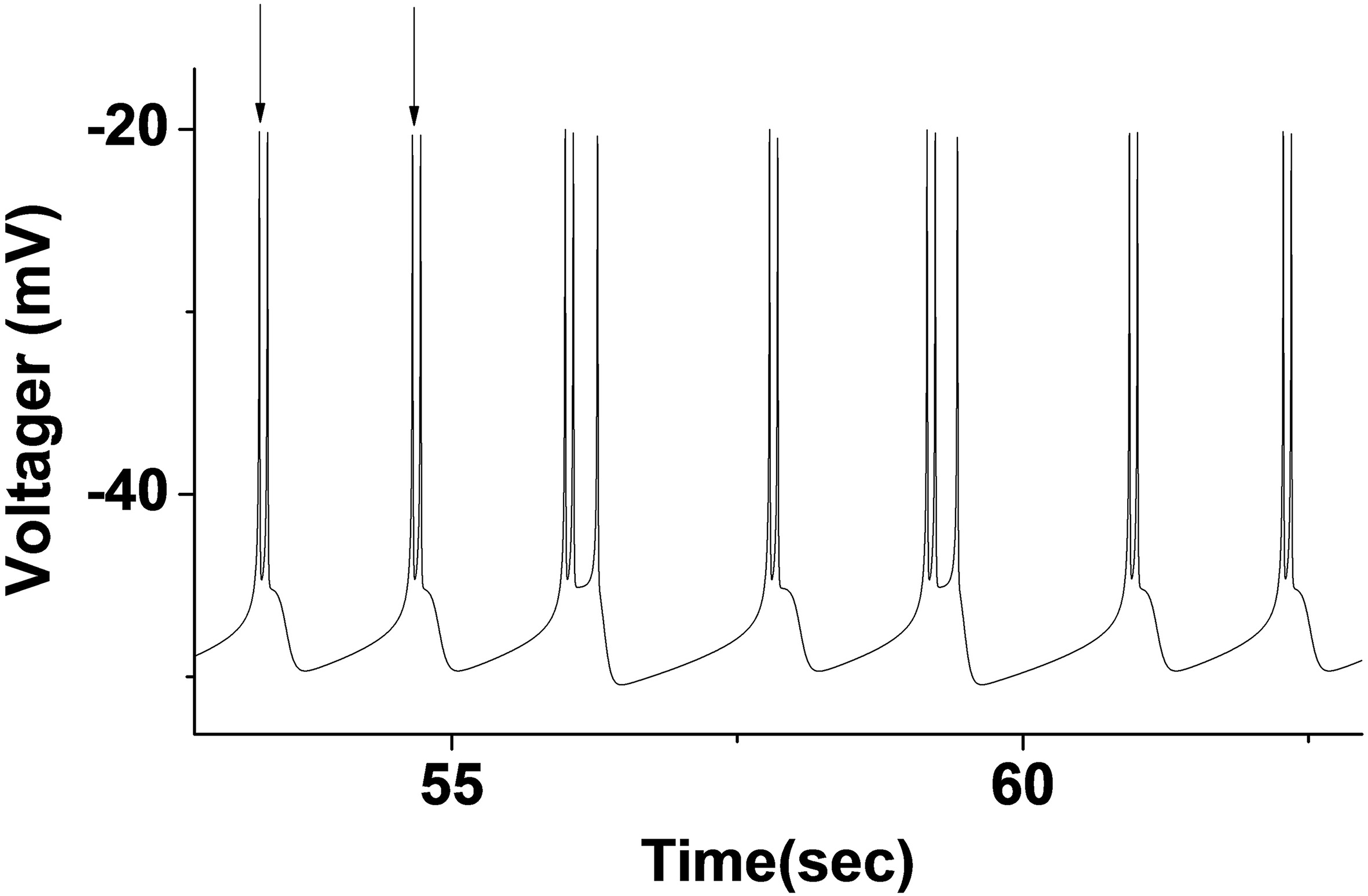
The alternating random rhythms of period 2 and 3 bursting in CM simulation with potassium channel noise were analyzed as examples. The ISI sequence of the discharge trajectory of this rhythm was analyzed by various indexes and the results are shown in Fig. 4. There were four kinds of ISI in this rhythm. The smallest ISI was the one by period 2 and 3 bursting, which was reflected by four independent peaks in its statistical histogram. The FRM of ISI sequence was four centralized point clusters, but the FRM of its SD presents 16 point clusters with approximately uniform distribution, which was significantly different from the FRM of the primitive ISI sequence. Because the SD were random, it showed that the ISI sequence had a definite structure and that the rhythm was deterministic. The autocorrelation coefficient of this rhythm decreased gradually from 1 to 0 with the increase of delay in a short time, and oscillates only near 0 when the delay was large. There was a strong self-correlation. When Lag
Figure 4.
Analysis on ISI. (a) ISI series, (b) ISI histogram, (c) FRM, (d) FRM of SD of ISI, (e) ACC, (f) NPE.
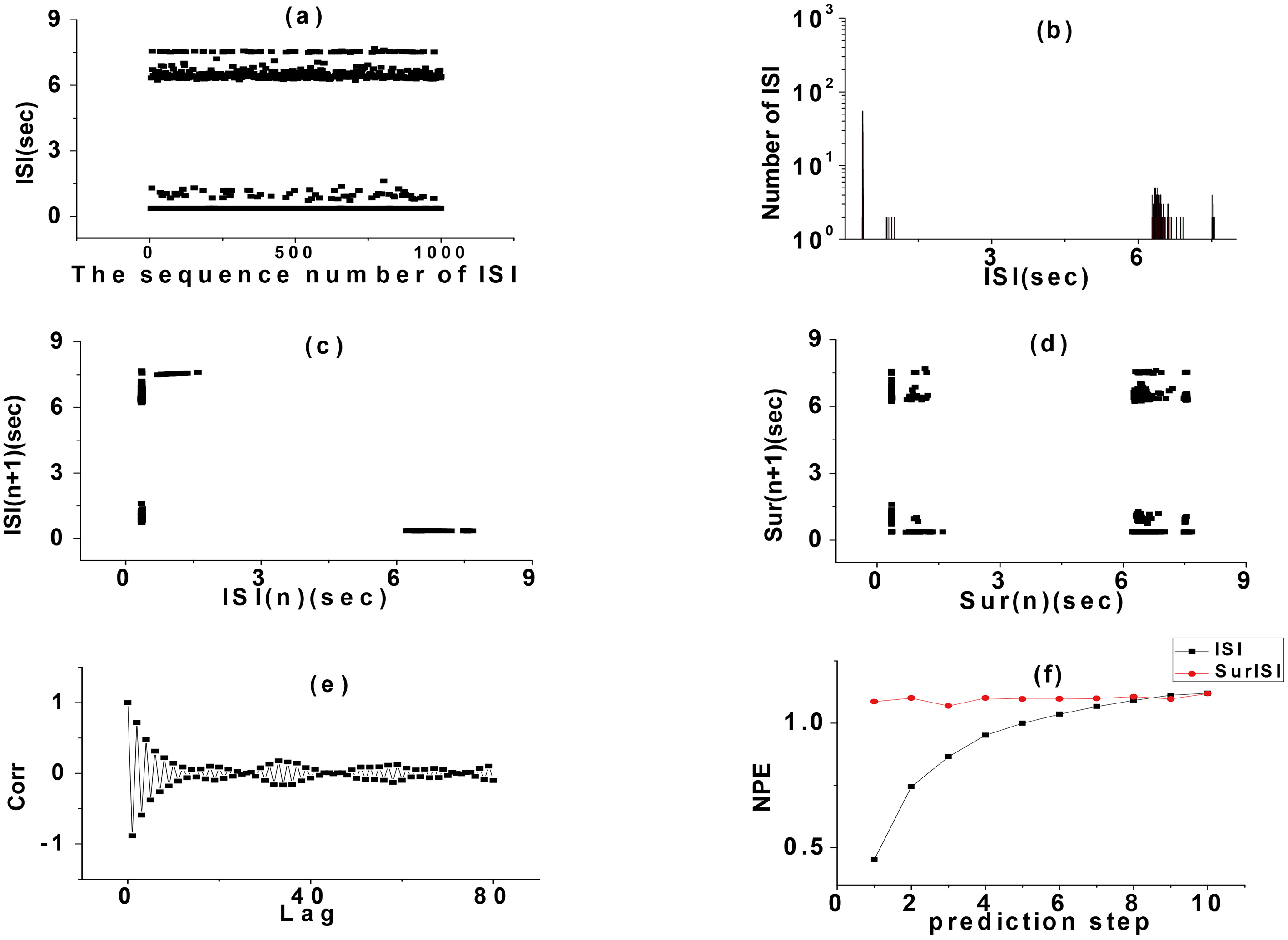
The results of the non-time series analysis of ISI of this random rhythm showed that the rhythm had a certain randomness. In order to further verified the randomness of the rhythm, we had carried out a more detailed analysis. Since the random rhythm only contains period 2 and bursting, a single period bursting could be defined as an event (period 2 or 3 bursting), and the time interval between two consecutive period 2 or 3 bursting was recorded as an inter-event interval (IEI). Taking period 2 bursting as an example, we considered the time interval between two consecutive period 2 bursting as IEI. As shown in the interval between two vertical arrows (Fig. 2), we analyzed the IEI of this random rhythm. The results of IEI sequence analysis of period 2 bursting are shown in Fig. 5. The IEI presented a typical integer multiple rhythm. The FRM of IEI was a typical lattice structure with integer multiple rhythm, which was not significantly different from the FRM of its SD, indicated the randomness of the IEI sequence. Its statistical histogram was approximately like the exponential attenuation multimodal distribution of integer multiple rhythm. The autocorrelation coefficient oscillates near zero when the delay was not zero. The nonlinear prediction was unpredictable regardless of the prediction step size error was close to 1. The approximate entropy of the IEI series was 0.479114, which also indicated that the IEI series was a stochastic non-renewal process.
Figure 5.
Analysis on IEI. (a) IEI series, (b) IEI histogram, (c) FRM, (d) FRM of SD of IEI, (e) ACC, (f) NPE.
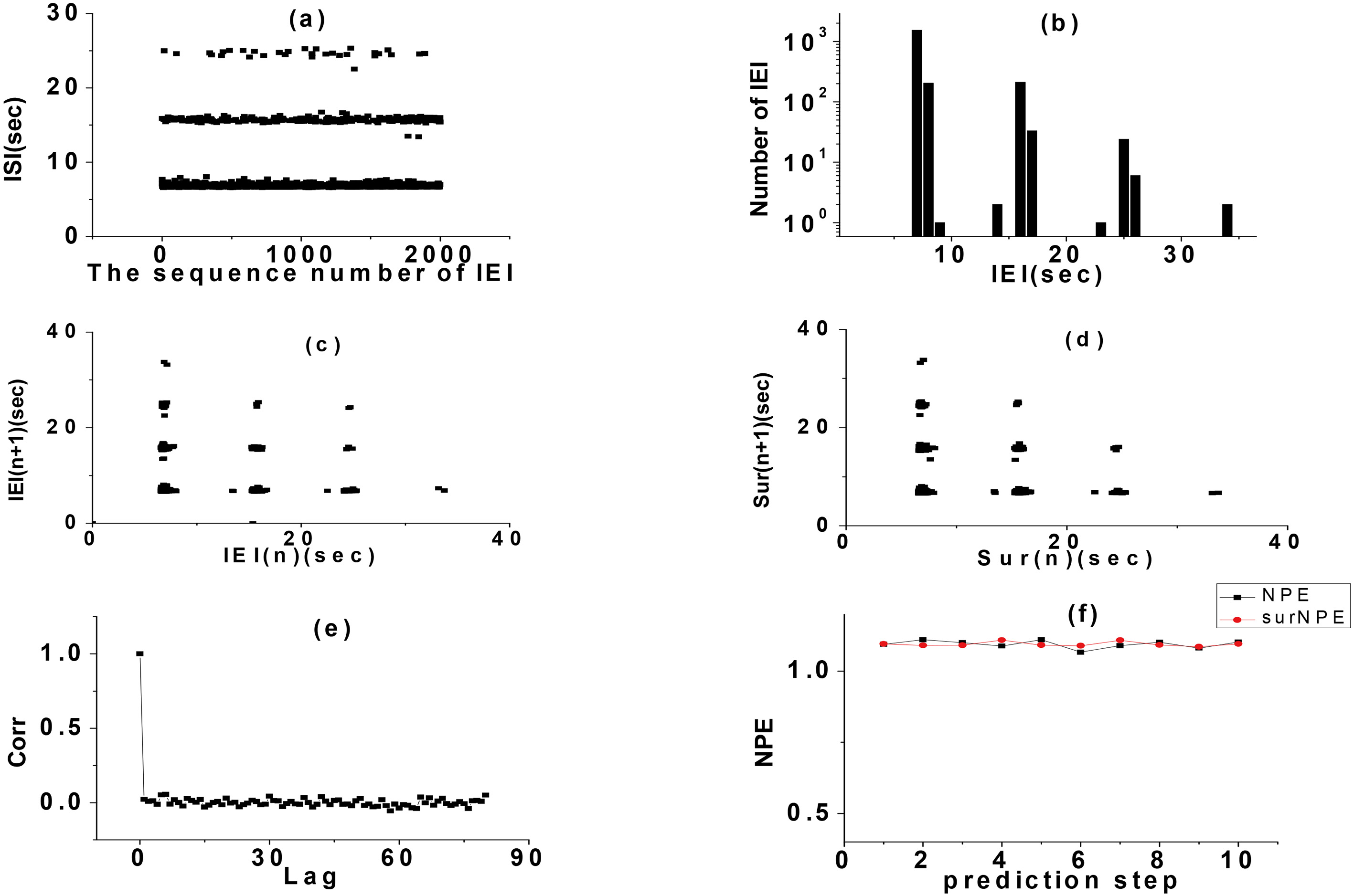
When
Figure 6.
PAB in the CM (
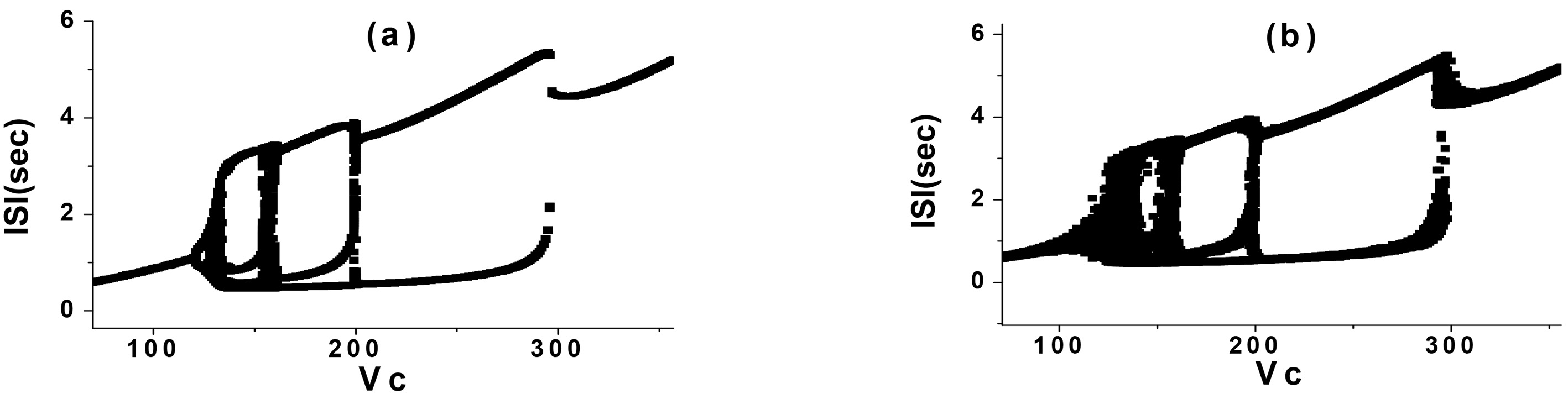
Figure 7.
Stochastic firing trajectory diagram with period 1 and 2 bursting (
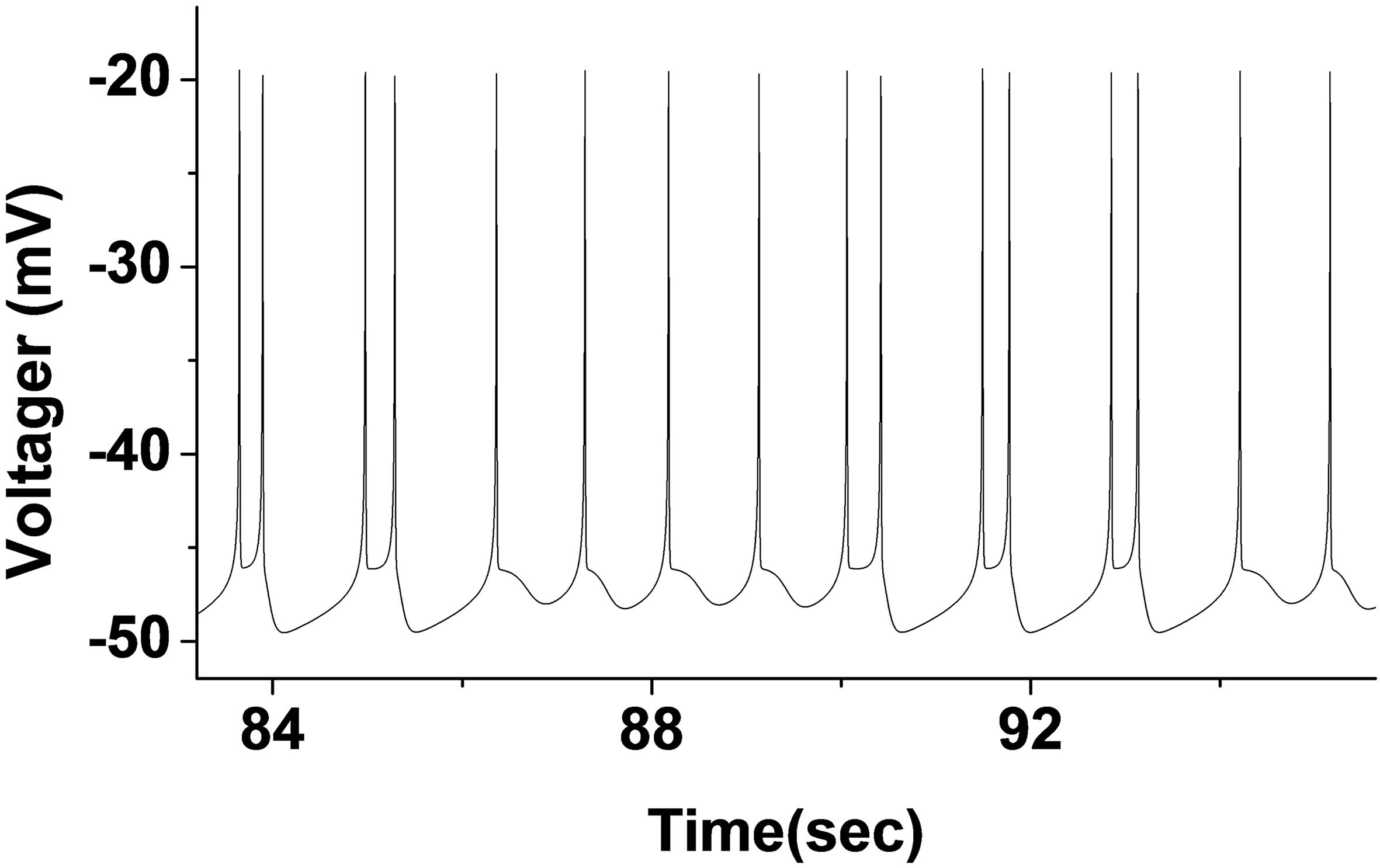
In order to analyze the characteristics of the chaotic discharge rhythm, we took the chaotic rhythm near the bifurcation points of the period 2 and 3 bursting (Fig. 6b) as an example. The amplification diagram is shown in Fig. 8a. We chose the discharge rhythm when
Figure 8.
(a) bifurcation sequence between period 2 and 3 bursting, (b) chaotic discharge rhythm trajectory diagram with period 2 and 3 bursting (
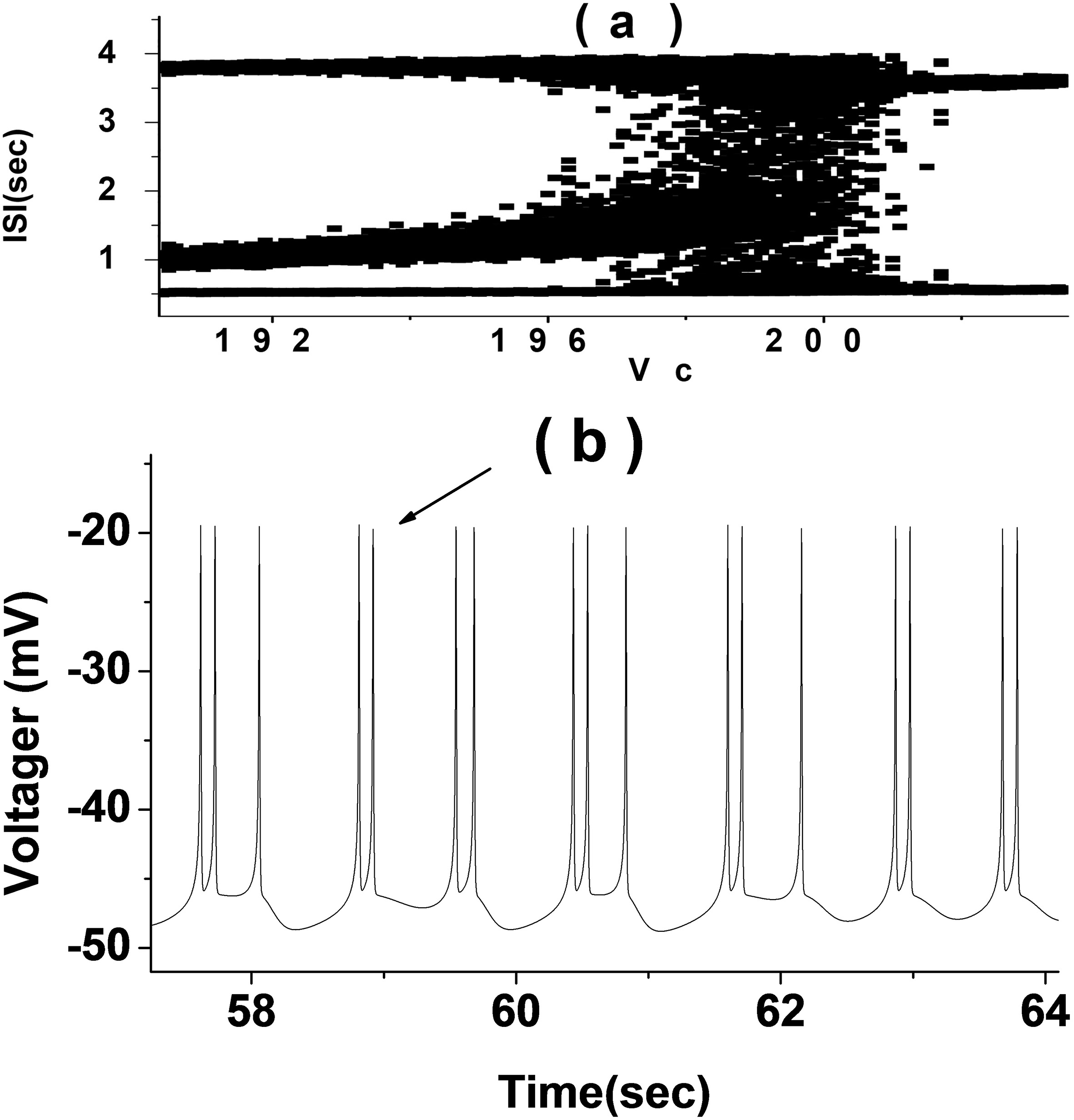
The ISI sequence of chaotic rhythm at bifurcation points of period 2 and 3 bursting was analyzed by non-time series, and the results are shown in Fig. 9. There were several ISI bands and some scattered points between bands in the sequence diagram of this rhythm, which were reflected in the continuous distribution in its statistical histogram. The FRM of ISI had obvious geometric shape, and the figure was horseshoe-shaped single-peak mapping, as shown in Fig. 9c. The autocorrelation coefficient of this rhythm decreased gradually from 1 to 0 with the increase of delay in a short time, and oscillated only near 0 when the delay was large. There was a strong self-correlation. When Lag
Figure 9.
Analysis of chaotic rhythm ISI between period 2 and 3 bursting. (a) ISI series, (b) ISI histogram, (c) ACC, (D) NPE.
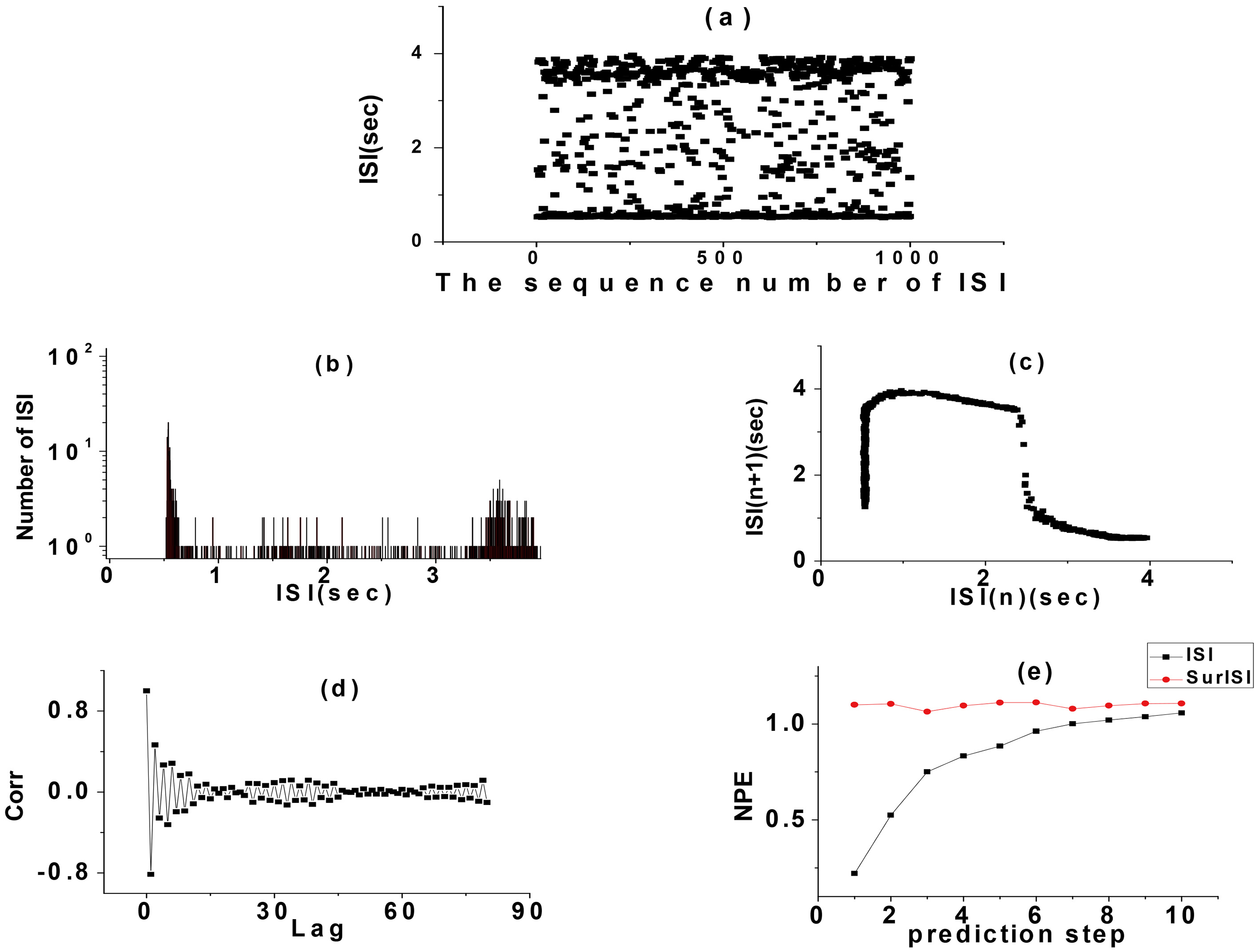
The nonlinear prediction of ISI was short-term predictable and long-term unpredictable, while the SD of ISI were unpredictable regardless of the forecasting step length. The approximate entropy of calculating the non-periodic rhythm ISI sequence was 0.435132. All the above indicators showed that the rhythm had certain certainty.
4.Discussion and conclusion
This study is based on a thorough investigation of previous studies. In order to depict a more realistic and biologically meaningful neuron discharge model, the effects of channel noise on neuron discharge were studied from ion channels. The sources of ion channel noise include many aspects. Based on the assumption of the dynamic behavior of ion channel, we considered that the random transition of the opening and closing of ion channel leads to the noise of ion channel. Thus, the noise of ion channel based on the dynamic behavior of ion channel was constructed to make the noise more specific and biologically meaningful, so that we could study the effect of specific noise on ion channel. Through simulation analysis, it was found that the generation of internal noise in this neuron could change the reliability of pulse sequence, increased random fluctuation of ion conductance, and eventually led to the generation of complex discharge rhythm.
By constructing potassium channel noise, we established a random CM based on potassium channel noise, which fully realized the discharging rhythm of neurons induced by potassium channel noise, and compared the dynamic characteristics with the data simulated by random model with simple additive global noise. The difference between potassium channel noise and global additive noise in generating neuron random discharge was clarified. It was found that under the same noise intensity, the noise of potassium channel was more likely to cause the random discharge rhythm near the bifurcation point of the adjacent period, which had a greater impact on the nerve discharge. CM with potassium channel noise could not only simulate periodic alternating random rhythm and chaotic discharge rhythm, but also effectively integrated random alternating rhythm and chaotic rhythm into the same bifurcation process, which was the biggest difference from CM with simple additive global noise. It not only clarified the effect of specific noise on neuronal discharge, but also provided a more reasonable explanation for the mechanism of nerve discharge rhythm. The joint analysis of random rhythms and chaotic discharges near bifurcation points using multiple indicators is expected to be an effective method for the analysis of irregular nerve discharges, and also provide reference for the identification of other similar physiological signals.
In this paper, the effect of potassium ion channel noise on nerve discharge was studied, which not only specified the noise, but also analyzed it on the basis of nerve discharge. The basis of discharge is the charged ion flow formed by the selective admission of different ions into and out of the cell membrane by various ion channels on the neuron cell membrane. Therefore, analyzing the phenomenon of nerve discharge from the perspective of ion channel behavior may essentially grasp the essence of information transmission by nerve coding. In this paper, we only studied the effects of potassium channel noise and total noise, because we assumed that channel noise is caused by random opening and closing of ion channels. The second equation in the Chay model describes the probability of opening potassium channels, so we studied the effect of noise on potassium channels based on this basis. Next, we can consider the effect of other channel noise on discharge, such as calcium ion and sodium ion.
Future work should continue to focus on ion channels. In our previous articles, we considered the mechanism of peak generation of nerve discharge, made reasonable assumptions about the probability of K
Acknowledgments
This research was supported by the Shandong Provincial Natural Science Foundation, China (no. ZR2018LF005), the National Key Research and Development Program of China (no. 2016YFC0106000), the Natural Science Foundation of China (nos 61302128, 61573166, 61572230), and the Youth Science and Technology Star Program of Jinan City (no. 201406003).
Conflict of interest
None to report.
References
[1] | Freeman WJ. Mesoscopic neurodynamics: From neuron to brain. Journal of Physiology-Paris. (2000) ; 94: (5-6): 303-322. |
[2] | Ma J, Xu J. An introduction and guidance for neurodynamics. Science Bulletin. (2015) ; 60: (22): 1969-1971. |
[3] | Yang Y, Solis-Escalante T, Van DHF, Schouten A. A generalized coherence framework for detecting and characterizing nonlinear interactions in the nervous system. IEEE Trans Biomed Eng. (2008) ; 25: (4): 401-410. |
[4] | Jiang Z, Wang D, Wu P, Chen Y, Shang H, Wang L, Xie H. Predicting subcellular localization of multisite proteins using differently weighted multi-label k-nearest neighbors sets. Technology and Health Care. (2019) ; 27: (S1): 185-193. |
[5] | Zhao Z, Gu H. Identifying time delay-induced multiple synchronous behaviours in inhibitory coupled bursting neurons with nonlinear dynamics of single neuron. Procedia IUTAM. (2017) ; 22: : 160-167. |
[6] | Vatanparast J, Andalib-Lari F. Camphor elicits epileptiform discharges in snail neurons: The role of ion channels modulation. NeuroToxicology. (2017) ; 60: : 299-307. |
[7] | David A. Brown: Regulation of neural ion channels by muscarinic receptors. Neuropharmacology. (2018) ; 136: : 383-400. |
[8] | Huang S, Zhang J, Wang M, Hu C. Firing patterns transition and desynchronization induced by time delay in neural networks. PHYSICA A. (2018) ; 499: : 88-97. |
[9] | Jia B, Gu H, Xue L. A basic bifurcation structure from bursting to spiking of injured nerve fibers in a two-dimensional parameter space. Cognitive Neurodynamics. (2017) ; 11: (2): 1-12. |
[10] | Bao BC, Wu PY, Bao H, Xu Q, Chen M. Numerical and experimental confirmations of quasi-periodic behavior and chaotic bursting in third-order autonomous memristive oscillator. Chaos Soliton Fract. (2018) ; 106: : 161-170. |
[11] | Fullick A. Edexcel A2 Biology. Pearson Education Limited, Harlow, United Kingdom, (2009) . |
[12] | Pongs O. Voltage-gated potassium channels: From hyperexcitability to excitement. FEBS Letters. (1999) ; 452: (1-2): 31-35. |
[13] | Rasmussen R, Jensen MH, Heltberg ML. Chaotic dynamics mediate brain state transitions, driven by changes in extracellular ion concentrations. Cell Systems. (2017) ; 5: (6): 591. |
[14] | Xu Y, Jia Y, Ge M, Lu L, Yang L, Zhan X. Effects of ion channel blocks on electrical activity of stochastic hodgkin-huxley neural network under electromagnetic induction. Neurocomputing. (2018) ; 283: : 196-204. |
[15] | Sharma SK, Haobijam D, Singh SS, Malik MZ, Singh RB. Neuronal communication: Stochastic neuron dynamics and multi-synchrony states. AEU – International Journal of Electronics and Communications. (2019) ; 100: : 75-85. |
[16] | Lindner B, García-Ojalvo J, Neiman A, Schimansky-Geier L. Effects of noise in excitable systems. Physics Reports. (2004) ; 392: (6): 321-424. |
[17] | Ren R, Deng K. Noise and periodic signal induced stochastic resonance in a Langevin equation with random mass and frequency. Physica A: Statistical Mechanics and its Applications. (2019) ; 523: : 145-155. |
[18] | Yang B, Zhang X, Zhang L, Luo M. Collective behavior of globally coupled langevin equations with colored noise in the presence of stochastic resonance. Phys Rev E. (2016) ; 94: (2): 022119. |
[19] | Shang H, Xu R, Wang D, Zhou J, Han S. a stochastic neural firing generated at a hopf bifurcation and its biological relevance. In: International Conference on Neural Information Processing, (2017) ; pp. 553-562. |
[20] | Shang H, Jiang Z, Wang D, Chen Y, Zhou J, Wu P, Han S. Dynamical analysis of a stochastic neuron spiking activity in the biological experiment and its simulation by I Na, P+ I K Model. In: International Symposium on Neural Networks, (2018) ; pp. 850-859. |
[21] | Shang H, Xu R, Wang D. Dynamic analysis and simulation for two different chaos-like stochastic neural firing patterns observed in real biological system. In: International Conference on Intelligent Computing, (2017) ; pp. 749-757. |
[22] | Shang H, Jiang Z, Xu R, Wang D, Wu P, Chen Y. The dynamic mechanism of a novel stochastic neural firing pattern observed in a real biological system. Cogn Syst Res. (2019) ; 53: : 123-136. |
[23] | Gutkin BS, Jürgen J, Tuckwell HC. Inhibition of rhythmic neural spiking by noise: the occurrence of a minimum in activity with increasing noise. Die Naturwissenschaften. (2009) ; 96: (9): 1091-1097. |
[24] | Li D, Cui X, Yang Y. Inverse stochastic resonance induced by non-Gaussian colored noise. Neurocomputing. (2018) ; 287: : 52-57. |
[25] | Yu H, Galán RF, Wang J, Cao Y, Liu J. Stochastic resonance, coherence resonance, and spike timing reliability of hodgkin – huxley neurons with ion-channel noise. Physica A: Statistical Mechanics and its Applications. (2017) ; 471: : 263-275. |
[26] | Jiang Z, Wang D, Sun J, Shi H, Shang H, Chen Y. Simulation of a chaos-like irregular neural firing pattern based on improved deterministic Chay model. In: International Symposium on Neural Networks, (2019) ; pp. 278-287. |