The effect of external voltage stimulation on absence seizures
Abstract
BACKGROUND:
Absence epilepsy (AE) is a systemic disease of the brain, which mainly occurs during childhood and adolescence. The control mechanism is still unclear, and few theoretical studies have been conducted to investigate this.
OBJECTIVE:
In this paper, we employed external direct voltage stimulation in the subthalamic nucleus to explore mechanisms that inhibit absence seizures.
METHODS:
All simulation results are obtained by the four-order Runge-Kutta method in the MATLAB environment. The inhibition mechanism can be inferred from the results.
RESULTS:
We found that the seizures may be inhibited by tuning the strength of the voltage to suitable ranges. This regulation may be achieved through the competition between the inhibitory projections from the basal ganglia to the thalamus.
CONCLUSION:
Because the mechanism underlying the treatment of epilepsy with a uniform direct current electric field is unclear, we hope that these results can inspire further experimental studies.
1.Introduction
Absence epilepsy (AE) is a systemic disease of the brain, which mainly occurs during childhood and adolescence [1] and without any previous symptoms. AE patients often exhibit loss of consciousness, activity interruption, motionlessness, a slight loss of tension, and tonic-clonic seizures for short durations. Its main clinical electrophysiological features are bilateral synchronous slow spike-wave discharges (SWDs), the frequency of which is very low, approximately 2–4 Hz [2]. Many experiments have implied that AE may be induced via abnormal discharge activity in the thalamocortical circuits (TC) of the bilateral parts of the brain. Many large-scale thalamocortical computational models have been employed to explore the onset mechanism of epilepsy, and the results obtained can be compared well to experimental experiments and observations [3, 4, 5]. Marten et al. showed that AE can result from abnormal excitatory inputs from the cortex to the thalamic relay nucleus (SRN) [6, 7]. Chen et al. found that by changing the inhibitory coupling strength and the signal transmission delay from the thalamic reticular nucleus (TRN) to the SRN, the brain may be changed into a seizure state [8]. Hu et al. observed that seizure states in a TC model can be induced by varying the coupling strengths of some excitatory pathways connected to the SRN [9]. However, the control mechanism of epilepsy is still unclear, and few theoretical studies have been conducted to investigate this mechanism.
Deep brain stimulation (DBS) is a common method to control intractable epilepsy with clear effects on the patient [10]. For example, Vesper et al. treated a patient with progressive myoclonic epilepsy by using chronic high frequency DBS and found that bilateral unipolar DBS can reduce the frequency and strength of seizures by 50% by stimulating the subthalamic nucleus (STN) [11]. In 2013, Jou et al. employed high frequency and low intensity current in the left anterior thalamic nucleus of a rat model and found that seizures can be effectively reduced [12]. Feng et al. used DBS on the dorsal part of the STN in a transgenic rat model to explore its controlling effects on drug-resistant epilepsy [13]. Pasnicu et al. showed that low- and high-frequency stimulation of the central nucleus of the thalamus (THA) can effectively suppress seizure phenomena and their frequencies [14]. This is the first time that DBS was shown to effectively inhibit local seizures by acting on the ipsilateral central nucleus of the thalamus. In theoretical research, Chen et al. showed that the basal ganglia (BAG) may have a bidirectional, mediated function on absence seizures [8] and that the direct pallido-cortical pathway can regulate seizures [15]. Recently, Sorokin et al. found a bidirectional adjustment phenomenon in a generalized epilepsy network via rapid real-time switching of phasic firing and tonic firing modes in the TC [16]. Hu et al. found that AE may be inhibited by adjusting the firing ability of neurons of the thalamus and striatum [17]. Due to technical challenges posed by uniform direct current (DC) electric field invasive cortical stimulation, few studies have investigated DC field control of seizures, especially in the field of mathematical theoretical modelling. Ghai et al. found that DC fields can mediate low-calcium epileptiform activity by directly polarizing CA1 pyramidal neurons [18]. Radman et al. found that cortical neuron morphology and type are key to determining the sensitivity to supra- and sub-threshold uniform electric fields [19]. Liebetanz et al. noted that the anticonvulsant roles of transcranial direct-current stimulation (tDCS) depend on stimulation duration and current strength, which may point to tDCS as a potential tool to control partial seizures [20]. Recently, some studies focused on the optogenetic control (OPC) of epilepsy [21]. Paz et al. found that closed-loop OPC of the thalamus may be an effective scheme for interrupting seizures after cortical injury [22]. However, the therapeutic mechanism of DC stimulation is still unclear and should be further explored.
In this paper, by employed a classical cerebral basal ganglia-thalamocortical (BGTC) network [8, 9, 15], we will explore the mechanism to control AE by employing external DC stimulation on the STN. We observed that the seizure may be well inhibited by adjusting the voltage strength properly, and the control mechanism may be inferred from the model together with the numerical results. This model and method may provide a unified framework to investigate similar problems in the future.
2.Model and method
2.1Mathematical theory model
Figure 1 shows the model used in this paper [8, 9, 15]. It consists of nine nerve nuclei; for the sake of brevity, we denote them as follows:
Figure 1.
The basal ganglia-thalamocortical network (BGTC).
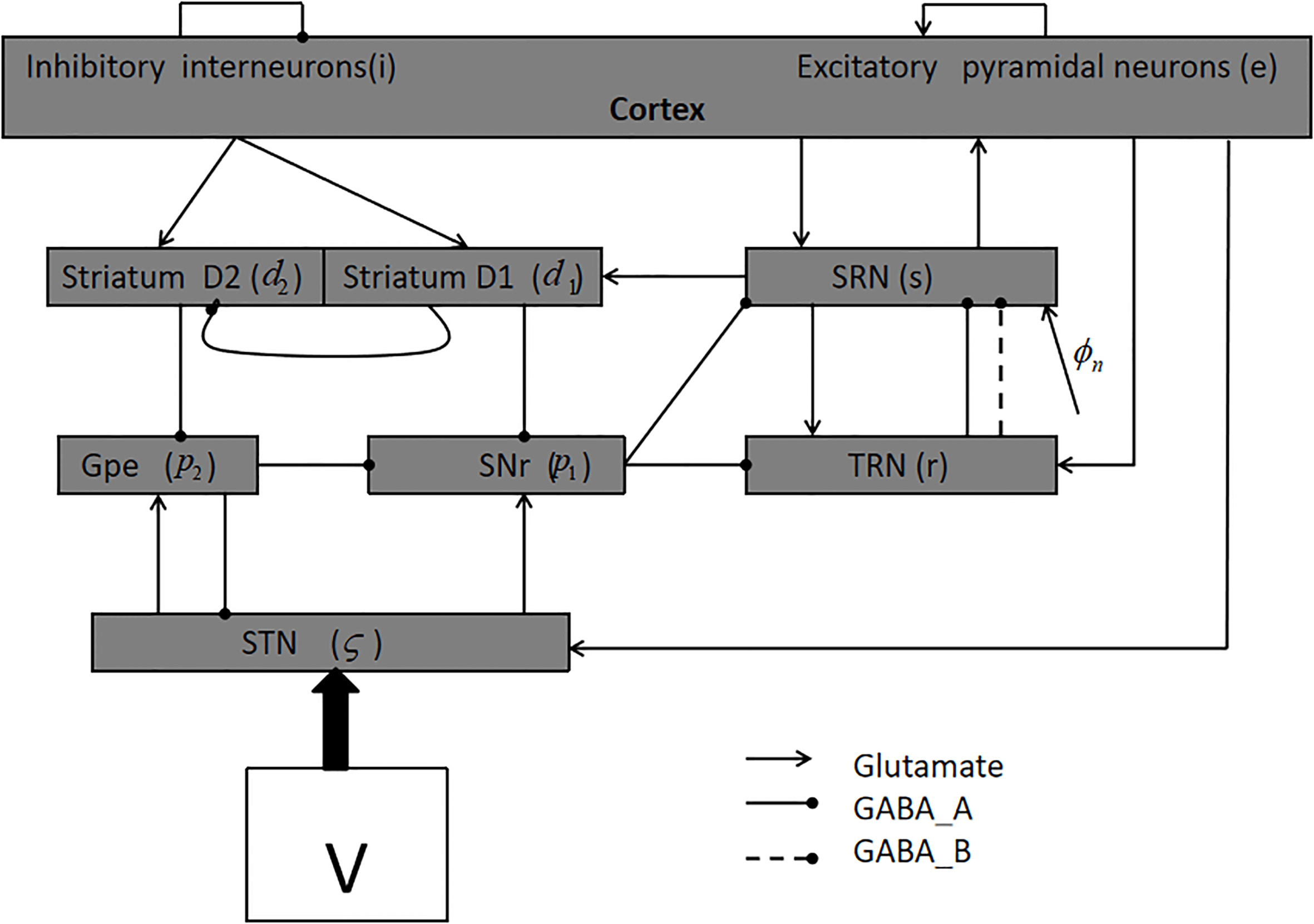
We use the following first-order equations in numerical calculations [8, 9, 15, 23]:
For each nerve nucleus “a”,
2.2Numerical calculation method and data
The four-order Runge-Kutta method is employed to solve the above equations with a step size of
3.Main results
Figure 2a describes the transition process between different states caused by changing
Figure 2.
(a): Brain state transition diagram caused by changing
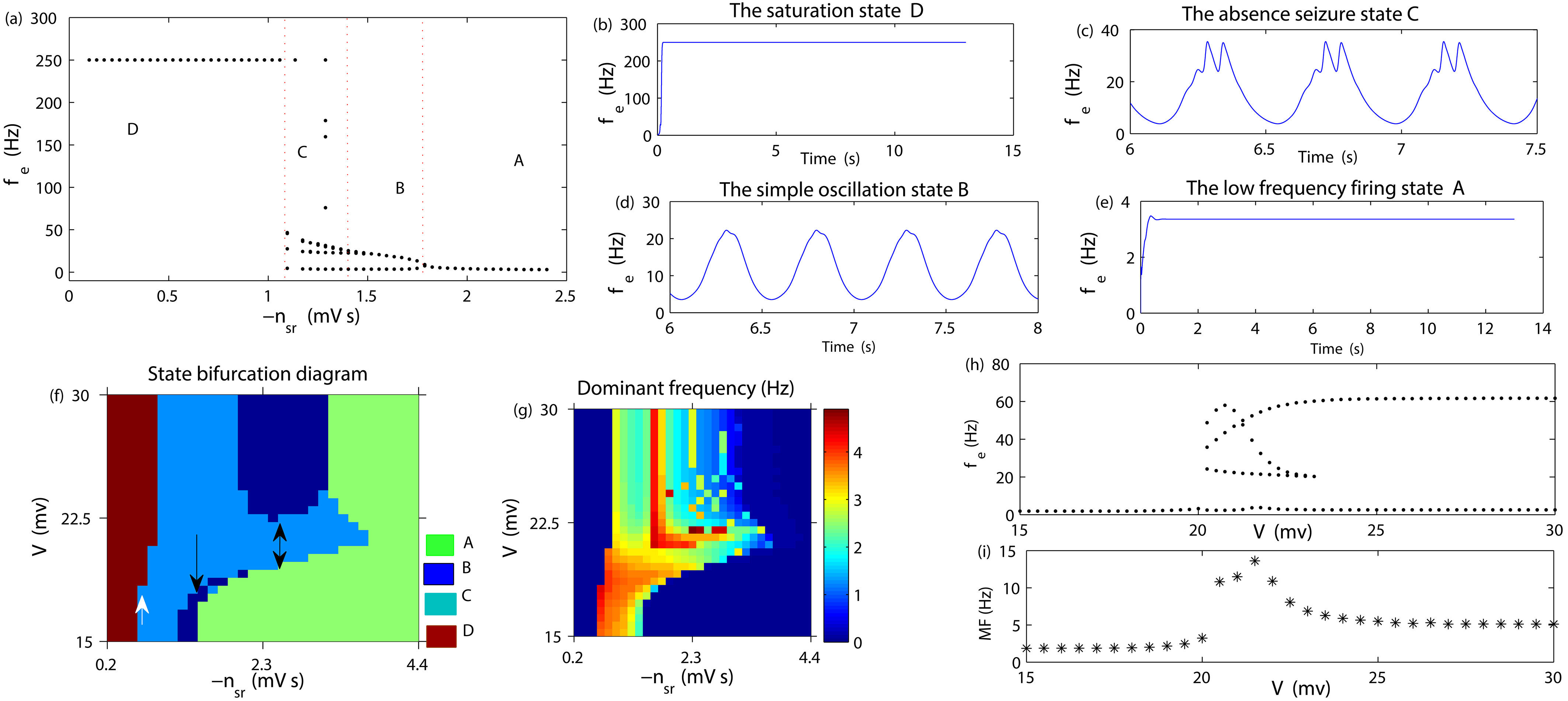
Now, we apply an external stimulation voltage V on the target STN to consider the mechanism for controlling AE by changing the stimulus intensity. Figure 2f represents the state bifurcation diagram for this situation. From Fig. 2f, we can see that when
4.Conclusion
The whole society should pay great attention to the care and treatment of epileptic patients. Externally invasive treatment is an important scheme in controlling intractable epilepsy, such as DBS. However, the relative mechanism is unknown, and theoretical results on the control effect of uniform direct current stimulation are still lacking. In this paper, by using a cortical thalamic-BG network, we investigated the control mechanism of AE by varying the intensity of the stimulus voltage. From the numerical simulation result, it can be seen that the seizure was relieved via reasonably adjusting the intensity of the stimulus, which may be realized through the mutual competitions between the projections in “
The mean field equation is the simplified mathematical model to reflect the biological phenomena, the advantages of which are tractable. However, it cannot describe a more complex brain physiological environment, such as ion channel concentration, receptor activation level, and network connection structure. Moreover, the absence seizure activities are observed to be synchronous in the brain. Therefore, in the partial differential equations used in this paper, we have omitted the spatial derivative for simplification. In this paper, we chose the STN as the stimulation target area. Future studies may be interested in the choice of the optimal target and the optimal stimulation scheme. All these should resort to the spike network. As the results can be applied to specific patients, future research can also resort to data-driven approaches, such as high-throughput omics data and network biomarkers, which can also be applied to similar fields.
Acknowledgments
This research was supported by the National Science Foundation of China (no. 11602092), the Natural Science Foundation of Hubei Province (no. 2018CFB628), and the China Postdoctoral Science Foundation (no. 2018M632184).
Conflict of interest
None to report.
References
[1] | Loiseau P, Duché B, Pédespan JM. Absence epilepsies. Epilepsia. (1995) ; 36: (12): 1182-1186. |
[2] | Crunelli V, Leresche N. Childhood absence epilepsy: Genes, channels, neurons and networks. Nat Rev Neurosci. (2002) ; 3: (5): 371. |
[3] | Rodrigues S, Barton D, Szalai R, et al. Transitions to spike-wave oscillations and epileptic dynamics in a human cortico-thalamic mean-field model. J Comput Neurosci. (2009) ; 27: (3): 507-526. |
[4] | Case M, Soltesz I. Computational modeling of epilepsy. Epilepsia. (2011) ; 52: (s8): 12-15. |
[5] | Dadok VM, Szeri AJ, Kirsch H, et al. Interpretation of seizure evolution pathways via a mean-field cortical model. BMC Neurosci. (2012) ; 13: (1): 95. |
[6] | Marten F, Rodrigues S, Benjamin O, et al. Onset of polyspike complexes in a mean-field model of human electroencephalography and its application to absence epilepsy. Philos Trans R Soc, A. (2009) ; 367: (1891): 1145-1161. |
[7] | Marten F, Rodrigues S, Suffczynski P, et al. Derivation and analysis of an ordinary differential equation mean-field model for studying clinically recorded epilepsy dynamics. Phys Rev E. (2009) ; 79: (2): 021911. |
[8] | Chen MM, Guo DQ, Wang TB, et al. Bidirectional control of absence seizures by the basal ganglia: A computational evidence. PLoS Comput Biol. (2014) ; 10: (3): e1003495. |
[9] | Hu B, Guo D, Wang Q. Control of absence seizures induced by the pathways connected to SRN in corticothalamic system. Cogn Neurodynamics. (2015) ; 9: (3): 279-289. |
[10] | Child ND, Stead M, Wirrell EC, et al. Chronic subthreshold subdural cortical stimulation for the treatment of focal epilepsy originating from eloquent cortex. Epilepsia. (2014) ; 55: (3): e18-e21. |
[11] | Vesper J, Steinhoff B, Rona S, et al. Chronic high-frequency deep brain stimulationof the STN/SNr for progressive myoclonic epilepsy. Epilepsia. (2007) ; 48: (10): 1984-1989. |
[12] | Jou SB, Kao IF, Yi PL, et al. Electrical stimulation of left anterior thalamic nucleus with high-frequency and low-intensity currents reduces the rate of pilocarpine-induced epilepsy in rats. Seizure. (2013) ; 22: (3): 221-229. |
[13] | Feng L, Liu TT, Ye DW, et al. Stimulation of the dorsal portion of subthalamic nucleus may be a viable therapeutic approach in pharmacoresistant epilepsy: A virally mediated transsynaptic tracing study in transgenic mouse model. Epilepsy Behav. (2014) ; 31: : 114-116. |
[14] | Pasnicu A, Denoyer Y, Haegelen C, et al. Modulation of paroxysmal activity in focal cortical dysplasia by centromedian thalamic nucleus stimulation. Epilepsy Res. (2013) ; (3): 264-268. |
[15] | Chen M, Guo D, Li M, et al. Critical roles of the direct GABAergic pallido-cortical pathway in controlling absence seizures. PLoS Comput Biol. (2015) ; 11: (10): e1004539. |
[16] | Sorokin JM, Davidson TJ, Frechette E, et al. Bidirectional control of generalized epilepsy networks via rapid real-time switching of firing mode. Neuron. (2017) ; 93: (1): 194-210. |
[17] | Hu B, Chen S, Chi H, et al. Controlling absence seizures by tuning activation level of the thalamus and striatum. Chaos Soliton Fract. (2017) ; 95: : 65-76. |
[18] | Ghai RS, Bikson M, Durand DM. Effects of applied electric fields on low-calcium epileptiform activity in the CA1 region of rat hippocampal slices. J Neurophysiol. (2000) ; 84: (1): 274-280. |
[19] | Radman T, Ramos RL, Brumberg JC, et al. Role of cortical cell type and morphology in subthreshold and suprathreshold uniform electric field stimulation in vitro. Brain Stimul. (2009) ; 2: (4): 215-228. |
[20] | Liebetanz D, Klinker F, Hering D, et al. Anticonvulsant effects of transcranial direct-current stimulation (tDCS) in the rat cortical ramp model of focal epilepsy. Epilepsia. (2006) ; 47: (7): 1216-1224. |
[21] | Bui AD, Alexander A, Soltesz I. Seizing control: From current treatments to optogenetic interventions in epilepsy. The Neuroscientist. (2017) ; 23: (1): 68-81. |
[22] | Paz JT, Davidson TJ, Frechette ES, et al. Closed-loop optogenetic control of thalamus as a tool for interrupting seizures after cortical injury. Nat Neurosci. (2013) ; 16: (1): 64-70. |
[23] | van Albada SJ, Gray RT, Drysdale PM, et al. Mean-field modeling of the basal ganglia-thalamocortical system. II: Dynamics of parkinsonian oscillations. J Theor Biol. (2009) ; 257: (4): 664-688. |