The future role of official statistics in the business data arena
Abstract
This paper aims at identifying the most promising avenues to maintain and possibly increase the relevance of Official Statistics in the competitive and globally interconnected business data arena. A successful approach should be based upon selective strategies with a strong cooperative attitude towards other institutional and private actors engaged in the production of business data. A Global Value Chain (GVC) approach, developed in the international business literature, is adopted here to identify possible strengths and quickness along all stages of the statistical production process set up and maintained by National Statistical Institutions (NSIs) in order to exploit the “value added” of data produced by Official statistics as compared to other data sources.
The adoption of a register based approach to increase both the efficiency and flexibility of statistical production processes, and more active data dissemination strategies finalised to reduce the gap between statistical data and real business information needs are identified as the two most promising avenues. More specifically, NSIs should keep a pivotal position within the business data eco-system not only as direct producers but also as providers of new data infrastructures such as classification schemes, consistency benchmarking services and data integration opportunities. Some concrete examples of high value added new business data developed in Italy are provided.
1.Introduction
Over the last years, a dramatic increase in the demand for statistical information has been recorded by institutions responsible for the production of official statistics, mainly national statistical systems and international organizations [1, 2, 3].
Yet, the usually predominant role of National Statistical Institutions (NSIs) has been challenged by the emergence of new data producers. These latter, mainly large multinational enterprises from the private sector, have acquired a considerable importance in the data ecosystem thus increasing the degree of competition in many statistical domains, and especially in the business sector domain where other producers of economic data, such as national or regional public bodies and private data providers have always provided statistical information [4].
In the production of business statistics, NSIs have recently used administrative records as input data in their statistical systems with successful results [5]. New sources of data in the Big Data domain provide a range of challenges and risks to the Substitute with NSIs but at the same time offer an opportunity to deliver a more efficient and effective statistical services [6, 7, 8].
In the business sector domain, competition between official statistics and alternative data sources has been greatly amplified by the contextual emergence of some relevant factors that have in fact already redesigned the characteristics and dynamical evolution of the business data arena. Without denying the societal role of official statistics in providing quality information as a public good, the need to redefine the strategic positioning of NSIs with respect to the business data arena clearly emerges, as already stated in many national and international conferences under the label of “staying relevant”, “expanding knowledge with official statistics” or “making official statistics more effective” (see for example [9, 10, 11, 12]).
This paper aims at contributing to the ongoing national and international debate on the future of business statistics produced by NSIs by introducing a consistent approach that embraces both the analysis of official statistics strategic positioning in the business data arena and some strategic and operations guidelines to reinforce it in the next few decades. Some relevant examples based on the Italian experience are also provided.
The paper is organized as follows. Section 2 describes which are the drivers of change in the business data arena. Section 3 introduces the Global Value Chains (GVC) approach as the most relevant analytical framework to define the strategic positioning of NSIs in the business data arena. Section 4 highlights the peculiar role of NSIs in reinforcing the value added of business data. Section 5 illustrates which are the most relevant actions for NSIs to reinforce their strategic positioning in the business data arena. Section 6 provides some examples based on the Italian experience of both production of new business statistics and intense cooperation and interaction between ISTAT (the Italian National Institute for Statistics) and the most relevant data providers and institutional stakeholders engaged in business data production and use. Section 7 draws some conclusions.
2.Factors that are changing the business data arena
By and large, the business data arena can be defined as the virtual place where business relevant information, hereafter business data, is offered by data producers to data users. Data producers do not necessarily sell data at a given price, such as in the case of NSIs and more recently of other business data producers. Business data produced by NSIs hold a high value in this market not only for their superior data quality as compared to other data sources, but foremost for their aptitude to concretely support a wide range of data users at different stages of their decision making process by reducing uncertainty and providing new valuable information. In this respect, private data and official statistics are alternative and competitive data sources in the business data arena.
Understanding the current and perspective evolution of the business data arena then become a crucial feature not only for profit-making private data providers, but also for NSIs since increasing budget constraints in the public sector call for a more careful evaluation of public spending beside the production of core official statistics as a public good.
Four factors can be identified as the predominant drivers of current changes in the business data arena: evolution in the perimeter of the market, technological innovation, changes in the structure and organization of the market supply, evolving nature and scope of users’ needs with respect to business data. Although these four drivers clearly interact, for simplicity sake they will be illustrated separately.
If we consider the perimeter of the business data arena to encompass all relevant business information that can support not only enterprises, but also institutions and citizens, in their decision making processes, it is clear that its geographical scope has been widely expanded over the last few decades. Indeed, enterprises are increasingly globalized in terms of both final markets and production processes. Therefore, they need to understand the evolution of their inputs, intermediates and final markets at a global scale to successfully carry out their own businesses. Given the increasing global interconnection of economic and social issues, public institutions and citizens need also to take their decisions based upon a set of information with a broader geographical scope with respect to the national one.
As far as the technological innovation issue is concerned, its pervasive impact goes far behind the direct effect of massively increased data storage and data computing capability. New technologies have also a pervasive impact in terms of data accessibility and integration. Although the full exploitation of data from different types of data sources is prevented by a substantial lack of standardization, the potential advantages of data integration are self-evident, such as in the case of data lakes.
The structure and organization of business data suppliers have sharply changed in the last few decades with the entry into the market of new private data providers that benefit from the increasing accessibility and integration of administrative and Big data. The most successful companies are multinational enterprises that operate at the global level by providing firm level commercial databases with a regional or global coverage. The recent acquisition of Bureau van Dijk, one of the leading global data providers of business data on firm level balance sheets and financial indicators, by Moody’s, the well-known financial rating and business analytic corporation, bear witness to the increasing opportunities in the market from the integration of different types of business data services.
The evolving nature and scope of user’s needs of business data play a crucial role, together with the other above-mentioned factors, in explaining the increasing complexity of the business data arena. The increasing global interdependency of final and intermediate markets, together with an apparently persistent high degree of uncertainty on future political and economic perspectives, have lead companies and public institutions to increasingly rely on quantitative information rather than on qualitative and subjective perception and evaluation routines. Furthermore, companies more actively engaged in the exploitation of business data have found in data analytics a source of specific competitive advantage in terms of faster and more accurate knowledge of new business opportunities. Although large enterprises are more involved in this process of quantitative evaluation of economic trends and scenarios, competitive pressures on businesses are making small and medium enterprises aware of the importance of adopting this data-focused approach [3].
The direct and interaction effects of all these different drivers have generated a huge pressure on NSIs as well as on international organizations to evolve from their traditional approach to collect, process and disseminate business data as official statistics. New investments made by NSIs should be finalized to increase the efficiency of statistical production processes, the capability to quickly adjust to new user needs and to exploit new data sources. In addition, NSIs and international organizations shell define strategic partnership with other national and local government bodies as well as private data suppliers with superior data capturing and computing capabilities.
3.Strategic positioning of nsis in the business data arena: A GVC approach
This section aims at evaluating the impact of changes in the business area on NSIs in the light of the analytical framework recently introduced in the business and economic literature by Gereffi and others [13, 14] and labelled as Global Value Chains (GVC). Value chain research focuses explicitly on how to manage a production process in the presence of global opportunities but also international threats in order to maximize a company output in general, and its profitability in particular. Broadly speaking, retaining all stages of the production process inside the domestic perimeter of an enterprise is not considered by this approach as an optimal solution, since it is economically more convenient and technically more efficient to fragment the production process among different economic actors (suppliers or affiliates) resident in different locations since they hold specific comparative (costs) or competitive (productivity) advantages. Indeed, the successful enterprise shell focus only upon those stages of the production process where it holds specific competitive advantages in terms of technology or managerial know-how. Therefore, only high value added activities shell be carried out by a company in order to increase its competitiveness.
The GVC approach also highlights the nature of the relationships among the various actors involved in the chain while stressing the concept of “governance”. The value chain defines the full range of activities that firms and workers carry out to develop a product, thus focusing on the sequences of value added within an industry, from conception to production and end use [15]. The comprehensive nature of this framework allows policy makers to answer questions regarding various development issues by formulating new programs and policies. While early use of GVC methodology focused mainly on economic and competitiveness issues, new dimensions and topics have been incorporated such as social and environmental dimensions, labour regulation issues and workforce development (for a recent review on GVC literature see [16]).
A statistical production process can be considered, at least conceptually, as an industrial process based upon as a sequence of production phases: data collection, data validation, production of final estimates and data dissemination. In particular, the value added of a statistical production process can be associated to the business relevance and data quality of business data disseminated as official statistics minus the business relevance and costs of data sources used as inputs in the statistical production process.
The rationale for choosing the GVC approach in this paper is twofold. Firstly, the GCV approach allows us to link each stage of the statistical production process to the value added it has effectively generated. Secondly, it is possible to identify for each stage of the statistical production process the specific sources of competitive advantage held by NSIs as compared to other organizations engaged in the production and dissemination of business data. As a result of this analysis based upon the GVC analytical framework, specific actions can be identified to reinforce the strategic positioning of official statistics in the business data arena.
Figure 1 introduces this discussion by analyzing the medium-long term impacts on NSIs of changes in the business data arena along the statistical production process value chain. Indeed, the process of creation of value added in the data starts from raw data, which by definition are not directly usable by data users, and consider different stages of data transformation until the data are disseminated as high quality official figures.
Figure 1.
The impact on NSIs of changes in the business data arena. Source: Authors’ elaborations.
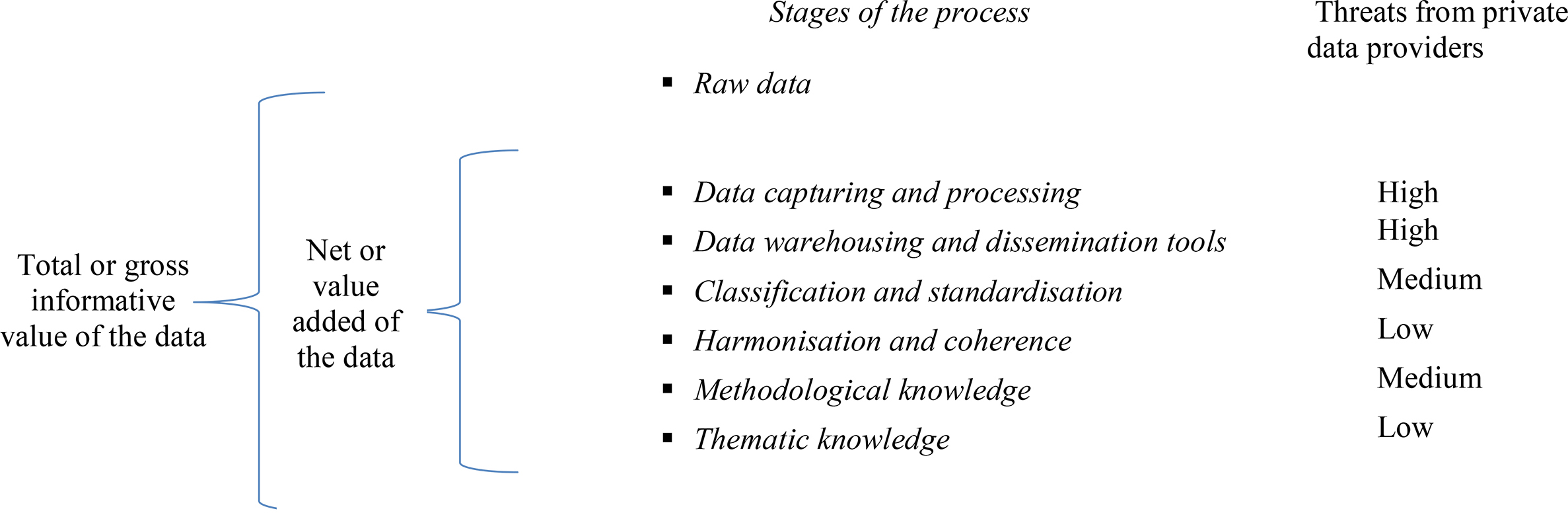
Data capturing and computational processing, as well as data warehousing and dissemination tools, present for NSIs a high threat of fast erosion of value added by private data providers, since these latter usually hold a superior technological capability and invest a considerable amount of economic resources in marketing and client customization tools.
Classification and standardization skills, and meth-odological knowledge, present a medium intensity threat for NSIs since they can be only partially duplicated by private data providers, since public or private organizations other than NSIs usually realize limited investments in ad hoc methodological solutions and have limited capability to define and maintain standard classification schemes according to high level standards.
The capability to plan and maintain a high degree of harmonization and coherence in the production of business data represent a peculiar characteristic of NSIs. Indeed, it represents the NSI’s genuine competitive advantage over other types of business data producers in terms of value added creation from input data. Other organizations engaged in the production and dissemination of business data usually neither plan a priori nor have developed standardized routines or manual checks to maintain a high level of harmonization and coherence in the production of business data. Therefore, these factors represent now a limited threat for NSIs. Nevertheless, as soon as private data providers of business data will reach a mature phase in their business, some investments in this area are expected to maintain or increase their profit margin, and indeed have already been realized by some companies, especially with regard the quality and coherence of business data on ownership linkages [17].
Thematic knowledge represents the set of competencies and skills on the measurement and analysis of a specific phenomenon that NSIs gain trough the setting up and maintenance of a business survey. This knowledge contributes to improving the quality of data in terms of coherence, accuracy but also relevance. The source of this knowledge can be either internal, based on the academic and professional experience of officers, technicians and researchers in charge of a specific survey, or external, generated by interactions with respondent units and data users. Although this type of knowledge, being based on tacit knowledge, is usually not fully perceived by NSIs as a key component of their immaterial assets, it is nevertheless essential to maintain and possibly increase a competitive advantage over other organizations engaged in the production of business data [18].
Some key suggestions to maintain and potentially increase the strategic positioning of NSIs as compared to other producers of business data seem to emerge from this preliminary analysis:
• The strengthening of the competition in business data arena cannot be managed by NSIs by adopting a monopolistic position that aims at dominating the market by offering all relevant business data. Given the increasing volume and complexity of business data available in the market this approach is unrealistic and unfeasible.
• Value added generated by NSIs in specific stages of the statistical production process is very likely to be eroded by commercial data providers and other organizations given the impact of new technologies and the increasing accessibility of administrative, open and web-based data.
• The global scope of the business data arena will make national figures less and less effective in understating the complexity of nowadays business situation.
• A well-focused strategy based on the reinforcement of specific stages of the production process where value added is more likely to be retained or potentially expanded seems to be the only successful and sustainable approach that can be taken by NSIs to reinforce their strategic positioning in the business data arena.
• The definition of networking and partnership with other organizations that hold specific technological, data capturing and dissemination assets can provide NSIs with additional resources to reinforce their strategic positioning in the business data arena, under the condition that free and immediate accessibility to official statistics will be guarantee to all data users [7].
4.Future evolution pattners for official business statistics
So far we have considered the strategic repositioning of official statistics in the business data arena essentially from an economic information market perspective. This approach is both incomplete and not fully consistent with the genuine nature of NSIs as public institutions devoted to the production of high quality official statistics as a public good. The motivation is twofold.
Firstly, NSIs cannot operate under fully competitive conditions, since they have some relevant financial and institutional constraints. All sources of external financial support that NSIs may receive for the development of new business data have a limited impact on their overall budget, where this latter is also defined in many countries independently from the capability of the NSIs to produce new business data beside official statistics national or international obligations. Secondly, the institutional goal of any NSI is to produce and disseminate high quality statistics as public goods that increase the knowledge of the national business and social communities rather than producing any possible typologies of business data.
Building upon these and previous considerations, the strategic repositioning of NSIs in the business data arena can be reformulated as follow: NSIs shell increase their strategic positioning by maximizing the value added of all business data available at both national and international level in terms of higher quality and more useful information for enterprises, citizens and policy makers.
This approach, that can be labelled as improvement of the systemic valued added of business data, seems to well balance the peculiar nature of the NSIs with their legitimate goal to be more relevant in the business data arena. As illustrated in Fig. 2, in a market based individual value-added environment, each NSI acts to maximize its individual contribution to the total value added of available business data by leveraging both market expansion and competition forces.
Figure 2.
NSIs repositioning in the business data arena: from individual to systemic value added. Source: Authors’ elaborations.
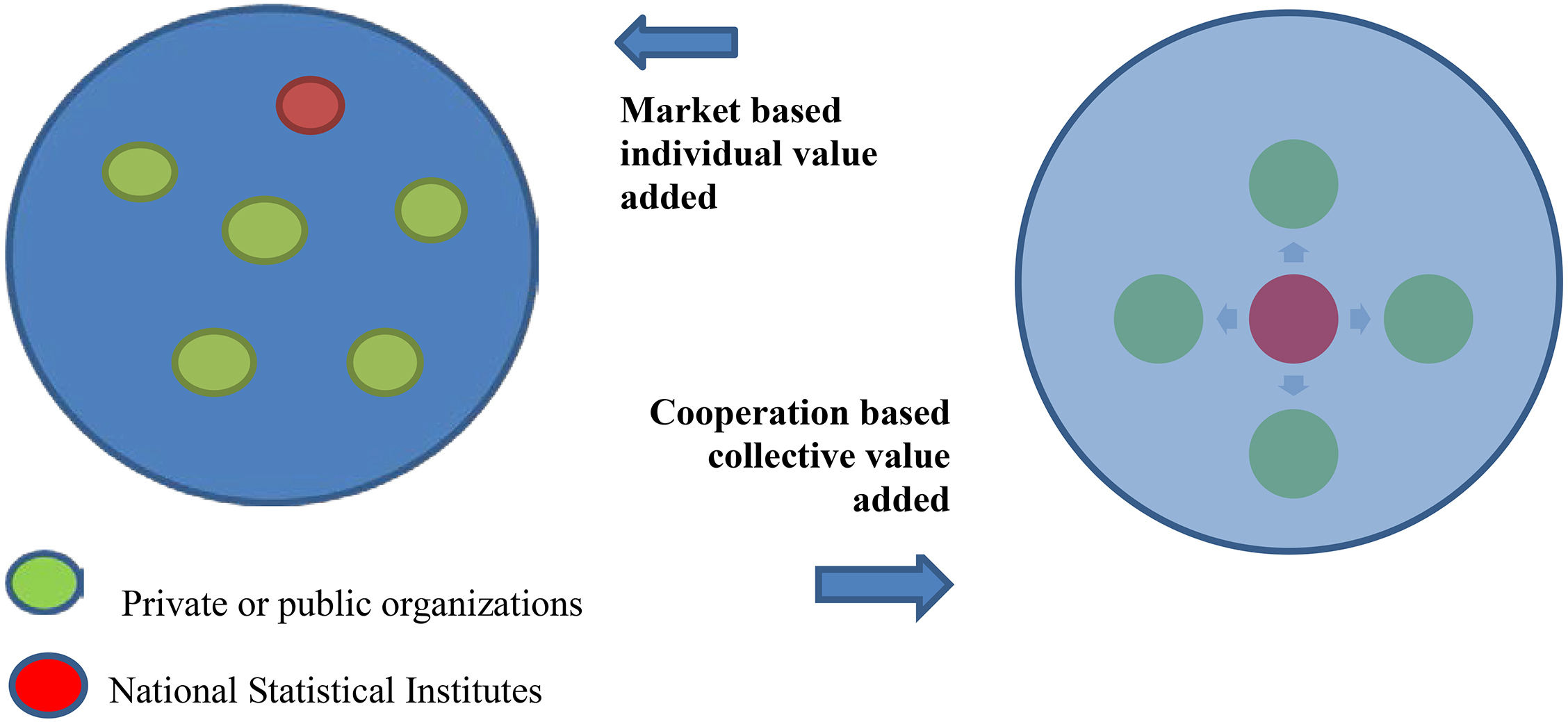
Following this individual approach, a NSI by expanding its output can temporally increase its market share as compared to other private or public organizations in the business data arena, but the overall value added of business data present in the arena does not necessarily improve. In contrast, in a cooperation based environment a NSI aims at reinforcing the value-added of all business data available either by expanding the production with new data integration strategies or by reinforcing the coherence and relevance of all already existing business data.
The implications of this approach are quite straightforward. In order to reinforce the collective value added of business data, NSIs shell evolve from a closed to an open and fully integrated system of business data. The transition from one to the other setting requires some substantial changes in the way NSIs interact with other private and public organizations engaged in the production of business data:
a)
a) NSIs should be more actively engaged in data comparability actions between official and not official sources with the scope of decreasing the uncertainty in the use of those different sources by business data users.
b) NSIs should also promote the transfer of scientific knowledge and competencies available in their organizations to improve the quality of all business data.
c) NSIs should increase the accessibility of their own data, with specific regard to the business register, by founding the most convenient institutional and technical solutions in full compliance with the national legislation.
d) NSIs shell also define joint projects with specific organizations active in the market of business data to boost the development of new data by sourcing new technologies, new business models and new data sources. This must be done in full compliance with its neutral and institutional position with respect to all data users and data producers active in the business data arena both in terms of data accessibility and market profit opportunities.
e) NSIs shell promote the development of new official statistics in specific areas of foremost interest for the business and social community at the national level or international level where market opportunities are insufficient for private organizations to produce new data or there is a strong need to develop official data in order to benchmark the data quality and reliability of not official data sources.
f) NSIs shell reinforce the cooperation with other NSIs and international organizations in the dissemination of official statistics by promoting the publication of data at the national level that incorporate international comparison with other countries, following the examples of Eurostat and OECD that systematically publish any statistical figures in the framework of an international comparison data dissemination approach. The scope of this action is not only to reinforce the relevance of official business statistics in the business data arena, but also to establish business statistics as the benchmark for checking the quality all any other business data sources independently from the geographical perimeter of those data. Indeed, most commercial data providers already made available in the market firm level business database with a European of global coverage [19].
The actions listed above will require a huge effort by NSIs to adjust their organization, technical and legal procedures and as well as human resources to successfully face these challenges. The process will take time, especially with regard to the accessibility of statistical data at the individual level for data quality assessment of external data sources, given the well-known legal constraints at the national level. However, the adoption of intermediate technical solutions to enrich the value added of not-official data or to compare official with non-official data sources, as mentioned in section six about the Italian case, will testify a change of direction in the way NSIs interact with other private or public organizations. This more cooperative approach will reinforce, in the medium-long terms, both the reputation and the business relevance of NSIs in the business data arena.
Table 1
A set of possible actions that can improve the positioning of NSIs in the business data arena
Stage of the production process | Suggested actions | Expected results |
---|---|---|
1. Production | Adoption of a new business model based on a GSBPM approach | Efficiency gains, reduction of costs, increased timeliness |
2. Data capturing | Definition of active partnership with different types of data providers | Efficiency gains, reduction of costs, increased timeliness |
3. Production | Shift from direct reporting to administrative data by leveraging the central role of business register | Statistical burden reduction, substantial gains in terms of accuracy, data granularity and flexibility of output |
4. Production | Integration of different outputs based on the business register as the pivotal asset | Substantial extension of output and possibility to explore complex phenomena |
5. Dissemination | Definition of partnership with output stakeholders | Increased relevance of the data, development of new indicators |
6. Dissemination | Definition of more complex and integrated outputs and more customized dissemination policies | More relevant data |
The adoption by NSIs of a more collaborative approach, by shifting from the production of data to the development of new data infrastructures open to the integration with other business data producers given confidentiality constraints, can be considered as a natural extension or evolution of their institutional mandate (the production of public goods). In contrast, the presence of the right incentives that can guarantee an active cooperation from private business data producers can be questioned, since these profit-seeking organizations tend to reinforce their competitive advantage by leveraging the uniqueness of their outputs. The effort currently made by NSIs, independently or jointly with international organizations, to access big data, scanner data or other business data information from large retail chains, web companies or digital platforms is an emblematic example in this respect. From one side, these private companies are, at least in principle, interested in cooperating with NSIs since this cooperation can provide a sound benchmark of the quality of their information with respect to official statistics and the accessibility to some useful but not confidential information from NSIs. From the other side, the risk of reducing the exclusivity of their data is considered relevant but not high, since NSIs hold a high reputation in confidentiality protection and are trustable in terms of limitation to data dissemination and sharing of data with other public institutions. This outcome “low incentive/medium risk” of the cost and benefit analysis carried out of private data providers may generate a limited cooperation aptitude. Nevertheless, the following factors, already in place in changing the current scenario of the business data market, can positively impact cost and benefit analysis by turning a moderate cooperation aptitude into a more proactive engagement by private data providers:
• Increasing relevance for data users of integrated data, data lakes and open data as essential components of new data ecosystems, thus reducing the relevance of unique but also isolated private or public databases.
• Possible changes in the national or international legal framework that include obligation for web companies and digital platforms to provide at the public sector in general and NSI in particular a well defined set of variables and data records.
• Fine-tuning of the legal framework and upgrading of IT data security technologies to secure the data provided by private data providers to NSI.
5.How to reinforce the positioning of nsis in the business data arena
Following the discussion developed in the previous sections, a well-defined set of actions can be identified on how to reinforce the positing of NSIs in the business data area. These actions refer to different stages of the statistical production process, but they can be linked to expected impacts on the overall quality of data produced by NSIs (Table 1).
This section is mainly descriptive of the scope and impact of proposed actions, while the next one also provides some concrete examples for each of them. Actions defined for data capturing essentially reflect a defensive approach finalized to reduce the efficiency and technological gap that usually plague NSIs as compared to private organizations in the production of business data. In contrast, actions proposed for the improvement of the efficiency and flexibility of production and dissemination are explicitly designed to reinforce the strategic positioning of NSIs as compared to other business data providers by expanding the value added embedded in official figures.
NSIs usually organize their production processes according to a stovepipe approach in order to maximize the efficiency and timeliness of a specific survey production line. This approach leads to local benefits, but it is usually not consistent with data integration strategies and the goal to achieve a high systemic efficiency across different production lines.
The adoption by NSIs of a more efficient and flexible production model that decompose and reorganize all statistical production lines based on well-defined business lines and business support functions can ensure large benefits in terms of costs reduction, increased efficiency and better timeliness in the production and dissemination of data. Furthermore, it can lead to additional benefits in terms of data quality based upon the pooling of methodological, data collection and analytical competencies that are dispersed in the stovepipe based production organization. Nevertheless, this approach requires huge investments in the start-up phase, a solid leadership and strong planning and routine management capabilities in order to reduce the increased costs of internal coordination. This approach of standardization and harmonization of statistical production processes has been pioneered by UNECE under the umbrella of the so called Generic Statistical Business Process Model (GSBPM) and adopted by some NSIs, such as ISTAT as described in the second part of this section.
The adoption of a GSBPM approach is intrinsically related to the central role of the business register and related administrative data sources in the new statistical production model of NSIs. The crucial role of the business register set up and maintained by NSIs is amplified moving from a survey based random sampling approach to a census-like intensive use of administrative data. To produce high quality figures, this latter approach requires as an a priori condition the exhaustive control of the list of the business units under investigation, including key classification variables as economic activity, business size and location. Indeed, commercial data providers usually build and maintain large databases of business data at the enterprise level independently from a business register. However, the absence of a high quality business register generates substantial bias in aggregated figures extracted from commercial databases as well as poor quality information on the state of activity and other business characteristics of each business unit. In contrast, the use of administrative data by NSIs rooted in the business register allows to control for potential biases and to keep high quality and fully consistent data at the micro level by increasing at the same time the granularity and flexibility of official statistics [20].
The integration of different outputs based on the business register as the pivotal asset essentially represents for NSIs a business development action fully consistent with the previous action. The integration of all available census like business data with their reference business register can be easily achieved since in most NSIs these data sources are highlight standardized as already built as standalone high-quality databases. The availability of a fully consistent and interoperable system of business registers (enterprises, local units, enterprise groups) allows to further expand this action that is finalized to increase the value added of business data produced by NSIs building upon one of their core assets, both in terms of unique data infrastructure and the presence of specific methodological and thematic knowledge in data integration and standardization also assuring consistent results within and across different business domains.
Given their strong focus on statistical data production according to high quality standards and the public good nature of official statistics, NSIs have usually paid limited attention to data dissemination policies. Nevertheless, data dissemination represents a crucial stage in the process of incrementing the value added of official statistics according to a GVC approach the goes behind the full accessibility to business statistics data and metadata. The increasing availability of business data from different types of official and not official data sources has dramatically augmented the costs for data users to identify, select and understand the information that are relevant for they decision processes. Competencies and skills for the correct and meaningful analysis of business data remain relatively rare for citizens and small size companies. In order to increase the value added of official figures, NSIs shell invest more in making their data more explicitly linked to data-user decision processes by using both technological (automatic customization of data dissemination according to data user characteristics that are publicly available) and analytical solutions (tools and dissemination products oriented to reduce the knowledge gap in understanding and effectively use the data). In particular, two different but highly complementary actions can be identified to develop more effective dissemination policies by NSIs.
The definition of an active partnership with different types of data providers in data capturing is also important to obtain some substantial advantages in terms of efficiency, costs reduction and better timeliness in data dissemination by externalizing some low value-added activities of the production process such as data standardization and preliminary data quality checks. This approach, that essentially reflects an upgrading process along the data processing value chain, requires strong institutional building with respect to other public institutions and negotiating skills with respect to private organizations as data providers. From one side, it requires NSIs the availability to transfer some knowledge and skills in data capturing and processing that will increase the capability of data providers to produce better quality input data, thus making the mutual benefit for data providers more persistent and explicit. From the other side, it can also generate beneficial spillovers for NSIs since better technological or organizational solutions adopted by data providers can lead to additional efficiency and data quality gains for the production of official figures [19]. The definition of active partnerships with output stakeholders is important to leverage the value added of official figures with respect to specific user needs and to develop new indicators that are of foremost interest for policy makers and the business community. Indeed, the interaction with stakeholders and policy makers increase the capability of NSIs to react in a more flexible and business relevant way to user’s needs. The definition of more complex and integrated outputs reflects the opportunity for NSIs to increase the value added of official statistics by decreasing the usual fragmentation of statistical outputs into specific domains that are now clustered around a given phenomenon and presented in a more effective way. This is achieved by providing an analytical framework to improve the understanding of the phenomena, which also highlights all meaningful interactions across variables and statistical domains.
6.Business relevance and accountability of the gvc approach
This section introduces some examples based on the experience of the Italian National Institute for Statistics (ISTAT) in the area of business statistics which are of foremost relevance to illustrate and further clarify the list of strategic and operational actions introduced in the previous sections as well as to highlight how valued added of official statistics can be calculated within the framework of a GVC approach. All these examples contributed to persistently expand the value added and to upgrade the positioning of Business data produced by ISTAT as compared to other organizations producing similar national or territorial business data as well as to reinforce the quality and value added of all available business data.
The new organization recently adopted by ISTAT following a GSBPM approach has introduced some substantial changes in the organization of statistical production for the main statistical domains: business statistics, social and population demography statistics, and territorial, agriculture and transport statistics [9, 21]. In the case of business statistics, all statistical production processes related to economic units, such as business enterprises, public and non-profit institutions, were clustered in a single directorate which also includes all the relevant basic business registers. Business support functions to the statistical production process were organized in a separate department which includes, among the others, the IT, the methodological and the data collection directorates. This latter directorate took in charge the data collection process of each survey carried out by ISTAT, including both direct reporting and the use of administrative or other data sources.
This new division of labor in the organization of statistical production process has already generated some substantial advantages for Business Statistics in Italy with a positive and persistent impact both on data quality and on the expansion of value added generated by official figures. The allocation of all data collection activities to a specific directorate with the active support of external suppliers (call centers) led to successfully exploit economies of scope and scale. On average, the response rate for all structural business surveys raised from 10% to 25% over the past two years. Skilled technicians and researchers from the business statistics directorate also supported data collection with a strong focus on data quality improvement, since contacts with companies and other respondent units on specific issues related to the correct and accurate completion of survey questionnaire are still under the responsibility of the Business Statistics Directorate. As a consequence of the allocation of all data collection activities to a specific directorate, skilled human resources of the Business Statistics Directorate, previously absorbed by standard data collection activities, were assigned to other tasks, including the development of new business statistics indicators.
The inclusion of the business register within the Business Statistics Directorate allowed to progressively exploit the full potential of data integration. After the realization of an extended business register that includes all the key variables for the compilation of Structural Business Statistics (SBS) with a firm level detail (Frame SBS),11 an extended register that includes all the key variables for the compilation of SBS with a local unit detail (Territorial Frame SBS)22 was implemented. Both frames share a similar approach in terms of data integration and estimation of variables by using a mixed model approach combining direct reporting with an intensive use of administrative data. While Frame SBS was designed and maintained to persistently increase the quality of SBS estimations at the national level and to provide a more detailed and flexible output to both standard users and firm level applied researchers, the Territorial Frame SBS was deliberatively conceived to expand the value added of official statistics in terms of greater territorial granularity as compared to standard statistical domains in one area where commercial data providers have always struggled to obtained high quality results both in terms of accuracy and relevance for data users. This is because the production of high-quality, economic coherent and granular territorial data on business indicators are almost impossible to be generated without a complex process of data integration of multiple data sources including high quality business registers and survey and administrative data.
By and large, the value added of official statistics can be defined as the difference between the market value of the outputs disseminated in different forms by NSIs and the economic value of raw data used as inputs in statistical production processes managed by NSIs. According the GVC approach, inputs data used by NSIs can evolve into intermediate data provided by private and public data providers, therefore an accurate and reliable estimate of the effective value added generated by NSI along a GVC represents a crucial goal to assess the real impact of NSI with respect to both the production of new data or quality improvement of already existing data.
An economic sound and statically accurate calculation of the value added of official statistics represents a quite difficult task from both a conceptual and a methodological point of view. Conceptually, the public good nature of official statistics raises a lot of relevant issues [24]. From a methodological perspective, two main approaches to calculate the value added of a good or service can be identified within the framework of [25], that is the income approach and the production approach.
In the production approach, the valued added is obtained as the difference between production and intermediate consumption:
(1)
where production is the economic value of produced commodities (output) and intermediate consumption is the economic value of commodities used in the production process that are external to NSIs.
In the income approach, value added is obtained as the sum of the monetary value (costs and margin) of inputs that are internal to NSI perimeter. That is:
(2)
where unit markup refers to the difference between the selling price of a good or service and its cost, usually expressed as a percentage above the cost. The total markup is then obtained by multiplying the unit markup by the volume of production (for instance number of databases, tables or data records).
In line with the conceptual debate on public goods, the first approach is mainly used for market-oriented activities while the income approach tends to be predominant for non-market activities [26]. In the income approach, the problem of estimating a correct market value for the output produced and delivered by non-market producers such as NSIs concerns a reliable and accurate estimation of the net operating surplus. While for other non-market producers that offer products and services that present similar characteristics as compared to market-oriented producers (homogeneous outputs), a standard approach is to derive a unit markup from private companies producing similar product, a sound solution is more difficult to be identified and implemented in the case of official statistics, since official data are considered heterogenous with respect to business data produced by private data providers or public bodies other than NSIs. The heterogeneity of business data produced by NSIs as compared to other data producers cannot be limited to differences in data characteristics (difference in volume, granularity, time series length, type and number of variables), but it also has to take into account the superior data quality of official statistics as compared to other business data in terms of accuracy, consistency and business relevance.
As a result, the standard income approach shall be adjusted in the case of the business data produced by NSI to consider the superior advantage of official statistics over other business data. In particular, the measurement of costs for standard internal input variables, such as
The production and dissemination by ISTAT of new business data and indicators from SBS Territorial Frame can provide a quite interesting example on how to calculate the value added generated by these new official statistics output following an income approach adjusted to take into account the peculiar characteristics of official statistics. The basic information that shall be used to produce this estimate are the following:
• Number of human resources of ISTAT directly involved in the project and implementation phases of Territorial Frame SBS: 15 FTE of which 5 with a Ph.d in methodology or geography/economics, 1 Master degree on big data.
• Number of human resources of ISTAT indirectly involved in the production of intermediate data (basic registers, extended registers) as inputs for the production of Territorial Frame SBS: 25 FTE of which 8 with a Ph.d or a Master in related fields.
• Material and immaterial assets involved in the production of Territorial Frame SBS: material costs of maintaining or upgrading IT instruments and procedures; immaterial costs: know-how and knowledge incorporated in the output. Some indicators based on patents or royalties equivalence can be used to assess the costs of immaterial assets.
• Markup based upon unit markup multiplied by the volume of new territorial data as compared to standard territorial data. The volume of new data is quite easy to calculate since traditional territorial data present on average 20 regions by 40 sectors (NACE 2 digits) up to a total of 800 records as standard breakdown, while the new territorial data set includes 8.500 municipalities by 300 sectors (NACE four digits) as the new breakdown. The net increase in volume is therefore of about 2.5 millions of new records. Standard unit markup can be derived from commercial information from private data providers that disseminate similar territorial databases. A premium to be added to the standard total markup should take into account the reputational effect of ISTAT plus the business relevance of Territorial Frame SBS for data users. This latter information shell be calculated by taking into account the download of data from our websites, the request of access to microdata by researchers, quotation on papers and on twitter.
Of course, this list of items illustrated above cannot be considered exhaustive since the public nature of this new territorial data is very likely to generate externalities and an overall impact on media, analysts and policy makers not very easy to quantify. Figures 3 and 4 illustrate some examples of data and indicators that can be directly produced from the Territorial Frame SBS which are of foremost interest for regional and urban planners, local development economists and policy makers.
Figure 3.
Location of value added of industrial robots’ manufacturing in Italy at the municipality level.
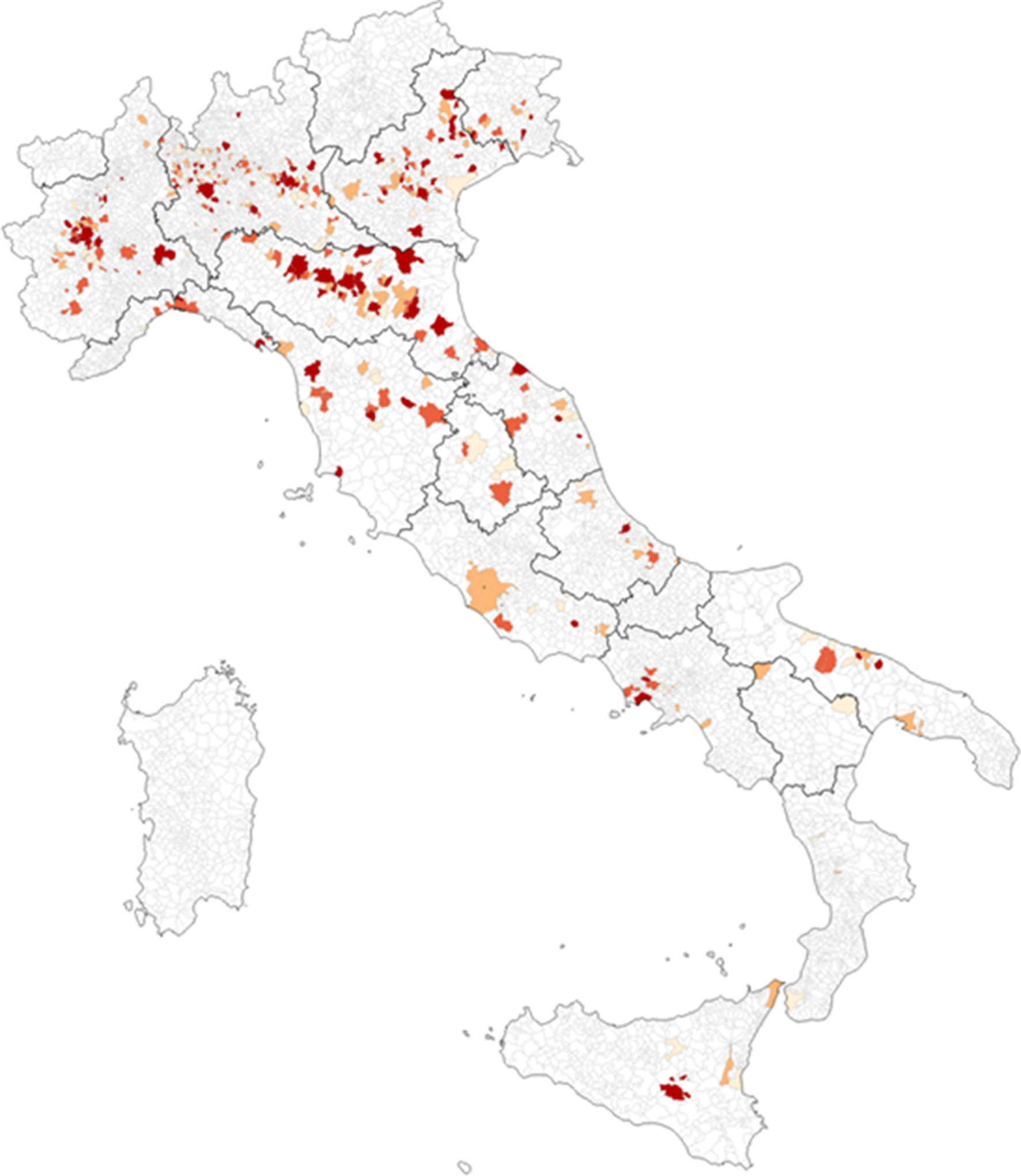
Top municipalities | Share in % of national value added | Share in % of national employment | Share in % of resident industrial plants |
---|---|---|---|
Top 5 municipalities | 30.0 | 24.7 | 2.2 |
Top 10 municipalities | 42.9 | 32.2 | 3.3 |
Top 20 municipalities | 56.7 | 46.1 | 12.2 |
Figure 4.
Export-led value added in manufacturing in the South of Italy at the municipality level.
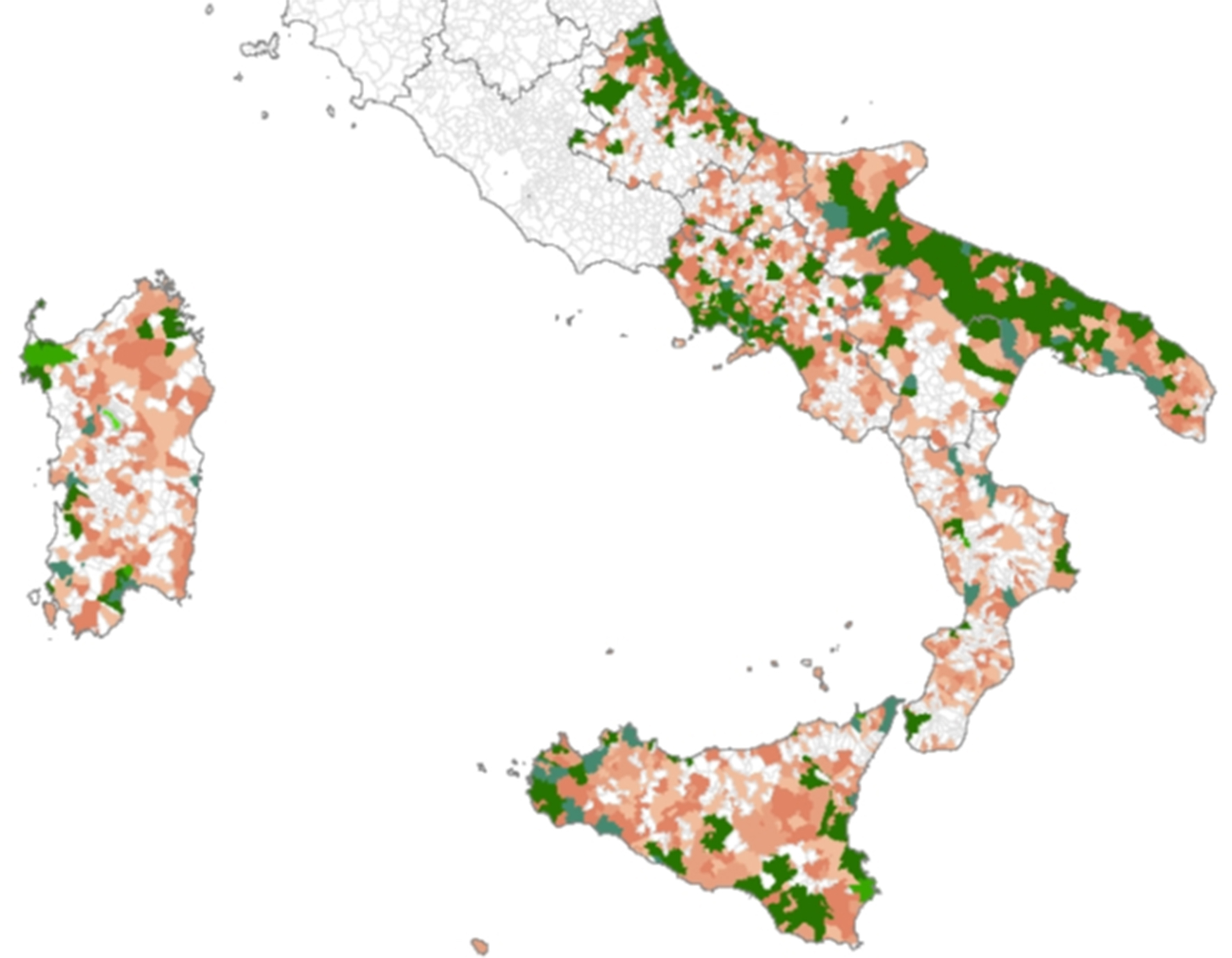
The definition of strategic agreements with some key institutional as well as commercial data providers, including the knowledge transfer on how to assess and improve the quality of raw data, helped ISTAT to reduce the timing of data processing as well as the amount of human resources traditionally devoted to input data quality checks with mutual benefits for data providers that have increased the overall quality and therefore the value added of their data. This is the case of the close cooperation since long time established between ISTAT and the Italian Customs Agency for the production of foreign trade in goods statistics, and more recently of technical and commercial agreements between ISTAT and private data suppliers for the reclassification of administrative data according to economic principles coherent with statistical variables definitions. This task is quite complex to be achieved given the difficulties in codifying internal routines and the time consuming of institutional building activities. It is nevertheless essential to support a persistent and efficient upgrading of NSI activities to more value added intense data production activities.
The definition of strategic partnership between ISTAT and key output stakeholders for the dissemination and economic analysis of business data has always been a crucial asset to promote the relevance of business statistics in Italy. The production for two decades of a joint statistical yearbook on foreign trade statistics and enterprise economic activities between ISTAT and the Italian Agency for the development of foreign trade and attraction of foreign investments (ICE) was originally conceived to increase the relevance as well as to reduce the gap in the dissemination of official statistics on international trade in goods. These data are since then presented every year with a
The definition of strategic agreements with some of the most relevant Italian Institutions that use business statistics for policy making has also generated some interesting spillovers in terms of reinforcing the overall coherence and relevance of business data used and disseminated by these organizations. Under the constraints of the national legal framework that, as in any other countries, protect and prevent the direct use of firm level data from official business statistics, these policy oriented Institutions have provided their own firm level data to ISTAT to enrich them with additional information from the NSI (economic performance indicators such as productivity and profitability, internationalization profiles, etc.), and obtained as the outcome of this process aggregated figures which not only incorporate these information but also made them consistent with the national business register and therefore with all the other official figures published by ISTAT.
The design and implementation by ISTAT over the last few years of more complex and integrated outputs in the area of business and social statistics materialized in a new series of analytical reports published on an annual basis, which include for instance the Report on the competitiveness of Italian firms and industries, the Report on knowledge economy, the Report on Labour Market in Italy [30]. These reports, realized as a joint effort by Statistical experts from data production directorates and analysts and economists from the ISTAT Research Unit, organize and integrate both at the micro and at the macro level all the relevant data sources available and exploit them for analytical and policy making purposes. By and large, these reports support policy makers and experts with relevant insights on the structure and evolution of the Italian economy as compared to main consolidated and emerging competitors. They also provide guidance to less expert data users and analysts on all available data, including new indicators recently produced by ISTAT, for a better understanding of a complex economic and social phenomenon.
In 2017 ISTAT has started the production of a set of experimental statistics based on the use of Internet data, one of the most relevant Big Data sources [31]. These statistics refer to the activities that enterprises carry out in their websites and are a strict subset of those currently produced by the “Survey on ICT in enterprises”. The idea is to calculate these estimates by making use of the websites content, that is collected by using web scraping tools, and processed by applying text mining techniques. Then, models are fitted in the subset of enterprises for which both sources are available: survey reported values, and relevant terms obtained by the web scraping/text mining procedures. Experimental statistics have been obtained by making use of two different estimators: the first one is a full model based estimator; the second one is an estimator that combines model based estimates and survey estimates. Considering the various domains for which they have been calculated, the three sets of estimates (survey, model and combined) in most cases are not distant.
7.Conclusions
The ongoing substantial changes in the business data arena have cast some doubt on the possibility for NSIs to maintain and eventually reinforce their role as relevant organisations with a high institutional reputation in business data production and dissemination.
The global value chain approach introduced in this paper leads to identify the strengths and weaknesses of NSIs as compared to other players in the market of business data information. It also provides some guidelines on how to improve the overall impact of NSIs in the business data arena by focusing on actions and projects that can generate a permanent and substantial increase in the valued added of data produced by NSIs. However, the role of NSIs in the business data arena cannot be limited to the development of better quality or new data as one of the most relevant players in the business data arena. The institutional scope of NSIs with respect to business data is indeed much broader since it involves the production of official data as public goods for national and international institutions, as well as the national business and social communities.
Therefore, NSIs need to balance the expansion of available official statistics in the business sector with a substantial effort to reinforce the quality of all business data available in the market. In particular, NSIs should aim to keep a pivotal position within the business data eco-system not only as direct producers but also as providers of new data infrastructures such as classification schemes, consistency benchmarking services and data integration opportunities.
The successful repositioning of NSI in the business data arena calls for some substantial changes that are both internal and external with respect to the NSI organization. The internal ones require an in-depth rethinking on how data are collected, produced, integrated and disseminated. The stovepipe approach shell be replaced by a fully integrated production system that allows to exploit both a higher level of production efficiency as well as greater output flexibility. External challenges concern the establishment of strategic partnerships with other public institutions as well as private companies to exploit external sources of competitiveness and efficiency in terms of data collection from new data sources, computational capability and data storage and data integration opportunities.
Notes
1 See [22] for more information on the data sources and the methodology adopted to produce Frame SBS.
2 See [23] for more information on the data sources and the methodology adopted to produce the Territorial Frame SBS.
References
[1] | Martín-Guzmán P. The growing demand for statistics: Challenges and opportunities, in statistics, knowledge and policy. OECD Publishing. (2005) ; 513-521. |
[2] | Jeskanen-Sundström H. ICT statistics at the new millennium – developing official statistics – measuring the diffusion of ICT and its impact. International Statistical Review. (2003) ; 71: (1): 5-15. |
[3] | Coleman SY. Data-mining opportunities for small and medium enterprises with official statistics in the UK. Journal of Official Statistics. (2016) ; 32: (4): 849-865. |
[4] | OECD. Development Co-operation Report 2017: Data for Development, OECD Publishing, Paris. (2017) ; doi: 10.1787/dcr-2017-en. |
[5] | Shlomo N, Goldstein H. Big data in social research. Journal of the Royal Statistical Society: Series A (Statistics in Society). (2015) ; 178: (4): 787-790. |
[6] | Kitchin R. The opportunities, challenges and risks of big data for official statistics. Statistical Journal of the IAOS. (2015) ; 31: (3): 471-481. |
[7] | Tam SM, Clarke F. Big data, official statistics and some initiatives by the australian bureau of statistics. International Statistical Review. (2015) ; 83: (3): 436-448. |
[8] | Braaksma B, Zeelenberg K. “Re-make/Re-model”: Should big data change the modelling paradigm in official statistics? Statistical Journal of the IAOS. (2015) ; 31: (2): 193-202. https://www.unece.org/fileadmin/DAM/stats/documents/ece/ces/ge.58/2017/mtg2/Theme_1_Italy_ver2.pdf. |
[9] | Alleva G. “Adding Value to Statistics in the Data Revolution Age” ISI Conference, Rio De Janeiro. (2015) ; https://www.istat.it/it/files/2015/10/GAlleva_IPS_ISI_WSC_2015_AddingValueStat_PAPER.pdf. |
[10] | IEAG (UN Independent Expert Advisory Group). A World that Counts: Mobilizing the Data Revolution for Sustainable Development. Independent Expert Advisory Group Secretariat. (2015) . Available from: www.undatarevolution.org/wp-content/uploads/2014/11/A-World-That-Counts.pdf. |
[11] | Robin N, Klein T., Jutting J. Public-Private Partnerships for Statistics. Lessons Learned, Future Steps: A Focus on the Use of Non-official Data Sources for National Statistics and Public Policy, OECD Development Co-operation Working Papers, No. 27, OECD Publishing, Paris. (2016) . doi: 10.1787/5jm3nqp1g8wf-en. |
[12] | Eurostat. European Business Statistics Manual. (2018) ; ISSN 2443-8219. |
[13] | Gereffi G. The Organization of Buyer-Driven Global Commodity Chains: How US Retailers Shape Overseas Production Networks. In G. Gereffi & M. Korzeniewicz (Eds.) (1994) . |
[14] | Gereffi G. “Global value chains in a post-washington consensus world”. Review of International Political Economy. (2014) ; 21: (1). |
[15] | Gereffi G, Fernandez-Stark K. Global value chain analysis: a primer. Center on Globalization, Governance & Competitiveness (CGGC), Duke University, North Carolina, USA (2011) . |
[16] | Hernández V, Pedersen T. Global value chain configuration: A review and research agenda. BRQ Business Research Quarterly. (2017) ; 20: (2): 137-150. |
[17] | Eurostat. Power from Statistics: data, information and knowledge. Guidance Report. (2018) . |
[18] | Menghinello S, Ceccarelli C, Fedeli M. A knowledge-based approach to the statistical production process, paper presented at Q2018, the European Conference on data quality in official statistics, Poland. (2018) ; web reference https://www.q2018.pl/wp-content/uploads/2018/06/Q2018_abstract_book.pdf |
[19] | Reimsbach-Kounatze C. “The Proliferation of “Big Data” and Implications for Official Statistics and Statistical Agencies: A Preliminary Analysis. OECD Digital Economy Papers, No. 245, OECD Publishing, Paris. (2015) ; doi: 10.1787/5js7t9wqzvg8-en. |
[20] | Wallgren A, Wallgren B. Register- based Statistics: Administrative Data for Statistical Purposes. John Wiley & Sons, Ltd, Chichester, UK (second edition) (2013) . |
[21] | Alleva G. Adopting a Process-oriented Approach at the Italian National Institute of Statistics, presentation for the High-level seminar. April (2017) ; 19-21. UNECE, Korea. |
[22] | Luzi O, e Monducci R. “The new statistical register “Frame SBS”: overview and perspectives, “ Rivista di statistica ufficiale, ISTAT- Italian National Institute of Statistics- (Rome, ITALY). (2016) . 18: (1): 5-14. web reference http://www.istat.it/it/files/2016/11/1_luzi.pdf. |
[23] | Istat. Evento di presentazione del Frame Territoriale SBS. (2018) ; web reference https://www.istat.it/it/archivio/216268 |
[24] | Rolland A. “The concept and commodity of official statistics”. Statistical Journal of the IAOS. (2017) ; 33: (2): 373-385. |
[25] | European Commission. EU Regulation n∘ 549/2013 on the European System of National and Regional Accounts (ESA 2010), Bruxelles, (2013) . |
[26] | Eurostat. “Manual on Regional Accounts Methods”, Luxembourg (2013) . |
[27] | Istat eIce., “Annuario statistico sugli scambi con l’estero e leattività internazionali delle imprese”, web Reference www.annuarioistatice.it. 2018. |
[28] | Alleva G. “Integration of business and trade statistics: limitations and opportunities” DGINS Conference, Riga. September (2014) ; 24-25. |
[29] | Istat and MISE. “Start-up Survey”, Joint Research Report on the structure and evolution of innovative start up enterprises in Italy. (2018) ; web reference https://www.istat.it/en/archive/216308. |
[30] | Istat. “Rapporto sulla competitività dei settori produttivi”. (2018) ; web reference https://www.istat.it/storage/settori-produttivi/2018/Rapporto-competitivita-2018.pdf. |
[31] | Barcaroli G, Golini N, Righi P. “Quality evaluation of experimental statistics produced by making use of Big Data” European Conference on quality in official statistics, Krakov, (2018) . |