Semantic Web and its role in facilitating ICT data sharing for the circular economy: An ontology survey
Abstract
The environmental pressure, CO2 emissions (including embodied energy) and delivery risks of our digital infrastructures are increasing. The exponentially growing digitisation of services that drive the transition from industry 4.0 to industry 5.0 has resulted in a rising materials demand for ICT hardware manufacturing. ICT devices such as laptops and data servers are being used on average for 3 and 4–5 years respectively (van Driel (2020)), while research shows that they should last 7 years before replacement (Journal of Cleaner Production 69 (2014), 10–16). A solution is to transition from a linear to a circular economy (CE), through which materials that were previously disposed of as waste are re-entered back into product life-cycles through processes such as reuse, recycling, remanufacturing, repurposing. However, the adoption of the CE in the ICT sector is currently limited due to the lack of tools that support knowledge exchange between sustainability, ICT and technology experts in a standardised manner and the limited data availability, accessibility and interoperability needed to build such tools. Further, the already existing knowledge of the domain is fragmented into silos and the lack of a common terminology restricts its interoperability and usability. These also lead to transparency and responsibility issues along the supply chain. For many years now, the Semantic Web has been known to provide solutions to such issues in the form of ontologies. Several ontologies for the ICT, materials and CE domains have been build and successfully utilised to support processes such as predictive maintenance. However, there is a lack of a systematic analysis of the existing ontologies in these domains. Motivated by this, we present a literature survey and analysis of, but not limited to, existing ontologies for ICT devices such as laptops, materials and the CE. In addition, we discuss the need for findable, accessible, interoperable, reusable (FAIR) data in the CE, different factors such as data privacy and security that affect this and the role of ontologies.
1.Introduction
Facing global issues surrounding resource scarcity, energy consumption and carbon emissions, and ever growing waste streams, there is an increase in societal and governmental focus on solutions that rethink the current linear economic structure. One such solution that has seen legislative progress over the last decade in particular is the idea of a circular economy (CE) [68], commonly understood to be a model through which materials that were previously disposed of as waste are re-entered back into product life-cycles through operations (or strategies) such as reuse [116], recycling [2], remanufacturing [121], and repurposing [79]. Figure 1, which is an adaptation of the CE Butterfly11 diagram by the Ellen MacArthur Foundation,22 visualises the possible circular strategies that can be used for products and materials. The maintenance phase includes all monitoring, software upkeep, cleaning, and minor repairs necessary over the use phase of a product. This phase occurs simultaneously with the use cycle and has a direct impact on its lifetime by allowing the maintainer to proactively catch and prevent major failures. Reuse and redistribution occur when the product owner has decided that the product has reached its end of first use, but the product is in (or can be returned to) good working condition. The product owner can, for instance, choose to redistribute the device to another internal department or otherwise sell or donate the device for reuse elsewhere. Organisations such as the Toronto Tool Library33 provide a centralised subscription-based access to an inventory of various tools. Refurbishment and remanufacturing also occur at the end of first use. However, the product is usually relinquished back to the producer, supplier, or an ICT asset disposition company where it will undergo functionality and safety testing and may undergo data wiping, repairs or parts replacement, upgrades, and cosmetic touch ups. The product is generally returned to a “like new” condition for resale. Recycling occurs when the product owner decides the product has reached its end of life, where the product will no longer be used and becomes waste. The decision of when a product has reached end of life is made by the product owner and does not necessarily denote the end of the product’s functional usability. The recycling process involves the dismantling of a product, through mechanical or chemical means, down to its smallest possible constituent parts with the intention to reuse these recycled materials. Current recycling processes are often technologically limited in how efficiently they can recover pure, undegraded materials. Finally, the molecular decomposition phase occurs where biodegradable materials, such as bio-based plastics, are broken down to a molecular level.
Information and communications technology (ICT) such as laptops, is a major stream of focus in the CE due to its increasing impact on the environment, society, and the economy [7,34]. The increasing digitisation and unprecedented amount of data that is generated on a daily basis has resulted in ICT hardware such as laptops being used on average for 3-4 years [113], while research shows that they should last 7 years before they are replaced [13]. Based on this, at its current state, the ICT sector is not sustainable as there is a discrepancy between the economic lifetime and technical lifetime of ICT hardware. The result is short lifetime replacement cycles that increase the carbon footprint. Further, it is responsible for 2.1%–3.9% of the global greenhouse gas emissions [42]. The composition of ICT hardware alone, made up of varying combinations of critical raw materials, makes an interesting and important case for the growing CE [115]. Access to these materials is often fraught with complications relating to limited natural supplies, difficult geographic deposit locations, and precarious political agreements. The issues surrounding ICT hardware continues to follow the product through its life-cycle impacting the health and safety of mining communities and their surrounding environments, intense energy consumption (see [55]) and CO2 emissions during manufacturing, further impacting environmental and human health at end of life as a hazardous waste, and resulting in the loss of financially and otherwise valuable materials through disposal and inefficient recycling technologies. The CE seeks to address these issues through both product lifetime extension, i.e., keeping the materials in their original product longer, closing resource loops, and through the reuse or re-purposing of products, product components, and materials.
Based on a report44 [113] by the Amsterdam Economic Board,55 the adoption of the CE in the ICT sector (in the Netherlands) is currently limited. The main challenges at hand are (i) siloed knowledge about the CE and ICT within and between organisations resulting in lack of transparency and traceability of ICT procurement (i.e. the process of obtaining goods or service for a business) and (ii) data’s findability, acessibility and interpretation. The lack of a single CE standard that can be used as a main reference point across the European Union (EU) is another pressing challenge. As a result, there is a lack of a unified terminology for the CE and guidelines/best practices for its implementation across different sectors.
In organisations many departments (e.g. sales, IT, finance, delivery) are involved in the decision making-process for the implementation of information technology (IT) infrastructures. ICT experts provide technical assistance, while CE experts develop sustainability strategies that procurers can follow. However, the explanations for each decision (and the data for it) often remain within specific expert groups. This contributes to forming knowledge silos, which also leads to lack of decision making transparency and data traceability. Knowledge exchange should be better facilitated in an understandable for all expert groups manner so that both technical requirements and sustainability factors are considered during ICT procurement and maintenance.
Data’s findability, acessibility and interpretation are also common barriers when data sharing needs to be facilitated between companies, suppliers and manufacturers. For example, the production of an iPhone involves more than 200 component suppliers, spread over 43 countries and 6 continents [4]. Each supplier has specialised knowledge about their products such as their material composition, design and hardware-software dependencies. Such information is rarely available to end users and decision makers (procurers). Inaccurate, incomplete and unavailable data about an ICT device can lead to misleading and incorrect sustainability recommendations during processes such as life cycle assessment (LCA) [111]. Several studies, namely [70,95,104] confirm that data’s availability, format standardisation and quality are some of the key challenges for LCAs. Motivated by the lack of data availability, studies such as [7,66] have made progress in publishing online ICT (e.g. laptops, tablets) material datasets in the form of a bill of materials (BoMs). The datasets present material data for a specific component or for a device as a whole and do not follow a standard format that can easily facilitate their federation and interpretation. Furthermore, there is data available for only several laptop brands (e.g. Dell, Apple and HP) with models manufactured between 1999–2011, which can be considered as already outdated.
Last but not least, establishing CE standard(s) could also help to derive clear requirements for what specific ICT data should be made available, to whom under what circumstances. ICT devices such as a laptops comprise of multiple components (e.g. display screen, keyboard, base panel, top panel, cooling fan, random-access memory (RAM), hard disk, palm rest assembly, battery, hinges, speaker, optical drive, antenna). Each component has sub-components such as sensors and specific material composition. When a product needs to be refurbished, repaired, remanufactured it has to go through multiple processes (e.g. hardware and software testing). Testing can be standardised via tools such as Aiken66 and/or personalised based on the model and manufacturer of the product and the needs of the end-users. In both cases, unprecedented amount of heterogeneous data about both the device itself, its performance over time and the testing performed on it are generated, which is expensive with regards to the computational power and additional support that might be needed to generate, process, store, interpret and maintain data.
These are also factors that need to be considered when developing software that aims to support ICT decision making in cases such as predictive maintenance and sustainable procurement recommendations. Further, with the transition from industry 4.0 to 5.0, having meaningful data is essential as it can not only drive the automation but also the optimisation of services (in terms of computational costs and even energy consumption [112]). As discussed in [63], to gather and process such data, collaboration between different domain experts from the ICT, materials and sustainability domains is needed.
Table 1
FAIR principles, ICT challenges and the Semantic Web
FAIR Principle | Current ICT and CE Challenges | Semantic Web Solution - Ontologies |
Findable | Lack of available information and knowledge about circular solutions among ICT decision-makers. | Ontologies, as a technology, can be used to support the discovery of new and traceability of existing information in the ICT and CE domains via the interpretation of the URIs defined for each concept. |
Accessible | Many departments (e.g. sales, IT, finance, delivery) are involved in the decision making process for the implementation of an ICT environment, which results in knowledge silos. Only authorised individuals can access specific data. | Ontologies help integrate disparate silos of data by defining a unified terminology of a domain, which represents all of the involved entities (people, organisations, software) their specific roles, access rights etc. |
Interoperable | It is challenging for a non-expert in the domain to interpret materials and ICT data and make truly informed and impactful sustainability decisions. | Ontologies transform data into information through the use of RDF triples and URIs and represent it in a machine interpretable format. This can help build more intelligent tools (with artificial intelligence) to support informed human-decision making in the CE. |
Reusable | Lack of standardisation and documentation that is publicly available. Data from one silo/department has to be translated and interpreted when used for other purposes by another department. For example, data on existing laptop types in use by an IT department of some organisation might be made available for the purpose of adopting CE strategies by the procurement department. However it first needs to be accessed, interpreted and translated for the purpose of circular decision making. | An ontology can be used as a standard unified data model within an organisation to showcase (in one place) what data is used and is needed for specific processing (e.g predictive-maintenance). As a recommendation, an ontology should be documented and can be publicly available to support its reusability (a recommended principle for ontology engineering) and extension. |
The Semantic Web can provide a findable, accessible, interoperable, reusable (FAIR) solution to these challenges in the form of ontologies that can technologically drive the data FAIRification [61] process (see Table 1). Through the years, research such as [14,40] has shown ontologies’ ability to not only organise and interconnect knowledge within organisations but to also make it accessible and interoperable across machines. Due to their ability to represent dynamic contexts in a machine-readable format ([15,58]), ontologies have been widely utilised as knowledge organisation schemas in diverse domains such as cultural heritage as discussed in [36,41]. Further, reseach has shown that ontologies can successfully assist and even improve machines’ decision making in scenarios such as recommendations [18,25,120], legal compliance [23,39,69,108], predictive maintenance [21,22,80,81], tourism [65], chemical safety and drug design [91] and intelligent surveillance [33]. This is due to ontologies’ ability to enrich data with context and provide information in a machine-readable format. Further, both organisations and individuals can benefit significantly from the utilisation of ontologies, which help establish common understanding (i.e. unified vocabulary of real world concepts and their meaning) of a domain. Technology-wise, when it comes to facilitating ICT data sharing for the CE, more and more experts have been utilising Semantic Web technologies such as ontologies. As a start, the focus has been on building simpler knowledge organisational systems such as taxonomies. However, there has been a rise in building and utilising more complex linked data models such as ontologies and knowledge graphs (see [5,78,98]). “Linked Data can function as an exchange medium for the CE driving the “push and pull” between diverse industry resources.” [99]. However, there is a lack of systematic semantic analysis (i.e. discussion on the mechanisms used for building the model, the followed linked data principles, the scope and guidelines towards their reuse) of the existing ontologies for ICT data and materials and their relation to the CE domain.
This paper presents a systematic survey and analysis of existing semantic models (e.g. ontologies, taxonomies) for ICT devices such as laptops, materials and the CE. The main use case that motivated our work is the increasing use and manufacturing of laptops, which are often disposed of (e.g. replaced) earlier than needed (e.g. due to planned obsolescence [1,52]) and the associated ecological footprint in terms of CO2 and e-waste. The paper is aimed at motivating and assisting ontology engineers in selecting ontologies (e.g. classes, sub-classes, object and data properties) for reuse or extension, when building semantic technology-based tools for the CE. Further, we present an overview of tools in the domain that utilise semantic technologies. By analysing the state-of-the-art, we provide guidelines for building and utilising ontologies for advancing the implementation of the CE. We believe that our work can help both CE experts and ontology engineers as it provides an overview of existing domain ontologies, information on how they were built, their limitations (Section 4), and how they can be reused (Section 5). Further, we provide examples of research work (Section 4.4) that showcases the successful utilisation of ontologies within CE software tools.
The rest of the paper is structured as follows. Section 2 outlines the followed methodology, while Section 3 presents a set of requirements used for the selection and analysis of relevant existing semantic models. Section 4 presents an overview of existing semantic models in the ICT, materials and CE domains. The section also presents examples of CE tools that utilise ontologies. The analysis of the related work is presented in Section 5 followed by a discussion in Section 6 and the conclusions in Section 7.
2.Methodology
Fig. 2.
Survey methodology.
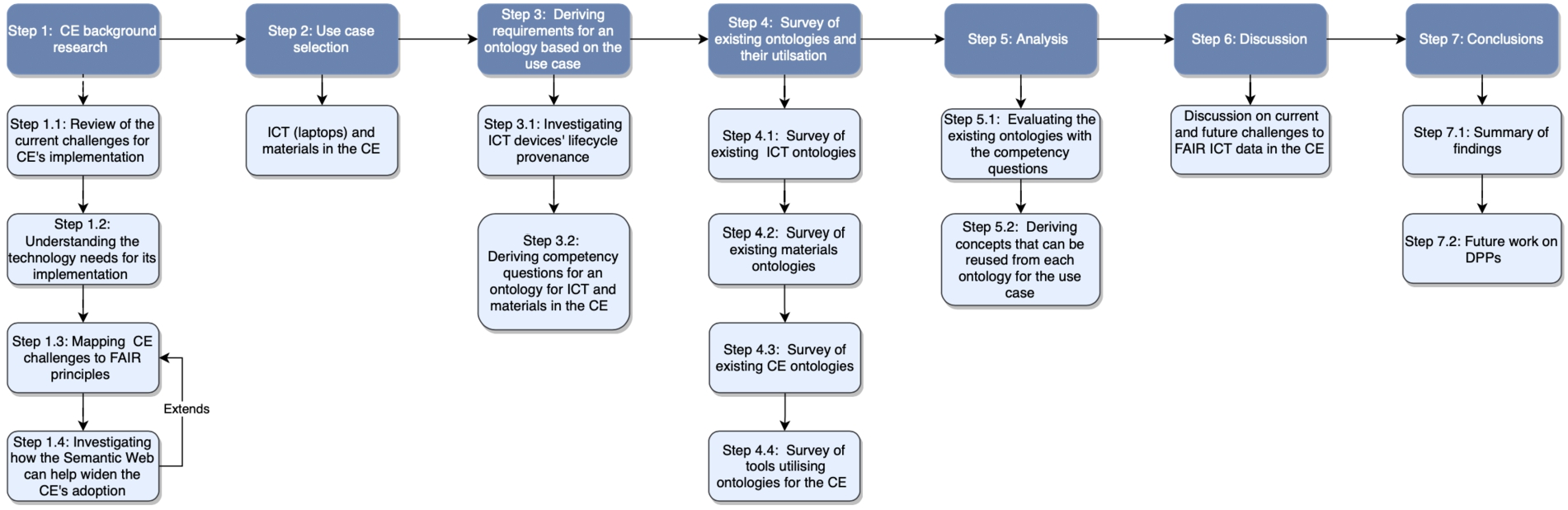
To compile this paper, guided by our previous survey in the Semantic Web domain (see [72]), we undertook several steps which are presented on Fig. 2. We began with background research into the CE to better understand its scope, goals and current level of implementation (Step 1). Next, based on the authors’ diverse expertise (computer science, CE, industrial design and sustainability), collaboration with refurbishment, ICT and CE domain experts and interviews with industry and government representatives (details in [84,85]), we reviewed some of the current challenges/barriers that limit the further adoption of the CE (Step 1.1). The collaboration has been done in the scope of the Circular Resource Planning for IT (RePlanIT)77 project, which aims to utilise ontologies to build digital product passports (DPPs) of ICT that can assist predictive maintenance and sustainable ICT procurement. During Step 1.2 we explored the technology needs of tools that support FAIR data implementations for the CE. Next, during Step 1.3, we mapped the existing CE challenges to each FAIR principle (Table 1). During Step 1.4, we extended Table 1 so that it clearly states how ontologies can be used to help solve each CE challenge to support the corresponding FAIR principle. To have a clear scope in the broad ICT domain, laptops (some of the most used and disposed of ICT devices nowadays) were selected as our main ICT use case (Step 2). During Step 3, we investigated the life-cycle of ICT devices and their components in the CE and designed an abstract model as presented on Fig. 3. Following this and focusing on laptops, we derived a set of competency questions (Step 3.2, Table 8 in the Appendix) that can be used as requirements for types of data needed to describe an ICT device, its components and materials in the CE. Next in Step 4, based on this we begin our survey of existing semantic models for the following three domains: ICT (with a focus on laptops), materials and the CE. We focused on ontologies that are open access or have been presented by a scientific publication or an official report/project deliverable. During our investigation, we encountered several studies that do not directly present ontologies but rather show their utilisation in the CE in practice. These studies have been briefly reviewed as well in Section 4.4. After the survey and summary of the related work, we evaluated (in Step 5) each one of the publicly available ontologies with the set of competency questions introduced in Step 3.2. This helped understand to what extent each ontology can model the domain and what specific concepts from it can be reused (to support best practices in ontology engineering). The analysis highlighted several discussion points, which we reflected on in Step 6 (see Section 6). Finally, all findings were concluded in Step 7 (Section 7).
The main sources for this survey were peer-reviewed scientific publications in the CE, ICT and Semantic Web domains, which we identified via Google Scholar,88 ACM Digital Library,99 IEEE Xplore,1010 Scopus1111 and DBLP.1212 A search for resources (e.g. updates on CE’s standartisaion, definitions) was also performed on the websites of standardisation bodies such as the British Standards Institution (BSI),1313 the EU Commision,1414 the International Electrotechnical Commission (IEC)1515 and the International Organisation for Standardisation (ISO).1616 The main keywords used were CE, CE strategies, CE data sharing, CE privacy, CE data model, CE ontology, CE taxonomy, CE standards, CE legislation, CE sustainability, linear economy, CE tools, sustainable ICT, circular ICT, ICT data model, ICT ontology, ICT taxonomy, ICT data sharing, ICT environmental impact, ICT manufacturing, materials, critical materials, raw materials, materials data model, materials ontology, materials taxonomy, FAIR principles, FAIR data sharing. Insights have also been gathered from the authors’ current involvement in RePlanIT and part participation in the CampaNeo1717 smashHit,1818 KI-NET1919 projects, which focused on IoT data sharing, digital twins and predictive maintenance enabled by ontologies and knowledge graphs.
3.Requirements for an ICT ontology for the CE
To illustrate the complexity of interlinking the ICT, materials and CE domains we present a graphical representation on Fig. 3 of and ICT device’s life-cycle in terms its life-cycle provenance. An ICT device (e.g. a laptop) can be represented in terms of the components that it is comprised of. Each component has provenance information, which is a record of its material, sustainability and physical properties and changes in them that have occurred as a result of an implemented CE strategy. ICT and materials provenance and lineage is vital for supporting product life-cycle assessment [8,62], establishing responsibility along the supply chain and for implementing CE strategies such as predictive maintenance.
Based on this and on our collaboration with the refurbishment, sustainability and CE experts during the RePlanIT7project, we present a set of competency questions (see Table 8 in the Appendix) that can be used as a starting point (or a guideline) when building an ontology for ICT’s and its materials’ lifecycle management in the CE. We have also used the competency questions to evaluate the relevance of each existing ontology (Section 5) with regards to our use case. The evaluation results can help to better understand which semantic model and which specific concepts from it can be reused to build a common shared vocabulary for ICT in the CE. Table 8 presents the competency questions (with focus on laptops) organised in six categories: (i) ICT devices, (ii) ICT device’s components, (iii) physical properties of ICT devices and their components such as weight, age, warranty duration, usually (specified by a manufacturer but can be updated after a CE strategy such as refurbishment is used) (iv) ICT sustainability properties related to the environmental impact of the device, (v) material properties of ICT devices and (vi) CE strategies that can be adopted. For example, the first category (ICT devices) comprises of questions such as “What is the type of the ICT device?, What is the brand of the device?, When was the device assembled?, What are the components of a device? etc.”, which assist in building a general semantic representation of a device. The next categories (e.g. ICT device component) focus on lower-level questions (e.g. “What is the serial number of the component?, What is the status of the device’s component? Has it been reused, remanufactured, or refurbished before?, What is the brand of the component?”) and help define a more granular laptop representation (one can answer the questions for each component of a laptop). To further support the ontology engineering process, we have derived the key concepts that an ontology should represent to answer each question (see Table 8 in the Appendix).
4.State of the art
This section presents an overview of existing semantic models in the ICT, materials and CE domains. Specific focus is put on ICT devices such as laptops and their hardware components. An overview of the existing CE tools that utilise semantics is presented as well. For each ontology, we presents its (i) purpose and scope, (ii) modelling language, (iii) conformace to best practices for ontology engineering, (iv) level of reuse of existing ontologies, (v) availability (open-access or private), (vi) limitations and (vii) possible applications.
4.1.Semantic models for ICT
This section presents an analysis of the existing semantic models in the ICT domain ranging from single device-focused ontologies to more generic semantic models such as top level-ontologies.
4.1.1.ICT energy resource management ontology by Daouadji et al. [31]
Daouadji et al. [31] present an ICT resource management ontology for the energy efficiency domain. The ontology, which was built with the Resource Description Framework (RDF),2020 models three of the most common types of ICT - Networking, Computing and Storage and generic resources related to them such as CPU and bandwith. The connection with the energy efficiency domain is set by the used-by object property, which relates an ICT resource to either Green or Dirty energy types. The ontology is not open access, which limits the analysis that can be performed on it. Competency questions, if used, are also not presented. Although, one could gain an idea of the data model at a taxonomy level, no specific URIs are presented in the paper. This limits the ontology’s reuse and application for other use cases. The reuse of existing ontologies has not been discussed as well. The ontology has been utilised for implementing a framework, which assists users in identifying the best energy resources that can be used for a specific task in terms of cleanliness [31]. Although the framework’s evaluation has shown promising results in terms of the use of semantics to improve the frameworks search performance further investigation is needed to fully grasp its scalability in terms of performance and accuracy. The ontology itself can be used as a starting point for building more complex semantic models for ICT use in the CE, where energy efficiency and use of renewable energy can be viewed as a sustainability indicator [64,89].
4.1.2.ICT governance and policy taxonomy by Lampathaki et al. [75]
Motivated by the technological advancements of government-supporting tools and the increasing need for data governance, Lampathaki et al. [75] propose a taxonomy that helps categorise ICT research. Being developed in the context of the CROSSROAD2121 project, the taxonomy’s domain is defined as ICT for governance and policy modelling. The authors focus on building a generic unified model for the different applications and research areas (e.g. social networking, linked data, IoT, cloud computing) for ICT. Five research areas have been defined in total, each with several sub-areas to provide a detailed specification of ICT applications. No details of ICT devices or components are presented as the taxonomy focuses on upper level knowledge representation. Although the data model is not openly available and is at a taxonomy level, it can be used as a guideline for an ontology or for extending existing ontologies with the possible applications of ICT, especially when security and privacy are the key focus.
4.1.3.ICT assistive technology taxonomy by Gower and Andrich [50]
The lack of standardisation in terminology in the ICT domain has also inspired Gower and Andrich [50], who propose a taxonomy for ICT devices’ features in the assistive technology (AT) domain. The taxonomy comprises of two main categories - features and clusters. Features represent different measures (e.g. weight, length) and attributes (boolean values) of ICT devices, while clusters are a combination of several features. Some of the modelled clusters, which help represent knowledge about an ICT device in detail, include browsers, licenses, price, visualisation and energy type. The taxonomy has been successfully adopted by the European Thematic Network on Assistive Information and Communication Technologies (ETNA)2222 framework, which aims to provide access to the different ICT products available on the market based on the needs of the end-users. The ISO 9999, ISO 24751 and ISO division 22.39.12 standards were taken into consideration when building the taxonomy. The taxonomy is not openly available, however, the presented hierarchy in [50] can be already reused for defining an ontological model of an ICT device, its functionalities, capacity, input and output. Further, the authors provide a discussion about the maintenance of the taxonomy, which is an often overlooked discussion in such papers.
4.1.4.Laptop ontology by Dhingra and Bhatia [35]
Dhingra and Bhatia [35] discuss the different requirements and tools that are available for building ontologies and propose three ontologies in the laptop domain, which represent laptop reviews, specifications and sellers. In the laptop review ontology, a laptop can be associated with specific advisors in the form of customer feedback, rating and reviews, which were collected from different media sources (i.e. newspapers and magazines). The laptop specification ontology models laptop specifications such as audio devices (microphone, stereo speakers), brand, camera, dimensions, display size. The laptop seller ontology, on the other hand, focuses on the selling process itself (from purchase to delivery). Different payment methods are modelled as well. Although the work in [35] follows the ontology engineering methodology from [88] it is not openly available, there is no mention of the specific object properties between the classes, how all three ontologies connect and if any existing ontologies were reused. Namespaces are not presented as well, which limits the reuse of the work. The evaluation of the ontology with a set of competency questions, which were translated into Description Logic (DL) queries, showed that it is expressive enough to be used in use cases such as buying of laptops.
4.1.5.ICT taxonomy by Inaba and Squicciarini [60]
By following the International Patent Classification (IPC)2323 [117] system, the ICT patents presented in it and by building upon the Japan Patent Office (JPO)2424 classification system [90], Inaba and Squicciarini [60] propose the J tag ICT taxonomy. The taxonomy categorises ICT products based on the technology area of their application. 13 such areas have been defined in [60] amongst which are mobile communication, security, sensor and device network, Each technology area is also divided into several subareas and is associated with specific IPC classes from the IPC classification system. For example, the ICT device area has as a subarea an electronic circuit, which is associated with IPC codes H03B (direct generation of oscillations), H03C (modulation) etc. The presented taxonomy in [60] is one of the most detailed that was encountered during our survey. The authors have analysed different standards and have provided a detailed specification of the whole taxonomy. Although, no ontology has been built, the J tag taxonomy documentation can be used as guidelines for any ICT practitioner and ontology engineer working in the domain.
4.1.6.oneM2M base ontology [107]
The ontology is the main semantic model used by the oneM2M2525 initiative, which is a collaboration between 8 leading IoT standardisation organisations. The base ontology, built with the Web Ontology Language (OWL),2626 models the concept of a device as a thing that has to achieve a specific task and which can also be a collection of several devices (with unique identifiers). Each device has a service and operation with input and output data associated with it. Services have specific functions (e.g. controlling and measuring). To achieve its task, a device needs to complete a specific function. Although an ontology is metadata by itself, the concept has been defined as a separate class to represents the values and measures of things. Based on the predefined namespaces, existing ontologies have not been reused. The oneM2M ontology by itself can be reused as an upper level ontology when building a more detailed semantic model of ICT devices. It can also be extended with specific concepts such as sensors and switches from the DogOnt [17] ontology to achieve higher granularity level. As discussed in [17], mapping of other ontologies such as the SmartAppliances REFerence (SAREF) [30], to oneM2M is possible and guidelines are provided in [110].
4.1.7.DogOnt ontology for IntelligentDomotic environments by Bonino and Russis [17]
One of the earliest and most expressive OWL ontologies that represents smart devices is DogOnt [17]. The ontology, which was built for the domotic domain, represents smart devices, their location, capabilities and technology-specific features and states. With this level of detail, DogOnt can assist ontology engineers in modelling complex Intelligent Enviroments (IEs) and devices such as home and office appliances that comprise them. However, ICT is not the main focus of the work, specific switches (e.g. on off, rocket and level control) and sensors (e.g. temperature, CO2, humidity and light detection) are modelled as well. Several ontologies such as the Semantic Sensor Network (SSN) [26], Dublin Core,2727 Creative Commons ,2828 Measurement Units Ontology(MUO)2929 and Unified Code for Units of Measure (UCUM)3030 have been reused. Further, as shown in [17], the authors provide reasoning mechanisms based on DogOnt (e.g. model instantiation), which show its successful utilisation. Detailed specification of the ontology is available online,3131 which supports its future reuse and extension - one of the core principles of the Semantic Web. On the other hand, DogOnt’s high granularity level raises the challenge of its reuse as well (how and what to reuse).
4.1.8.Laptop ontology by Ayundhita et al. [6]
Similarly to [35], Ayundhita et al. propose an ontology for the laptop domain aimed at assisting a conversational recommender system (CRS) in making better product recommendations. The developed ontology, which builds upon the work in [11], models three main laptop-related concepts - functional requirements, the product itself and the products’ specification (gathered from its manufacturer). Several types of products such as notebooks and ultrabooks have been modelled. The instances of these classes can be categorised as high, mid and low-end products based on product specifications such as RAM memory. The ontology is not open access and it is not clear if the authors followed Semantic Web standards such as OWL and RDF when building it. However, the authors have shown its successful utilisation and improvement of the accuracy of CRS’s recommendations.
4.1.9.High-level ontology network for ICT infrastructures by Corcho et al. [29]
To solve some of the challenges within ICT services such as lack of common understanding, heterogeneous data and the presence of knowledge silos, Corcho et al. [29] propose a network of 9 interconnected ontologies that model different entities (organisations, data centers), hardware and software components and network security. These ontologies fall under a top-level ontology which has the specific goal to present a high-level model of ICT component configurations, resources and the relationships that hold between in a machine readable format that supports ICT service management. The ontology was built by following the Linked Open Terms (LOT) methodology with OWL. Dublin Core and the Simple Knowledge Organisation System (SKOS) [86] have been reused. The ontology is openly available online3232 which eases its reuse. The modular design that was adopted supports the reuse of the ontology network at different levels (each one of the 9 ontologies can be reused). Further, the ontologies were used as a schema for a knowledge graph which has also been used for question answering by a chatbot deployed at Huawei3333 [29].
4.1.10.Hardware and DevOps ontology by Corcho et al. [28]
In addition to the work in [29], Corcho et al. have built an ontology [28] that focuses on representing hardware items related to software development and IT operations (DevOps) infrastructures. The ontology, built with OWL, represents several types of hardware items (e.g. disk, frame, network card, server hardware), server hardware types such as firewalls and switches and characteristics such as bandwith, port, power, disk size that can be used to describe the items in detail. Similarly to [29], concepts from Dublin Core, SKOS, DevOps [29] have been reused. Both the ontology and its documentation are available online,3434 which promotes its reuse within the Semantic Web community. Currently, several inconsistencies in the labeling are evident. Further, due to the limited documentation online, it is not clear if the ontology has been evaluated with competency questions and if it has already been utilised in specific use cases. Several of the defined concepts such as disk, switch and network card and their object properties can be reused to increase the granularity of top-level ontologies or when following a bottom-up methodology for ontology engineering.
4.1.11.Laptop ontology by Yowe and Astawa [118]
Yowe and Astawa [118] recognise the need for information organisation and its machine-readable representation in the ICT domain and prose a laptop hardware-focused ontology. This is one of the few ontologies that represents laptop’s specific hardware components (e.g. processor, screen, types of storage, GPU) and their functional characteristics (e.g. storage size, screen size.) The concepts of brand and price, which can significantly influence one’s decision making when selecting a laptop for procurement or personal use have been represented as well. By far this ontology is one of the few that fit our use case. However, it is not publicly available and has not been documented. According to [118], the ontology has been evaluated in terms of its ability to be used for data annotation and querying. Details on the ontology’s evaluation in terms of its engineering, however, have not been provided.
4.1.12.Summary
Table 2 presents a summary of the overviewed ICT semantic models based on the criteria that was specified in the beginning of Section 4. For each model, the table provides information on its type (taxonomy, schema, ontology), scope and year of last update. We have also reviewed each model’s conformace to best practices for ontology engineering in terms of the followed Semantic Web standard, the model’s availability and the level of reuse of existing ontologies (also noted in the table). Known limitations, current and possible applications for each model are presented as well. The information has been derived from each models’ online documentation and scientific publication and by using the OOPS! [94] pitfall scanner (for the public ontologies). A “–” symbol is used when no information has been found in the resources.
Table 2
Overview of ICT semantic models
Study | Type | Year of Latest Update | Semantic Web Standard | Scope | Reuse of Existing Ontologies | Availability | Limitations | Applications |
Daouadji et al. [31] | Schema | 2010 | RDF | Energy efficiency; low carbon grid networks. | – | Private | No public URIs, unclear if competency questions were used and if existing ontologies were reused. | Used within a framework, which assists users in identifying the best resources that can be used for a specific task in terms of carbon footprint and energy consumption. |
Lampathaki et al. [75] | Taxonomy | 2010 | – | ICT governance and policy management. | – | Private | Upper level (general) taxonomy for the ICT domain. No specific ICT devices and components are modelled. | Assisting ICT governance. |
Gower and Andrich [50] | Taxonomy | 2014 | – | ICT for AT. | – | Private | Focus mainly on ICT features and clusters in the AT domain. | Used to support AT information interoperability within the ETNA framework. |
Dhingra and Bhatia [35] | Ontology | 2015 | OWL | ICT; laptops (specification, review, selling). | – | Private | Focus on laptops for the e-commerce domain. Specific URIs, namespaces and object properties were not mentioned. | Used for modelling laptop information needed for the online selling. |
Inaba and Squicciarini [60] | Taxonomy | 2017 | – | ICT technology based on IPC and JPO standards. | – | Public | Remains a taxonomy. Focus mainly on ICT software, ICT services and areas of applications and not on ICT types and their hardware. | Represents specific electrical components in ICT and can be used to classify ICT based on its applications. |
oneM2M Consortium [110] | Ontology | 2019 | OWL | IoT and oneM2M device ecosystem. | – | Public | Generic ICT device representation with focus on operations, services, functions and their inputs and outputs. Missing class annotations and object property characteristics, inconsistent labelling. | Can be used to extend SAREF [30] ontology with more context for ICT use. Guidelines have been provided as well. |
Bonino and Russis [17] | Ontology | 2019 | OWL | Smart devices and IEs. | SSN, Dublin Core, Creative Commons, MUO, UCUM | Public | Focus primarily on home and office appliances and sensors in them. Properties missing domain and range, unconnected concepts, inconsistent reuse and naming of concepts. | Used to assist in auto-completion mechanisms, which based on reasoning can help model dynamic house modelling processes. |
Table 2
(Continued)
Study | Type | Year of Latest Update | Semantic Web Standard | Scope | Reuse of Existing Ontologies | Availability | Limitations | Applications |
Ayundhita et al. [6] | Ontology | 2019 | OWL | Laptops and laptop CRS. | – | Private | Focus only on laptops and information that can help improve online retail recommendations (e.g. functional specifications). Not available online and not documented. | Used for improving laptop retail recommendations based. |
Corcho et al. [28] | Ontology | 2021 | OWL | Hardware and DevOps. | Dublin Core, SKOS | Public | High-level ICT representations. A complex ontology network that although built in a modular manner has many interdependencies. | Used as a basis for a knowledge graph that assists question answering by a chatbot. |
Corcho et al. [29] | Ontology | 2021 | OWL | ICT infrastructures and configuration management systems. | Dublin Core, DevOps | Public | As part of the ontology network in [29] it is dependent on other modules in it. Labelling inconsistencies and unconnected concepts within the ontology. | Used to extend [29] with specific ICT hardware such as hard disks, switches, networks cards, some of which are components of devices such as laptops and data servers. |
Yowe and Astawa [118] | Ontology | 2021 | OWL | Laptops and their components | – | Private | Limited access to the ontology. Unknown evaluation details. Has not been documented. | Can be used to model laptops, their specific hardware components and functional characteristics. |
11 semantic models (3 taxonomies, 1 RDF schema and 7 ontologies) have been identified as revelant to the ICT domain. The literature review has shown that the available semantic models vary in terms of the granularity level of the represented knowledge. Some taxonomies such as [50] and [60] are highly expressive (represent numerous ICT-related concepts) and even follow specific ICT standards. However, they have not been implemented into ontologies yet thus their true benefit for machines (e.g. utilisation for decision making) is unexplored. Other models such as the hardware and DevOps ontology [28], DogOnt [17] represent less concepts but are already encoded as ontologies, are openly availabe and ready for reuse. Ontologies such as [29,31,75] focus on representing ICT infrastructures and their management as a whole, while others (see [17,28]) represent specific ICT hardware components and their capabilities. Only 4 of the ontologies [17,28,29,110] are openly available, which allows one to reuse specific namespaces and URIs. Although the rest of the semantic models (taxonomies and ontologies) have been documented in the form of public reports or scientific publications, namespaces and URIs, to support their reuse, are rarely available online. OWL was the most used Semantic Web standard. In conclusion, the analysis has shown that modelling the ICT domain is a complex use case dependent task. The reuse of the existing ontologies, which is currently at a low level, should be encouraged (e.g. making ontologies and taxonomies public).
4.2.Semantic models for materials
This subsection presents an overview of semantic models for materials motivated by the existing work that was reviewed in [102]. The importance of ICT and materials in the CE is discussed in Section 1.
4.2.1.MaTOnto by Cheung et al. [20]
Motivated by the increased availability of material data and the lack of its standartisation, Cheung et al. [20] present the MatOnto ontology, which aims to ease data-driven material discovery. The ontology follows the OWL Semantic Web standard and is based on the DOLCE [44] upper level ontology. MatOnto models several categories (ceramic, glass, polymer, metal) of materials, their properies (magnetic, chemical, mechanical, biological) and data measured during the materials’ modelling and evaluation. To model specific scientific activities and experiments related to material discovery several other ontologies have been reused as well. These include the Ontolingua’s Standard Units and Dimensions [51], W3C’s Time,3535 ABC Metadata [73] and the EXPO [103] ontologies. In addition to making the MatOnto openly availabe, the authors include specific namespaces in its specification in [20] as well. Such information eases ontology engineers when reusing existing work and saves time as one can directly see if the concept that is describes in the study has been reused or not. MatOnto can be used to semantically describe the whole process of discovering new materials, their characteristics and possible interactions and entities such as projects and organisations involved in the process.
4.2.2.Materials ontology by Ashino [5]
Data heterogeneity is also a challenge in the materials domain. In [5], Ashino sees this as an opportunity to create an infrastructure that supports material knowledge exchange. The author presents a materials ontology, built with OWL, that comprises of 7 sub-ontologies related to materials and their properties. The ontologies are organised in three groups - core ontologies, material information and peripheral ontologies. The core ontologies model various materials, processes, properties and the environment. The substances ontology, for example, can represent different substances as either pure or mixture. Each material can be associated with its relevant chemical, thermal and mechanical properties, which are modelled by the property ontology. From an ontology engineering perspective, the naming (or labels) of some sub-ontologies (e.g. core ontologies and peripehrial ontologies) lack consistency. Although several existing ontologies have been discussed, it is not clear if they have been reused and the level of their reuse. The modularity of the ontologies in [5] supports their reuse and extension. However, the ontologies are private and no specific namespaces are presented in [5]. The ontology has been successfully utilised as a way to synchronise material data exchange amongst three different databases and can be informative with regards to the types of properties that can be modelled.
4.2.3.eNanoMapper by Hastings et al. [53]
As part of the eNanoMapper3636 project, Hasting et al. focus on the semantic representation of nanomaterials and propose the eNanoMapper3737 ontology. The ontology, built with OWL, provides a detailed schema of nanoparticles based on their properties, constituency and shape [53]. It also models physicochemical and biological characteristics of engineered nanomaterials, which is useful for building nanomaterials for specific purposes such as drug delivery. eNanoMapper was built based on ontology reuse. Specifically, BFO3838 and ChEBI [32] have been reused. Further, the authors have implemented an ontology “slimming” library 3939 that supports the reuse by selecting only concepts and relationships that meet a predefined set or requirements. The library and the ontology are both openly available which is a step towards their reuse in the nanomaterial safety domain.
4.2.4.Metallic materials ontology (MMOY) by Zhang et al. [119]
To built the MMOY ontology, Zhang et al. [119] undertake a slightly different approach for ontology engeneering to the existing traditional (manual) ones. The authors utilise the String Matching on Ordered Alphabets (SMOA) [105] algorithm, which extracts existing metallic material related concepts from the Yago4040 knowledge base. This is done with the help of keywords and synonyms generated with WordNet.4141 The process is also supported by logic rules, which model the specific requirements that need to be met when extracting both hierarchical and non-hierarchical structures. The resulting ontology was evaluate with regards to precision, recall, F1 measure and time performance. The results showed the feasibility and correctness of the approach. However, although MMOY represents diverse metallic materials (e.g. alloy, iron), it lacks concept descriptions [119] and consistent utilisation of RDF and URIs as data was extracted from various Yago files. Further, MMOY is private, which restricts its analysis from an ontology engineering perspective. Finally, to show the successful application of the ontology, Zhang et al. [119] have built a prototype visualisation system based on it, which helps individuals explore specific metals and their properties.
4.2.5.Elemental multi-perspective material ontology (EMMO) [27]
Developed as part of several European projects (e.g. SimDOME ,4242 OntoCommons4343) that focus on material science standartisation, EMMO was built to represent even the smallest 4D world object that exists from different perspectives. The ontology comprises of several top and middle layer ontologies. The top level EMMO ontologies model quantum, physical and void items and collection of items, while the middle level ontologies focus on supporting the application of EMMO to specific domains. By being built with OWL, EMMO also supports the semantic representation of materials and is already reused by ontologies such as the Battery Interface Ontology (BattINFO),4444 the ontology for the Battery Value Chain (BVC) 4545 and the Mappings ontology.4646 On its own, EMMO reuses Dublin Core and is openly available, which supports its further extension and reuse for modelling of materials at physical and chemistry levels. However, due to its abstract nature and domain complexity, reusing or extending EMMO might require iterative collaboration between domain and ontology experts.
4.2.6.The BIM-based holistic tools for energy-driven existing residences (BIMMER) ontology [109]
The BIMMER ontology, built with OWL, is a modular ontology that focuses on representing several domains such as the building, material, energy consumption and weather in order to assist the integration of multiple external data sources for building model generations. Sensor data (e.g. occupancy measurements) has been modelled as well, which is an extension of existing such ontologies. Several ontologies have been reused (e.g. GEO,4747 Smart Appliances REFerence (SAREF) [30], SKOS, Time 4848). The ontology represents both high- and low-level concepts in the domains mentioned above and is openly available. To ease its reuse even further, within the BIMMER project, the authors have provided a detailed documentation in [109] and have transformed the OWL serialisation to JSON-LD format.
4.2.7.Materials graph ontology by Voigt and Kalidindi [114]
The work of Voigt and Kalidindi [114] presents a materials graph and an ontology based on it that aim to support the fomalisation and merge of knowledge in the domain. The authors follow the suggested material definition in [19] based on which four components (i.e. processing, structure, properties, performance) define a material. Provenance of the material’s process history and process hierarchies can be modelled as well. This is helpful when determining the sequence of process execution (e.g. heat treat, soak, ramp) for each material. The ontology presents the minimum set of concepts needed to model existing and new materials and their dependencies (i.e. relationships that hold between the materials). The ontology has been sucessfully used to generate a materials graph by utilising the dataset from [67]. Although several standards and ontologies have been discussed, it is not clear if the authors have reused any of them. Supplementary materials, including the ontology itself are also available online .4949
4.2.8.Materials design ontology (MDO) by Lambrix et al. [74]
One of the latest ontologies in the domain is the MDO5050 ontology, which was built to help integrate heterogeneous databases and extend the existing efforts of the Databases Integration for Materials Design (OPTIMADE) [3] community. The main goal of OPTIMADE is to make materials databases interoperable by setting a standardised REST APIs [3]. By following the NeOn [106] methodology for ontology engineering, Lambrix et al. have built the MDO ontology in a modular way. MDO models knowledge in the materials domain, specifically solid state physics, condensed matter theory and various material calculations. The ontology comprises of a Core module representing top-level concepts, Structure module representing structural material information (e.g. composition, lattice, occupancy) and a calculation module categorising the different computational methods used for creating the materials. Materials’ provenance information such as the agent associated with it and the date and time it was published can be represented with the Provenance module (reused from the PROV-O5151 [76] ontology). Quantities, Units, Dimensions and Data Types Ontologies (QUADT) [56] ontology has been reused as well. Extending MDO with concepts modelling experimental data used for calculating diffraction of X rays and the elasticity of materials has been set as future work [74].
4.2.9.Dislocation ontology by Ihsan et al. [59]
Ihsan et al. [59] narrow the focus of their work down to specific class of materials called crystalline and the commonly encountered disclocations (i.e. “a line-like defect” [59]) in their structure. The main goal of the ontology is to formalise the existing knowledge on crystallines based on their crystallography and to encourage future research in the domain. The proposed ontology has been published online .5252 Several concepts (e.g. lattice, occupancy) from existing ontologies such as the Materials Design Ontology (MDO)5353 [74] have been reused. The study in [59] presents initial steps for the development of such ontology thus deeper exploration of the domain is needed to build a more mature ontology.
4.2.10.Materials and molecules basic ontology (MAMBO) by Piane et al. [93]
Piane et al. present the MAMBO ontology that semantically represents materials at molecular level to support community’s material development efforts at nanoscale level. In MAMBO, a material has a structure, which can comprise of different molecular units. Each such unit can be modelled down to particle and atomic level. Materials can also be related to measurements and calculations. Existing ontologies have not been reused. Due to its modularity, MAMBO can be easily extended and reused for other domains such as molecular materials, nanomaterials, supramolecular and bio-organic systems as suggested by the authors. More specifically, MAMBO can be integrated with already existing ontologies such as EMMO and MDO. Although the ontology is still in its initial development stages, it is openly available5454 and can already be used as a guideline for future work in the domain.
4.2.11.Summary
By following the same criteria as presented in Section 4.1.12, in this section we present a summary of all findings regarding the state of the art of materials’ semantic models. In the past few years, several material ontologies have been built as shown in Table 3. Apart from, the MMOY [119] ontology, which was built automatically, all other ontologies were built manually by domain experts. This relates to the need for human involvement in the ontology engineering process. Although with automatic methods ontologies can be built faster, they usually lack the human knowledge of the domain, the iterative collaboration between several domain experts and URIs. Regarding the scope of the ontologies, some such as [53] and [93] model materials at nanoscale, while [20] focuses on modelling materials in a generic way. The EMMO [27] ontology, on the other hand, look at materials from a philosophical perspective, while [119] and [59] focus on specific materials and their properties (metals and crystalline materials). As shown in Table 3, most of the ontologies are openly available, which is a good Semantic Web practice for knowledge exchange. Finally, the reuse of these ontologies is also supported by their modular design.
Table 3
Overview of materials semantic models
Study | Type | Year of Latest Update | Semantic Web Standard | Scope | Reuse of Existing Ontologies | Availability | Limitations | Applications |
Cheung et al. [20] | Ontology | 2008 | OWL | Materials and material discovery. | Standards Units and Dimensions, Time, ABC Metadata, EXPO | Public | Represents materials from a chemistry and physics perspectives. Reuse in generic cases such as materials in ICT will require the support of a material scientist. Several classes miss domain and range, definition of wrong equivalent classes, missing annotations. | Semantically represent the process of material discovery. |
Ashino et al. [5] | Ontology | 2010 | OWL | Material’s composition and properties. | – | Private | Unavailability of the ontology. Inconsistent naming of classes and properties in the different modules of the ontology. | Used to synchronise material data exchange between databases. |
Hastings et al. [53] | Ontology | 2015 | OWL | Nanomaterials and nanosafity. | BFO, ChEBI | Public | Limited use cases it can be reused in due to its focus mainly on a specific type of materials - nanomaterials. | Used for evaluating nanomaterisls’ safety. |
Zhang et al. [119] | Ontology | 2016 | RDF | Metals and metallic properties. | – | Private | Focuses only on metals and their chemical properties, which limits its reuse. Class and object property URIs are not available. Limited semantics. Generated automatically from Yago data at a specific time and is subject to structural change everytime the data source is updated. | Used to validate an approach for automatic ontology generation. |
EMMO Consortium [27] | Ontology | 2021 | OWL | 4D objects, materials, material types, physics and chemistry. | Dublin Core | Public | Abstract representation of objects from analytical philosophy and physics perspectives. Limited details about ICT and materials are modelled. Its wider reuse for more generic use cases can be a challenge. | Reused by the BattNFO, BVC and the Mappings ontology etc. as an upper level ontology. Can be used to represent different perspectives of the world at different complexity levels. |
BIMMER Project [109] | Ontology | 2021 | OWL | Buildings, building materials and weather. | GEO, SAREF, SKOS, Time | Public | Limited scope on building materials (e.g. building boards) and not on material’s composition itself. Several modules of the ontology need to be reused to represent concrete material use. | Used to model renovation processes in buildings. |
Table 3
(Continued)
Study | Type | Year of Latest Update | Semantic Web Standard | Scope | Reuse of Existing Ontologies | Availability | Limitations | Applications |
Voigt et al. [114] | Ontology | 2021 | OWL | Material’s processes, performance, properties and structure. | – | Public | No specific materials have been represented. Lack of annotations and object property characteristics. | Can be used as an upper level ontology for modelling generic material discovery. |
Ihsan et al. [59] | Ontology | 2021 | OWL | Crystaline materials and crystaline dislocations. | MDO | Private | The ontology is in the initial stages of its development and is focused on only one material and one of its properties. | Used as a vocabulary for crystalline dislocations. |
Piane et al. [93] | Ontology | 2022 | OWL | Materials and nanomaterials. | – | Public | Initial stage of developments. | Used as a vocabulary and in assisting the integration of data for molecular materials, nanomaterials and calculations with them. |
Lambrix et al. [74] | Ontology | 2022 | OWL | Materials, solid state physics and condensed matter theory. | PROV-O, QUDT | Public | Structural changes possible due to it being in early stages of development with envisioned iterations. | To support data harmonisation and federation between several materials databases. |
4.3.Semantic models for the CE
This section presents an overview of the existing semantic models that represent the CE domain.
4.3.1.CE business models (CEBMs) by Chiaroni et al. [24]
As a result of an in depth analysis of the CE, Chiaroni et al. [24] present a taxonomony for it. The main goal of the taxonomy is to help determine the degree of adoption of the CE based on two factors - customer value proposition and the value network. Product or service price and promotion, features related to them and the degree of circularity have been defined as the most important criteria for business categorisation based on customer value proposition. For the value network, the following variable types have been defined - design for recycling (DfR), design for remanufacturing and reuse (DfRe), design for disassembly (DfD) and design for environment (DfE). Further, three levels of CE adoption have been distinguished, namely linear, upstream, downstream and full circular. Although the proposed taxonomy is used as a framework for the evaluation of the CE’s adoption, it presents CE terminology and processes that can be modelled with an ontology to support machines. The proposed taxonomy is documented in detail but from a business and CE domain expert perspective. Technology utilisation has not been discussed.
4.3.2.CE conceptual model by Sauter and Witjes [99]
Motivated by the potential benefits of utilising Linked Data for data sharing in the CE, Sauter and Witjes present a taxonomy and ontology in [99] for the CE to help standardise product passport data exchange. The developed taxonomy focuses on a retail use case in the CE and on the combination of Linked Data and QR codes. It models resources and actors. Resources can be bio-based or technological, while actors can be organisations and individuals (e.g. designers, farmers, consumers). Each resource has product parts and material composition. Provenance information such as the products’ creation company, certifications (e.g. Fair Trade) and use activities are modelled too. Specific CE stages such as repair, recycling and reuse are modelled as post-use activities related to reverse logistics services. Based on this taxonomy and with a set of competency questions, the authors have proposed an ontology. Discussion about the possible reuse and extension of the Good Relations [54] ontology is present as well. Although, the ontology’s implementation is set as future work, the current taxonomy presents the minimum information that is needed for modelling generic product passports. It can be used as a base ontology that can be extended for more complex use cases.
4.3.3.Circular exchange ontology (CEO) and the circular materials and activities ontology (CAMO)[98]
Following their previous work in [99], Sauter et al. [98] extend the existing CEO ontology and propose the CAMO ontology. Similarly to [74], the NeOn methodology is followed. The CEO ontology, which models agents, activities and referents involved in CE processes has been extended with new concepts (e.g. post-use, reverse logistics, product, resource) that help specify the processes in detail. The updated ontology has been later used for building specific product passports. The CAMO ontology, on the other hand, is modular and focuses on classifying different materials, products and activities [98]. The Place Reference Theory (PRT) has been reused and extended by CEO to model in detail agent and their CE actions. After RDF serialisation, both CEO and CAMO have been used to annotate a small dataset from the Madaster5555 as a proof of concept. The evaluation of the ontologies have concluded that Linked Data can successfully support data exchange and data traceability in the CE. However, validation on a wider scale with larger dataset is needed as suggested by the authors. Currently, both CEO and CAMO are no longer accessible, which is a challenge for their reuse and further development by the scientific community.
4.3.4.CE indicators taxonomy by Saidani et al. [97]
Led by the lack of standardisation for the adoption of the CE, Saidani et al. present a set of indicators (in the form of a taxonomy) for the evaluation of its performance. The concept categories that the authors focus on are CE loops, CE implementation, performance and perspective of circularity. For example, the CE loop represents the life-cycle (i.e. maintain, reuse, remanufacture, recycle stages) of a product within the CE, while the CE implementation focuses on the CE level (micro, meso, macro) of its implementation. Several groups of indicators have been defined as well-descriptive, performance, efficiency, policy effectiveness and total well-fare. The proposed indicators have been selected by following specific, measurable, achievable, relevant and time-Bound (SMART) [82] and clear, relevant, economic, adequate, monitorable (CREAM) mnemonics and have been utilised in the macro-based open-access Microsoft Excel5656 tool - the Circularity Indicators Advisor (CIA).5757 However, no efforts have been made yet to translate the taxonomy into a machine-readable format (e.g. RDF).
4.3.5.CE core ontology network (CEON) by Blomqvist et al. [16]
Following a modular approach Blomqvist et al. [16] present the CEON5858 ontology network that represent and interlinks products, materials, processes, created value and actors in CE settings. A module has been defined for each one of these topics. For example, the Actor module represents different roles entities can have such as recycler, remanufacturer. Specific processes related to products such as assembly, refurbishemnt, repair, production (some of which are well known CE strategies for lifetime extension) have been represented as well. In contrast the module representing materials5959 used for/in each product is more generic (specific types of materials such as metals, ceramics, plastics have not been defined). In a similar manner, the product module represents semantically the concepts of a product and a product component. Although the authors have not reused existing ontologies when defining the main modules and concepts in CEON the ontology network has been documented following best practices, which supports its further utilisation, reuse and extension. Further, the authors present examples of CEON’s application and connection with other ontologies (e.g. with PROV [76] and QUDT Units6060) in the construction and textile domains to validate their modular approach.
4.3.6.CE model for supplier selection (SS) by Echefaj et al. [37]
Another recent work is the CE ontology presented in [37], which is aimed at assisting organisations and their staff (e.g. managers) in selecting most optimal suppliers in terms of several factors such as sustainability. To help realise this, the authors have focused on semantically representing five categories of criteria, namely economic, environmental, social, resilience and circular, that can be used to evaluate potential suppliers. Examples of criteria have been defined for each category as well. For instance, circularity criteria include CE awareness and training, CE practices such as repair, refurbishment, recycling, reuse, reduce, while the economic criteria include the cost of a service, type of payments, reputation, warranty, transit time etc. Minimal evaluation of the ontology has been performed with the HermiT reasoner and OOPS!. However, the ontology is not openly available and has not been yet utilised in a real-world setting to truly evaluate its usefulness for decision making.
4.3.7.Summary
Similarly to the analysis in Section 4.1.12, in this section we summarise the findings from the literature review of existing CE semantic models (see Table 4). Our survey has shown that there is a limited number of studies available. Most of them present taxonomies based on specific use case analysis and standards. The provided CE terminology and process information from [24] and [97] can be used to define an initial set of competency questions for ontology engineers. We were able to identify 3 ontologies for the CE (see [16,37,98]). The work in [98], specifically the extended CEO and proposed CAMO ontologies, provide a generic data model down to material level. However, specific types of products and materials, which can be critical have not been modelled. The CEO and CAMO ontologies are no longer accessible, which is a barrier to their current reuse and possible extension with ICT ontologies such as [17,28,29] and material ontologies such as [5,114,119]. The CEON ontology network, on the other hand, is publicly available and provides a high-level coverage of a product’s lifetime in the CE. CEON can be extended with more domain-specific ontologies as well for capturing the lifetime of specific ICT devices such as laptops and their materials in the CE.
Table 4
Overview of CE semantic models
Study | Type | Year of Latest Update | Semantic Web Standard | Scope | Reuse of Existing Ontologies | Availability | Limitations | Applications |
Chiaroni et al. [24] | Taxonomy | 2016 | – | CE, its adoption and evaluation. | – | Private | Focused on e-commerce business models and processes and not on products. Validated with limited number of explorative studies. Implementing the taxonomy into an ontology or its combination with technology has not been discussed. | Used as a framework to evaluate CE’s adoption and specify different modes of CE’s adoption. |
Sauter and Witjes [99] | Taxonomy | 2017 | – | CE in the retail sector. | – | Private | Limited focus on textiles as a product in the CE. Early stage proof of concept taxonomy build based on one of CE’s activities (recycling) as a main use case. | To represent the life-cycle of textiles in the CE. Sets the foundation for a DPPs ontology for textiles in retail. |
Sauter et al. [98] | Ontology | 2019 | OWL | CE and CE processes for construction sector. | PTR | No longer accessiblea | Proof of concept ontology specification. Wide scale validation with data and real-world use cases is needed. | Aligning of product descriptions for the construction sector. Proposed to be used as a standard model for publishing CE data for buildings in decentralised settings. |
Saidani et al. [97] | Taxonomy | 2019 | – | CE and CE indicators. | – | Private | Products in the CE have not been considered as the main focus is on defining CE indicators. | Utilised as a database within the C-Indicators Advisorbtool to guide users’ selection of indicators. |
Blomqvist et al. [16] | Ontology | 2023 | OWL | Products in the CE | – | Public | General representation of the CE and products in it with limited examples of specific types of products and materials. | Built to support CE cross-domain data interoperability. Examples of utilisation in the textile and construction domain. |
Echefaj et al. [37] | Ontology | 2023 | OWL | Supplier selection indicators (circular, economic, environmental etc.) | – | Private | Limited evaluation in terms of ontology engineering. Real-world applications showcasing the usefulness of the ontology are unknown. Limited coverage of criteria dimensions. | To support the (manual and automated) selection of suppliers based on pre-defined sustainability, resilience and circularity criteria. |
a Online resources (http://ld-ce.com/vocab/CAMO) and http://ld-ce.com/vocab/CEO) not accessible since May 11th 2023.
4.4.Ontology utilisation for the CE
This section presents an overview of software tools that utilise ontologies to aid the further adoption of the CE. Common applications include building DPPs, supporting CE decision making in the IoT domain and recommender systems.
4.4.1.Laptop recommender system by Ayundhita [6]
Motivated by the lack of knowledge about the technical specifications (e.g. hard drive capacity, processor types) of hardware such as laptops and based on their previous research in the field (see [9–11]), Ayundhita [6] propose an ontology-based conversational recomender system (CRS) that aims to support end users in buying laptops. The CRS promts users with questions about the desired random access memory (RAM), processor, camera and makes recomendations to the user. Users can also give ratings for each recommendation, which helps optimise and improve the system with regards to the quality of the recommendations. To generate the questions, the system utilises an ontology that models laptops’ functional requirements and product specifications such as RAM. The system was evaluated with regards to its performance and user satisfaction. The analysis showed that the ontology-based CRSM achieved both better recomendation accuracy (84.6%) and higher user satisfaction in comparison to general e-commerce systems. Although an ontology was sucessfully utilised, it is not openly available and implementation details about it’s specific use are not provided in [6].
4.4.2.SmartTags IoT product passport for the CE by Gligoric et al. [47]
To support the transition from linear to CE, Glicoric et al. propose a method for building DPPs based on the combination of physical components (i.e. barcodes printed with functional ink) and software. On the software side, the authors propose a modular ontology for the CE. The ontology comprises of several sub-ontologies that model virtual entities, smart tags, users, services and sensor observations made by each tag. The work in [47] focus primarily on the development of the tags with thermochromic and photochromic ink and on the description of the ontologies. From a technology perspective, it is unclear how exactly the ontologies were utilised and how the product passport was built. However, the proposed work is one of the few on the topic and justifies the advantages of using ontologies in the CE.
4.4.3.IoT-enabled decision support system (DSS) for the CE by Mboli et al. [83]
A recent work, which has set as one of its main goals to raise awareness about the CE and assist its implementation within industry, is the ontology-driven IoT decision support system (DSS) by Mboli et al. [83]. The authors present a novel approach for supporting circularity decision making by combining the semantic representation of all CE-related processes, forward and backward logistics and rule-based reasoning. With the help of the ontology, each IoT component (also referred to as product) can be associated with different stages of the CE based on its usecycle and life-cycle. For example, the DSS uses rules such as “if the usecycle is low and life-cycle is very high, recommendation will be direct reuse” [83] have been defined with the ROWL [43] rule language in OWL. Implementation details regarding the DSS system and the utilisation of semantics have not been presented. However, the proposed approach has been evaluated with three scenarios focused on the status quo in linear economies, the reuse and the remanufacture CE stages. The results have justified the soundness of the proposed approach for a DSS and the use of an ontology to support data interoperability within it.
4.4.4.Summary
Although several ontologies for the CE have been built, there is limited work on their utilisation. The existing work briefly discusses their development and use but does not provide specific implementation details on exactly how the ontologies were utilised. It’s unclear if they were used just as a schema and guidelines or actually integrated (and how) within the systems. Most of the work presents approaches and their prototype implementation. To conclude, our survey into the field has confirmed that “the work on ontology for the CE is under-researched and there are only a few studies on this topic” [47].
5.Analysis
Ontology reuse is one of the recommended practices in ontology engineering that many follow. Our overview of the related work has shown that currently there is no unified consistent model that can represent the life-cycle of ICT and its materials in the CE. In order to build such (interdisciplinary) ontology, reuse can be a key strategy. To support this, we have evaluated each one of the open-access ontologies from Section 4 against the competency questions from Table 8.
The analysis was carried out manually by an experienced ontology engineer. The results were validated by all authors. Each publicly available ontology was downloaded and explored in Protégé. We also investigated the OWL encoding of the ontology itself with OOPS!. Online documentations often generated with WIDOCO and the scientific publications accompanying the ontology were also considered. Each ontology was investigated in terms of its ability to represent information (i.e. the key concepts) needed to answer each competency question. When a key concept was found it was listed as defined by the ontology to ease its future reuse. However, some concepts can be used interchangeably or as synonyms (depending on the domain). For example, in the CE experts think of product’s lifetime and view many things such as ICT devices as products. In such cases (e.g. with CEON [16]), we have noted down the namespace of the higher level or synonym concepts.
As expected, the ICT ontologies can answer most of the questions focused on ICT devices and their components, while the materials ontologies can answer the questions about materials. There is a clear domain knowledge separation. This might not be an issue for domain-specific research, but is a barrier for cross-domain collaboration (e.g. circular ICT). Table 5, 6 and 7 present each ontology from its corresponding domain, the competency questions that it can answer and the relevant concepts and object properties that can be reused.
Table 5
ICT ontology evaluation with the competency questions
Ontology | Which competency questions can be answered? | Relevant Concepts and Object Properties |
oneM2M Base Ontology [110] | Q1, Q10 | base_ontology:Device, base_ontology:InterworkedDevice, base_ontology:consistsOf, base_ontology:isPartOf |
DogOnt by Bonino and Rusiss [17] | Q1, Q33 | dogont:Appliances, dogont:WhiteGoods, dogont:BrownGoods, dogont:Computer, dogont:Sensor, dogont:Co2Sensor, dogont:HumiditySensor, dogont:TemperatureSensor, dogont:isSensorOf |
Corcho et al. [28] and Corcho et al. [29] | Q1, Q8, Q12 | devops-infra:hardwareType, devopsnet:HardwareItem, devopsnet:HardwareBatch, devopsnet:F5Hardware, devopsnet:Disk, devopsnet:unitPrice, devopsnet:serialNumber, devopsnet:highAvailabilityStatus, devopsprod:PhysicalServer, devopsprod:Server |
Yowe and Astawa [118] | Q1, Q2, Q10, Q11, Q13, Q22, Q23, Q30, Q34, Q35, Q41, Q56, Q57 | uni:Unit_Name, uni:Laptop, uni:Brand, uni:HasBrand, uni:HasOperatingSystem, uni:OperatingSystem, uni:Processor, uni:Screen, uni:Storage, uni:Baterei (i.e. Battery), uni:GPU, uni:GraphicsCard, uni:RAM, unit:Weight, unit:HasDesign_Weight, unit:HasWeight, uni:HasStorage_Storage_Type, uni:HasStorage_Storage_Size, uni:Price, uni:HasPrice |
Table 6
Materials ontology evaluation with the competency questions
Ontology | Competency Questions | Concepts |
Cheung et al. [20] | Q46 | matonto:Material, matonto:Ceramics, matonto:Polymers, matonto:Glasses, matonto:Composites, matonto:Metals, matonto:Structure, matonto:ChemicalQuality, matonto:formula |
Hastings et al. [53] | Q10, Q45, Q46, Q47 | ncitname:Name, bfo:materialEntity, chebi_ontology:chemicalsubstance, chebi_ontology:mixture, chebi_ontology:PureSubstance, envo:EnvironmentalMaterial, envo:OrganicMaterial, envo:MetallicMaterial, envo:Plastic, envo:Resin, quality:Mass, enanomapper:has_component_part, enanomapper:has_part |
EMMO [27] | Q47, Q55 | emmo_material:NanoMaterial, emmo_material:NaturalMaterial, emmo_material:EngineeredMaterial, emmo_manufacturing:ContinuumManufacturing, emmo_manufacturing:DiscreteManufacturing |
BIMMER [109] | Q45, Q46, Q47, | mat:Material, mat:MaterialProfile, mat:MaterialConstituent, mat:MaterialConstituentSet, mat:Measurement, mat:MaterialLayer, mat:hasMaterial |
Voigt et al.a[114] | Q45, Q46 | ex:Material, ex:Process, ex:composed_of, ex:next_in_process, ex:used_in |
Piane et al. [93] | Q45, Q46 | mambo:Material, mambo:atom, mambo_is_partOf, mambo:hasStructure, mambo:formula, mambo:is_part_of, mambo:formula |
Lambrix et al. [74] | Q45, Q46 | base: relatesToMaterial, base: relatesToStructure, structure:Composition, structure:hasElement, structure:hasComposition, structure:ElementRatio |
a The ontology does not have a namespace. For readability purposes, we have assigned the namespace “ex”.
Table 7
CE ontology evaluation with the competency questions
Ontology | Competency Questions | Concepts |
Sauter et al. [98] | Q45, Q46, Q51, Q59 | camo:biological, camo:technological, camo:alloy, camo:metal, ceo:Activity, ceo:Creation, ceo:PostUse, ceo: ReverseLogistics |
Blomqvist et al. [16] | Q4, Q6, Q7, Q10, Q42, Q43, Q44, Q45, Q53, Q56, Q57 | ceon:Product, ceon:AssemblingProcess, ceon:occursInLocation, ceon:owner, ceon:provider, ceon:ProductComponent, ceon:Energy, ceon:needsEnergy, ceon:CO2Emission, ceon:producesCO2, ceon:Material, ceon:MaterialComponent, ceon:ChemicalEntity, ceon:ChemicalSubstance, ceon:RecycleProcesses, ceon:RefurbishmentProcesses, ceon:RepairProcesses, ceon:ReuseProcesses, ceon:Value, ceon:ValueProposition |
Echefaj et al.a[37] | Q38, Q46, Q53, Q56, Q57, Q58, Q59 | ss:Waranty, ss:Price, ss:Cost, ss:Discount, ss:Is_A, ss:RecyclableMaterial, ss:Recycle, ss:Reduce, ss:Recover, ss:Refurbish, ss:Reuse, ss:Remanufacture, ss:Repair, ss:Repurpose, ss:Rethink, ss:IsStrategyOf, ss:Capacity, ss:CrisisStock |
a Unknown namespace thus we have assigned “ss” (short for supplier selection).
When evaluating the existing ontologies with the competency questions, the main challenges we encountered were the lack of public access and standard documentation to them. The evaluation was performed by examining either the ontology’s online documentation or its source file when a documentation was not available. Most of the ontologies were built to support specific software functionalities and no ontological evaluation in terms of quality (with HermiT [48], OOPS! [94]) was performed within the associated scientific publications. Discussion and guidelines for reuse for other use cases are, usually, not provided. The ontologies were evaluated through their successful software utilisation and use case-specific expressivity (the granularity of the represented knowledge). The common pitfalls that we encountered when analysing them with OOPS! include: missing annotations of classes and properties, missing inverse properties, inconsistent naming conventions, no specification of object property’s characteristics (e.g. if the property is functional, symmetric, asymmetric, transitive), missing domain and range of object and data properties between and of classes. In addition, most of the publicly available ontologies have not been documented using standard tools such as WIDOCO6161 [45], which is good practice for ontology publishing.
The ICT ontologies in Table 5 can answer several of the competency questions regarding a device’s hardware components (e.g. sensors) and processing. For example, DogOnt [17] represents the concept of a computer, which is relevant for our use case (i.e. laptops). Several types of sensors such as CO2, have been represented as well. Both [28] and [29] represent ICT hardware components such as a hard disk and server, which can be reused to extend our use case. The laptop ontology by Yowe and Astawa [118] can answer significantly more questions focused on the device itself and its components. However, it is not publicly available and has only been briefly described in its accompanying publication, which can be seen a disadvantage and main barrier to its wider reuse by the community.
The materials ontologies (see Table 6), although varying in expressivity, are generic enough to be reused or extended for our use case. The ontologies in [20,93,109,114] represent the concept of a material, while [27,53] and [74] can be reused to extend them with specific types of materials (e.g. natural, engineered, organic, plastic, resin, metallic) and their composition. However, none of the ontologies provides information about the criticality of the materials, which is an economic indicator in our case.
In the CE domain, the ontologies in [16,98] and [37] can be noted. The work of Sauter et al. [98] has a limited expressivity in terms of the modelled ontological concepts (see Table 7). However, by representing several generic types of materials and CE activities, it successfully connects the materials and CE domains. The ontology can be seen as a starting point for an ICT and materials ontology for the CE and can be extended to represent different hardware by reusing concepts from the existing ICT ontologies from Table 5. The CEON [16] ontology network is by far the most advanced ontology that allows one to interlink concepts from several domains needed to capture a product’s lifetime in the CE. However, CEON captures this knowledge at a high-level. It can be used as a top-level ontology, which can be extended with more specific product categories (e.g. brown, white goods from DogOnt [17]), types of materials from MatOnt [87] and CAMO [98]. Last but not least, the ontology by Echefaj et al. [37] provides an extensive list of indicators/criteria for selecting suppliers. However, the restricted access to it limits its reuse as an independent tool for decision making or as an extension for the above-mentioned mentioned ontologies.
6.Discussion
Following the ontology analysis, this section presents several discussion points on ontology engineering, availability and on data accessibility, privacy and security. During our survey, these were highlighted as important factors for the successful implementation of the CE with semantics.
6.1.Ontology reuse and alignment
To interlink the ICT, materials and CE domains, the existing ontologies can be aligned (e.g with an upper-level ontology) or can be reused separately (e.g. reuse of specific classes, object and data properties or extension with missing concepts). Aligning the ontologies requires an expert to monitor the quality of the alignment as duplicate concepts, inconsistencies in labelling and lack of background knowledge can occur [57,101]. The reuse of a specific ontology also requires an ontology engineer working closely with domain experts to derive use case requirements and select the most suitable ontology for reuse (from each domain) as each varies in its level of granularity and scope. Although this survey focused on laptops (as an example of ICT), their materials and life-cycle in the CE, we believe that the use case can be a good starting point in bridging the gap between the domains. Many ICT devices such as laptops and data servers have multiple hardware components and materials in common (e.g. hard drives, central processing units (CPUs), and power supply). Following a modular ontology engineering approach such [100], using the competency questions (Table 8) and analysis (Table 5, 6, 7) as guidelines, paves the way towards an ontology that can harmonise the domains.
6.2.Ontology availability
Current challenge to the reuse of the existing ontologies is the lack of online documentation and public availability. Many of the publications that present ontologies outline their structure in a generic way that does not support reuse. Including specific namespaces and URIs of classes, their object and data properties in the publications that outline the ontologies can be a minimum viable solution. If an ontology cannot be made public due to institutional ownership rights or legal concerns, it should be clearly stated in its scientific publication. Specific creative commons (CC)6262 licences (e.g. CC-BY, CC-BY-SA, CC BY-NC) can also be applied to ontologies to protect their ownership and to specify in what cases and how they can be shared, reused and adapted. Another possibility in such cases is that access to the ontology itself can be granted upon request as well. Finally, an ontology’s availability should be considered as an important criteria when reviewing scientific publications that present ontologies as their main contributions.
6.3.Data accessibility
Despite the benefits of having FAIR data in the CE, data accessibility for external to an organisation entities (e.g. researchers, third-party party service providers) remains an obstacle. Many companies are reluctant to freely share their data due to various reasons such as market competition and security. The accessibility to data is also affected by the organisation’s internal digital IT infrastructure and the types of database used (e.g relational or graph). Data is spread between different departments and databases. The access to it requires specific access rights even within organisation. Even when such access is granted, federating data from different relational databases can be a cumbersome time-consuming task. Linked data and semantics can help in this regard as discussed in the introduction (Section 1). Data licensing and contract-based subscriptions to it can be a solution that facilitates external access to it. An example of a technology solution that supports this is the Data Licenses Clearance Center (DALICC)6363 [92]. Last but not least, legislation itself can have a significant impact on organisations’s motivation to make such data available for reuse and analysis especially for data sharing and processing that support sustainability.
6.4.Data privacy and security
ICT data sharing for the CE also raises privacy concerns as any data that can be related back to an individual (e.g laptop’s usage behaviour and performance over time) is considered personal under the General Data Protection Regulation (GDPR) [96] (Art. 4(1)). Such data should be protected and processed in a GDPR-compliant manner. Establishing privacy preserving mechanisms through the implementation of specific privacy enhancing technologies (PETs) [49] can be a solution that enables (sensitive) data to be shared when building DPPs in both business to business (B2B) and business to consumer (B2C) use cases. In the case that ICT DPPs have been implemented and are actively used, ontologies can be used to defining specific agreed upon data access and usage rights (e.g. policies) as discussed in [38,69]. This will enable different levels of DPP data transparency and accessibility to support the growth of the CE.
7.Conclusions
This paper presented a survey of existing semantic models in the ICT, materials and CE domains. While there is a variety of such models, they have been built for use cases within their domain and rarely connect to knowledge from other domains. Many of the existing models remain taxonomies thus their full potential, from a semantic and technological perspective, has not been realised yet. The surveyed ICT ontologies model specific hardware and rarely reach hardware’s material composition level, while the materials ontologies focus on the materials themselves (their discoverability, chemical properties, compatibility, reactions). There is a clear partition of the domains, which are, however, significantly interrelated within the CE domain. The recent survey by Li et al. [77] further looks into the topic of general cross-domain ontologies for the CE. The authors’ work confirms our findings that the lack of data interoperability and unified agreed upon vocabulary for products (in our case ICT devices) in the CE are some of the key challenges limiting the further adoption of the CE and that ontologies can aid this.
A current limitation of our work is that it analyses mainly ontologies that have been published as scientific publications and reports and/or have been publicly documented. We acknowledge that ontology engineering is a dynamic iterative process and we envision that more work will be done in the field as CE standardisation and legislation become more prominent. In spite of this, we believe that this survey can be useful to both sustainability domain experts (e.g. industrial ecologists) and ontology engineers. For sustainability experts, our work is a source of information on how the field of the Semantic Web can provide technology such as ontologies that can be used to advance the implementation of the CE. For instance, Ghose et al. [46] present an upper level ontology that models data needed for LCAs. Insights form our survey can be used in the future to extended the work in [46] with specific ICT, materials and CE concepts. Doing so will allow one to semantically representing detailed ICT data for LCAs, which can potentially result in more precise and insightful results. Ontology engineers, on the other hand, can benefit from the systematic analysis of existing work in the domain, which aims to support and ease ontology reuse and the implementation of FAIR ICT data sharing for the CE. The digitization of CE processes such as maintenance (or predictive maintenance) that support service optimisation at scale is highly dependent on data’s availability and interoperability. Reusing and further sharing insights from such processes in a consistent machine-readable format can help optimise production and manufacturing supply chains.
Current standardisation efforts are leading to the development of DPPs, which aim to bring more transparency of products’ lifetime provenance in terms of its manufacturing, materials and their sources, use etc. DPPs, which as we discuss in [71] can be represented with Semantic Web technology (e.g. ontologies and knowledge graphs) to store diverse data about ICT such as functional specifications, manufacturing and materials details and more dynamic data such as performance (e.g. energy consumption) over time. Knowledge graph-based DPPs as a technology solution can help establish better transparency and traceability into ICT supply chains by making data about material mining, ICT device manufacturing and its use accessible, reusable and interoperable. Building such digital infrastructures that support FAIR principles can boost the transition to a CE and can help cultivate a more sustainable digital economy driven by data reuse.
Notes
4 https://amsterdameconomicboard.com/en/news/circular-ict-procurement-is-to-drastically-reduce-waste/
Acknowledgements
This work is supported by the Circular Resource Planning for IT (RePlanIT) project, which is funded by a Topsector Energy subsidy from the Ministry of Economic Affairs and Climate Policy in the Netherlands. We would like to express our gratitude to the whole project consortium (Aliter Networks, Ideal&Co, WCooliT, Amsterdam Institute for Advanced Metropolitan Solutions (AMS), KPN, GreenIT Amsterdam Foundation, Amsterdam Economic Board Foundation, Municipality of Amsterdam and Rijkswaterstaat) that helped shape and motivate our work. Further, we thank Ingrid de Pauw and Joppe van Driel for the constructive feedback.
Appendices
Appendix
A set of competency questions for building an ontology that represents ICT devices such as laptops and their materials in the CE. The questions have been organised in six categories: ICT device, its components, physical, sustainability, material properties and CE strategy. For each question, examples of key concepts that an ontology should represent, have been provided. The first category questions help to represent an ICT device and its components in generalised way. The second category presents component specific questions. The physical properties of ICT such as overall weight, component weight, warranty etc. are usually assigned by the device’s manufacturer or refurbisher. The sustainability properties relate to the environmental impact of the ICT device and its components. The material properties questions are aimed at an ICT device’s material composition. We present material questions at a device component level. However, when such information for an ICT device is available for all of its components, the overall material composition of the device can be derived as well. The final category questions help represent CE processes for a specific device and/or its component(s) and the monetary value of a device (or component) before or after a CE strategy has been carried out.
Table 8
Competency questions for an ICT, materials and CE domains ontology
No | Question | Key Concepts |
1. ICT Device | ||
Q1 | What is the type of the ICT device? | Device, laptop |
Q2 | What is the brand of the device? | Device, brand |
Q3 | What is the brand model of the device? | Device, brand, model |
Q4 | Where was the device assembled? | Device, location, country, state, region, city |
Q5 | When was the device assembled? | Device, assembly date, year, date, time |
Q6 | Who owns the device? (Refers to the agent that has ownership rights over the device.) | Device owner, organisation, person |
Q7 | Who provides the hardware? (Refers to the agent that provides hardware components needed for repair, refurbishment etc.) | Device provider, organisation, person |
Q8 | What is the current status of the device? (Has it been reused, remanufactured, refurbished, recycled or is it new?) | Device, status, reused, remanufactured, refurbished, recycled, new |
Q9 | What is the device’s grade after refurbishment, repair, remanufacturing? | Grade, pristine, damaged, damage level, damage type |
Q10 | What are the components of a device? | Device, components, hardware, software |
Q11 | What is the device’s operating system? | Device, operating system |
2. ICT Device Components | ||
Q12 | What is the serial number of the component? | Device component, component serial number |
Q13 | What is the brand of the component? | Component, brand |
Q14 | What is the brand model of the component? | Component, brand, model |
Q15 | What is the type of the component in terms of its location within a device? | Device, component, location, peripheral (i.e. external), integrated |
Q16 | What is the status of the device’s component? Has it been reused, remanufactured, or refurbished before? | Component status, refurbished, remanufactured, repaired, reused |
Q17 | Why was the component reused, remanufactured, repaired or refurbished before? | Component, circular strategy, repair, remanufacture, refurbishment, reason |
Q18 | What is the current age of the component? | Component age, year, month, day |
Q19 | What type of network card is used? | Network card, type, brand, model |
Q20 | What type of chipset is used? | Chipset, type, brand, model |
Q21 | What type of expansion slots are used? | Expansion slot, type, brand, model |
Q22 | What type of storage is used? | Storage, type, brand, model |
Q23 | What is the display type? | Display, type |
Q24 | What is the display’s resolution? | Display, resolution |
Q25 | What is the device’s screen-to-body ratio? | Device, screen-to-body ratio |
Q26 | What type of sound card is used? | Audio, sound card, type |
Q27 | What type of keyboard is used? | Keyboard, type |
Q28 | What type of pointing device is used? | Pointing device, type |
Q29 | What type of wireless technology is used? | Wifi technology |
Q30 | What type of battery is used? (e.g. alkaline, NiCad, Li-Ion) | Battery, type |
Q31 | What types of ports are used? (e.g. USB-A, USB-C) | Port, type |
Q32 | What type of camera is used? | Camera, type, brand, model |
Q33 | What types of sensors are used? | Sensors, sensor types |
Table 8
(Continued)
No | Question | Key Concepts |
3. ICT Physical Properties | ||
Q34 | What is the weight of a device? | Device, weight |
Q35 | What is the weight of a device’s component? | Component, weight |
Q36 | What is the current age of a device? | Device, age, year, month, day |
Q37 | What is the current age of a device’s component? | Component, age, year, month, day |
Q38 | What is the duration of the device’s warranty? | Device, warranty, type, duration |
Q39 | What type of damage does the device’s warranty cover? | Warranty, coverage, damage type |
Q40 | What is the duration of the device component’s warranty? | Device, component, warranty, type, duration |
Q41 | What is the memory capacity of the device? | Memory capacity (e.g. in Megabytes, Gygabytes) |
4. ICT Sustainability Properties | ||
Q42 | What is the energy consumption of the device? | Device, energy consumption in Watthour (Wh), kilo watthour (kWh) |
Q43 | What is the device’s energy efficiency? | Device, Energy efficiency grade/rating (A-G) |
Q44 | What is the device’s carbon dioxide (CO2) footprint? (During the production and/or use phase) | Device, carbon dioxide (CO2) footprint in kg per year |
5. ICT Material Properties | ||
Q45 | What material is used? | Material, name, chemical formula |
Q46 | What is the type of the used material? | Material, type (e.g. metal, composite, polymer, ceramics) |
Q47 | How much material (in grams) is present in the component? | Material, component, availability, weight |
Q48 | What is the criticality of the material? | Material, status, critical, criticality level |
Q49 | What is the cost of the material? E.g. 1g of gold is around 54.346 Euro | Material, material cost, weight |
6. CE Strategy | ||
Q50 | What CE strategy is recommended for the specific device? | Device, CE strategy, recommendation |
Q51 | What CE strategy is recommended for the specific device’s component? | Device component, CE strategy, recommendation |
Q52 | Why is the specific CE strategy recommended? | Recommended CE strategy, reason |
Q53 | What CE strategy is selected? | CE strategy, refurbish, remanufacture, repair, share, reuse, recycle |
Q54 | Why is the specific CE strategy used? | Selected CE strategy, reason |
Q55 | How many times has as a CE strategy been performed? | Device, component, CE strategy, number of times performed |
Q56 | What is the (monetary) value of the device before and after a CE strategy is used? | Device, manufacturer’s monetary value, monetary value after repair, refurbishment, remanufacturing |
Q57 | What is the (monetary) value of the component before and after a CE strategy is used? | Component, manufacturer’s monetary value, monetary value after repair, refurbishment, remanufacturing |
Q58 | What is the stock availability of the device? | Number of devices in stock, purchase availability |
Q59 | What is the stock availability of the component? | Number of device components in stock, purchase availability |
References
[1] | M. Adrion and J. Woidasky, Planned obsolescence in portable computers-empirical research results, in: Cascade Use in Technologies 2018: Internationale Konferenz zur Kaskadennutzung und Kreislaufwirtschaft–Oldenburg 2018, Springer, (2019) , pp. 13–20. doi:10.1007/978-3-662-57886-5_3. |
[2] | J.M. Allwood, Squaring the circular economy: The role of recycling within a hierarchy of material management strategies, in: Handbook of Recycling, Elsevier, (2014) , pp. 445–477. doi:10.1016/B978-0-12-396459-5.00030-1. |
[3] | C.W. Andersen, R. Armiento, E. Blokhin, G.J. Conduit, S. Dwaraknath, M.L. Evans, Á. Fekete, A. Gopakumar, S. Gražulis, A. Merkys et al., OPTIMADE, an API for exchanging materials data, Scientific data 8: (1) ((2021) ), 1–10. doi:10.1038/s41597-020-00786-7. |
[4] | Apple Inc., Supplier list (2022), available at: https://www.apple.com/supplier-responsibility/pdf/Apple-FY21-Supplier-List.pdf. |
[5] | T. Ashino, Materials ontology: An infrastructure for exchanging materials information and knowledge, Data Science Journal 9: ((2010) ), 54–61. doi:10.2481/dsj.008-041. |
[6] | M. Ayundhita, Z. Baizal and Y. Sibaroni, Ontology-based conversational recommender system for recommending laptop, Journal of Physics: Conference Series, Vol. 1192: , IOP Publishing, (2019) , p. 012020. |
[7] | C.W. Babbitt, H. Madaka, S. Althaf, B. Kasulaitis and E.G. Ryen, Disassembly-based bill of materials data for consumer electronic products, Scientific Data 7: (1) ((2020) ), 1–8. doi:10.1038/s41597-020-0573-9. |
[8] | G. Bailey, P.J. Joyce, D. Schrijvers, R. Schulze, A.M. Sylvestre, B. Sprecher, E. Vahidi, W. Dewulf and K. Van Acker, Review and new life cycle assessment for rare Earth production from bastnäsite, ion adsorption clays and lateritic monazite, Resources, Conservation and Recycling 155: ((2020) ), 104675. doi:10.1016/j.resconrec.2019.104675. |
[9] | Z. Baizal, A. Iskandar and E. Nasution, Ontology-based recommendation involving consumer product reviews, in: 2016 4th International Conference on Information and Communication Technology (ICoICT), IEEE, (2016) , pp. 1–6. doi:10.1109/ICoICT.2016.7571890. |
[10] | Z.A. Baizal, Y.R. Murti et al., Evaluating functional requirements-based compound critiquing on conversational recommender system, in: 2017 5th International Conference on Information and Communication Technology (ICoIC7), IEEE, (2017) , pp. 1–6. doi:10.1109/ICoICT.2017.8074656. |
[11] | Z.A. Baizal, D.H. Widyantoro and N.U. Maulidevi, Design of knowledge for conversational recommender system based on product functional requirements, in: 2016 International Conference on Data and Software Engineering (ICoDSE), IEEE, (2016) , pp. 1–6. |
[12] | C. Bakker and R. Balkenende, Chapter 7 - A renewed recognition of the materiality of design in a circular economy: The case of bio-based plastics, in: Materials Experience 2, O. Pedgley, V. Rognoli and E. Karana, eds, Butterworth-Heinemann, (2021) , pp. 193–206. ISBN 978-0-12-819244-3. doi:10.1016/B978-0-12-819244-3.00020-X. |
[13] | C. Bakker, F. Wang, J. Huisman and M. Den Hollander, Products that go round: Exploring product life extension through design, Journal of Cleaner Production 69: ((2014) ), 10–16. doi:10.1016/j.jclepro.2014.01.028. |
[14] | R. Benjamins, D. Fensel and A. Gómez-Pérez, Knowledge management through ontologies, (1998) , CEUR Workshop Proceedings (CEUR-WS. Org). |
[15] | T. Berners-Lee, J. Hendler and O. Lassila, The Semantic Web, Scientific american 284: (5) ((2001) ), 34–43. |
[16] | E. Blomqvist, H. Li, R. Keskisärkkä, M. Lindecrantz, M.A.N. Pour, Y. Li and P. Lambrix, Cross-Domain Modelling – A Network of Core Ontologies for the Circular Economy, (2023) . |
[17] | D. Bonino and F. Corno, Dogont-ontology modeling for intelligent domotic environments, in: International Semantic Web Conference, Springer, (2008) , pp. 790–803. |
[18] | A. Breitfuss, K. Errou, A. Kurteva and A. Fensel, Representing emotions with knowledge graphs for movie recommendations, Future Generation Computer Systems 125: ((2021) ), 715–725. doi:10.1016/j.future.2021.06.001. |
[19] | W.D. Callister and D. Rethwisch Jr., Materials Science and Engineering, John Wiley and Sons, SBN, (2010) . |
[20] | K. Cheung, J. Drennan and J. Hunter, Towards an ontology for data-driven discovery of new materials, in: AAAI Spring Symposium: Semantic Scientific Knowledge Integration, (2008) , pp. 9–14. |
[21] | T.R. Chhetri, S. Aghaei, A. Fensel, U. Göhner, S. Gül-Ficici and J. Martinez-Gil, Optimising manufacturing process with Bayesian structure learning and knowledge graphs, in: Computer Aided Systems Theory – EUROCAST 2022, R. Moreno-Díaz, F. Pichler and A. Quesada-Arencibia, eds, Springer Nature, Switzerland, Cham, (2022) , pp. 594–602. ISBN 978-3-031-25312-6. doi:10.1007/978-3-031-25312-6_70. |
[22] | T.R. Chhetri, A. Kurteva, J.G. Adigun and A. Fensel, Knowledge graph based hard drive failure prediction, Sensors 22: (3) ((2022) ), 985. doi:10.3390/s22030985. |
[23] | T.R. Chhetri, A. Kurteva, R.J. DeLong, R. Hilscher, K. Korte and A. Fensel, Data protection by design tool for automated GDPR compliance verification based on semantically modeled informed consent, Sensors 22(7) ((2022) ). doi:10.3390/s22072763. |
[24] | D. Chiaroni and A. Urbinati, Circular economy business models: towards a new taxonomy of the degree of circularity, 27th Edition of the Annual Scientific Meeting of the Italian Association of Management Engineering (AiIG) ((2016) ). |
[25] | L.O. Colombo-Mendoza, R. Valencia-García, A. Rodríguez-González, G. Alor-Hernández and J.J. Samper-Zapater, RecomMetz: A context-aware knowledge-based mobile recommender system for movie showtimes, Expert Systems with Applications 42: (3) ((2015) ), 1202–1222. doi:10.1016/j.eswa.2014.09.016. |
[26] | M. Compton, P. Barnaghi, L. Bermudez, R. Garcia-Castro, O. Corcho, S. Cox, J. Graybeal, M. Hauswirth, C. Henson and A. Herzog, The SSN ontology of the W3C semantic sensor network incubator group, Journal of Web Semantics 17: ((2012) ), 25–32. doi:10.1016/j.websem.2012.05.003. |
[27] | E. Consortium, Elementary multiperspective material ontology (EMMO), (2021) , available at: https://github.com/emmo-repo/EMMO. |
[28] | O. Corcho, R. Alcazar, D.C.-F.J. Toledo, J. Arenas, M. Wang, H. Peng, N. Burrett, J. Mora and P. Zhang, Ontology for the representation of the hardware items related to a DevOps infrastructure, (2021) , available at: http://w3id.org/devops-infra/hardware. |
[29] | O. Corcho, D. Chaves-Fraga, J. Toledo, J. Arenas-Guerrero, C. Badenes-Olmedo, M. Wang, H. Peng, N. Burrett, J. Mora and P. Zhang, A high-level ontology network for ICT infrastructures, in: The Semantic Web – ISWC 2021, A. Hotho, E. Blomqvist, S. Dietze, A. Fokoue, Y. Ding, P. Barnaghi, A. Haller, M. Dragoni and H. Alani, eds, Springer International Publishing, Cham, (2021) , pp. 446–462. ISBN 978-3-030-88361-4. doi:10.1007/978-3-030-88361-4_26. |
[30] | L. Daniele, F.d. Hartog and J. Roes, Created in close interaction with the industry: The smart appliances reference (SAREF) ontology, in: International Workshop Formal Ontologies Meet Industries, Springer, (2015) , pp. 100–112. doi:10.1007/978-3-319-21545-7_9. |
[31] | A. Daouadji, K.-K. Nguyen, M. Lemay and M. Cheriet, Ontology-based resource description and discovery framework for low carbon grid networks, in: 2010 First IEEE International Conference on Smart Grid Communications, IEEE, (2010) , pp. 477–482. doi:10.1109/SMARTGRID.2010.5622090. |
[32] | P. de Matos, A. Dekker, M. Ennis, J. Hastings, K. Haug, S. Turner and C. Steinbeck, ChEBI: A chemistry ontology and database, Journal of cheminformatics 2: (1) ((2010) ), 1–1. doi:10.1186/1758-2946-2-1. |
[33] | E. Della Valle, S. Schlobach, M. Krötzsch, A. Bozzon, S. Ceri and I. Horrocks, Order matters! Harnessing a world of orderings for reasoning over massive data, Semantic Web 4: (2) ((2013) ), 219–231. doi:10.3233/SW-2012-0085. |
[34] | L. Deng, C.W. Babbitt and E.D. Williams, Economic-balance hybrid LCA extended with uncertainty analysis: Case study of a laptop computer, Journal of Cleaner Production 19: (11) ((2011) ), 1198–1206. doi:10.1016/j.jclepro.2011.03.004. |
[35] | V. Dhingra and K.K. Bhatia, Development of ontology in laptop domain for knowledge representation, Procedia Computer Science 46: ((2015) ), 249–256. doi:10.1016/j.procs.2015.02.018. |
[36] | M. Doerr, Ontologies for cultural heritage, in: Handbook on Ontologies, Springer, (2009) , pp. 463–486. doi:10.1007/978-3-540-92673-3_21. |
[37] | K. Echefaj, A. Charkaoui, A. Cherrafi, J.A. Garza-Reyes, S.A.R. Khan and A.C. Benabdellah, Sustainable and resilient supplier selection in the context of circular economy: An ontology-based model, Management of Environmental Quality: An International Journal 34: (5) ((2023) ), 1461–1489. doi:10.1108/MEQ-02-2023-0037. |
[38] | B. Esteves and V. Rodríguez-Doncel, Analysis of ontologies and policy languages to represent information flows in GDPR, Semantic Web ((2022) ), 1–35. |
[39] | K. Fatema, E. Hadziselimovic, H.J. Pandit, C. Debruyne, D. Lewis and D. O’Sullivan, Compliance through informed consent: semantic based consent permission and data management model, Privacy and the Semantic Web, policy and technology workshop (PrivOn 2017), Co-located with ISWC 2017 (2017). |
[40] | D. Fensel, Ontology-based knowledge management, Computer 35: (11) ((2002) ), 56–59. doi:10.1109/MC.2002.1046975. |
[41] | N. Freire and S.d. Valk, Automated interpretability of linked data ontologies: An evaluation within the cultural heritage domain, in: 2019 IEEE International Conference on Big Data (Big Data), (2019) , pp. 3072–3079. doi:10.1109/BigData47090.2019.9005491. |
[42] | C. Freitag, M. Berners-Lee, K. Widdicks, B. Knowles, G.S. Blair and A. Friday, The real climate and transformative impact of ICT: A critique of estimates, trends, and regulations, Patterns 2: (9) ((2021) ), 100340. doi:10.1016/j.patter.2021.100340. |
[43] | F. Gandon, M. Sheshagiri and N.M. Sadeh, ROWL: Rule language in OWL and translation engine for JESS, Mobile Commerce Laboratory ((2004) ). |
[44] | A. Gangemi, N. Guarino, C. Masolo, A. Oltramari and L. Schneider, Sweetening ontologies with DOLCE, in: International Conference on Knowledge Engineering and Knowledge Management, Springer, (2002) , pp. 166–181. doi:10.1007/3-540-45810-7_18. |
[45] | D. Garijo, WIDOCO: A wizard for documenting ontologies, in: The Semantic Web–ISWC 2017: 16th International Semantic Web Conference, Proceedings, Part II 16, Vienna, Austria, October 21–25, 2017, Springer, (2017) , pp. 94–102. doi:10.1007/978-3-319-68204-4_9. |
[46] | A. Ghose, M. Lissandrini, E.R. Hansen and B.P. Weidema, A core ontology for modeling life cycle sustainability assessment on the semantic web, Journal of Industrial Ecology 26: (3) ((2022) ), 731–747. doi:10.1111/jiec.13220. |
[47] | N. Gligoric, S. Krco, L. Hakola, K. Vehmas, S. De, K. Moessner, K. Jansson, I. Polenz and R. Van Kranenburg, Smarttags: IoT product passport for circular economy based on printed sensors and unique item-level identifiers, Sensors 19: (3) ((2019) ), 586. doi:10.3390/s19030586. |
[48] | B. Glimm, I. Horrocks, B. Motik, G. Stoilos and Z. Wang, HermiT: An OWL 2 reasoner, Journal of Automated Reasoning 53: ((2014) ), 245–269. doi:10.1007/s10817-014-9305-1. |
[49] | I. Goldberg, D. Wagner and E. Brewer, Privacy-enhancing technologies for the Internet, in: Proceedings IEEE COMPCON 97. Digest of Papers, IEEE, (1997) , pp. 103–109. doi:10.1109/CMPCON.1997.584680. |
[50] | V. Gower and R. Andrich, A taxonomy for ICT assistive technology products, Technology and Disability 26: (2–3) ((2014) ), 127–136. doi:10.3233/TAD-140409. |
[51] | T.R. Gruber and G.R. Olsen, An ontology for engineering mathematics, in: Principles of Knowledge Representation and Reasoning, Elsevier, (1994) , pp. 258–269. doi:10.1016/B978-1-4832-1452-8.50120-2. |
[52] | J. Harris, Planned obsolescence: The outrage of our electronic waste mountain’, The Guardian 15 ((2020) ). |
[53] | J. Hastings, N. Jeliazkova, G. Owen, G. Tsiliki, C.R. Munteanu, C. Steinbeck and E. Willighagen, eNanoMapper: Harnessing ontologies to enable data integration for nanomaterial risk assessment, Journal of biomedical semantics 6: (1) ((2015) ), 1–15. doi:10.1186/2041-1480-6-1. |
[54] | M. Hepp, Goodrelations: An ontology for describing products and services offers on the web, in: Knowledge Engineering: Practice and Patterns: 16th International Conference, EKAW 2008, Springer, Acitrezza, Italy, (2008) , pp. 329–346. |
[55] | R. Hischier, V.C. Coroama, D. Schien and M. Ahmadi Achachlouei, Grey energy and environmental impacts of ICT hardware, in: ICT Innovations for Sustainability, Springer, (2015) , pp. 171–189. doi:10.1007/978-3-319-09228-7_10. |
[56] | R. Hodgson, P.J. Keller, J. Hodges and J. Spivak, Qudt-quantities, units, dimensions and data types ontologies, USA Available http://qudt.org March 156 ((2014) ). |
[57] | L. Hollink, M. Van Assem, S. Wang, A. Isaac and G. Schreiber, Two variations on ontology alignment evaluation: Methodological issues, in: ESWC, (2008) , pp. 388–401. |
[58] | I. Horrocks, Semantic web: The story so far, in: Proceedings of the 2007 International Cross-Disciplinary Conference on Web Accessibility (W4A), W4A’07, Association for Computing Machinery, New York, NY, USA, (2007) , pp. 120–125. ISBN 1595935908. doi:10.1145/1243441.1243469. |
[59] | A.Z. Ihsan, D. Dessì, M. Alam, H. Sack and S. Sandfeld, Steps towards a dislocation ontology for crystalline materials, in: SeDiT 2021-Semantic Digital Twins 2021-Proceedings of the Second International Workshop on Semantic Digital Twins Co-Located with the 18th Extended Semantic Web Conference (ESWC 2021), Hersonissos, Greece, June 6, 2021, R. García-Castro, ed., (2021) . |
[60] | T. Inaba and M. Squicciarini, ICT: A new taxonomy based on the international patent classification, (2017) , OECD Science, Technology and Industry Working Papers. doi:10.1787/ab16c396-en. |
[61] | A. Jacobsen, R. Kaliyaperumal, L.O.B. da Silva Santos, B. Mons, E. Schultes, M. Roos and M. Thompson, A generic workflow for the data FAIRification process, Data Intelligence 2: (1–2) ((2020) ), 56–65. doi:10.1162/dint_a_00028. |
[62] | H. Jin, K. Frost, I. Sousa, H. Ghaderi, A. Bevan, M. Zakotnik and C. Handwerker, Life cycle assessment of emerging technologies on value recovery from hard disk drives, Resources, Conservation and Recycling 157: ((2020) ), 104781, https://www.sciencedirect.com/science/article/pii/S0921344920301026. doi:10.1016/j.resconrec.2020.104781. |
[63] | G. Kamiya, Data centres and data transmission networks, (2022) , available at: https://www.iea.org/reports/data-centres-and-data-transmission-networks. |
[64] | S.S. Karakutuk, S. Akpinar and M.A. Ornek, An application of a circular economy approach to design an energy-efficient heat recovery system, Journal of Cleaner Production 320: ((2021) ), 128851. doi:10.1016/j.jclepro.2021.128851. |
[65] | E. Kärle, U. Şimşek, O. Panasiuk and D. Fensel, Building an ecosystem for the Tyrolean tourism knowledge graph, in: International Conference on Web Engineering, Springer, (2018) , pp. 260–267. |
[66] | B.V. Kasulaitis, C.W. Babbitt, R. Kahhat, E. Williams and E.G. Ryen, Evolving materials, attributes, and functionality in consumer electronics: Case study of laptop computers, Resources, conservation and recycling 100: ((2015) ), 1–10. doi:10.1016/j.resconrec.2015.03.014. |
[67] | A. Khosravani, L. Morsdorf, C.C. Tasan and S.R. Kalidindi, Multiresolution mechanical characterization of hierarchical materials: Spherical nanoindentation on martensitic Fe-Ni-C steels, Acta Materialia 153: ((2018) ), 257–269. doi:10.1016/j.actamat.2018.04.063. |
[68] | J. Kirchherr, D. Reike and M. Hekkert, Conceptualizing the circular economy: An analysis of 114 definitions, Resources, conservation and recycling 127: ((2017) ), 221–232. doi:10.1016/j.resconrec.2017.09.005. |
[69] | S. Kirrane, J.D. Fernández, P. Bonatti, U. Milosevic, A. Polleres and R. Wenning, The SPECIAL-K personal data processing transparency and compliance platform, (2020) , arXiv preprint arXiv:2001.09461. |
[70] | L.L. Kjaer, A. Pagoropoulos, J.H. Schmidt and T.C. McAloone, Challenges when evaluating product/service-systems through life cycle assessment, Journal of Cleaner Production 120: ((2016) ), 95–104. doi:10.1016/j.jclepro.2016.01.048. |
[71] | A. Kurteva, Towards FAIR ICT data sharing in the circular economy with knowledge graphs, in: NWO ICT Open Conference, Utrecht, The Netherlands, (2023) , available at: https://www.researchgate.net/publication/370106721_Towards_FAIR_ICT_Data_Sharing_in_the_Circular_Economy_with_Knowledge_Graphs. |
[72] | A. Kurteva, T.R. Chhetri, H.J. Pandit and A. Fensel, Consent through the lens of semantics: State of the art survey and best practices, Semantic Web 15: (3) (647)–(673) ((2024) ). doi:10.3233/SW-210438. |
[73] | C. Lagoze and J. Hunter, The ABC ontology and model, Journal of Digital Information 2(2) ((2002) ). |
[74] | P. Lambrix, R. Armiento, H. Li, O. Hartig, M.A.N. Pour and Y. Li, The Materials Design Ontology, Transport 8(10), 24. |
[75] | F. Lampathaki, Y. Charalabidis, S. Passas, D. Osimo, M. Bicking, M.A. Wimmer and D. Askounis, Defining a taxonomy for research areas on ICT for governance and policy modelling, in: International Conference on Electronic Government, Springer, (2010) , pp. 61–72. doi:10.1007/978-3-642-14799-9_6. |
[76] | T. Lebo, S. Sahoo, D. McGuinness, K. Belhajjame, J. Cheney, D. Corsar, D. Garijo, S. Soiland-Reyes, S. Zednik and J. Zhao, in: PROV-O: The PROV Ontology, W3C Recommendation, World Wide Web Consortium, United States, (2013) . |
[77] | H. Li, M. Abd Nikooie Pour, Y. Li, M. Lindecrantz, E. Blomqvist and P. Lambrix, A survey of general ontologies for the cross-industry domain of circular economy, in: 2023 World Wide Web Conference, WWW 2023, Austin, Texas, USA, 30 April–4 May 2023, ACM, (2023) . |
[78] | H. Li, R. Armiento and P. Lambrix, An ontology for the materials design domain, in: International Semantic Web Conference, Springer, (2020) , pp. 212–227. doi:10.1007/978-3-030-62466-8_14. |
[79] | E. Long, S. Kokke, D. Lundie, N. Shaw, W. Ijomah and C.-c. Kao, Technical solutions to improve global sustainable management of waste electrical and electronic equipment (WEEE) in the EU and China, Journal of Remanufacturing 6: ((2016) ), 1–27. doi:10.1186/s13243-015-0023-6. |
[80] | O. Mamoutova, M. Uspenskiy, S. Smirnov and M. Bolsunovskaya, Ontological approach to automated analysis of enterprise data storage systems log files, Acta Polytechnica Hungarica 18: (9) ((2021) ), 27–47. doi:10.12700/APH.18.9.2021.9.3. |
[81] | O.V. Mamoutova, M.B. Uspenskiy, A.V. Sochnev, S.V. Smirnov and M.V. Bolsunovskaya, Knowledge based diagnostic approach for enterprise storage systems, in: 2019 IEEE 17th International Symposium on Intelligent Systems and Informatics (SISY), (2019) , pp. 207–212. doi:10.1109/SISY47553.2019.9111617. |
[82] | B. Marr, Big data: Using SMART big data, analytics and metrics to make better decisions and improve performance, John Wiley & Sons, (2015) . |
[83] | J.S. Mboli, D. Thakker and J.L. Mishra, An Internet of things-enabled decision support system for circular economy business model, Software: Practice and Experience 52: (3) ((2022) ), 772–787. |
[84] | K. McMahon, E.J. Hultink and R. Mugge, Identifying barriers and enablers for circular ICT practices: An exploratory study, PLATE: Product Lifetimes and the Environment, in: Product Lifetimes and the Environment, (PLATE) Conference, 2023, In Press. |
[85] | K. McMahon, R. Mugge and E.J. Hultink, Overcoming barriers to circularity for internal ICT management in organizations: A change management approach, Resources, Conservation and Recycling 205: ((2024) ), 107568. doi:10.1016/j.resconrec.2024.107568. |
[86] | A. Miles and J.R. Pérez-Agüera, Skos: Simple knowledge organisation for the web, Cataloging & Classification Quarterly 43: (3–4) ((2007) ), 69–83. doi:10.1300/J104v43n03_04. |
[87] | B. Miller, The MatOnto Domain Ontology for Materials Science, (2021) , available at: https://matportal.org/ontologies/MATONTO. |
[88] | N.F. Noy, D.L. McGuinness et al., Ontology development 101: A guide to creating your first ontology, Stanford knowledge systems laboratory technical report KSL-01-05, (2001) . |
[89] | A.-G. Olabi, Circular Economy and Renewable Energy, Energy 181: (2019) pp. 450–454. doi:10.1016/j.energy.2019.05.196. |
[90] | J. Patent, Office, Outline of Japanese patent classification systems, (2013) , available at: https://www.wipo.int/edocs/mdocs/aspac/en/wipo_reg_ip_tyo_13/wipo_reg_ip_tyo_13_t18.pdf. |
[91] | A. Pavel, L.A. Saarimäki, L. Möbus, A. Federico, A. Serra and D. Greco, The potential of a data centred approach & knowledge graph data representation in chemical safety and drug design, Computational and Structural Biotechnology Journal 20: ((2022) ), 4837–4849. doi:10.1016/j.csbj.2022.08.061. |
[92] | T. Pellegrini, G. Havur, S. Steyskal, O. Panasiuk, A. Fensel, V. Mireles, T. Thurner, A. Polleres, S. Kirrane and A. Schönhofer, DALICC: A license management framework for digital assets, in: Proceedings of the Internationales Rechtsinformatik Symposion (IRIS), Vol. 10: , (2019) . |
[93] | F.L. Piane, M. Baldoni, M. Gaspari and F. Mercuri, Introducing MAMBO: Materials and molecules basic ontology, (2021) , arXiv preprint arXiv:2111.02482. |
[94] | M. Poveda-Villalón, A. Gómez-Pérez and M.C. Suárez-Figueroa, Oops!(ontology pitfall scanner!): An on-line tool for ontology evaluation, International Journal on Semantic Web and Information Systems (IJSWIS) 10: (2) ((2014) ), 7–34. doi:10.4018/ijswis.2014040102. |
[95] | J. Reap, F. Roman, S. Duncan and B. Bras, A survey of unresolved problems in life cycle assessment: Part 2: Impact assessment and interpretation, The International Journal of Life Cycle Assessment 13: ((2008) ), 374–388. doi:10.1007/s11367-008-0009-9. |
[96] | E.U. Regulation, 2016/679 of the European Parliamentand of the Council of 27 April 2016 on the protectionof natural persons with regard to the processing of per-sonal data and on the free movement of such data, andrepealing Directive 95/46/EC (General Data ProtectionRegulation), Official Journal of the European Union L 119 ((2016) ), https://eur-lex.europa.eu/eli/reg/2016/679/oj. |
[97] | M. Saidani, B. Yannou, Y. Leroy, F. Cluzel and A. Kendall, A taxonomy of circular economy indicators, Journal of Cleaner Production 207: ((2019) ), 542–559. doi:10.1016/j.jclepro.2018.10.014. |
[98] | E. Sauter, R. Lemmens and P. Pauwels, CEO and CAMO ontologies: A circulation medium for materials in the construction industry, in: 6th International Symposium on Life-Cycle Civil Engineering (IALCCE), CRC Press, (2019) , pp. 1645–1652. |
[99] | E. Sauter and M. Witjes, Linked spatial data for a circular economy: Exploring its potential through a textile use case, in: SEMANTICS Posters&Demos, (2017) . |
[100] | C. Shimizu, K. Hammar and P. Hitzler, Modular ontology modeling, Semantic Web ((2021) ), 1–31. doi:10.3233/SW-210453. |
[101] | P. Shvaiko and J. Euzenat, Ten challenges for ontology matching, in: On the Move to Meaningful Internet Systems: OTM 2008: OTM 2008 Confederated International Conferences, CoopIS, DOA, GADA, IS, and ODBASE 2008, Proceedings, Part II, Monterrey, Mexico, November 9–14, 2008, Springer, (2008) , pp. 1164–1182. |
[102] | R. Sileryte, A. Wandl and A. van Timmeren, A bottom-up ontology-based approach to monitor circular economy: Aligning user expectations, tools, data and theory ((2021) ). |
[103] | L.N. Soldatova and R.D. King, An ontology of scientific experiments, Journal of the Royal Society Interface 3: (11) ((2006) ), 795–803. doi:10.1098/rsif.2006.0134. |
[104] | B. Sprecher, R. Kleijn and G.J. Kramer, Recycling potential of neodymium: The case of computer hard disk drives, Environmental science & technology 48: (16) ((2014) ), 9506–9513. doi:10.1021/es501572z. |
[105] | G. Stoilos, G. Stamou and S. Kollias, A string metric for ontology alignment, in: International Semantic Web Conference, Springer, (2005) , pp. 624–637. doi:10.1007/11574620_45. |
[106] | M.C. Suárez-Figueroa, A. Gómez-Pérez and M. Fernández-López, The NeOn methodology for ontology engineering, in: Ontology Engineering in a Networked World, Springer, (2012) , pp. 9–34. doi:10.1007/978-3-642-24794-1_2. |
[107] | J. Swetina, G. Lu, P. Jacobs, F. Ennesser and J. Song, Toward a standardized common M2M service layer platform: Introduction to oneM2M, IEEE Wireless Communications 21: (3) ((2014) ), 20–26. doi:10.1109/MWC.2014.6845045. |
[108] | A. Tauqeer, A. Kurteva, T.R. Chhetri, A. Ahmeti and A. Fensel, Automated GDPR contract compliance verification using knowledge graphs, Information 13: (10) ((2022) ), 447. doi:10.3390/info13100447. |
[109] | The BIMERR Project, Material properties Ontology, 2021, available at: https://bimerr.eu/wp-content/uploads/pdf/4.3%20BIMERR%20Ontology%20%26%20Data%20Model%202.pdf. |
[110] | The oneM2M Consortium, oneM2M base ontology specification V3.7.3, (2019), available at: https://www.onem2m.org/images/pdf/TS-0012-Base_Ontology-V3_7_3.pdf. |
[111] | K. Troullaki, S. Rozakis and V. Kostakis, Bridging barriers in sustainability research: A review from sustainability science to life cycle sustainability assessment, Ecological Economics 184: ((2021) ), 107007. doi:10.1016/j.ecolecon.2021.107007. |
[112] | M. Uddin and A.A. Rahman, Energy efficiency and low carbon enabler green IT framework for data centers considering green metrics, Renewable and Sustainable Energy Reviews 16: (6) ((2012) ), 4078–4094. doi:10.1016/j.rser.2012.03.014. |
[113] | J. van Driel, Naar een circulaire keten voor ICT-hardware [Towards a circular chain for ICT hardware], (2020) , available at: https://usi.nl/wp-content/uploads/2021/02/Eindrapport-Naar-een-circulaire-keten-voor-ICT-def.pdf. |
[114] | S.P. Voigt and S.R. Kalidindi, Materials graph ontology, Materials Letters 295: ((2021) ), 129836. doi:10.1016/j.matlet.2021.129836. |
[115] | P.A. Wäger, R. Hischier and R. Widmer, The material basis of ICT, in: ICT Innovations for Sustainability, Springer, (2015) , pp. 209–221. doi:10.1007/978-3-319-09228-7_12. |
[116] | E. Williams, R. Kahhat, B. Allenby, E. Kavazanjian, J. Kim and M. Xu, Environmental, social, and economic implications of global reuse and recycling of personal computers, Environmental science & technology 42: (17) ((2008) ), 6446–6454. doi:10.1021/es702255z. |
[117] | World Intellectual Property Organization (WIPO), International patent classification (IPC), (2022), Available at: https://tind.wipo.int/record/44834. |
[118] | A.C.G. Yowea and I.G.S. Astawa, Ontology-based approach for laptop semantic knowledge representation, Jurnal Elektronik Ilmu Komputer Udayana ((2021) ). |
[119] | X. Zhang, D. Pan, C. Zhao and K. Li, MMOY: Towards deriving a metallic materials ontology from Yago, Advanced Engineering Informatics 30: (4) ((2016) ), 687–702. doi:10.1016/j.aei.2016.09.002. |
[120] | S. Zhou, X. Dai, H. Chen, W. Zhang, K. Ren, R. Tang, X. He and Y. Yu, Interactive recommender system via knowledge graph-enhanced reinforcement learning, in: Proceedings of the 43rd International ACM SIGIR Conference on Research and Development in Information Retrieval, (2020) , pp. 179–188. doi:10.1145/3397271.3401174. |
[121] | G.I. Zlamparet, W. Ijomah, Y. Miao, A.K. Awasthi, X. Zeng and J. Li, Remanufacturing strategies: A solution for WEEE problem, Journal of Cleaner Production 149: ((2017) ), 126–136. doi:10.1016/j.jclepro.2017.02.004. |