Brain-machine interfaces for rehabilitation in stroke: A review
Abstract
BACKGROUND:
Motor paralysis after stroke has devastating consequences for the patients, families and caregivers. Although therapies have improved in the recent years, traditional rehabilitation still fails in patients with severe paralysis. Brain-machine interfaces (BMI) have emerged as a promising tool to guide motor rehabilitation interventions as they can be applied to patients with no residual movement.
OBJECTIVE:
This paper reviews the efficiency of BMI technologies to facilitate neuroplasticity and motor recovery after stroke.
METHODS:
We provide an overview of the existing rehabilitation therapies for stroke, the rationale behind the use of BMIs for motor rehabilitation, the current state of the art and the results achieved so far with BMI-based interventions, as well as the future perspectives of neural-machine interfaces.
RESULTS:
Since the first pilot study by Buch and colleagues in 2008, several controlled clinical studies have been conducted, demonstrating the efficacy of BMIs to facilitate functional recovery in completely paralyzed stroke patients with noninvasive technologies such as the electroencephalogram (EEG).
CONCLUSIONS:
Despite encouraging results, motor rehabilitation based on BMIs is still in a preliminary stage, and further improvements are required to boost its efficacy. Invasive and hybrid approaches are promising and might set the stage for the next generation of stroke rehabilitation therapies.
1Introduction
Even the most basic behavioral responses are created through the integrative activity of large networks in cortical and sub-cortical brain systems. Disturbances in the dynamic structures that constitute the motor network result in disorders of movement. The most frequent example of such a break-down consists of limb paralysis in stroke after the interruption of fiber tracts connecting intentional motor systems with the peripheral motor pathways.
Stroke, caused by ischemic or hemorrhagic injury to the brain, is one of the main causes of long-term motor disability worldwide, and in more than 85% of these cases, functional deficits in motor control remain (Langhorne, Bernhardt, & Kwakkel, 2011). These deficits result in major changes in the quality of life of the patients and their families as patients will require a support in their daily life that can range from occasional to full-time assistance. Furthermore, the treatments after a stroke cause enormous economic costs for the families and the health care systems (Kolominsky-Rabas et al., 2006; Lee, Hwang, Jeng, & Wang, 2010).
Promoting the autonomy of these patients is key to improve their quality of life and their re-integration in the work force and social networks. Compensation or substitution of the lost motor function can sometimes help those with stroke to become more independent, by changing the remaining motor patterns or by using technical aids such as canes or walkers (Rupp, 2017). On the other hand, rehabilitation programs try to restore the original or compensatory motor function.
Physical and behavioral therapy is the accepted method of motor rehabilitation for stroke patients. Bilateral arm training and constraint-induced movement therapy (CIMT) are two representative examples of therapies to promote motor recovery in people with stroke (Belda-Lois et al., 2011). However, sometimes motor rehabilitation treatments and their efficacy are limited, and their long-term effect is controversial (Bell, Wolke, Ortez, Jones, & Kerr, 2015; Kwakkel et al., 2004; Wu, Guarino, Lo, Peduzzi, & Wininger, 2016). For instance, patients with severe motor impairment have very limited treatment options and often remain with severe activity limitations at the chronic stage (Byblow, Stinear, Barber, Petoe, & Ackerley, 2015; Winters, van Wegen, Daffertshofer, & Kwakkel, 2015). Therefore, there remains a clear need for better motor rehabilitation interventions, tested in well-designed randomized clinical trials (RCTs). Furthermore, initiating the intervention early after stroke to exploit neuroplastic mechanisms is likely the best strategy to maximize recovery potential (Stinear & Byblow, 2014).
In order to improve the success of traditional motor rehabilitation, novel therapies have been proposed, trying to reactivate brain functional plasticity mechanisms and to promote neuronal repair and regeneration in lesioned neural networks, even in the chronic stage of stroke. One efficient and feasible way to stimulate the central/peripheral nervous system that might assist reactivating functional plasticity mechanisms are brain-machine interfaces (BMI). A BMI translates brain signals into computerized commands, which can be then used to stimulate the paralyzed limbs of the body, establishing a contingent link between the brain and the movement. These closed-loop neural interfaces activate neuroplastic mechanisms (e.g., Hebbian learning) (Jackson & Zimmermann, 2012). This is especially relevant for stroke patients, as the neuroplasticity effects are reinforced by the fact that the brain-controlled limb movements generate natural proprioceptive activity via the remaining afferents, facilitating instrumental learning. BMI-mediated motor recovery in stroke patients could therefore induce rewiring, reconnection or reactivation of silent pathways at any level of the nervous system.
2Stroke rehabilitation
2.1Existing stroke rehabilitation therapies
Brain reorganization in chronic stroke patients results normally in the over-use of the contralesional hemisphere and the limited-use of the lesioned hemisphere, leading to an increased inhibitory activity from the contralesional to the ipsilesional hemisphere. The increased inhibitory influence blocks excitatory reorganization of the remaining intact areas around the lesion and retards recovery of the affected motor system (Ward & Cohen, 2004). Behaviorally, individuals with stroke often choose to use the intact limb to achieve functional goals, neglecting to incorporate the impaired limb in any activity. This learning concept (i.e., learned non-use) created by Edward Taub explains the positive effects of therapies that force the patients to use the paretic arm and hand and “strengthen” the excitatory neural activity in the lesioned hemisphere.
Bilateral arm training (BAT) and constrained-induced movement therapy (CIMT) are the two most established methods to treat stroke-related motor impairments (for a comparison see (Lin, Chang, Wu, & Chen, 2009)). BAT engages both arms simultaneously in symmetrical or alternating patterns (Luft et al., 2004), whereas CIMT restraints the intact limb to force a patient to use the paretic one (Taub, Crago, & Uswatte, 1998). These rehabilitation strategies are bottom-up approaches, and rely on manipulating the limbs at the distal level to elicit a subsequent change in the neural circuits, in order to generate motor recovery (Belda-Lois et al., 2011).
Injury-related and treatment-related heterogeneities lead different patients to exhibit a wide range of responses to various rehabilitation therapies. Nonetheless, those with severe injuries generally present with limited or no recovery in response to traditional therapies (Belda-Lois et al., 2011). For this reason, many of the bottom-up approaches are not suitable for stroke patients with very low upper-limb motor ability (e.g., Fugl-Meyer score <20) and who display limited residual hand movement. For instance, CIMT has been proven inefficient for patients without residual movement one year after stroke (Wolf et al., 2008), and residual active movement is often a necessary prerequisite for such interventions (Birbaumer, Ramos-Murguialday, & Cohen, 2008).
As a consequence, there is no single rehabilitation technique that has been identified as being completely effective (Langhorne et al., 2011). It is generally accepted that task-specific rehabilitation strategies, when delivered in the appropriate context, are more efficacious than conventional rehabilitation therapies (Langhorne et al., 2011); yet conversely, the therapeutic effect of such techniques does not generalize to unrelated tasks (Belda-Lois et al., 2011).
To prime the effects of upper-limb therapy after stroke, intensive exercise and augmented feedback is usually suggested (with not much evidence behind this suggestion). Newer technologies are now available to facilitate standard therapy approaches to arm and hand recovery post-stroke (Asín Prieto et al., 2014). Robotic devices allow providing therapy for long periods of time in a consistent and measurable manner (Kwakkel, Kollen, & Krebs, 2008; Turner, Ramos-Murguialday, Birbaumer, Hoffmann, & Luft, 2013). Functional electrical stimulation (FES) can be used to generate action potentials in the motor nerves of the affected muscles, exercising the paralyzed limb and promoting the activation of the somatosensory cortex involved in the motor neural control loop (Barsi, Popovic, Tarkka, Sinkjaer, & Grey, 2008; Jackson & Zimmermann, 2012; Quandt & Hummel, 2014). Virtual reality environments are now being used to improve patients’ engagement and motivation by providing an enriched feedback, which can facilitate achieving the outcomes of standard rehabilitation therapies (Lohse, Shirzad, Verster, Hodges, & Van der Loos, 2013; Weiss, Kizony, Feintuch, & Katz, 2006).
Recent trends in stroke rehabilitation have begun to focus on assisting the reorganization of neural circuits in order to restore motor function. These top-down rehabilitation methods assume that the recovery is partly a consequence of peripheral mechanisms, but mostly due to the mechanism of brain plasticity (Belda-Lois et al., 2011). Therefore, the main challenge is to find the optimal ways to boost neuroplasticity during the therapy, to reinforce the peri-infarct connections and to potentiate the generation of new ones to improve long-term functional recovery.
2.2Neuroplasticity and stroke rehabilitation
Neuroplasticity has been defined as the ability of the nervous system to re-structure as a consequence of learning and stimulation (Cramer et al., 2011). One of the key learning mechanisms by which neuronal activity drives plasticity was first explained by Donald Hebb in 1949. According to his words: “When an axon of cell A is near enough to excite a cell B and repeatedly or persistently takes part in firing it, some growth process or metabolic change takes place in one or both cells such that A’s efficiency, as one of the cells firing B, is increased” (Hebb, 1949). His theory suggested that the connectivity between two neurons is strengthened when their activities have a persistent associative relationship, which was summarized in “cells that fire together, wire together”. The reorganization of the neural structures can be due to the modification of the strength in existing synapses or the formation of new synapses (Gazzaniga, 2006; Gould, Tanapat, Hastings, & Shors, 1999; Sampaio-Baptista et al., 2013).
Neural reorganization processes occur at multiple temporal and spatial levels during learning (e.g., by increasing the efficacy of information transfer or to boost control efficiency and accuracy), as a means to cope with injuries to the central nervous system (CNS) (Oweiss & Badreldin, 2015). During motor recovery after a stroke, different molecular, cellular and physiological changes have been identified (Ganguly, Byl, & Abrams, 2013). However, their specific contributions to different functional neuroplastic changes remain unclear.
Synapse-based learning rules are necessary to create compensatory circuits that allow regaining the lost motor function (Murphy & Corbett, 2009). More specifically, Hebbian mechanisms might play a fundamental role in generating activity-dependent plasticity. Coincident activation of presynaptic and postsynaptic neurons activates these Hebbian mechanisms. Depolarizing responses in the peri-infarct region induced by prolonged sensory inputs can therefore boost the excitability of those neurons. Neurons become more prone to generate action potentials when exposed to functionally related inputs, and those coincident activations can reinforce behaviorally-relevant circuits (Murphy & Corbett, 2009).
These changes in synaptic connectivity can be artificially imposed by using three stimulation paradigms: repetitive stimulation, paired stimulation, and closed-loop stimulation (Jackson & Zimmermann, 2012). The most precise way to induce this activity-dependent plasticity would be to carefully identify the two neural populations whose connections we want to reinforce and force them to excite in synchrony. In fact, it has been shown that rehabilitation paradigms based upon the generation of activity-dependent plasticity can improve long-term functional recovery (McPherson, Miller, & Perlmutter, 2015). Recovery of the motor function in stroke requires readjustment in the damaged networks responsible for coordinating motor tasks. One way is to maximize the likelihood of coincident activation of all the components of the motor network. To that end, we need a robust system to measure and decode the brain activity related to motor intentions and a precise timing of activation of motor and sensory nerves. Brain-machine interfaces constitute a promising technology for rehabilitation, since they can associate volition and action, exciting the motor network and reinforcing its synaptic elements.
3Brain-machine interfaces
A brain-machine interface (BMI) is a system that records, decodes, and ultimately translates brain signals into an effector action or behavior, without necessarily involving the motor system (see Fig. 1). Over the past 2 decades, an increasing number of BMI systems have been developed for communication, control of different types of devices and for rehabilitation (Chaudhary, Birbaumer, & Ramos-Murguialday, 2016; Lebedev & Nicolelis, 2017; Millán et al., 2010; Wolpaw, Birbaumer, McFarland, Pfurtscheller, & Vaughan, 2002).
Fig. 1
General diagram of a brain-machine interface for upper-limb motor rehabilitation. During the BMI therapy, the patient with upper-limb paralysis would be asked to imagine/attempt to move his/her paralyzed arm, and those intentions would be translated into the actual movement of the patient’s limb. The activity from the brain is recorded with noninvasive or invasive electrodes. Then, it is processed in a computer that extracts relevant features and decodes information from the imagined/attempted motor task, based on a calibration procedure performed with previously-recorded examples of movement imaginations/attempts. The information decoded from the brain activity is translated into control commands for the robotic or prosthetic device, which mobilizes the paralyzed limb of the patient, exciting his/her afferent pathways.
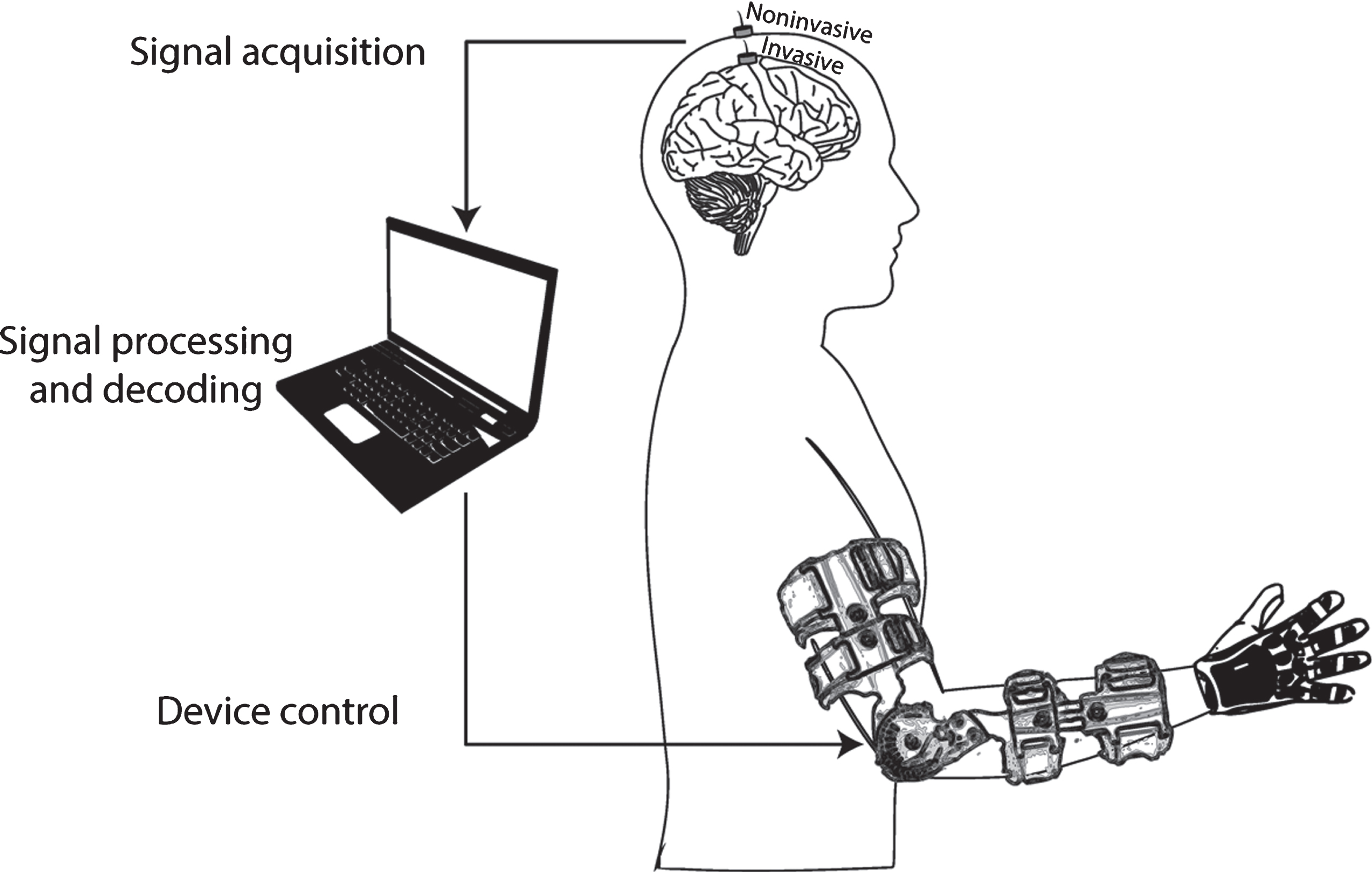
In the context of motor rehabilitation or substitution, the brain activity originated during movement intentions can be used to control external devices. These devices can perform those movements themselves with the purpose of substituting the lost motor function (e.g., a robotic arm). On the other hand, the devices can be used to guide the paralyzed limb of a patient (e.g., a mechanical orthosis), so that a desired movement can be performed, which in turn stimulates the damaged neural network and might have a neuroplastic and rehabilitative effect, as explained in the previous section.
3.1Signal acquisition techniques
Technologies to record the brain activity include invasive approaches, which offer high-quality signals with good spatial resolution, and noninvasive approaches, preferred for their lower cost and for not requiring a surgical intervention.
Existing invasive BMI systems have used intracranial microelectrode arrays, recording single-unit spiking activity and local field potentials, and electrode arrays for electrocorticography (ECoG) recordings. Microelectrodes have been successfully used in BMI experiments in the laboratory for the closed-loop control of robotic arms and electrical stimulation (Bouton et al., 2016; Collinger et al., 2013; Hochberg et al., 2012). ECoG recordings have been used for BMI research by using subdural electrodes (Schalk et al., 2007, 2008; Scherer, Graimann, Huggins, Levine, & Pfurtscheller, 2003), or epidural electrodes (Ramos-Murguialday et al., 2011), even in chronic stroke patients (Spüler et al., 2014). However, all these invasive recordings have yielded limited success outside the laboratory (Mestais et al., 2015). This is mainly due to problems with the long-term robustness of the signals (Suner, Fellows, Vargas-Irwin, Nakata, & Donoghue, 2005), making the efficacy of life-long implantation questionable. In fact, most of the studies relying on implantable systems to record brain activity are limited to short-term experiments, generally in patients with implants prior to epilepsy or surgery (Mestais et al., 2015). Fully implantable devices are currently under development (Borton, Yin, Aceros, & Nurmikko, 2013; Hirata et al., 2011; Mestais et al., 2015; Schuettler, Kohler, Ordonez, & Stieglitz, 2012), and great hope is placed in them for their potential to expand BMI technology to a large number of completely paralyzed patients, such as those with locked-in syndrome.
Noninvasive signal recordings for BMIs usually employ electroencephalography (EEG) due to its safety, portability and lower cost. It requires the user to wear a cap with multiple electrodes that is repositioned every session, and in general, conductive gel is applied to reduce the impedances between the electrodes and the skin. Wireless EEG systems are gaining importance as they simplify the setups and reduce artifacts generated by movements of the wires (Lee, Shin, Woo, Kim, & Lee, 2013). Dry or semi-dry electrodes have also been recently proposed to reduce the time necessary to prepare the recordings (Grozea, Voinescu, & Fazli, 2011; Zander et al., 2011), although they still need to reach comparable levels of signal quality and response to contamination as gel-based electrodes (Rupp, 2014). Other noninvasive approaches used in BMI studies are magnetoencephalography (MEG) (Buch et al., 2008), blood-oxygen-level dependent functional MRI (Weiskopf et al., 2003), and near infrared spectroscopy (NIRS) (Sitaram et al., 2007).
3.2Signal processing and decoding
In a standard closed-loop BMI scenario, the recorded brain signals are processed with spectral and spatial filters; some features are extracted from the filtered signals to discriminate between the different actions to be decoded; and finally, a pattern recognition algorithm translates those features into information that can be used to control the external device, such as movement commands.
There are several papers reviewing the most representative algorithms that can be used to improve the performance of BMI systems (Bashashati, Fatourechi, Ward, & Birch, 2007; Bashashati, Ward, Birch, & Bashashati, 2015; Lotte, Congedo, Lécuyer, Lamarche, & Arnaldi, 2007). However, the element that has the largest influence on the precision and function of the BMI is still the recording of the neural activity.
On the one hand, invasive recordings have a high signal-to-noise ratio and allow the decoding of different movements of the same limb (Pistohl, Schulze-Bonhage, Aertsen, Mehring, & Ball, 2012; Spüler et al., 2014) and even permit reconstruction of 2D and 3D trajectories (Collinger et al., 2013; Hochberg et al., 2006, 2012). On the other hand, noninvasive technologies have been used to discriminate single movement commands from rest. For instance, the onset of movements of the upper- and lower-limbs can be decoded (Ibáñez et al., 2014; Jiang, Gizzi, Mrachacz-Kersting, Dremstrup, & Farina, 2015; Niazi et al., 2011; Sburlea et al., 2015), with performances that vary depending on the type of movement classified (López-Larraz, Montesano, Gil-Agudo, & Minguez, 2014). More recent works are proposing methodologies to decode different movements from the same limb (Ofner, Schwarz, Pereira, & Müller-Putz, 2017; Shiman et al., 2017), although their integration in closed-loop scenarios is yet to be demonstrated.
Two of the main challenges for the establishment of this technology in clinical practice or for neuroprosthetics control, especially with noninvasive recordings, are the session-to-session non-stationarities (i.e., the characteristics of the signals change with time) (López-Larraz et al., 2018; Shiman et al., 2017) and the signal contaminations by artifacts. These artifacts can be generated by the devices controlled with the BMI (e.g., noise generated by actuators based on electric/magnetic neurostimulation, or on robotic devices) (Insausti-Delgado et al., 2017; Walter et al., 2012) or have a physiological origin (e.g., compensatory movements, cranial and neck muscle activity, eye movements, swallowing, etc.) (López-Larraz, Bibián, Birbaumer, & Ramos-Murguialday, 2017).
3.3Device control
The objective of neuroprostheses applied in a motor rehabilitative context is to excite the specific peripheral nervous systems responsible for a particular movement in order to facilitate neuroplasticity, especially by engaging proprioceptive mechanisms (Mrachacz-Kersting, Kristensen, Niazi, & Farina, 2012; Ramos-Murguialday et al., 2012). Decoding simple binary commands from EEG activity has allowed to control different rehabilitative devices, and in fact, is a methodology that has already shown some efficacy in a laboratory setting for inducing functional recovery in stroke patients (Ang et al., 2015; Ono et al., 2014; Pichiorri et al., 2015; Ramos-Murguialday et al., 2013).
Robotic systems are available to guide the movements of paralyzed limbs of the patients. They constitute a very relevant clinical tool as they allow performing repetitive tasks with a very fine precision (Krebs et al., 2003). Since the appearance of the MIT Manus in the early 90s (Hogan, Krebs, Charnnarong, Srikrishna, & Sharon, 1992), hundreds of robotic devices have been proposed with different degrees of freedom and complexity. Robotic exoskeletons controlled with EEG have also been used in the upper-limb to open and close the hand of the patients (Pfurtscheller, Guger, Müller, Krausz, & Neuper, 2000; Ramos-Murguialday et al., 2013), flex their arm (Ang et al., 2015), or perform complex reaching movements (Sarasola-Sanz et al., 2016); and in the lower-limb to facilitate gait, with andwithout body-weight support (Do, Wang, King, Chun, & Nenadic, 2013; K. Lee, Liu, Perroud, Chavarriaga, & Millán, 2017; López-Larraz et al., 2016).
Functional electrical stimulation (FES), also called neuromuscular electrical stimulation (NMES), utilizes surface electrodes and electrical discharges above motor-threshold to artificially activate the nerves and muscles (Lynch & Popovic, 2008). For upper-limb paralysis, this technology has been used to facilitate grasping (Pfurtscheller, Müller, Pfurtscheller, Gerner, & Rupp, 2003; Trincado-Alonso et al., 2017) and reaching movements (Ibáñez et al., 2017), and even to assist walking in patients with lower-limb paralysis (King et al., 2015). Recent trends also propose the use of low-intensity NMES (i.e., below the motor threshold) to provide an enriched sensory feedback (Corbet, Iturrate, Pereira, Perdikis, & Millán, 2018), enough to stimulate the skin and joint mechanoreceptors that might alsosupport neuroplasticity.
Virtual reality environments have been integrated in BMI systems to engage and motivate patients (Ron-Angevin & Díaz-Estrella, 2009). In fact, visual feedback-mediated BMI therapies can be used to guide motor imagery training, which has positive effects in stroke recovery (Pichiorri et al., 2015).
3.4BMI control and learning
Learning to control a BMI entails changes in the brain activity, which can be reflected, for instance, as the enhancement of slow cortical potentials (Birbaumer, 1999), focusing of sensorimotor-rhythms (Buch et al., 2008; McFarland, Sarnacki, & Wolpaw, 2010) and BOLD topographies (Enzinger et al., 2008; Ramos-Murguialday et al., 2013). Although it has been shown that it is possible to change the neural network involved in motor recovery (Ramos-Murguialday et al., 2013), further experimental work needs to be done to link neurophysiological changes and motor function recovery at a behavioral level.
In order to learn a neuroprosthetic skill, the brain modifies the connections to the neurons involved in the BMI (i.e., causally related to motor output) and minimizes the error in the motor output through a process of cortical plasticity (Carmena et al., 2003). Enacting skillful control of a prosthetic device (i.e., achieving control and dexterity comparable to natural movements) involves the incorporation of a disembodied device in the brain. Recent advances highlight the importance of the acquisition and retention of neuroprosthetic skills, i.e., accurate, readily-recalled neural control of disembodied actuators, irrespective of natural physical movements (Carmena, 2012). These concepts might be different for brain-controlled actuators moving the user’s body (e.g., using an exoskeleton), because the causal connection between brain and motor output is artificial but generates feedback comparable to the natural case; i.e., the neuroprosthetic skill will be more“natural”.
Each hemisphere of the motor cortex (i.e., the side of the brain typically believed to control the contralateral limb) contains significant information about the state of the ipsilateral limb (Ganguly et al., 2009). This is paramount for stroke patients, since the activity of the contralesional hemisphere can be used as control signals for BMI in cases when the ipsilesional hemisphere is severely damaged (Antelis, Montesano, Ramos-Murguialday, Birbaumer, & Minguez, 2017; López-Larraz, Ray, et al., 2017). However, the role of the contralesional hemisphere in motor rehabilitation after stroke is still not well understood, and to our knowledge, no study has proven the efficacy of using the contralesional hemisphere activity only in a BMI based rehabilitation trial.
The performance of the rehabilitative BMI system linking movement-related brain activity and movement is assumed to play a pivotal role in recovery. For this reason, research is being conducted to maximize the BMI effectiveness, in which brain and machine adaptation are key. On the one hand, neuroprosthetic motor memory is facilitated through cortical plasticity. On the other hand, the adaptation can also take place in the machine instead of the brain, with the BMI adapting itself to the specific characteristics of each particular patient to optimize performance (Iturrate, Chavarriaga, Montesano, Minguez, & Millán, 2015). The optimal and synergistic combination of both adaptive mechanisms might constitute a breakthrough for the skillful and naturalistic control of disembodied devices, comparable to natural movements (Dangi, Orsborn, Moorman, & Carmena, 2013; Orsborn, Dangi, Moorman, & Carmena, 2012), especially to elicit motor functional plasticity.
4BMIs for motor rehabilitation in stroke
At the heart of the BMI paradigm lays the operant (“volitional”) control of neural activity, initially proposed by Fetz and collaborators (Fetz, 1969; Fetz & Baker, 1973). Recent experiments have demonstrated corticospinal synaptic plasticity in vivo at the level of single neurons by the use of a recurrent neural interface (Nishimura, Perlmutter, Eaton, & Fetz, 2013). Furthermore, artificial afferent feedback can reorganize motor cortex outputs, suggesting that, under normal conditions, cortico-muscular relations are maintained through physiological feedback loops (Lucas & Fetz, 2013). These results suggest that artificial neural connections involving afferent and efferent contingency (bidirectionally consistent) activate neuroplastic mechanisms. Motor rehabilitative BMI systems rely on a neurofeedback training paradigm, establishing such a contingent link between the brain and the paralyzed limbs to re-establish the damaged pathways, which might facilitate the recovery of lost motor functions (Chaudhary et al., 2016; Daly & Wolpaw, 2008).
4.1Current results of BMI-based stroke rehabilitation
The first pilot study applying a BMI in the context of stroke rehabilitation appeared in 2008 (Buch et al., 2008). An MEG-based BMI was used to measure the amplitude of the mu rhythm originated in the sensorimotor cortex and link it with a visual feedback in the form of a cursor on a screen. Somatosensory feedback was provided to the patients only at the end of each trial, as long as the trial had been successful: i.e., if the patient had been able to modulate the mu rhythm long enough. Therefore, in this case the somatosensory feedback was not provided online and in a contingent manner, which might be the reason why the 8 studied patients did not experience any clinical improvement (Buch et al., 2008).
It was not until the year 2013 that the first double-blinded controlled clinical trial demonstrating the rehabilitative efficacy of a BMI for completely paralyzed stroke patients was published (Ramos-Murguialday et al., 2013). In this study, the EEG-based BMI intervention was conducted immediately before behavioral physiotherapy. Thirty-two patients were recruited and assigned to two matched groups: the intervention group received contingent proprioceptive feedback associated to the modulation of their ipsilesional mu rhythm, while the control group received sham feedback unrelated to their brain activity. The proprioceptive feedback was provided by means of arm and hand robotic devices, supporting reaching and hand opening movements. The patients learned over 20 sessions to control the BMI by decreasing the power of the mu rhythm of the ipsilesional motor cortex while attempting to move their paralyzed arm, even if no movement was possible. Both groups received identical physiotherapy treatment right after the BMI sessions. Only the experimental group showed significant motor learning (i.e., increase in orthosis brain control), significant improvement in motor function (i.e., Fugl-Meyer upper limb scores), significant improvement in muscle control and significant brain activity reorganization reflected by BOLD activity (Ramos-Murguialday et al., 2013).
Subsequent studies have confirmed these positive results, providing more evidence of the rehabilitative potential of BMI for stroke. Table 1 summarizes some of the most relevant and recent articles on BMIs for stroke motor rehabilitation. In this review of the state of the art, we present studies published up to September 2017 of BMI therapies for stroke motor rehabilitation, involving (at least) a control group and reporting clinical scales pre- and post-intervention.
Table 1
Characteristics and outcome of the most recent and relevant articles on BMIs for motor rehabilitation after stroke
Study | N | Chronicity | Severity | Therapy duration | Feedback/ target structure | BMI modality | Exp. Design | Control group | Outcome measures | Significant motor improvement? | |||
---|---|---|---|---|---|---|---|---|---|---|---|---|---|
R | Bl (P) | Bl (C) | Bl (E) | ||||||||||
Ang et al., (2014) | 21 (6/8/7) | Chronic | Moderate to severe | 6 weeks, 3d/w, 90m/d | Haptic knob/ Hand and wrist | EEG-MI (initiation) | X | X | C1) Haptic knob C2) Standard therapy | FMA-UE | Yes, in all groups, no intergroup differences. ΔFMA = 8.2 (IG); ΔFMA = 6.5 (C1); ΔFMA = 3.6(C2) | ||
Ang et al., (2015) | 25 (11/14) | Chronic | Moderate to severe | 4 weeks, 4d/w, 1h/d | MIT-Manus/ Shoulder and elbow | EEG-MI (initiation) | X | X | MIT-Manus only | FMA-UE | Yes, in both groups, no intergroup differences. ΔFMA = 4.5 (IG); ΔFMA = 6.3 (CG) | ||
Frolov et al., (2017) | 74 (55/19) | Subacute and chronic | Severe | 10 days, 40m/d | Exoskeleton/ Hand | EEG-MI (continuous) | X | X | X | Sham feedback | FMA-UE, ARAT | Yes, in both groups, no intergroup differences. ΔFMA = 5.0, ΔARAT = 2.0 (IG); ΔFMA = 5.0, ΔARAT = 3.0 (CG) | |
Kasashima-Shindo et al., (2015) | 18 (11/7) | Chronic | Severe | 2 weeks, 5d/w, 45m/d | Orthosis/ Hand | EEG-MI + tDCS (initiation) | X | MI-BCI only (no tDCS) | FMA-UE | Yes, in both groups, no intergroup differences. ΔFMA = 6.0 (IG); ΔFMA = 6.6 (CG) | |||
Kim et al., (2016) | 30 (15/15) | Chronic | Moderate | 4 weeks, 5d/w, 30m/d | FES/ Wrist | EEG-MI (initiation) | X | X | Conventional therapy | FMA-UE, MAL, MBI, ROM | Yes, in both groups, significantly bigger improvements in IG. ΔFMA = 7.9 (IG); ΔFMA = 2.9 (CG) | ||
Li et al., (2014) | 14 (7/7) | Subacute | Severe | 8 weeks, 3d/w, 1h/d | FES/ Ext. Carpi Radialis | EEG-MI (initiation) | X | X | X | FES | FMA-UE, ARAT | Yes, both groups. No intergroup differences in FMA, but differences in ARAT. ΔFMA = 12.7, ΔARAT = 18.0 (IG); ΔFMA = 6.7, ΔARAT = 7.6 (CG) | |
Mihara et al., (2013) | 20 (10/10) | Subacute | Severe | 2 weeks, 3d/w | Visual feedback/ Upper limb | NIRS-MI (continuous) | X | X | X | X | Sham feedback | FMA-UE, ARAT, MAL, KVIQ-10 | Yes, in both groups, significantly bigger improvements in IG. ΔFMA = 5.0 (IG); ΔFMA = 2.3 (CG) |
Mrachacz-Kersting et al., (2016) | 22 (13/9) | Chronic | Moderate | 3 days, 10m/d | FES / Lower limb | EEG-MA (MRCP paired stimulation) | X | X | Sham feedback | FMA-LE, 10-MWS, hand/foot tapping | Yes, in the IG only: ΔFMA = 0.8 (IG) | ||
Mukaino et al., (2014) | 1: A-B-A-B design | Chronic | Severe | 2 weeks each phase, 5d/w, 1h/d | FES/ Hand | EEG-MA (initiation) | X | FES | FMA-UE, MAS | Larger improvements during the BMI than during the control phases. ΔFMA = 3.5 (IG); ΔFMA = 0.5 (CG) | |||
Ono et al., (2014) | 12 (6/6) | Chronic | Severe | 12-20 days, 1h/d | Orthosis/ Hand | EEG-MA (continuous) | BMI + Visual feedback | SIAS | Three patients in the IG improved one step in the SIAS, while no changes in the CG | ||||
Pichiorri et al., (2015) | 28 (14/14) | Subacute | Severe | 4 weeks, 3d/w | Visual feedback/ Hand | EEG-MI (continuous) | X | X | MI only | FMA-UE, MRC, NIHSS | Yes, in both groups, significantly bigger improvements in IG. ΔFMA = 44.0 (IG); ΔFMA = 19.8 (CG) | ||
Ramos-Murguialday et al., (2013) | 32 (16/16) | Chronic | Severe | 4 weeks, 5d/w | Hand & arm orthoses/ Upper limb | EEG-MA (continuous) | X | X | X | X | Sham feedback | FMA-UE, GAS, MAL, MAS | Yes, in the IG group only. ΔFMA = 3.4 (IG) |
Rayegani et al., (2013) | 30 (10/10/10) | Subacute | Moderate | 2 weeks, 5d/w, 1h/d | Visual feedback/ Upper limb | EEG-MI (continuous) | X | X | C1) Occupational therapy (OT) C2) OT + EMG-biofeedback | JHFT | Yes, in all groups, no intergroup differences. ΔJHFT = 38 (IG); ΔJHFT = 30 34 (C1); ΔFMA = 40 (C2) |
[i] Values are given depending on the provided information on the article. N: Sample size (after removing possible dropouts); in brackets are shown the number of patients within each group, with the number of patients of the intervention group marked in bold, followed by the number of patients in the control group or groups (if more than one). Chronicity and severity are given based on the inclusion criteria and the pre-intervention clinical scales. The therapy duration is given in weeks, days per week (d/w) and minutes or hours per day (m/d or h/d), when available. Feedback modality and target structure are shown together. BMI modality provides information about the technology used to record the brain activity, the task that the patients were requested to perform (either motor imagery— MI— or motor attempt— MA), and how the feedback was timed with respect to the brain activity (e.g., feedback was “triggered” by brain activity or continuous). Columns with details about the experimental design denote if the patients were assigned to the different groups by randomization procedures (R); if the patients were blinded to the intervention (Bl (P)); if the clinicians performing the clinical assessments were blinded (Bl (C)); and if the experimenters guiding the therapy were blinded (Bl (E)). The outcome measures are provided for the intervention (IG) and control groups (CG), including: Fugl-Meyer scales of the upper or lower extremity (FMA-UE or FMA-LE), Motor Activity Log (MAL), Action Research Arm Test (ARAT), Goal Attainment Score (GAS), Modified Ashworth Scale (MAS), Kinesthetic and Visual lmagery Questionnaire-10 (KVIQ-10), Modified Barthel Index (MBI), Range of Motion (ROM), National Institute Health Stroke Scale (NIHSS), Medical Research Council scale for muscle strength (MRC), the Stroke Impairment Assessment Scale (SIAS) and the Jebsen Hand Function Test (JHFT). The motor improvements are provided as mean differences (Post-Pre) in the corresponding clinical scales.
4.1.1Sample characteristics
The number of subjects included in the reviewed studies varied widely from 1 to 74 (mean±std: 25.15±17.01; median: 22). Eight out of the thirteen studies involved chronic patients only (Ang et al., 2014, 2015; Kasashima-Shindo et al., 2015; Kim, Kim, & Lee, 2016; Mrachacz-Kersting et al., 2016; Mukaino et al., 2014; Ono et al., 2014; Ramos-Murguialday et al., 2013), whereas one included chronic and subacute (Rayegani et al., 2014), and four included subacute patients only (Frolov et al., 2017; Li et al., 2014; Mihara et al., 2013; Pichiorri et al., 2015). The results in chronic stroke patients do not have the confound of spontaneous recovery that may be seen in the sub-acute and acute phases. For this reason, most of the studies try to demonstrate the efficacy of BMIs in chronic patients, despite knowing that the spontaneous activation of neuroplastic mechanisms could potentially boost the BMI effect.
Since the main advantage of a BMI is that it can provide patients having no residual movement with a movement-related control signal, most of the studies, i.e., eight out of thirteen, recruited only severely paralyzed stroke patients (Frolov et al., 2017; Kasashima-Shindo et al., 2015; Li et al., 2014; Mihara et al., 2013; Mukaino et al., 2014; Ono et al., 2014; Pichiorri et al., 2015; Ramos-Murguialday et al., 2013); two studies recruited patients with moderate or severe stroke (Ang et al., 2014, 2015), while three studies recruited only patients with moderate stroke (Kim et al., 2016; Mrachacz-Kersting et al., 2016; Rayegani et al., 2014), where some residual voluntary movement was present.
4.1.2Type of intervention
The duration of the interventions ranged from 1 week (Mrachacz-Kersting et al., 2016) to 8 weeks (Li et al., 2014) and each of the sessions lasted between 30 and 90 minutes. Twelve out of the thirteen studies targeted the upper limb, while only one focused on the lower limb (Mrachacz-Kersting et al., 2016). Regarding the technology used to record the brain activity, only one study used NIRS (Mihara et al., 2013), while all the others relied on EEG because of its time resolution, cost and ease of use. The type of actuator used to provide feedback varied between studies, with six utilizing robotic or orthotic devices (Ang et al., 2014, 2015; Frolov et al., 2017; Kasashima-Shindo et al., 2015; Ono et al., 2014; Ramos-Murguialday et al., 2013), four using electrical stimulation (Kim et al., 2016; Li et al., 2014; Mrachacz-Kersting et al., 2016; Mukaino et al., 2014), and three using visual feedback only (Mihara et al., 2013; Pichiorri et al., 2015; Rayegani et al., 2014). Four of the studies requested subjects to attempt to move their paralyzed limb to elicit a signal for the BMI (Mrachacz-Kersting et al., 2016; Mukaino et al., 2014; Ono et al., 2014; Ramos-Murguialday et al., 2013), and the remaining nine relied on motor imagery, which does not include top-down descending volleys reaching the muscles.
The variability in experimental design between studies is also evident. Nine of the studies followed randomization procedures to allocate the patients into different experimental groups (Ang et al., 2014, 2015; Frolov et al., 2017; Kim et al., 2016; Li et al., 2014; Mihara et al., 2013; Pichiorri et al., 2015; Ramos-Murguialday et al., 2013; Rayegani et al., 2014). With respect to blinding procedures, we studied three parameters: 1) if patients were blinded to the intervention they were performing; 2) if the clinicians performing the clinical assessment of each patient were blinded to the subject’s group assignment; and 3) if the experimenters conducting the BMI therapy were blinded to group assignment. In four of the studies the patients were blinded to the type of intervention provided (Frolov et al., 2017; Mihara et al., 2013; Mrachacz-Kersting et al., 2016; Ramos-Murguialday et al., 2013). The personnel performing the clinical assessment were blinded in eleven of the studies (Ang et al., 2014, 2015; Frolov et al., 2017; Kasashima-Shindo et al., 2015; Kim et al., 2016; Mihara et al., 2013; Mrachacz-Kersting et al., 2016; Mukaino et al., 2014; Pichiorri et al., 2015;Ramos-Murguialday et al., 2013; Rayegani et al., 2014), and the experimenters were blinded in only two of the studies (Mihara et al., 2013; Ramos-Murguialday et al., 2013).
Several types of control conditions were included. Some of the studies included a control condition in which the actuator was moved without involving a BMI (Ang et al., 2014, 2015; Li et al., 2014; Mukaino et al., 2014); in these cases, the patients were never blinded to the intervention. Perhaps a better control strategy was used in studies where patients in the control group performed a pseudo-BMI blinded therapy, where the movement of the actuator was not linked to the brain activity, but rather to a sham feedback (Frolov et al., 2017; Mihara et al., 2013; Mrachacz-Kersting et al., 2016; Ramos-Murguialday et al., 2013). Three of the studies compared the BMI therapy with conventional physical or occupational therapy (Ang et al., 2014; Kim et al., 2016; Rayegani et al., 2014). The rest of the studies used other approaches for study control: comparing proprioceptive BMI versus visual BMI (Ono et al., 2014); motor imagery BMI with visual feedback versus motor imagery without feedback (Pichiorri et al., 2015); BMI versus EMG-based biofeedback (Rayegani et al., 2014); and comparing the combination of BMI and transcranial direct current stimulation (tDCS) with BMI only (Kasashima-Shindo et al., 2015).
4.1.3Outcome measures and improvement
Eleven out of the thirteen studies reported Fugl-Meyer score as the primary outcome measure (Ang et al., 2014, 2015; Frolov et al., 2017; Kasashima-Shindo et al., 2015; Kim et al., 2016; Li et al., 2014; Mihara et al., 2013; Mrachacz-Kersting et al., 2016; Mukaino et al., 2014; Pichiorri et al., 2015; Ramos-Murguialday et al., 2013), although there were some differences in the sub-scale presented. The other two studies reported the Stroke Impairment Assessment Set (SIAS) (Ono et al., 2014) or the Jebsen Hand Function Test (JHFT) (Rayegani et al., 2014) to assess the impairment of the patients.
All the studies reported improvements of the motor function after the use of the BMI. Only two of the studies reported significant motor improvements (e.g., Fugl-Meyer) in the patients undergoing BMI therapy and no improvements in the control group (Mrachacz-Kersting et al., 2016; Ramos-Murguialday et al., 2013). Four of the studies reported significant improvements both in the intervention (i.e., BMI therapy) and in the control group, but significantly higher improvements in the BMIintervention (Kim et al., 2016; Li et al., 2014; Mihara et al., 2013; Pichiorri et al., 2015). Five studies showed significant improvements in the intervention and control groups, with no significant differences between groups (Ang et al., 2014, 2015; Frolov et al., 2017; Kasashima-Shindo et al., 2015; Rayegani et al., 2014). From these studies, and excluding the one by Rayegani et al., 2015 that did not include Fugl-Meyer values, the patients in the intervention groups presented an average improvement in Fugl-Meyer of 5.9 points, while the patients in the control groups showed an average improvement of 5.6 points. The remaining two studies lacked statistical comparisons. In the study by Ono et al., the authors reported improvements in the Stroke Impairment Assessment Scale (SIAS) in three out of six patients within the intervention group and in none of the six in the control group (Ono et al., 2014). In the case study performed by Mukaino and colleagues with an A-B-A-B design, they observed greater improvements in the patient during the two two-week BMI intervention phases than during the two control (i.e., electrical stimulation irrespective of the brain activity) phases (Mukaino et al., 2014).
4.1.4Summary
In general, the BMI experimental interventions led to better recovery than the control conditions, resulting in higher gains in Fugl-Meyer score or other clinical scales. From the thirteen studies presented in this review, no direct conclusion can be extracted regarding the best type of feedback or BMI modality for achieving better functional outcomes. The six studies demonstrating higher improvements in the intervention group than in the control group include therapies based on FES (Kim et al., 2016; Li et al., 2014; Mrachacz-Kersting et al., 2016), robotics (Ramos-Murguialday et al., 2013) or visual feedback (Mihara et al., 2013; Pichiorri et al., 2015); utilizing EEG (Kim et al., 2016; Li et al., 2014; Mrachacz-Kersting et al., 2016; Pichiorri et al., 2015; Ramos-Murguialday et al., 2013) or NIRS (Mihara et al., 2013); relying on motor attempt (Mrachacz-Kersting et al., 2016; Ramos-Murguialday et al., 2013) and motor imagery (Kim et al., 2016; Li et al., 2014; Mihara et al., 2013; Pichiorri et al., 2015); and with the feedback being continuous during several seconds (controlling online movement of the robot or cursor) (Mihara et al., 2013; Pichiorri et al., 2015; Ramos-Murguialday et al., 2013) or discrete, triggering a preprogrammed activation of a robot or FES (Kim et al., 2016; Li et al., 2014; Mrachacz-Kersting et al., 2016). The duration of the intervention might not be a critical issue either, since the studies with the shortest (Mrachacz-Kersting et al., 2016) and longest (Li et al., 2014) duration are also part of these six selected works showing BMI superiority over control intervention. From these studies, the typology of the patients who respond best to BMI with respect to chronicity and severity was not conclusive, as they included chronic (Kim et al., 2016; Mrachacz-Kersting et al., 2016; Ramos-Murguialday et al., 2013) and sub-acute (Li et al., 2014; Mihara et al., 2013; Pichiorri et al., 2015) patients, with severe (Li et al., 2014; Mihara et al., 2013; Pichiorri et al., 2015; Ramos-Murguialday et al., 2013) or moderate (Kim et al., 2016; Mrachacz-Kersting et al., 2016) paralysis.
Experimental rigor and methodology might influence the interpretability of the studies presented in this literature review. There is a clear need for more randomized controlled BMI interventional clinical trials in stroke patients. Future trials would greatly benefit from a larger sample size and a standardized control condition/group that could be used as a reference for any variation in the BMI concept tested. Furthermore, correlations between neurophysiological changes, BMI performance (i.e., brain-to-muscle link) and functional clinical scores are needed to understand the differences in results among studies, and to better characterize the functional neuroplastic changes involved in motor recovery. The feedback and signal processing methodologies, experimental protocols and subject instructions are key elements of BMI clinical trials for motor rehabilitation, and larger consensus regarding the technological approach to be used in research (i.e., innovating in one front only in the next clinical trials) in the field is needed in order to progress adequately.
5Conclusions and future perspectives
Although significant and promising, the functional motor recovery achieved with novel BMI technology remains modest. Studies involving BMI interventions that included sham feedback, in which stimulation may or may not coincide with the brain activity (Frolov et al., 2017; Mihara et al., 2013; Mrachacz-Kersting et al., 2016; Ramos-Murguialday et al., 2013), showed that the measured improvements are better when the afferent stimulation is associated with the degree of brain activation. This underlines the importance of having a precise and accurate feedback to boost the learning.
5.1Cortico-muscular hybrid BMIs
There is a general consensus about the need of new methodologies to improve the contingent link between the brain and the paralyzed muscles in order to maximize Hebbian plasticity and subsequent motor recovery. The improvement of such cortico-muscular link would require a precise identification of the intended motor task, to link the activation of the neural populations responsible for that task with the proprioceptive feedback in the form of the actual movement. There is evidence showing that different neural populations modulate movements of the arm in different directions (Georgopoulos, Schwartz, & Kettner, 1986), and recent invasive studies have shown that the brain activity of different movements of the same limb can be decoded (Collinger et al., 2013; Spüler et al., 2014). Classification of different motor tasks of the same limb with EEG has been recently demonstrated (Ofner et al., 2017; Shiman et al., 2017), although performances are still far from providing a natural and skilled control.
The electromyography (EMG) has shown its feasibility for decoding arm, wrist and individual finger movements (Irastorza-Landa et al., 2017; Parker, Englehart, & Hudgins, 2006; Sarasola-Sanz et al., 2015; Tenore et al., 2009). It has been used for the control of prosthetic and robotic rehabilitative devices (Hesse et al., 2005; Zecca, Micera, Carrozza, & Dario, 2002; Zhou, Wang, Bao, Lü, & Wang, 2016). However, EMG activity of stroke patients during motor attempts is not easy to decode, and might not be a feasible option for all of them (Cesqui, Tropea, Micera, & Krebs, 2013). A recent study demonstrated that movement decoding using EMG is possible in approximately 45% of severely paralyzed chronic stroke patients (Ramos-Murguialday et al., 2015). These patients can also present pathologic muscle synergies (García-Cossio, Broetz, Birbaumer, & Ramos-Murguialday, 2014), and therefore, reinforcing them might result in maladaptive plasticity.
Therefore, cortico-muscular hybrid BMIs (hBMIs) have been recently devised, aiming at building more robust rehabilitation systems that overcome the limitations of BMI and isolated myoelectric interfaces. Such hBMIs include residual muscle activity in the BMI control, and hence, in the contingent connection between perilesional cortical areas and movement related afferent feedback. These enriched hBMIsystems can lead to a higher decoding accuracy (Kiguchi & Hayashi, 2012; Leeb, Sagha, Chavarriaga, & Millán, 2011; Li et al., 2017) and more degrees of freedom (Kiguchi, Lalitharatne, & Hayashi, 2013), reflected in a richer and smoother control of actuators. A recent study proposed a biologically-inspired hybrid strategy, involving brain and muscle activity, to control a 7 degrees-of-freedom robotic arm, and demonstrated its viability in a moderately paralyzed stroke patient (Sarasola-Sanz et al., 2017). The hierarchical control used the EEG activity to monitor the intended movement of the subject (Sarasola-Sanz et al., 2016), and when the attempt of movement was detected, the EMG activity was sequentially used to estimate the kinematics of the robot (Sarasola-Sanz et al., 2015).
Despite the encouraging results, hBMIs are still in a preliminary development stage, and further experiments in larger number of patients are necessary to assess their effectiveness in eliciting motor rehabilitation.
5.2Current challenges
Neural interfaces have provided us with knowledge of how the brain generates behavior and the mechanisms involved in functional neural plasticity. Recent data in severely impaired chronic stroke patients indicate that contingent neural linkage between brain activity and movements of the paralyzed extremity can induce significant functional motor recovery. However, full motor function recovery has not been achieved yet. These neural interfaces are in their early stage of development and present several limitations.
From the signal recording point of view, the ability to accurately decode all the degrees of freedom of the arm and leg to provide dexterous and natural control (e.g., grasping and manipulation of different objects) still remains a challenge. While implantable electrodes can provide a very accurate decoding of movement intentions, user acceptance of invasive BMIs is still low, mainly due to the risks related to the neurosurgery and postsurgical complications (Waldert, 2016). On the other hand, noninvasive approaches have generally provided low accuracies. Hybrid BMIs might be able to improve the results achieved so far with EEG-based BMIs linking brain and residual muscle activity with movements, although they still have to be tested in real rehabilitation interventions to show their potential.
The way the neural activity is processed to extract relevant information from a generally noisy signal plays a key role in the rehabilitative outcome. The huge variability in how the brain is damaged after a stroke, added up to the already-unique cortical patterns of each individual, results in diverse cortical activities among patients during the performance of the same task (López-Larraz, Ray, et al., 2017; Park, Kwon, Kim, Lee, & Kim, 2016; Ray, López-Larraz, Figueiredo, Birbaumer, & Ramos-Murguialday, 2017; Stępień et al., 2011). Detailed screenings of each patient for the personalization of the BMIs and the therapies to the specific characteristics of each individual might be key to boost the effect of these therapies.
Another key mechanism to enhance the rehabilitative effects of BMI therapies is the contingent stimulation of afferent pathways. Somatosensory and proprioceptive feedback is a critical component of motor control and learning (Ramos-Murguialday et al., 2012). Stroke patients present relatively well preserved afferent pathways that should be used to exploit functional neuroplastic mechanisms. A better understanding of how the motor networks are stimulated and combined feedback modalities (e.g., a robotic exoskeleton and electrical stimulation) might allow a more precise stimulation of the neural network involved in movement (Del-Ama et al., 2012; Hortal et al., 2015; Resquín et al., 2016). Furthermore, stimulation at the brain level might also address the pathological stroke-related interhemispheric imbalances, boost the effects of BMI therapies, and facilitate plasticity and recovery (Johnson et al., 2017).
The efficacy of the BMI intervention, as any other rehabilitative interventions, might also be increased by exciting the neural networks involved in the sensorimotor integration to facilitate functional neuroplasticity (Krakauer, Carmichael, Corbett, & Wittenberg, 2012). There are many groups working on cell therapies or brain stimulation as means to re-activate the neuroplasticity mechanisms that facilitate neuroplastic changes the first weeks after the stroke allowing spontaneous recovery. The combination of plasticity enhancement or reactivation methods with BMI therapy and physiotherapy strategies, hold great promise to boost stroke recovery interventions.
Conflict of interest
None to report.
Acknowledgments
This study was funded by the Bundesministerium für Bildung und Forschung BMBF MOTORBIC (FKZ 13GW0053) and AMORSA (FKZ 16SV7754), the Deutsche Forschungsgemeinschaft (DFG), the fortüne-Program of the University of Tübingen (2422-0-0 and 2452-0-0), and the Basque Government Science Program (EXOTEK: KK 2016/00083). NIL was supported by the Basque Government’s scholarship for predoctoral students.
References
1 | Ang, K. K. , Chua, K. S. G. , Phua, K. S. , Wang, C. , Chin, Z. Y. , Kuah, C. W. K. , & Guan, C. ((2015) ). A Randomized Controlled Trial of EEG-Based Motor Imagery Brain-Computer Interface Robotic Rehabilitation for Stroke. Clinical EEG and Neuroscience, 46: (4), 310–320. 10.1177/1550059414522229 |
2 | Ang, K. K. , Guan, C. , Phua, K. S. , Wang, C. , Zhou, L. , Tang, K. Y. , & Chua, K. S. G. ((2014) ). Brain-computer interface-based robotic end effector system for wrist and hand rehabilitation: Results of a three-armed randomized controlled trial for chronic stroke. Frontiers in Neuroengineering, 7: , 30. 10.3389/fneng.2014.00030 |
3 | Antelis, J. M. , Montesano, L. , Ramos-Murguialday, A. , Birbaumer, N. , & Minguez, J. ((2017) ). Decoding Upper Limb Movement Attempt from EEG Measurements of the Contralesional Motor Cortex in Chronic Stroke Patients. IEEE Transactions on Biomedical Engineering, 64: (1), 99–111. 10.1109/TBME.2016.2541084 |
4 | Asín Prieto, G. , Cano-de-la-Cuerda, R. , López-Larraz, E. , Metrot, J. , Molinari, M. , & van Dokkum, L. E. H. ((2014) ). Emerging perspectives in stroke rehabilitation. In Pons J. L. & Torricelli D. (Eds.), Emerging Therapies in Neurorehabilitation, (Vol. 1: , pp. 3–21). Berlin: Springer Berlin Heidelberg. 10.1007/978-3-642-38556-8 |
5 | Barsi, G. I. , Popovic, D. B. , Tarkka, I. M. , Sinkjaer, T. , & Grey, M. J. ((2008) ). Cortical excitability changes following grasping exercise augmented with electrical stimulation. Experimental Brain Research, 191: (1), 57–66. 10.1007/s00221-008-1495-5 |
6 | Bashashati, A. , Fatourechi, M. , Ward, R. K. , & Birch, G. E. ((2007) ). A survey of signal processing algorithms in brain-computer interfaces based on electrical brain signals. Journal of Neural Engineering, 4: (2), 32–57. 10.1088/1741-2560/4/2/R03 |
7 | Bashashati, H. , Ward, R. K. , Birch, G. E. , & Bashashati, A. ((2015) ). Comparing Different Classifiers in Sensory Motor Brain Computer Interfaces. PLoS One, 10: (6), e0129435. 10.1371/journal.pone.0129435 |
8 | Belda-Lois, J. M. , Mena-del Horno, S. , Bermejo-Bosch, I. , Moreno, J. C. , Pons, J. L. , Farina, D. , & Rea, M. ((2011) ). Rehabilitation of gait after stroke: A review towards a top-down approach. Journal of Neuroengineering and Rehabilitation, 8: (1), 66. 10.1186/1743-0003-8-66 |
9 | Bell, J. A. , Wolke, M. L. , Ortez, R. C. , Jones, T. A. , & Kerr, A. L. ((2015) ). Training Intensity Affects Motor Rehabilitation Efficacy Following Unilateral Ischemic Insult of the Sensorimotor Cortex in C57BL/6 Mice. Neurorehabilitation and Neural Repair, 29: (6), 590–598. 10.1177/1545968314553031 |
10 | Birbaumer, N. ((1999) ). Slow cortical potentials: Plasticity, operant control, and behoral effects. The Neuroscientist, 5: (2), 74–78. 10.1177/107385849900500211 |
11 | Birbaumer, N. , Ramos-Murguialday, A. , & Cohen, L. ((2008) ). Brain-computer interface in paralysis. Curr Opin Neurol, 21: (6), 634–638. 10.1097/WCO.0b013e328315ee2d |
12 | Borton, D. A. , Yin, M. , Aceros, J. , & Nurmikko, A. ((2013) ). An implantable wireless neural interface for recording cortical circuit dynamics in moving primates. Journal of Neural Engineering, 10: (2), 26010. 10.1088/1741-2560/10/2/026010 |
13 | Bouton, C. E. , Shaikhouni, A. , Annetta, N. V. , Bockbrader, M. A. , Friedenberg, D. A. , Nielson, D. M. , & Rezai, A. R. ((2016) ). Restoring cortical control of functional movement in a human with quadriplegia. Nature, 533: (7602), 247–250. 10.1038/nature17435 |
14 | Buch, E. , Weber, C. , Cohen, L. G. , Braun, C. , Dimyan, M. A. , Ard, T. , & Birbaumer, N. ((2008) ). Think to move: A neuromagnetic brain-computer interface (BCI) system for chronic stroke. Stroke, 39: , 910–917. 10.1161/STROKEAHA.107.505313 |
15 | Byblow, W. D. , Stinear, C. M. , Barber, P. A. , Petoe, M. A. , & Ackerley, S. J. ((2015) ). Proportional recovery after stroke depends on corticomotor integrity. Annals of Neurology, 78: (6), 848–859. 10.1002/ana.24472 |
16 | Carmena, J. M. ((2012) ). Becoming Bionic. IEEE Spectrum, 49: (3), 24–29, 10.1109/MSPEC.2012.6156860. |
17 | Carmena, J. M. , Lebedev, M. a. , Crist, R. E. , O’Doherty, J. E. , Santucci, D. M. , Dimitrov, D. F. , & Nicolelis, M. A. L. ((2003) ). Learning to control a brain-machine interface for reaching and grasping by primates. PLoS Biology, 1: (2), e42. 10.1371/journal.pbio.0000042 |
18 | Cesqui, B. , Tropea, P. , Micera, S. , & Krebs, H. ((2013) ). EMG-based pattern recognition approach in post stroke robot-aided rehabilitation: A feasibility study. Journal of NeuroEngineering and Rehabilitation, 10: (1), 75. 10.1186/1743-0003-10-75 |
19 | Chaudhary, U. , Birbaumer, N. , & Ramos-Murguialday, A. ((2016) ). Brain-computer interfaces for communication and rehabilitation. Nature Reviews Neurology, 12: (9), 513–525. 10.1038/nrneurol.2016.113 |
20 | Collinger, J. L. , Wodlinger, B. , Downey, J. E. , Wang, W. , Tyler-Kabara, E. C. , Weber, D. J. , & Schwartz, A. B. ((2013) ). High-performance neuroprosthetic control by an individual with tetraplegia. The Lancet, 381: (9866), 557–564. 10.1016/S0140-6736(12)61816-9 |
21 | Corbet, T. , Iturrate, I. , Pereira, M. , Perdikis, S. , & Millán, J. del R. ((2018) ). Sensory threshold neuromuscular electrical stimulation fosters motor imagery performance. Neuroimage, 176: , 268–276, 10.1016/j.neuroimage.2018.04.005 |
22 | Cramer, S. C. , Sur, M. , Dobkin, B. H. , O’Brien, C. , Sanger, T. D. , Trojanowski, J. Q. , & Vinogradov, S. ((2011) ). Harnessing neuroplasticity for clinical applications. Brain, 134: (6), 1591–1609. 10.1093/brain/awr039 |
23 | Daly, J. J. , & Wolpaw, J. R. ((2008) ). Brain-computer interfaces in neurological rehabilitation. Lancet Neurology, 7: (11), 1032–1043. 10.1016/S1474-4422(08)70223-0 |
24 | Dangi, S. , Orsborn, A. L. , Moorman, H. G. , & Carmena, J. M. ((2013) ). Design and Analysis of Closed-Loop Decoder Adaptation Algorithms for Brain-Machine Interfaces. Neural Computation, 25: (7), 1693–1731. 10.1162/NECO_a_00460 |
25 | Del-Ama, A. J. , Koutsou, A. D. , Moreno, J. C. , De-los-Reyes, A. , Gil-Agudo, Á. , & Pons, J. L. ((2012) ). Review of hybrid exoskeletons to restore gait following spinal cord injury. The Journal of Rehabilitation Research and Development, 49: (4), 497–514. 10.1682/JRRD.2011.03.0043 |
26 | Do, A. H. , Wang, P. T. , King, C. E. , Chun, S. N. , & Nenadic, Z. ((2013) ). Brain-computer interface controlled robotic gait orthosis. Journal of Neuroengineering and Rehabilitation, 10: , 111. 10.1186/1743-0003-10-111 |
27 | Enzinger, C. , Ropele, S. , Fazekas, F. , Loitfelder, M. , Gorani, F. , Seifert, T. , & Müller-Putz, G. ((2008) ). Brain motor system function in a patient with complete spinal cord injury following extensive brain-computer interface training. Experimental Brain Research, 190: (2), 215–223. 10.1007/s00221-008-1465-y |
28 | Fetz, E. E. ((1969) ). Operant Conditioning of Cortical Unit Activity. Science, 163: (3870), 955–958. 10.1126/science.163.3870.955 |
29 | Fetz, E. E. , & Baker, M. A. ((1973) ). Operantly conditioned patterns on precentral unit activity and correlated responses in adjacent cells and contralateral muscles. Journal of Neurophysiology, 36: (2), 179–204. 10.1152/jn.1973.36.2.179 |
30 | Frolov, A. A. , Mokienko, O. , Lyukmanov, R. , Biryukova, E. , Kotov, S. , Turbina, L. , & Bushkova, Y. ((2017) ). Post-stroke rehabilitation training with a motor-imagery-based brain-computer interface (BCI)-controlled hand exoskeleton: A randomized controlled multicenter trial. Frontiers in Neuroscience, 11: , 400. 10.3389/fnins.2017.00400 |
31 | Ganguly, K. , Byl, N. N. , & Abrams, G. M. ((2013) ). Neurorehabilitation: Motor recovery after stroke as an example. Annals of Neurology, 74: (3), 373–381. 10.1002/ana.23994 |
32 | Ganguly, K. , Secundo, L. , Ranade, G. , Orsborn, A. , Chang, E. F. , Dimitrov, D. F. , & Carmena, J. M. ((2009) ). Cortical representation of ipsilateral arm movements in monkey and man. The Journal of Neuroscience, 29: (41), 12948–12956. 10.1523/JNEUROSCI.2471-09.2009 |
33 | García-Cossio, E. , Broetz, D. , Birbaumer, N. , & Ramos-Murguialday, A. ((2014) ). Cortex integrity relevance in muscle synergies in severe chronic stroke. Frontiers in Human Neuroscience, 8: , 744. 10.3389/fnhum.2014.00744 |
34 | Gazzaniga, M. S. ((2006) ), The Cognitive Neurosciences, MIT press, 10.1136/bmj.312.7024.193 |
35 | Georgopoulos, A. P. , Schwartz, A. B. , & Kettner, R. E. ((1986) ). Neuronal population coding of movement direction. Science, 233: (1986), 1416–1419. 10.1126/science.3749885 |
36 | Gould, E. , Tanapat, P. , Hastings, N. B. , & Shors, T. J. ((1999) ). Neurogenesis in adulthood: A possible role in learning. Trends in Cognitive Sciences, 3: (5), 186–192. 10.1016/S1364-6613(99)01310-8 |
37 | Grozea, C. , Voinescu, C. D. , & Fazli, S. ((2011) ). Bristle-sensors— low-cost flexible passive dry EEG electrodes for neurofeedback and BCI applications. Engineering Journal of Neural, 8: (2), 25008. 10.1088/1741-2560/8/2/025008 |
38 | Hebb, D. O. ((1949) ) The organization of behor: A neuropsychological theory, New York: John Wiley & Sons, 10.2307/1418888 |
39 | Hesse, S. , Werner, C. , Pohl, M. , Rueckriem, S. , Mehrholz, J. , & Lingnau, M. L. ((2005) ). Computerized arm training improves the motor control of the severely affected arm after stroke: A single-blinded randomized trial in two centers. Stroke, 36: (9), 1960–1966. 10.1161/01.STR.0000177865.37334.ce |
40 | Hirata, M. , Matsushita, K. , Suzuki, T. , Yoshida, T. , Sato, F. , Morris, S. , & Yoshimine, T. ((2011) ). A fully-implantable wireless system for human brain-machine interfaces using brain surface electrodes: W-HERBS. IEICE Trans- 1181 actions on Communications, E94: -B: (9), 2448–2453. 10.1587/transcom.E94.B.2448 |
41 | Hochberg, L. R. , Bacher, D. , Jarosiewicz, B. , Masse, N. Y. , Simeral, J. D. , Vogel, J. , & Donoghue, J. P. ((2012) ). Reach and grasp by people with tetraplegia using a neurally controlled robotic arm. Nature, 485: (7398), 372–375. 10.1038/nature11076 |
42 | Hochberg, L. R. , Serruya, M. D. , Friehs, G. M. , Mukand, J. A. , Saleh, M. , Caplan, A. H. , & Donoghue, J. P. ((2006) ). Neuronal enble control of prosthetic devices by a human with tetraplegia. Nature, 442: (7099), 164–171. 10.1038/nature04970 |
43 | Hogan, N. , Krebs, H. I. , Charnnarong, J. , Srikrishna, P. , & Sharon, A. ((1992) ). MIT - MANUS: A Workstation for Manual Therapy and Training I., In IEEE International Workshop on Robot and Human Communication(pp. 161–165), 10.1109/ROMAN.1992.253895 |
44 | Hortal, E. , Planelles, D. , Resquin, F. , Climent, J. M. , Azorin, J. M. , & Pons, J. L. ((2015) ). Using a brain-machine interface to control a hybrid upper limb exoskeleton during rehabilitation of patients with neurological conditions. Journal of Neuroengineering and Rehabilitation, 12: , 92. 10.1186/s12984-015-0082-9 |
45 | Ibáñez, J. , Monge-Pereira, E. , Molina-Rueda, F. , Serrano, J. I. , del Castillo, M. D. , Cuesta-Gómez, A. , & Pons, J. L. ((2017) ). Low Latency Estimation of Motor Intentions to Assist Reaching Movements along Multiple Sessions in Chronic Stroke Patients: A Feasibility Study. Frontiers in Neuroscience, 11: , 126. 10.3389/fnins.2017.00126 |
46 | Ibáñez, J. , Serrano, J. I. , del Castillo, M. D. , Monge-Pereira, E. , Molina-Rueda, F. , Alguacil-Diego, I. , & Pons, J. L. ((2014) ). Detection of the onset of upper-limb movements based on the combined analysis of changes in the sensorimotor rhythms and slow cortical potentials. Journal of Neural Engineering, 11: (5), 56009. 10.1088/1741-2560/11/5/056009 |
47 | Insausti-Delgado, A. , López-Larraz, E. , Bibián, C. , Nishimura, Y. , Birbaumer, N. , & Ramos-Murguialday, A. ((2017) ). Influence of trans-spinal magnetic stimulation in electrophysiological recordings for closed-loop rehabilitative systems, In 39th Annual International Conference of the IEEE Engineering in Medicine and Biology Society (EMBC), 2518–2521. 10.1109/EMBC.2017.8037369 |
48 | Irastorza-Landa, N. , Sarasola-Sanz, A. , López-Larraz, E. , Bibián, C. , Shiman, F. , Birbaumer, N. , & Ramos-Murguialday, A. ((2017) ). Design of Continuous EMG Classification approaches towards the Control of a Robotic Exoskeleton in Reaching Movements. In IEEE International Conference on Rehabilitation Robotics, 128–133. 10.1109/ICORR.2017.8009234 |
49 | Iturrate, I. , Chavarriaga, R. , Montesano, L. , Minguez, J. , & Millán, J. D. R. ((2015) ). Teaching brain-machine interfaces as an alternative paradigm to neuroprosthetics control. Scientific Reports, 5: , 13893. 10.1038/srep13893 |
50 | Jackson, A. , & Zimmermann, J. B. ((2012) ). Neural interfaces for the brain and spinal cord— restoring motor function. Nature Reviews Neurology, 8: (12), 690–699. 10.1038/nrneurol.2012.219 |
51 | Jiang, N. , Gizzi, L. , Mrachacz-Kersting, N. , Dremstrup, K. , & Farina, D. ((2015) ). A brain-computer interface for single-trial detection of gait initiation from movement related cortical potentials. Clinical Neurophysiology, 126: (1), 154–159. 10.1016/j.clinph.2014.05.003 |
52 | Johnson, N. N. , Carey, J. , Edelman, B. J. , Doud, A. , Grande, A. , Lakshminarayan, K. , & He, B. ((2017) ). Combined rTMS and virtual reality brain-computer interface training for motor recovery after stroke. Journal of Neural Engineering, 15: (1), 016009. 10.1088/1741-2552/aa8ce3 |
53 | Kasashima-Shindo, Y. , Fujiwara, T. , Ushiba, J. , Matsushika, Y. , Kamatani, D. , Oto, M. , & Liu, M. ((2015) ). Brain - Computer Interface Training Combined With Transcranial Direct Current Stimulation in Patients With Chronic Severe Hemiparesis: Proof of Concept Study. Journal of Rehabilitation Medicine, 47: (4), 318–324. 10.2340/16501977-1925 |
54 | Kiguchi, K. , & Hayashi, Y. ((2012) ). A study of EMG and EEG during perception-assist with an upper-limb power-assist robot. In IEEE International Conference on Robotics and Automation, 2711–2716. 10.1109/ICRA.2012.6225027 |
55 | Kiguchi, K. , Lalitharatne, T. D. , & Hayashi, Y. ((2013) ). Estimation of Forearm Supination/Pronation Motion Based on EEG Signals to Control an Artificial Arm. Journal of Advanced Mechanical Design, Systems, and Manufacturing, 7: (1), 74–81. 10.1299/jamdsm.7.74 |
56 | Kim, T. , Kim, S. , & Lee, B. ((2016) ). Effects of Action Observational Training Plus Brain-Computer Interface-Based Functional Electrical Stimulation on Paretic Arm Motor Recovery in Patient with Stroke: A Randomized Controlled Trial. Occupational Therapy International, 23: (1), 39–47. 10.1002/oti.1403 |
57 | King, C. E. , Wang, P. T. , McCrimmon, C. M. , Chou, C. C. , Do, A. H. , & Nenadic, Z. ((2015) ). The feasibility of a brain-computer interface functional electrical stimulation system for the restoration of overground walking after paraplegia. Journal of NeuroEngineering and Rehabilitation, 12: (1), 80. 10.1186/s12984-015-0068-7 |
58 | Kolominsky-Rabas, P. L. , Heuschmann, P. U. , Marschall, D. , Emmert, M. , Baltzer, N. , Neundörfer, B. , & Krobot, K. J. ((2006) ). Lifetime cost of ischemic stroke in Germany: Results and national projections from a population-based stroke registry. Stroke, 37: (5), 1179–1183. 10.1161/01.STR.0000217450.21310.90 |
59 | Krakauer, J. W. , Carmichael, S. T. , Corbett, D. , & Wittenberg, G. F. ((2012) ). Getting Neurorehabilitation Right: What Can Be Learned From Animal Models?. Neurorehabilitation and Neural Repair, 26: (8), 923–931. 10.1177/1545968312440745 |
60 | Krebs, H. I. , Palazzolo, J. J. , Dipietro, L. , Ferraro, M. , Krol, J. , Rannekleiv, K. , & Hogan, N. ((2003) ). Rehabilitation robotics: Performance-based progressive robot-assisted therapy. Autonomous Robots, 15: (1), 7–20. 10.1023/A:1024494031121 |
61 | Kwakkel, G. , Kollen, B. J. , & Krebs, H. I. ((2008) ). Effects of robot-assisted therapy on upper limb recovery after stroke: A systematic review. Neurorehabilitation and Neural Repair, 22: (2), 111–21. 10.1177/1545968307305457 |
62 | Kwakkel, G. , Van Peppen, R. , Wagenaar, R. C. , Dauphinee, S. W. , Richards, C. , Ashburn, A. , & Langhorne, P. ((2004) ). Effects of augmented exercise therapy time after stroke: A meta-analysis. Stroke, 35: (11), 2529–2536. 10.1161/01.STR.0000143153.76460.7d |
63 | Langhorne, P. , Bernhardt, J. , & Kwakkel, G. ((2011) ). Stroke rehabilitation. The Lancet, 377: (9778), 1693–1702. 10.1016/S0140-6736(11)60325-5 |
64 | Lebedev, M. A. , & Nicolelis, M. A. L. ((2017) ). Brain-Machine Interfaces: From Basic Science to Neuroprostheses and Neurorehabilitation. Physiological Reviews, 97: (2), 767–837, 10.1152/physrev.00027.2016. |
65 | Lee, H. Y. , Hwang, J. S. , Jeng, J. S. , & Wang, J. D. ((2010) ). Quality-adjusted life expectancy (QALE) and loss of qale for patients with ischemic stroke and intracerebral hemorrhage: A 13-year follow-up. Stroke, 41: (4), 739–744. 10.1161/STROKEAHA.109.573543 |
66 | Lee, K. , Liu, D. , Perroud, L. , Chavarriaga, R. , Millán, J. , & del, R. ((2017) ). A brain-controlled exoskeleton with cascaded event-related desynchronization classifiers. Robotics and Autonomous Systems, 90: , 15–23. 10.1016/j.robot.2016.10.005 |
67 | Lee, S. , Shin, Y. , Woo, S. , Kim, K. , & Lee, H.-N. ((2013) ). Review of Wireless Brain-Computer Interface Systems. In Fazel-Rezai R. (Ed.), In Tech Brain-Computer Interface Systems-Recent Progress and Future Prospects, 215–238. 10.5772/67458 |
68 | Leeb, R. , Sagha, H. , Chavarriaga, R. , Millán, J. , & del, R. ((2011) ). A hybrid brain-computer interface based on the fusion of electroencephalographic and electromyographic activities. Journal of Neural Engineering, 8: (2), 25011. 10.1088/1741-2560/8/2/025011 |
69 | Li, M. , Liu, Y. , Wu, Y. , Liu, S. , Jia, J. , & Zhang, L. ((2014) ). Neurophysiological substrates of stroke patients with motor imagery-based brain-computer interface training. International Journal of Neuroscience, 124: (6), 403–415. 10.3109/00207454.2013.850082 |
70 | Li, X. , Samuel, O. W. , Zhang, X. , Wang, H. , Fang, P. , & Li, G. ((2017) ). A motion-classification strategy based on G-EEG signal combination for upper-limb amputees. Journal of NeuroEngineering and Rehabilitation, 14: (1), 2. 10.1186/s12984-016-0212-z |
71 | Lin, K. , Chang, Y. , Wu, C. , & Chen, Y. ((2009) ). Effects of constraint-induced therapy versus bilateral arm training on motor performance, daily functions, and quality of life in stroke survivors. Neurorehabilitation and Neural Repair, 23: (5), 441–448. 10.1177/1545968308328719 |
72 | Lohse, K. , Shirzad, N. , Verster, A. , Hodges, N. , & Van der Loos, H. M. ((2013) ). Video games and rehabilitation: Using design principles to enhance engagement in physical therapy. Journal of Neurologic Physical Therapy, 37: (4), 166–175. 10.1097/NPT.0000000000000017 |
73 | López-Larraz, E. , Bibián, C. , Birbaumer, N. , & Ramos-Murguialday, A. ((2017) ). Influence of artifacts on movement intention decoding from EEG activity in severely paralyzed stroke patients, 15th International Conference Rehabilitation Robotics (ICORR), 901–906. 10.1109/ICORR.2017.8009363 |
74 | López-Larraz, E. , Ibáñez, J. , Trincado-Alonso, F. , Monge-Pereira, E. , Pons, J. L. , & Montesano, L. ((2018) ). Comparing recalibration strategies for electroencephalography-based decoders of movement intention in neurological patients with motor disability. International Journal of Neural Systems, 28: (7), 10.1142/S0129065717500605 |
75 | López-Larraz, E. , Montesano, L. , Gil-Agudo, Á. , & Minguez, J. ((2014) ). Continuous decoding of movement intention of upper limb self-initiated analytic movements from pre-movement EEG correlates. Journal of Neuroengineering and Rehabilitation, 11: (1), 153. 10.1186/1743-0003-11-153 |
76 | López-Larraz, E. , Ray, A. M. , Figueiredo, T. C. , Bibián, C. , Birbaumer, N. , & Ramos-Murguialday, A. ((2017) ). Stroke lesion location influences the decoding of movement intention from EEG. In 39th Annual International Conference of the IEEE Engineering in Medicine and Biology Society (EMBC), 3065–3068. 10.1109/EMBC.2017.8037504 |
77 | López-Larraz, E. , Trincado-Alonso, F. , Rajasekaran, V. , Del-Ama, A. J. , Aranda, J. , Minguez, J. , & Montesano, L. ((2016) ). Control of an ambulatory exoskeleton with a brain-machine interface for spinal cord injury gait rehabilitation. Frontiers in Neuroscience, 10: , 359. 10.3389/fnins.2016.00359 |
78 | Lotte, F. , Congedo, M. , Lécuyer, A. , Lamarche, F. , & Arnaldi, B. ((2007) ). A review of classification algorithms for EEG-based brain-computer interfaces. Journal of Neural Engineering, 4: (2), R1–3. 10.1088/1741-2560/4/2/R01 |
79 | Lucas, T. H. , & Fetz, E. E. ((2013) ). Myo-cortical crossed feedback reorganizes primate motor cortex output. The Journal of Neuroscience, 33: (12), 5261–5274. 10.1523/JNEUROSCI.4683-12.2013 |
80 | Luft, A. R. , McCombe-Waller, S. , Whitall, J. , Forrester, L. W. , Macko, R. , Sorkin, J. D. , & Hanley, D. F. ((2004) ). Repetitive bilateral arm training and motor cortex activation in chronic stroke: A randomized controlled trial. JAMA: The Journal of the American Medical Association, 292: (15), 1853–61. 10.1001/jama.292.15.1853 |
81 | Lynch, C. L. , & Popovic, M. R. ((2008) ). Functional electrical stimulation. IEEE Control Systems Magazine, 28: (2), 40–50. 10.1109/MCS.2007.914689 |
82 | McFarland, D. J. , Sarnacki, W. A. , & Wolpaw, J. R. ((2010) ). Electroencephalographic (EEG) control of three-dimensional movement. Journal of Neural Engineering, 7: (3), 36007. 10.1088/1741-2560/7/3/036007 |
83 | McPherson, J. G. , Miller, R. R. , & Perlmutter, S. I. ((2015) ). Targeted, activity-dependent spinal stimulation produces long-lasting motor recovery in chronic cervical spinal cord injury. Proceedings of the National Academy of Sciences, 112: (39), 12193–12198. 10.1073/pnas.1505383112 |
84 | Mestais, C. S. , Charvet, G. , Sauter-Starace, F. , Foerster, M. , Ratel, D. , & Benabid, A. L. ((2015) ). WIMAGINE: Wireless 64-channel ECoG recording implant for long term clinical applications. IEEE Transactions on Neural Systems and Rehabilitation Engineering, 23: (1), 10–21. 10.1109/TNSRE.2014.2333541 |
85 | Mihara, M. , Hattori, N. , Hatakenaka, M. , Yagura, H. , Kawano, T. , Hino, T. , & Miyai, I. ((2013) ). Near-infrared spectroscopy-mediated neurofeedback enhances efficacy of motor imagery-based training in poststroke victims: A pilot study. Stroke, 44: (4), 1091–1098. 10.1161/STROKEAHA.111.674507 |
86 | Millán, J. del R. , Rupp, R. , Müller-Putz, G. R. , Murray-Smith, R. , Giugliemma, C. , Tangermann, M. , & Mattia, D. ((2010) ). Combining brain-computer interfaces and assistive technologies: State-of-the-art and challenges. Frontiers in Neuroscience, 4: , 161. 10.3389/fnins.2010.00161 |
87 | Mrachacz-Kersting, N. , Jiang, N. , Stevenson, A. J. T. , Niazi, I. K. , Kostic, V. , Pavlovic, A. , & Farina, D. ((2016) ). Efficient neuroplasticity induction in chronic stroke patients by an associative brain-computer interface. Journal of Neurophysiology, 115: (3), 1410–1421. 10.1152/jn.00918.2015 |
88 | Mrachacz-Kersting, N. , Kristensen, S. R. , Niazi, I. K. , & Farina, D. ((2012) ). Precise temporal association between cortical potentials evoked by motor imagination and afference induces cortical plasticity. The Journal of Physiology, 590: (7), 1669–1682. 10.1113/jphysiol.2011.222851 |
89 | Mukaino, M. , Ono, T. , Shindo, K. , Fujiwara, T. , Ota, T. , Kimura, A. , & Ushiba, J. ((2014) ). Efficacy of brain-computer interface-driven neuromuscular electrical stimulation for chronic paresis after stroke. Journal of Rehabilitation Medicine, 46: , 378–382. 10.2340/16501977-1785 |
90 | Murphy, T. H. , & Corbett, D. ((2009) ). Plasticity during stroke recovery: From synapse to behour. Nature Reviews Neuroscience, 10: (12), 861–872. 10.1038/nrn2735 |
91 | Niazi, I. K. , Jiang, N. , Tiberghien, O. , Nielsen, J. F. , Dremstrup, K. , & Farina, D. ((2011) ). Detection of movement intention from single-trial movement-related cortical potentials. Journal of Neural Engineering, 8: (6), 66009. 10.1088/1741-2560/8/6/066009 |
92 | Nishimura, Y. , Perlmutter, S. I. , Eaton, R. W. , & Fetz, E. E. ((2013) ). Spike-timing-dependent plasticity in primate corticospinal connections induced during free behor. Neuron, 80: (5), 1301–1309. 10.1016/j.neuron.2013.08.028 |
93 | Ofner, P. , Schwarz, A. , Pereira, J. , & Müller-Putz, G. R. ((2017) ). Upper limb movements can be decoded from the time-domain of low-frequency EEG. PLoS One, 12: (8), e0182578. 10.1371/journal.pone.0182578 |
94 | Ono, T. , Shindo, K. , Kawashima, K. , Ota, N. , Ito, M. , Ota, T. , & Ushiba, J. ((2014) ). Brain-computer interface with somatosensory feedback improves functional recovery from severe hemiplegia due to chronic stroke. Frontiers in Neuroengineering, 7: , 19. 10.3389/fneng.2014.00019 |
95 | Orsborn, A. L. , Dangi, S. , Moorman, H. G. , & Carmena, J. M. ((2012) ). Closed-loop decoder adaptation on intermediate time-scales facilitates rapid BMI performance improvements independent of decoder initialization conditions. IEEE Transactions on Neural Systems and Rehabilitation Engineering, 20: (4), 468–477. 10.1109/TNSRE.2012.2185066 |
96 | Oweiss, K. G. , & Badreldin, I. S. ((2015) ). Neuroplasticity subserving the operation of brain machine interfaces. Neurobiology of Disease, 83: , 161–171. 10.1111/nyas.12666.Saccadic |
97 | Park, W. , Kwon, G. H. , Kim, Y.-H. , Lee, J.-H. , & Kim, L. ((2016) ). EEG response varies with lesion location in patients with chronic stroke. Journal of NeuroEngineering and Rehabilitation, 13: (1), 21. 10.1186/s12984-016-0120-2 |
98 | Parker, P. , Englehart, K. , & Hudgins, B. ((2006) ). Myoelectric signal processing for control of powered limb prostheses. Journal of Electromyography and Kinesiology, 16: (6), 541–548. 10.1016/j.jelekin.2006.08.006 |
99 | Pfurtscheller, G. , Guger, C. , Müller, G. , Krausz, G. , & Neuper, C. ((2000) ). Brain oscillations control hand orthosis in a tetraplegic. Neuroscience Letters, 292: (3), 211–214. 10.1016/S0304-3940(00)01471-3 |
100 | Pfurtscheller, G. , Müller, G. R. , Pfurtscheller, J. , Gerner, H. J. , & Rupp, R. ((2003) ). “Thought” - Control of functional electrical stimulation to restore hand grasp in a patient with tetraplegia. Neuroscience Letters, 351: (1), 33–36. 10.1016/S0304-3940(03)00947-9 |
101 | Pichiorri, F. , Morone, G. , Petti, M. , Toppi, J. , Pisotta, I. , Molinari, M. , & Mattia, D. ((2015) ). Brain-computer interface boosts motor imagery practice during stroke recovery. Annals of Neurology, 77: (5), 851–865. 10.1002/ana.24390 |
102 | Pistohl, T. , Schulze-Bonhage, A. , Aertsen, A. , Mehring, C. , & Ball, T. ((2012) ). Decoding natural grasp types from human ECoG. NeuroImage, 59: (1), 248–260. 10.1016/j.neuroimage.2011.06.084 |
103 | Quandt, F. , & Hummel, F. C. ((2014) ). The influence of functional electrical stimulation on hand motor recovery in stroke patients: A review.&. Translational Stroke Medicine, 6: , 9. 10.1186/2040-7378-6-9 |
104 | Ramos-Murguialday, A. , Broetz, D. , Rea, M. , Läer, L. , Yilmaz, O. , Brasil, F. L. , & Birbaumer, N. ((2013) ). Brain-machine interface in chronic stroke rehabilitation: A controlled study. Annals of Neurology, 74: (1), 100–108. 10.1002/ana.23879 |
105 | Ramos-Murguialday, A. , García-Cossio, E. , Walter, A. , Cho, W. , Broetz, D. , Bogdan, M. , & Birbaumer, N. ((2015) ). Decoding upper limb residual muscle activity in severe chronic stroke. Annals of Clinical and Translational Neurology, 2: (1), 1–11. 10.1002/acn3.122 |
106 | Ramos-Murguialday, A. , Hill, J. , Bensch, M. , Martens, S. , Halder, S. , Nijboer, F. , & Gharabaghi, A. ((2011) ). Transition from the locked in to the completely locked-in state: A physiological analysis. Clinical Neurophysiology, 122: (5), 925–933. 10.1016/j.clinph.2010.08.019 |
107 | Ramos-Murguialday, A. , Schürholz, M. , Caggiano, V. , Wildgruber, M. , Caria, A. , Hammer, E. M. , & Birbaumer, N. ((2012) ). Proprioceptive feedback and brain computer interface (BCI) based neuroprostheses. e. PLoS One, 7: (10), 47048. 10.1371/journal.pone.0047048 |
108 | Ray, A. M. , López-Larraz, E. , Figueiredo, T. C. , Birbaumer, N. , & Ramos-Murguialday, A. ((2017) ). Movement-related brain oscillations vary with lesion location in severely paralyzed chronic stroke patients. In 39th Annual International Conference of the IEEE Engineering in Medicine and Biology Society (EMBC), 1664–1667. 10.1109/EMBC.2017.8037160 |
109 | Rayegani, S. M. , Raeissadat, S. A. , Sedighipour, L. , Mohammad Rezazadeh, I. , Bahrami, M. H. , Eliaspour, D. , & Khosrawi, S. ((2014) ). Effect of Neurofeedback and Electromyographic-Biofeedback Therapy on Improving Hand Function in Stroke Patients. Topics in Stroke Rehabilitation, 21: (2), 137–151. 10.1310/tsr2102-137 |
110 | Resquín, F. , Ibañez, J. , Gonzalez-Vargas, J. , Dimbwadyo, I. , Carrasco, L. , Alves, S. , & Pons, J. L. ((2016) ). Combining a Hybrid Robotic System with a Bain-Machine Interface for the Rehabilitation of Reaching Movements: A case study with a Stroke Patient, 38th International Conference of the IEEE Engineering in Medicine and Biology Society (EMBC), 6381–6384. 10.1109/EMBC.2016.7592188 |
111 | Ron-Angevin, R. , & Díaz-Estrella, A. ((2009) ). Brain-computer interface: Changes in performance using virtual reality techniques. Neuroscience Letters, 449: (2), 123–127. 10.1016/j.neulet.2008.10.099 |
112 | Rupp, R. ((2014) ). Challenges in clinical applications of brain computer interfaces in individuals with spinal cord injury. Frontiers in Neuroengineering, 7: , 38. 10.3389/fneng.2014.00038 |
113 | Rupp, R. ((2017) ). Brain-Computer Interfaces for Motor Rehabilitation. In Müller B., Wolf S. I., Brueggemann G.-P., Deng Z., McIntosh A., Miller F., & Selbie W. S. (Eds.), Handbook of Human Motion, (pp. 1–31), 10.1007/978-3-319-30808-1 |
114 | Sampaio-Baptista, C. , Khrapitchev, A. A. , Foxley, S. , Schlagheck, T. , Scholz, J. , Jbabdi, S. , Johansen-Berg H. ((2013) ). Motor skill learning induces changes in white matter microstructure and myelination. The Journal of Neuroscience, 33: (50), 19499–19503. 10.1523/jneurosci.3048-13.2013 |
115 | Sarasola-Sanz, A. , Irastorza-Landa, N. , López-Larraz, E. , Bibián, C. , Helmhold, F. , Broetz, D. , Ramos-Murguialday A. ((2017) ). A Hybrid Brain-Machine Interface based on EEG and EMG activity for the Motor Rehabilitation of Stroke Patients. In th International Conference on Rehabilitation Robotics (ICORR), 895–900. 10.1109/ICORR.2017.8009362 |
116 | Sarasola-Sanz, A. , Irastorza-Landa, N. , Shiman, F. , López-Larraz, E. , Spüler, M. , Birbaumer, N. , & Ramos-Murguialday, A. ((2015) ). EMG-based multi-joint kinematics decoding for robot-aided rehabilitation therapies. In 14th International Conference on Rehabilitation Robotics (ICORR), 229–234. 10.1109/ICORR.2015.7281204 |
117 | Sarasola-Sanz, A. , López-Larraz, E. , Irastorza-Landa, N. , Klein, J. , Valencia, D. , Belloso, A. , Ramos-Murguialday A. ((2016) ). An EEG-based brain-machine interface to control a 7-degrees of freedom exoskeleton for stroke rehabilitation. In International Conference on NeuroRehabilitation (ICNR). 10.1007/978-3-319-46669-9_183 |
118 | Sburlea, A. I. , Montesano, L. , de la Cuerda, R. C. , Alguacil Diego, I. M. , Miangolarra-Page, J. C. , & Minguez, J. ((2015) ). Detecting intention to walk in stroke patients from pre-movement EEG correlates. Journal of NeuroEngineering and Rehabilitation, 12: (1), 113. 10.1186/s12984-015-0087-4 |
119 | Schalk, G. , Miller, K. J. , Anderson, N. R. , Wilson, J. A. , Smyth, M. D. , Ojemann, J. G. , & Leuthardt, E. C. ((2008) ). Two-dimensional movement control using electrocorticographic signals in humans. Journal of Neural Engineering, 5: (1), 75–84. 10.1088/1741-2560/5/1/008 |
120 | Schalk, G. , Kubánek, J. , Miller, K. J. , Anderson N. R., Leuthardt E. C., Ojemann J. G., . . . Wolpaw, J. R. ((2007) ). Decoding two-dimensional movement trajectories using electrocorticographic signals in humans. Journal of Neural Engineering, 4: (3), 274–275. 10.1088/1741-2560/4/3/012 |
121 | Scherer, R. , Graimann, B. , Huggins, J. E. , Levine, S. P. , & Pfurtscheller, G. ((2003) ). Frequency component selection for an ECoG-based brain-computer interface. Biomedizinische Technik. Biomedical Engineering, 48: , 31–36. 10.1515/bmte.2003.48.1-2.31 |
122 | Schuettler, M. , Kohler, F. , Ordonez, J. S. , & Stieglitz, T. ((2012) ). Hermetic electronic packaging of an implantable brain-machine-interface with transcutaneous optical data communication. In 34th Annual International Conference of the IEEE Engineering in Medicine and Biology Society (EMBC), 3886–3889. 10.1109/EMBC.2012.6346816 |
123 | Shiman, F. , López-Larraz, E. , Sarasola-Sanz, A. , Irastorza-Landa, N. , Spueler, M. , Birbaumer, N. , & Ramos-Murguialday, A. ((2017) ). Classification of different reaching movements from the same limb using EEG. Journal of Neural Engineering, 14: (4), 046018. 10.1088/1741-2552/aa70d2 |
124 | Sitaram, R. , Zhang, H. , Guan, C. , Thulasidas, M. , Hoshi, Y. , Ishikawa, A. , & Birbaumer, N. ((2007) ). Temporal classification of multichannel near-infrared spectroscopy signals of motor imagery for developing a brain-computer interface. NeuroImage, 34: (4), 1416–1427. 10.1016/j.neuroimage.2006.11.005 |
125 | Spüler, M. , Walter, A. , Ramos-Murguialday, A. , Naros, G. , Birbaumer, N. , Gharabaghi, A. , & Bogdan, M. ((2014) ). Decoding of motor intentions from epidural ECoG recordings in severely paralyzed chronic stroke patients. Journal of Neural Engineering, 11: (6), 66008. 10.1088/1741-2560/11/6/066008 |
126 | Stepień M. , Conradi, J. , Waterstraat, G. , Hohlefeld, F. U. , Curio, G. , & Nikulin, V. V. ((2011) ). Event-related desynchronization of sensorimotor EEG rhythms in hemiparetic patients with acute stroke. Neuroscience Letters, 488: (1), 17–21. 10.1016/j.neulet.2010.10.072 |
127 | Stinear, C. M. , & Byblow, W. D. ((2014) ). Predicting and accelerating motor recovery after stroke. Current Opinion in Neurology, 27: (6), 624–630. 10.1097/WCO.0000000000000153 |
128 | Suner, S. , Fellows, M. R. , Vargas-Irwin, C. , Nakata, G. K. , & Donoghue, J. P. ((2005) ). Reliability of signals from a chronically implanted, silicon-based electrode array in non-human primate primary motor cortex. IEEE Transactions on Neural Systems and Rehabilitation Engineering, 13: (4), 524–541. 10.1109/TNSRE.2005.857687 |
129 | Taub, E. , Crago, J. E. , & Uswatte, G. ((1998) ). Constraint-induced movement therapy: A new approach to treatment in physical rehabilitation. Rehabilitation Psychology, 43: (2), 152–170. 10.1037/0090-5550.43.2.152 |
130 | Tenore, F. , Ramos, A. , Fahmy, A. , Acharya, S. , Etienne-Cummings, R. , & Thakor, N. V. ((2009) ). Decoding of individuated finger movements using surface Electromyography. IEEE Transactions on Biomedical Engineering, 56: (5), 1427–1434. 10.1109/TBME.2008.2005485 |
131 | Trincado-Alonso, F. , López-Larraz, E. , Resquín, F. , Ardanza, A. , Pérez-Nombela, S. , Pons, J. L. , & Gil-Agudo, Á. ((2017) ). A pilot study of brain-triggered electrical stimulation with visual feedback in patients with incomplete spinal cord injury. Journal of Medical and Biological Engineering, 10.1007/s40846-017-0343-0 |
132 | Turner, D. L. , Ramos-Murguialday, A. , Birbaumer, N. , Hoffmann, U. , & Luft, A. ((2013) ). Neurophysiology of robot-mediated training and therapy: A perspective for future use in clinical populations. Frontiers in Neurology, 4: , 184. 10.3389/fneur.2013.00184 |
133 | Waldert, S. ((2016) ). Invasive vs. non-invasive neuronal signals for brain-machine interfaces: Will one prevail?. Frontiers in Neuroscience, 10: , 295. 10.3389/fnins.2016.00295 |
134 | Walter, A. , Ramos-Murguialday, A. , Spüler, M. , Naros, G. , Leatilde;o, M. T. , Gharabaghi, A. , & Bogdan, M. ((2012) ). Coupling BCI and cortical stimulation for brain-state-dependent stimulation: Methods for spectral estimation in the presence of stimulation after-effects. Frontiers in Neural Circuits, 6: , 87. 10.3389/fncir.2012.00087 |
135 | Ward, N. S. , & Cohen, L. G. ((2004) ). Mechanisms underlying recovery of motor function after stroke. Archives of Neurology, 61: (12), 1844–1848. 10.1136/pgmj.2004.030809 |
136 | Weiskopf, N. , Veit, R. , Erb, M. , Mathiak, K. , Grodd, W. , Goebel, R. , & Birbaumer, N. ((2003) ). Physiological self-regulation of regional brain activity using real-time functional magnetic resonance imaging (fMRI): Methodology and exemplary data. NeuroImage, 19: (3), 577–586. 10.1016/S1053-8119(03)00145-9 |
137 | Weiss, P. L. , Kizony, R. , Feintuch, U. , & Katz, N. ((2006) ). Virtual Reality in Neurorehabilitation. In(Vol.,). Textbook of neural repair and rehabilitation, 182–197. 10.4172/2376-0281.1000e117 |
138 | Winters, C. , van Wegen, E. E. H. , Daffertshofer, A. , & Kwakkel, G. ((2015) ). Generalizability of the Proportional Recovery Model for the Upper Extremity After an Ischemic Stroke. Neurorehabilitation and Neural Repair, 29: (7), 614–622. 10.1177/1545968314562115 |
139 | Wolf, S. L. , Winstein, C. J. , Miller, J. P. , Thompson, P. A. , Taub, E. , Uswatte, G. , & Clark, P. C. ((2008) ). Retention of upper limb function in stroke survivors who have received constraint-induced movement therapy: The EXCITE randomised trial. The Lancet Neurology, 7: (1), 33–40. 10.1016/S1474-4422(07)70294-6 |
140 | Wolpaw, J. R. , Birbaumer, N. , McFarland, D. J. , Pfurtscheller, G. , & Vaughan, T. M. ((2002) ). Brain-computer interfaces for communication and control. Clinical Neurophysiology, 113: (6), 767–791. 10.1016/S1388-2457(02)00057-3 |
141 | Wu, X. , Guarino, P. , Lo, A. C. , Peduzzi, P. , & Wininger, M. ((2016) ). Long-term Effectiveness of Intensive Therapy in Chronic Stroke. Neurorehabilitation and Neural Repair, 30: (6), 583–590. 10.1177/1545968315608448 |
142 | Zander, T. O. , Lehne, M. , Ihme, K. , Jatzev, S. , Correia, J. , Kothe, C. , & Nijboer, F. ((2011) ). A dry EEG-system for scientific research and brain-computer interfaces. Frontiers in Neuroscience, 5: , 53. 10.3389/fnins.2011.00053 |
143 | Zecca, M. , Micera, S. , Carrozza, M. C. , & Dario, P. ((2002) ). Control of Multifunctional Prosthetic Hands by Processing the Electromyographic Signal. Critical Reviews in Biomedical Engineering, 30: (4-6), 459–485. 10.1615/CritRevBiomedEng.v30.i456.80 |
144 | Zhou, Y.-X. , Wang, H.-P. , Bao, X.-L. , Lü, X.-Y. , & Wang, Z.-G. ((2016) ). A frequency and pulse-width co-modulation strategy for transcutaneous neuromuscular electrical stimulation based on G time-domain features. Journal of Neural Engineering, 13: (1), 16004. 10.1088/1741-2560/13/1/016004 |