The Prognostic Value of DNA Methylation Markers in Renal Cell Cancer: A Systematic Review
Abstract
Introduction:
DNA methylation is an epigenetic event that occurs in normal tissues but changes in both the early and late stages of multiple tumor entities, including renal cell cancer (RCC). Many studies have shown that DNA methylation markers are relevant to RCC prognosis, but none of these markers have entered into clinical routine. Furthermore, because of its potential reversibility, DNA methylation might provide a new target for RCC therapy strategies.
Materials and methods:
Following PRISMA guidelines, we performed a systematic literature search up to February 2019. After selection for eligibility, a total of 56 studies were identified for analysis. Each study was categorized and the level of evidence assessed. Only articles reporting on DNA methylation markers and their association with survival were included. Descriptive statistical analyses were conducted with R statistical software.
Results:
We identified promoter methylation of SFRP1, GATA5, NEFH, GREM1, and BCN1 as associated with survival in RCC. Moreover, we found evidence that methylation signatures, i.e., grouping of different potential gene markers, might be of better prognostic value than single gene marker investigations. Nevertheless, because of the heterogeneous features of the studies in terms of design, methodology, patient cohorts, and statistics, the true clinical impact of these methylation markers for prognosis in RCC patients remains uncertain.
Conclusion:
This systematic review elucidates the potential impact of DNA methylation on survival of patients with RCC. Several promising prognostic markers, especially methylation signatures, were identified, which is encouraging, but prospective validations are necessary to establish their true clinical value.
INTRODUCTION
Renal cell cancer (RCC) is the twelfth most common cancer worldwide. In 2012, it accounted for about 338,000 new cases [1]. Clear cell RCC (ccRCC) represents 75% – 80% of all RCCs and is the major histological subtype. Currently, the University of California Los Angeles (UCLA) Integrated Staging System (UISS) and the Stage, Size, Grade, Necrosis (SSIGN) Score are clinical models used for estimating prognosis and survival for patients with localized RCC. Both models are based on clinicopathological features rather than molecular-based biomarkers, and despite their high predictive competence [2, 3], they carry the risk of omitting important patient-dependent factors. Numerous molecular markers are currently under investigation with the aim of improving these models. A main focus is on epigenetic alterations, such as DNA methylation.
DNA methylation is the covalent addition of a methyl group to a cytosine adjacent to a guanine (CpG dinucleotide), which changes the chemical properties of the DNA without altering the DNA sequence. Gene promoter regions often contain a high density of CpG dinucleotides, or so-called CpG islands, and methylation in these regions is frequently linked to gene silencing because it renders them inaccessible for transcription [4]. In addition to DNA hypermethylation, global and gene-specific hypomethylation represents a second frequent aberration. This process can lead to proto-oncogene activation and genomic instability in repetitive sequences if there is a decrement in genome-wide methylation (global hypomethylation) [5, 6].
The Cancer Genome Atlas (TCGA) Kidney consortium recently conducted a comprehensive characterization of the molecular alterations associated with RCC. In addition to genetic mutations, the consortium found, along with multiple other reports, that ccRCC [7] and papillary RCC (PRCC) [8] characteristically display DNA hypermethylation of gene promoters and concurrent loss of gene function from transcriptional silencing. Of interest, this group found only limited associations between gene mutations and RCC prognosis. These data are in line with previously conducted genetic studies demonstrating inconsistent results regarding survival [9].
In contrast, for a significant number of genes, DNA hypermethylation occurs with high frequency and to a degree that correlates with clinicopathological features and survival in RCC [10]. For example, the tumor suppressor genes RAS-associated domain family 1 (RASSF1A) and secreted frizzled-related protein 1 (SFRP1) are each found in 30% to >70% of tumors. These tumor suppressors are associated with both clinical pathological features and outcomes [12–15]. A recent study showed that tumor-specific hypermethylation in RCC correlated with adverse pathology and was associated with overall survival (OS) among patients undergoing targeted therapy [16]. Detecting DNA methylation–based biomarkers is therefore of substantial interest because these markers could serve diagnostic, prognostic, and predictive purposes [17]. In the last two decades, several published studies have focused on the prognostic and predictive value of DNA methylation markers in RCC. Because of widespread heterogeneity among them in (i) design, (ii) materials and methods, and (iii) statistical analyses, a direct comparison and proof of reproducibility is unfortunately not possible.
This systematic review provides an overview of the current literature on DNA methylation and its role as a prognosticator in RCC. Included articles were categorized and graded using the level of evidence (LOE) approach. We also critically discuss the studies in terms of methodology and perform a descriptive statistical analysis to reveal the most promising methylation markers for future clinical evaluation.
MATERIALS AND METHODS
Search strategy
We conducted this review according to PRISMA guidelines [18, 19]. A systematic literature search was performed on PubMed, including Medline, and EMBASE from 2005 through February 2019. The following terms were used for the search: renal cell carcinoma, kidney cancer, renal cell cancer, DNA methylation, prognosis, survival, and methylation. Either one term or several combinations were used for screening all relevant publications. Titles and abstracts were screened to identify eligible articles. Step two continued with scanning all bibliographies of articles identified to retrieve additional important publications that were not listed in PubMed, EMBASE, or Medline.
Inclusion criteria and study selection
We included all articles reporting on DNA methylation of one or more genes and their association with patient survival. In this review, DNA methylation refers to genome-wide evaluations. DNA methylation is found in so-called CpG islands that are typically located in the promoter region of genes and show a high density of CpG dinucleotides. Articles meeting the following inclusion criteria were included: original articles (not included: reviews, case reports, editorials, conference abstracts); English language; biomarker research performed on RCC tissues or on body fluids from patients with RCC; and inclusion of statistical analysis on patient survival. Analyses not reporting on DNA methylation of a specific gene or marker were excluded. Studies investigating marker panels or methylation signatures were included if the analyzed genes were specified.
Data extraction
Two authors (IP and CVK) initially extracted the data independently and collected the information in a standardized registration form. This form included the following information: first author, year of publication, PubMed identifier (PMID), gene/marker, source of material, study design, tumor subtype, sample size (total), sample size (survival), method used for detection of methylation, outcome measures (hazard ratio [HR], 95% confidence interval [CI]), and reported association of the marker with patient outcome. The term “patient outcome” refers to progression free survival (PFS), OS, cancer-specific survival (CSS), and recurrence free survival (RFS). Summarized results and characteristics of data extraction are shown in Supplementary Table 1.
Assessment of study categories and level of evidence
Studies were categorized as A) prospective randomized controlled trials specifically designed to address tumor markers, B) prospective randomized controlled trials not specifically addressing tumor markers, C) prospective, observational studies, and D) retrospective, observational studies. LOE was assessed as follows: briefly, studies from category A or one or more studies from category B with concordant results were defined as LOE I; one study from B or two or more studies from C were defined as LOE II; one study from category C was LOE III; and studies from category D were designated as LOE IV/V, as proposed by Hayes et al. [20].
Statistical analysis
For descriptive analyses, we created a three-dimensional (3D) matrix using numbers at risk, HRs, and p values as primary parameters. The functions scatter3D and box3D from the R-package plot3D were used to visualize data in a 3D scatterplot. The function forest plot from R-package forest plot [21] was used to visualize HRs and corresponding 95% CIs. Data management and data visualization were conducted using R statistical software version 3.5.0 [22].
RESULTS
Results of electronic database search
Figure 1 shows a diagram of the selection process of the included studies. The initial database search revealed 633 papers in total. After removal of duplicates and articles with irrelevant titles or abstracts, 170 eligible full-text articles were identified. Out of these, 114 articles were excluded after the second assessment. The final selection comprised 56 studies.
Fig. 1
PRISMA flow chart presenting the selection process
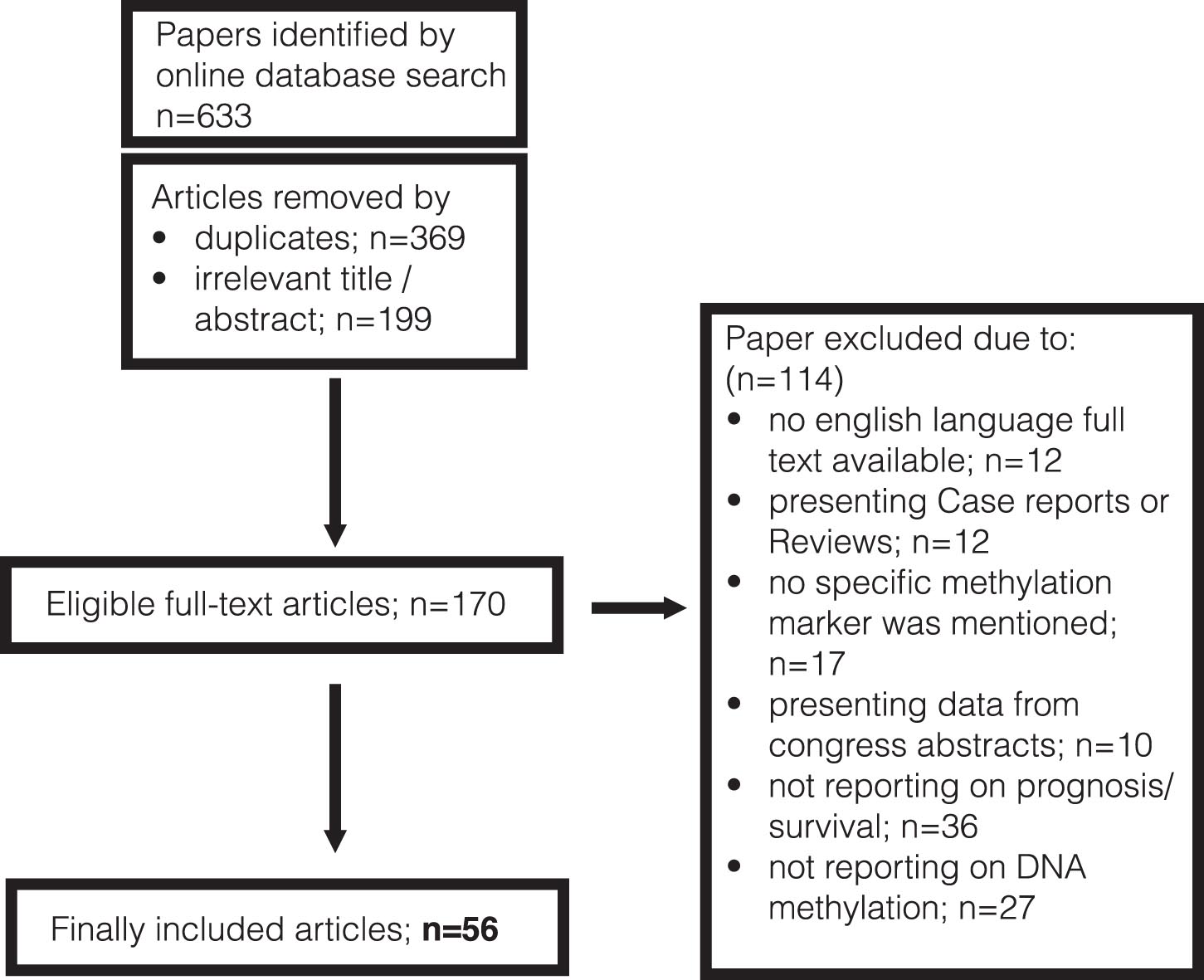
Study characteristics and level of evidence
Supplementary Table 1 summarizes the basic characteristics of reported studies. Included articles were published between 2003 and 2019. The majority were retrospective, whereas four studies were conducted using a prospective trial design. Sample sizes ranged from 18 to 993 patients in total, and the sample sizes of patients with follow-up data and who therefore were eligible for survival analyses ranged from 10 to 445. In one study, the sample size was not provided. In 19 studies, authors investigated various subtypes of RCC (mixed RCC). The term “mixed RCC” described tissues originating from patients suffering from ccRCC, PRCC, or chromophobe RCC. Thirty-six articles exclusively studied ccRCC and one study analyzed the tissues of PRCC.
Fifty-four articles involved investigations of tissue from primary tumors (PT), one study used preoperative blood serum of patients, and another one used blood-based cell-free DNA for analyses. For outcome measurements, PFS, RFS, CSS, and OS were assessed. Most studies (n = 39) reported on only one parameter, and only 17 reported on two and more.
Only six studies reached LOE grade III; all other studies were classified as LOE grade IV/V, as defined above. Category A or B studies (LOE I and II) were not identified. An internal or external validation of study findings was found for 28 studies. Figure 2A illustrates the distribution of studies with validated and non-validated outcome findings. In Fig. 2B, the 3D plot focuses exclusively on internally or externally validated outcome results.
Fig. 2
3D plots for visualization of the significance level of studies in terms of survival. A) 3D plot giving an overview of all publications on epigenomic markers in renal cell carcinoma (RCC). As expected, a large number of studies had relatively low patient numbers, often resulting in an overemphasized hazard rate for the outcome variable. The green dots () indicate studies with internally or externally validated results. Grey dots represent data with no validation (
). For this review, we focused on validated data. B) 3D plot with a closer look at validated studies with p values reaching the significance level and color-coded marker representations. The horizontal grid separates hazard ratios by ≤ and >1. As can be seen, most methylation markers are associated with an unfavorable effect on patient hazard. For the z-axis (hazard ratio), a logarithmic scale was used to better visualize values with hazard ratios of ≤1. LOE = level of evidence. Circles represent studies with LOE IV/V (∘), and squares represent studies with LOE III (□). Definitions of the different LOEs are given in the material and methods section.
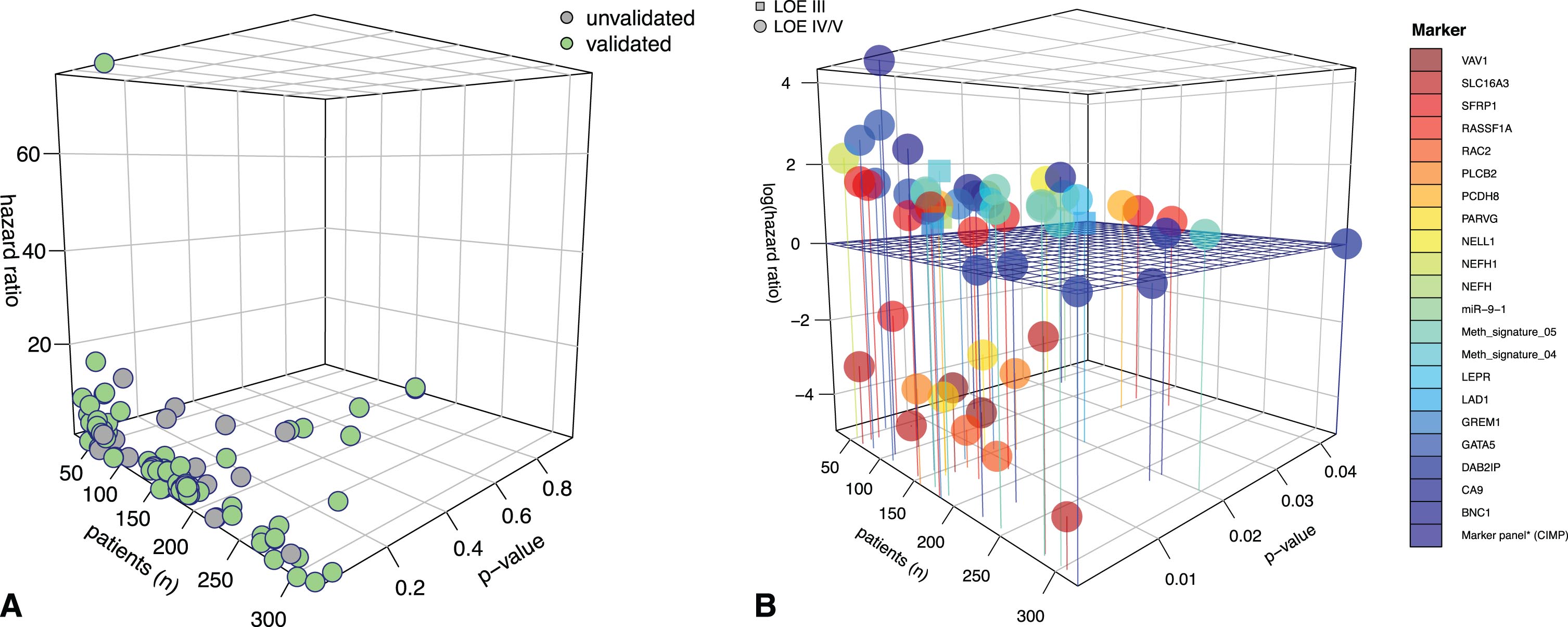
Outcome reports
All relevant data on the reported methylation markers and their associations with survival are summarized in Supplementary Table 2 and illustrated in the corresponding forest plots in Figs. 3A–D. Promoter regions of multiple genes were found to be tumor-specifically methylated in various subtypes of RCC, but for ccRCC in most cases. For our descriptive statistical analysis of reported outcomes, we focused on studies in which results were externally or internally validated. SFRP1, GATA5, BNC1, GREM1, LAD1, and NEFH showed frequent promoter methylation, which was associated with survival. Morris et al. found promoter methylation of SFRP1, along with BNC1 and COL14A1, to be associated with poorer CSS and OS (HR: 4.44; 95% CI: 2.75–86.2; and HR: 4.88; 95% CI: 2.76–87.95, respectively) in univariate Cox regression analyses. In multivariate analyses, SFRP1 (p = 0.4) was not an independent predictor of OS, but BNC1 (HR (multi) : 4.87; 95% CI: 1.14–20.88) and COL14A1 (HR: 6.56; 95% CI: 1.69–25.38) were independently associated with survival [12]. These results were confirmed by Ricketts and colleagues [23] in a large study with two independent cohorts from the TCGA KIRC dataset. In both cohorts, SRFP1 promoter methylation was statistically associated with worse OS and an independent predictor for OS (HR (multi) : 2.40; 95% CI: 1.25–4.64) and HR (multi) : 1.92; 95% CI: 1.05–3.50).
On the other hand, our research group found an association of SFRP1 methylation with prolonged RFS for patients (HR: 0.13; 95% CI: 0.03–0.58) [14]. GATA5 promoter methylation in ccRCC was associated with OS (HR: 2.82; 95% CI: 1.02–7.82), RFS (HR: 13.00; 95% CI: 3.57–47.4), and PFS (HR: 4.59; 95% CI: 1.57–13.4) in univariate Cox regression analysis [23–25]. GREM1, which encodes gremlin 1, a protein that directly binds to VEGFR2 and consecutively stimulates neoangiogenesis, is frequently methylated in RCC. Ricketts et al. identified GREM1 promoter methylation as an independent predictor of OS (HR: 3.05; 95% CI: 1.36–6.86) [23]. Van Vlodrop and colleagues confirmed these data in a huge training and validation cohort (HR: 2.3; 95% CI: 1.5–3.6, p < 0.0001) [26]. In contrast, Morris et al. found no association of GREM1 methylation with survival [12]. Dubrowinskaja and colleagues, as well as van Vlodrop et al., found NEFH methylation to be statistically associated with OS (HR (multi) : 2.6; 95% CI: 1.5–4.6) [26] in patients with ccRCC and with PFS (HR: 8.61, 95% CI: 3.03–24.5) [16] in patients within an overall RCC cohort.
Methylation signatures, i.e., combinations of markers with potential prognostic value, seem to be of special interest. Van Vlodrop’s group showed a robust association of hypermethylation of a four-gene panel consisting of NEFH, GREM1, LAD1, and NEURL with worse OS in patients with ccRCC (HR (multi) : 6.6; 95% CI: 2.1–20.1) [26]. Furthermore, a methylation signature reported by Wei et al. consistently showed a strong association with OS in various test and validation cohorts with sample sizes >150 patients, and a high-risk score was correlated with a poorer outcome (Fig. 3A) [27]. Tian et al. investigated 23 CpG units out of a seven-gene panel in a ccRCC cohort. A positive methylation status, i.e., more than 16 CpG methylated units, was correlated in univariate Cox regression analysis with shorter RFS and CSS (Fig. 3B and D) [28]. CpG island methylator phenotype (CIMP) positivity and its associations with outcome were also shown in a TCGA PRCC analysis. In a cohort of 159 PRCC patients, OS was significantly decreased in patients with CIMP positivity (suppl. Table 2) [8]. Of interest, methylation of PARVG (HR: 0.09; 95% CI: 0.00027–0.29), RAC2 (HR: 0.016; 95% CI: 0.00059–0.061), PLCB2 (HR: 0.047; 95% CI: 0.0039–0.57), and VAV1 (HR: 0.0226; 95% CI: 0.0013–0.372) was associated with longer OS in patients from a TCGA KIRC dataset, both solely and in a four-gene panel risk score (p = 0.00163) (Fig. 3A, suppl. Table 2) [29].
TCGA KIRC analysis provided an extensive dataset of different single gene as well as multi-gene panel analyses. In 445 patients and a Cox univariate model, a 25-gene panel analysis showed an association with worse outcome whereas an analysis of a three-gene panel was statistically linked with better OS (p < 0.05; suppl. Table 2) [7].
Fig. 3
Forest plots. Forest plot for 58 publications on epigenomic markers in renal cell carcinoma (RCC) with regard to: A) overall survival (OS), B) recurrence-free survival (RFS), C) progression-free survival (PFS), and D) cancer-specific survival (CSS). Only publications that were internally or externally validated were included. Studies with cohort sample sizes of <150 and ≥150 are illustrated with small and large squares, respectively (■, ■). HR = hazard ratio; 95% CI = 95% confidence interval; PT = primary tumor; marker panel* = CpG island methylator phenotype (CIMP) with more than >16CpG sites methylated.
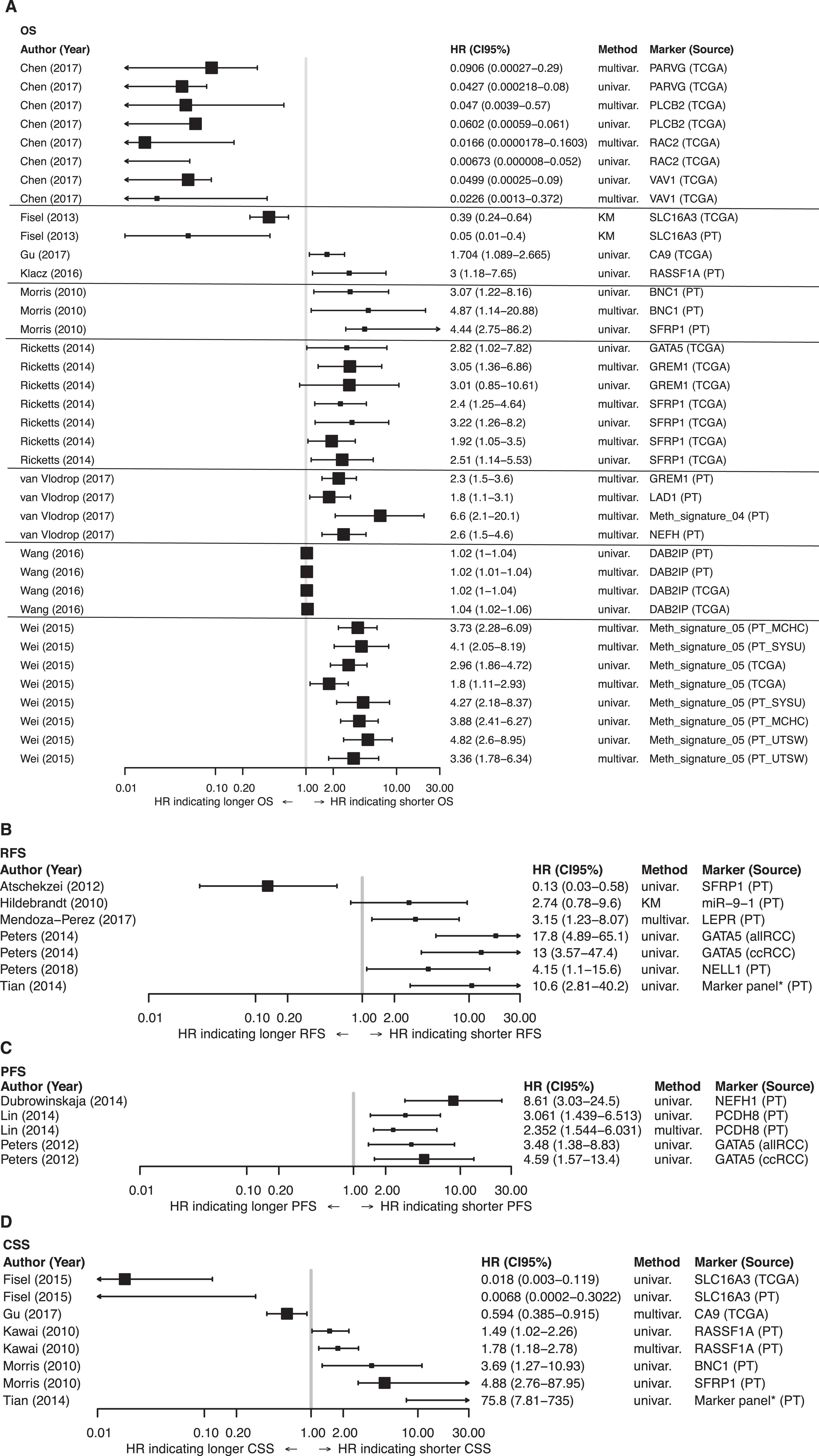
DISCUSSION
With an increasing number of incidentally detected small renal masses (<4 cm), treatment stratification in terms of surgical options vs. surveillance can be difficult, especially among older patients. For this reason, predictive tools are needed to estimate prognosis and tumor aggressiveness on an individual basis. As a cancer biomarker, DNA methylation is attractive for several reasons, including the fact that it is a stable event, and analysis is time and cost-effective compared with most of genetic analyses. Meanwhile, multiple studies support and underline the importance of epigenetic changes in RCC development and disease progression.
Exome-wide sequencing approaches have revealed only a few genes that are frequently mutated (frequency >10%) in sporadic RCC, among them PBRM1 with about 20% –41% [30, 31], BAP1 with 8% –15% [30, 32, 33], and SETD2 with 3% –12% [34–36]. In contrast, DNA hypermethylation occurs as a frequent and more homogeneous event in RCC [27, 37]. Therefore, epigenetic changes as potential drivers for RCC tumorigenesis have moved into the focus of current research activities. In addition to DNA methylation, post-translational histone modification and non-coding RNAs are the main epigenetic regulatory processes. The underlying mechanism of DNA methylation, especially de novo methylation of distinct areas in the genome, is not yet fully understood. Nevertheless, a vast number of studies have investigated the single locus methylation status of promoter regions of candidate methylation markers and identified some statistically significant associations with clinical and histopathological factors and with survival [10, 38–40].
In this systematic review, we performed an electronically based literature search on DNA methylation markers with potential prognostic value. We identified SFRP1, GATA5, BNC1, GREM1, LAD1, and NEFH as promising individual prognostic methylation markers that have been internally or externally validated and therefore are of interest for further investigations. Moreover, van Vlodrop and colleagues tested the combination of putative markers (GREM1, LAD1, NEURL, and NEFH) in three independent patient cohorts and found that performing analysis with methylation signatures leads to more robust statistical results [26]. Wei et al. also demonstrated that a CpG-based classifier consisting of five genes (PITX1, FOXE3, TWF2, EHBP1L1, and RIN1) predicted OS in ccRCC patients in a cohort of almost 1000 patients [27]. However, prospective validations in randomized trials are urgently needed to transfer these results into clinical practice.
The total increase in DNA methylation across a CpG island is designated as a CIMP. In a cohort of 100 ccRCC patients, CIMP positivity was associated with worse outcome in terms of CSS (p = 0.0001) and OS (p < 0.0001) [28]. Furthermore, analysis of a TCGA PRCC dataset also demonstrated an association between OS and CIMP positivity in non-ccRCC patient [8]. The TCGA PRCC dataset includes 159 patients with PRCC and is currently the largest comprehensive molecular characterization of non-ccRCC. A CIMP was observed in a subcohort with PRCC type II showing methylation of the CDKN2A promoter. This CIMP was also associated with mutations in the fumarate hydratase gene and with poor OS [8]. This study emphasizes the need for further analyses of distinct DNA methylation patterns in non-ccRCC cohorts, likely revealing them as possible drivers of tumorigenesis in these subtypes. Moreover, CIMP positivity in ccRCC was linked to a defect in the spindle checkpoint pathway, so targeting this pathway might be of clinical interest [41].
As noted, DNA hypomethylation also plays a relevant role in RCC tumorigenesis. A retrospective case–control study from Mendoza-Pérez and colleagues revealed a significantly lower 5-methyldeoxycytosin content in peripheral blood samples from RCC patients compared to healthy individuals [42]. Furthermore, in a TCGA KIRC analysis, an association between SETD2 mutations and promoter region–independent global DNA hypomethylation was linked to histone H3K36 trimethylation, which sustains the heterochromatin structure [7, 43]. Nevertheless, most studies found that DNA hypermethylation, rather than global hypomethylation, was associated with RCC [44].
In addition to these encouraging findings, we found some inconsistent results. These inconsistencies included heterogeneity in terms of methodology, clinical, and statistical factors. More than eight methylation analysis techniques were used, including non-quantitative methods, precluding the need for cut-off calculations. Ideally, in clinical routine, a practicable test relies mainly on three aspects: costs, applicability, and reliability. In recent years, DNA methylation detection approaches have made considerable improvements. A review by Umer et al. nicely summarizes the most frequently used methods. These authors also explain the underlying mechanisms of the different methods for DNA methylation analysis and describe the advantages and disadvantages of each method in detail [45]. For example, bisulfite-treated DNA in combination with array-based assays like GoldenGate or Illumina Infinium Bead Chip assays are widely used. Their coverage rate is high, but the need for bisulfite pretreatment may cause bisulfite PCR bias and higher costs because of handling. All methods have their shortcomings: if the genome coverage is excellent, as for the shotgun bisulfite sequencing on high-throughput platforms, which is the current gold standard for DNA methylation analysis, the costs and efforts per patient are still immense. Recent approaches in next-generation sequencing will prospectively allow sufficient DNA methylation analyses in a near-patient and cost-effective way.
In terms of clinical factors, more than 90% of the studies were retrospective. Matched paired cohorts or complete survival data were often not available. Also, the numbers of patients were relatively small in most studies (<100 patients in about 50% of the studies). Because retrospective trial designs mostly involved no predefined inclusion or exclusion criteria and inclusion was often based on availability of tissues, a variety of studies were prone to selection bias, resulting in a non-representative study population.
Furthermore, almost all studies investigated various RCC subtypes, but subgroup analyses by RCC type were subsequently not performed or reported in most cases. Methylation markers and their prognostic values can vary among different subtypes, so it is prognostically relevant to perform subgroup analyses [46, 47]. Nevertheless, subtypes other than ccRCC are actually underrepresented in the research activities concerning DNA methylation markers or other epigenetic alterations.
We also recognized serious statistical differences among the articles, including differing endpoints. Inconsistency in the definition of outcome measures leads to distortion. A direct comparison between studies was not viable given the absence of standardized and predefined clinical endpoints.
In conclusion, our systematic review of DNA methylation in RCC reveals a number of promising candidates with prognostic value, but none of them are ready for transfer into clinical practice. Therefore, the clinical management of RCC patients based on distinct DNA methylation markers remains unchanged. We urgently need prospective biomarker trials that are ideally incorporated into clinical trials with randomized controlled patient cohorts, homogeneous and standardized analytical and statistical methods, and clearly defined clinical endpoints. Results should be reported according to the REMARK (Reporting Recommendations for Tumor Marker Prognostic Studies) guidelines, enabling comparative analyses and meta-analyses.
FUNDING
The authors report no funding.
AUTHOR CONTRIBUTIONS
All authors contributed to the study conception, performance and interpretation of data.
CONFLICT OF INTEREST
The authors declare no competing interest.
ACKNOWLEDGMENTS
None.
SUPPLEMENTARY MATERIAL
[1] The supplementary material is available in the electronic version of this article: https://dx.doi.org/10.3233/KCA-190069.
REFERENCES
[1] | Ferlay J , Soerjomataram I , Dikshit R , Eser S , Mathers C , Rebelo M , et al. Cancer incidence and mortality worldwide: sources, methods and major patterns in GLOBOCAN 2012. International Journal of Cancer. (2014) ;136: :E359–E386. |
[2] | Patard J-J , Kim HL , Lam JS , Dorey FJ , Pantuck AJ , Zisman A , et al. Use of the University of California Los Angeles integrated staging system to predict survival in renal cell carcinoma: an international multicenter study. Journal of Clinical Oncology. (2004) ;22: :3316–22. |
[3] | Zigeuner R , Hutterer G , Chromecki T , Imamovic A , Kampel-Kettner K , Rehak P , et al. External validation of the Mayo Clinic stage, size, grade, and necrosis (SSIGN) score for clear-cell renal cell carcinoma in a single European centre applying routine pathology. European Urology. (2010) ;57: :102–9. |
[4] | Herman JG , Baylin SB . Gene silencing in cancer in association with promoter hypermethylation. New England Journal of Medicine. (2003) ;349: :2042–54. |
[5] | Mulero-Navarro S , Esteller M . Epigenetic biomarkers for human cancer: the time is now. Critical Reviews in Oncology / Hematology. (2008) ;68: :1–11. |
[6] | Sharma S , Kelly TK , Jones PA . Epigenetics in cancer. Carcinogenesis. (2010) ;31: :27–36. |
[7] | Creighton CJ , Morgan M , Gunaratne PH , Wheeler DA , Gibbs RA , Gordon Robertson A , et al. Comprehensive molecular characterization of clear cell renal cell carcinoma. Nature. (2013) ;499: :43–9. |
[8] | Cancer Genome Atlas Research Network, Linehan WM , Spellman PT , Ricketts CJ , Creighton CJ , Fei SS , et al. Comprehensive Molecular Characterization of Papillary Renal-Cell Carcinoma. New England Journal of Medicine. (2016) ;374: :135–45. |
[9] | Cheng L , Zhang S , MacLennan GT , Lopez-Beltran A , Montironi R . Molecular and cytogenetic insights into the pathogenesis, classification, differential diagnosis, and prognosis of renal epithelial neoplasms. Human Pathology. (2009) ;40: :10–29. |
[10] | Morris MR , Maher ER . Epigenetics of renal cell carcinoma: the path towards new diagnostics and therapeutics. Genome medicine. (2010) ;2: :59. |
[11] | Henrique R . The epigenetics of renal cell tumors: from biology to biomarkers. (2012) ;1–13. |
[12] | Morris MR , Ricketts C , Gentle D , Abdulrahman M , Clarke N , Brown M , et al. Identification of candidate tumour suppressor genes frequently methylated in renal cell carcinoma. Oncogene. (2010) ;29: :2104–17. |
[13] | Peters I , Rehmet K , Wilke N , Kuczyk MA , Hennenlotter J , Eilers T , et al. RASSF1A promoter methylation and expression analysis in normal and neoplastic kidney indicates a role in early tumorigenesis. Molecular Cancer. (2007) ;6: :49. |
[14] | Atschekzei F , Hennenlotter J , Jänisch S , Großhennig A , Tränkenschuh W , Waalkes S , et al. SFRP1 CpG island methylation locus is associated with renal cell cancer susceptibility and disease recurrence. Epigenetics. (2012) ;7: :447–57. |
[15] | Onay H , Pehlivan S , Koyuncuoglu M , Kirkali Z , Ozkinay F . Multigene methylation analysis of conventional renal cell carcinoma. Urol Int. (2009) ;83: :107–12. |
[16] | Dubrowinskaja N , Gebauer K , Peters I , Hennenlotter J , Abbas M , Scherer R , et al. Neurofilament Heavy polypeptideCpG island methylation associates with prognosis of renal cell carcinoma and prediction of antivascular endothelial growth factor therapy response. Cancer Medicine. (2014) ;3: :300–9. |
[17] | Laird PW . The power and the promise of DNA methylation markers. Nature reviews. Cancer. (2003) ;3: :253–66. |
[18] | Moher D , Liberati A , Tetzlaff J , Altman DG , PRISMA Group. Preferred reporting items for systematic reviews and meta-analyses: the PRISMA statement. British Medical Journal Publishing Group; (2009) . |
[19] | Liberati A , Altman DG , Tetzlaff J , Mulrow C , Gøtzsche PC , Ioannidis JPA , et al. The PRISMA statement for reporting systematic reviews and meta-analyses of studies that evaluate health care interventions: explanation and elaboration. PLoS medicine. Università di Modena e Reggio Emilia,Modena, Italy. alesslib@mailbase.it; Public Library of Science; 2009. p. e1000100. |
[20] | Hayes DF , Bast RC , Desch CE , Fritsche H , Kemeny NE , Jessup JM , et al. Tumor marker utility grading system: a framework to evaluate clinical utility of tumor markers. JNCI Journal of the National Cancer Institute. (1996) ;88: :1456–66. |
[21] | Gordon M , Lumley T . forestplot: advanced Forest Plot Using ’grid’ Graphics. (2017) . |
[22] | R Core Team. R: A language and environment for statistical computing [Internet]. Vienna, Austria: R Foundation for Statistical Computing; 2019. Available from: https://www.R-project.org/ |
[23] | Ricketts CJ , Hill VK , Linehan WM . Tumor-specific hypermethylation of epigenetic biomarkers, including SFRP1, predicts for poorer survival in patients from the TCGA Kidney Renal Clear Cell Carcinoma (KIRC) project. PLoS ONE. (2014) ;9: :e85621–13. |
[24] | Peters I , Gebauer K , Dubrowinskaja N , Atschekzei F , Kramer M , Hennenlotter J , et al. GATA5 CpG island hypermethylation is an independent predictor for poor clinical outcome in renal cell carcinoma. Oncology Reports. (2014) ;1–8. |
[25] | Peters I , Eggers H , Atschekzei F , Hennenlotter J , Waalkes S , Tränkenschuh W , et al. GATA5CpG island methylation in renal cell cancer: a potential biomarker for metastasis and disease progression. BJU International. (2012) ;110: :E144E152. |
[26] | Vlodrop IJH van , Joosten SC , De Meyer T , Smits KM , Neste L van , Melotte V , et al. A Four-Gene Promoter Methylation Marker Panel Consisting of GREM1, NEURL, LAD1, and NEFH Predicts Survival of Clear Cell Renal Cell Cancer Patients. Clinical Cancer Research. (2017) ;23: :2006–18. |
[27] | Wei J-H , Haddad A , Wu K-J , Zhao H-W , Kapur P , Zhang Z-L , et al. A CpG-methylation-based assay to predict survival in clear cell renal cell carcinoma. Nature communications. (2015) ;6: :8699. |
[28] | Tian Y , Arai E , Gotoh M , Komiyama M , Fujimoto H , Kanai Y . Prognostication of patients with clear cell renal cell carcinomas based on quantification of DNA methylation levels of CpG island methylator phenotype marker genes. BMC Cancer. (2014) ;14: :772. |
[29] | Chen G , Wang Y , Wang L , Xu W . Identifying prognostic biomarkers based on aberrant DNA methylation in kidney renal clear cell carcinoma. Oncotarget. (2017) ;8: :5268–80. |
[30] | Guo G , Gui Y , Gao S , Tang A , Hu X , Huang Y , et al. Frequent mutations of genes encoding ubiquitin-mediated proteolysis pathway components in clear cell renal cell carcinoma. Nature Publishing Grou. (2011) ;44: :17–9. |
[31] | Varela I , Tarpey P , Raine K , Huang D , Ong CK , Stephens P , et al. Exome sequencing identifies frequent mutation of the SWI/SNF complex gene PBRM1 in renal carcinoma. Nature. (2011) ;469: :539–42. |
[32] | Hakimi AA , Chen Y-B , Wren J , Gonen M , Abdel-Wahab O , Heguy A , et al. Clinical and pathologic impact of select chromatin-modulating tumor suppressors in clear cell renal cell carcinoma. European Urology. (2013) ;63: :848–54. |
[33] | Peña-Llopis S , Vega-Rubín-de-Celis S , Liao A , Leng N , Pavía-Jiménez A , Wang S , et al. BAP1 loss defines a new class of renal cell carcinoma. Nat Genet. (2012) ;44: :751–9. |
[34] | Dalgliesh GL , Furge K , Greenman C , Chen L , Bignell G , Butler A , et al. Systematic sequencing of renal carcinoma reveals inactivation of histone modifying genes. Nature. (2010) ;463: :360–3. |
[35] | Sato Y , Yoshizato T , Shiraishi Y , Maekawa S , Okuno Y , Kamura T , et al. Integrated molecular analysis of clear-cell renal cell carcinoma. Nat Genet. (2013) ;45: :860–7. |
[36] | Hakimi AA , Ostrovnaya I , Reva B , Schultz N , Chen Y-B , Gonen M , et al. Adverse outcomes in clear cell renal cell carcinoma with mutations of 3p21 epigenetic regulators BAP1 and SETD a report by MSKCC and the KIRC TCGA research network. Clinical Cancer Research.. (2013) ;19: :3259–67. |
[37] | Stewart GD , Powles T , Van Neste C , Meynert A , O’Mahony F , Laird A , et al. Dynamic epigenetic changes to VHL occur with sunitinib in metastatic clear cell renal cancer. Oncotarget. (2016) ;7: :25241–50. |
[38] | Ricketts CJ , Morris MR , Gentle D , Brown M , Wake N , Woodward ER , et al. Genome-wide CpG island methylation analysis implicates novel genes in the pathogenesis of renal cell carcinoma. Epigenetics. (2014) ;7: :278–90. |
[39] | Baldewijns MML , Vlodrop IJH van , Schouten LJ , Soetekouw PMMB , Bruïne AP de , Engeland M van . Genetics and epigenetics of renal cell cancer. Biochimica et Biophysica Acta (BBA) - Reviews on Cancer. (1785) ;133–55. |
[40] | Joosten SC , Deckers IA , Aarts MJ , Hoeben A , Roermund JG van , Smits KM , et al. Prognostic DNA methylation markers for renal cell carcinoma: a systematic review. Epigenomics. (2017) ;9: :1243–57. |
[41] | Arai E , Gotoh M , Tian Y , Sakamoto H , Ono M , Matsuda A , et al. Alterations of the spindle checkpoint pathway in clinicopathologically aggressive CpG island methylator phenotype clear cell renal cell carcinomas. International Journal of Cancer. (2015) ;137: :2589–606. |
[42] | Mendoza-Pérez J , Gu J , Herrera LA , Tannir NM , Matin SF , Karam JA , et al. Genomic DNA Hypomethylation and Risk of Renal Cell Carcinoma: a Case-Control Study. Clinical Cancer Research. (2016) ;22: :2074–82. |
[43] | Wagner EJ , Carpenter PB . Understanding the language of Lys36 methylation at histone H3. Nature reviews. Molecular cell biology. (2012) ;13: :115–26. |
[44] | Ramakrishnan N , Bose R . Analysis of distribution of DNA methylation in kidney-renal-clear-cell-carcinoma specific genes using entropy. Genomics data. (2016) ;10: :109–13. |
[45] | Umer M , Herceg Z . Deciphering the epigenetic code: an overview of DNA methylation analysis methods. Antioxidants & redox signaling. (2013) ;18: :1972–86. |
[46] | Ellinger J , Holl D , Nuhn P , Kahl P , Haseke N , Staehler M , et al. DNA hypermethylation in papillary renal cell carcinoma. BJU International. (2011) ;107: :664–9. |
[47] | Kawai Y , Sakano S , Suehiro Y , Okada T , Korenaga Y , Hara T , et al. Methylation level of the RASSF1A promoter is an independent prognostic factor for clear-cell renal cell carcinoma. Annals of oncology: official journal of the European Society for Medical Oncology / ESMO. (2010) ;21: :1612–7. |
[48] | Arai E , Kanai Y , Ushijima S , Fujimoto H , Mukai K , Hirohashi S . Regional DNA hypermethylation and DNA methyltransferase (DNMT) 1 protein overexpression in both renal tumors and corresponding nontumorous renal tissues. International Journal of Cancer. (2006) ;119: :288–96. |
[49] | Awakura Y , Nakamura E , Ito N , Kamoto T , Ogawa O . Methylation-associated silencing of TU3A in human cancers. International journal of oncology. (2008) ;33: :893–9. |
[50] | Breault JE , Shiina H , Igawa M , Ribeiro-Filho LA , Deguchi M , Enokida H , et al. Methylation of the gamma-catenin gene is associated with poor prognosis of renal cell carcinoma. Clinical Cancer Research. (2005) ;11: :557–64. |
[51] | Chen E , Zheng F , Yuan X , Ye Y , Li X , Dai Y , et al. The effect of TMEFF2 methylation on the tumor stage and survival outcome of clear cell renal cell carcinoma. Cancer biomarkers: section A of Disease markers. (2017) ;19: :207–12. |
[52] | Christoph F , Weikert S , Kempkensteffen C , Krause H , Schostak M , Köllermann J , et al. Promoter hypermethylation profile of kidney cancer with new proapoptotic p53 target genes and clinical implications. Clinical Cancer Research. (2006) ;12: :5040–6. |
[53] | Martino M de , Klatte T , Haitel A , Marberger M . Serum cell-free DNA in renal cell carcinoma: a diagnostic and prognostic marker. Cancer. (2012) ;118: :82–90. |
[54] | Deckers IAG , Schouten LJ , Neste L van , Vlodrop IJH van , Soetekouw PMMB , Baldewijns MMLL , et al. Promoter Methylation of CDO1 Identifies Clear-Cell Renal Cell Cancer Patients with Poor Survival Outcome. Clinical Cancer Research. (2015) ;21: :3492–500. |
[55] | Eggers H , Steffens S , Großhennig A , Becker JU , Hennenlotter J , Stenzl A , et al. Prognostic and diagnostic relevance of hypermethylated in cancer 1 (HIC1) CpG island methylation in renal cell carcinoma. International journal of oncology. (2012) ;40: :1650–8. |
[56] | Evelönn EA , Landfors M , Haider Z , Köhn L , Ljungberg B , Roos G , et al. DNA methylation associates with survival in non-metastatic clear cell renal cell carcinoma. BMC Cancer. (2019) ;19: :65. |
[57] | Fabrizio FP , Costantini M , Copetti M , Torre A la , Sparaneo A , Fontana A , et al. Keap1/Nrf2 pathway in kidney cancer: frequent methylation of KEAP1 gene promoter in clear renal cell carcinoma. Oncotarget. (2017) ;8: :11187–98. |
[58] | Fisel P , Stühler V , Bedke J , Winter S , Rausch S , Hennenlotter J , et al. MCT4 surpasses the prognostic relevance of the ancillary protein CD147 in clear cell renal cell carcinoma. Oncotarget. (2015) ;6: :30615–27. |
[59] | Fisel P , Kruck S , Winter S , Bedke J , Hennenlotter J , Nies AT , et al. DNA methylation of the SLC16A3 promoter regulates expression of the human lactate transporter MCT4 in renal cancer with consequences for clinical outcome. Clinical Cancer Research. (2013) ;19: :5170–81. |
[60] | Gebauer K , Peters I , Dubrowinskaja N , Hennenlotter J , Abbas M , Scherer R , et al. Hsa-mir-124-3 CpG island methylation is associated with advanced tumours and disease recurrence of patients with clear cell renal cell carcinoma. British Journal of Cancer. (2013) ;108: :131–8. |
[61] | Girgis AH , Iakovlev VV , Beheshti B , Bayani J , Squire JA , Bui A , et al. Multilevel Whole-Genome Analysis Reveals Candidate Biomarkers in Clear Cell Renal Cell Carcinoma. Cancer Research. (2012) ;72: :5273–84. |
[62] | Golovastova MO , Tsoy LV , Bocharnikova AV , Korolev DO , Gancharova OS , Alekseeva EA , et al. The cancer-retina antigen recoverin as a potential biomarker for renal tumors. Tumour biology: the journal of the International Society for Oncodevelopmental Biology and Medicine. (2016) ;37: :9899–907. |
[63] | Gu Y , Zou YM , Lei D , Huang Y , Li W , Mo Z , et al. Promoter DNA methylation analysis reveals a novel diagnostic CpG-based biomarker and RAB25 hypermethylation in clear cell renel cell carcinoma. Scientific reports. (2017) ;7: :14200. |
[64] | Hildebrandt MAT , Gu J , Lin J , Ye Y , Tan W , Tamboli P , et al. Hsa-miR-9 methylation status is associated with cancer development and metastatic recurrence in patients with clear cell renal cell carcinoma. (2010) ;29: :5724–8. |
[65] | Kagara I , Enokida H , Kawakami K , Matsuda R , Toki K , Nishimura H , et al. CpG hypermethylation of the UCHL1 gene promoter is associated with pathogenesis and poor prognosis in renal cell carcinoma. The Journal of Urology. (2008) ;180: :343–51. |
[66] | Klacz J , Wierzbicki PM , Wronska A , Rybarczyk A , Stanislawowski M , Slebioda T , et al. Decreased expression of RASSF1A tumor suppressor gene is associated with worse prognosis in clear cell renal cell carcinoma. International journal of oncology. (2016) ;48: :55–66. |
[67] | Li H , Wang J , Xiao W , Xia D , Lang B , Yu G , et al. Epigenetic alterations of Krüppel-like factor 4 and its tumor suppressor function in renal cell carcinoma. Carcinogenesis. (2013) ;34: :2262–70. |
[68] | Lin Y-L , Gui S-L , Guo H , Ma J-G , Li W-P . Protocadherin17 Promoter Methylation is a Potential Predictive Biomarker in Clear Cell Renal Cell Carcinoma. Medical science monitor: international medical journal of experimental and clinical research. (2015) ;21: :2870–6. |
[69] | Lin Y-L , Wang Y-L , Fu X-L , Ma J-G . Aberrant methylation of PCDH8 is a potential prognostic biomarker for patients with clear cell renal cell carcinoma. Medical science monitor: international medical journal of experimental and clinical research. (2014) ;20: :2380–5. |
[70] | Lin Y-L , Wang Y-P , Li H-Z , Zhang X . Aberrant Promoter Methylation of PCDH17 (Protocadherin 17) in Serum and its Clinical Significance in Renal Cell Carcinoma. Medical science monitor: international medical journal of experimental and clinical research. (2017) ;23: :3318–23. |
[71] | Mendoza-Pérez J , Gu J , Herrera LA , Tannir NM , Zhang S , Matin S , et al. Prognostic significance of promoter CpG island methylation of obesity-related genes in patients with nonmetastatic renal cell carcinoma. Cancer. (2017) ;123: :3617–27. |
[72] | Mitsui Y , Hirata H , Arichi N , Hiraki M , Yasumoto H , Chang I , et al. Inactivation of bone morphogenetic protein 2 may predict clinical outcome and poor overall survival for renal cell carcinoma through epigenetic pathways. Oncotarget. (2015) ;6: :9577–91. |
[73] | Morris MR , Ricketts CJ , Gentle D , McRonald F , Carli N , Khalili H , et al. Genome-wide methylation analysis identifies epigenetically inactivated candidate tumour suppressor genes in renal cell carcinoma. Oncogene. (2011) ;30: :1390–401. |
[74] | Peters I , Dubrowinskaja N , Abbas M , Seidel C , Kogosov M , Scherer R , et al. DNA Methylation Biomarkers Predict Progression-Free and Overall Survival of Metastatic Renal Cell Cancer (mRCC) Treated with Antiangiogenic Therapies. PLoS One. (2014) ;9: :e91440–8. |
[75] | Peters I , Dubrowinskaja N , Hennenlotter J , Antonopoulos WI , Klot CAJ von , Tezval H , et al. DNA methylation of neural EGFL like∼1 (NELL1) is associated with advanced disease and the metastatic state of renal cell cancer patients. Oncology Reports. (2018) ;40: :3861–8. |
[76] | Pires-Luís AS , Costa-Pinheiro P , Ferreira MJ , Antunes L , Lobo F , Oliveira J , et al. Identification of clear cell renal cell carcinoma and oncocytoma using a three-gene promoter methylation panel. Journal of translational medicine. (2017) ;15: :149. |
[77] | Pompas-Veganzones N , Sandonis V , Perez-Lanzac A , Beltran M , Beardo P , Juárez A , et al. Myopodin methylation is a prognostic biomarker and predicts antiangiogenic response in advanced kidney cancer. Tumour biology: the journal of the International Society for Oncodevelopmental Biology and Medicine. (2016) ;37: :14301–10. |
[78] | Ricketts CJ , Morris MR , Gentle D , Shuib S , Brown M , Clarke N , et al. Methylation profiling and evaluation of demethylating therapy in renal cell carcinoma. (2013) ;5: :1. |
[79] | Smits KM , Schouten LJ , Dijk BAC van , Kaa CA de , Wouters KAD , Oosterwijk E , et al. Genetic and epigenetic alterations in the von hippel-lindau gene: the influence on renal cancer prognosis. Clinical Cancer Research. (2008) ;14: :782–7. |
[80] | Su X , Zhang J , Mouawad R , Comperat E , Rouprêt M , Allanic F , et al. NSD1 Inactivation and SETD2 Mutation Drive a Convergence toward Loss of Function of H3K36 Writers in Clear Cell Renal Cell Carcinomas. Cancer Research. (2017) ;77: :4835–45. |
[81] | Urakami S , Shiina H , Enokida H , Hirata H , Kawamoto K , Kawakami T , et al. Wnt antagonist family genes as biomarkers for diagnosis, staging, and prognosis of renal cell carcinoma using tumor and serum DNA. Clinical Cancer Research. (2006) ;12: :6989–97. |
[82] | Vlodrop IJH van , Baldewijns MML , Smits KM , Schouten LJ , Neste L van , Criekinge W van , et al. Prognostic Significance of Gremlin1 (GREM1) Promoter CpG Island Hypermethylation in Clear Cell Renal Cell Carcinoma. The American Journal of Pathology. (2010) ;176: :575–84. |
[83] | Vidaurreta M , Maestro ML , Sanz-Casla MT , Maestro C , Rafael S , Veganzones S , et al. Inactivation of p16 by CpG hypermethylation in renal cell carcinoma. URO. (2008) ;26: :239–45. |
[84] | Wang Z-R , Wei J-H , Zhou J-C , Haddad A , Zhao L-Y , Kapur P , et al. Validation of DAB2IP methylation and its relative significance in predicting outcome in renal cell carcinoma. Oncotarget. (2016) ;7: :31508–19. |
[85] | Xu B , Zhang L , Luo C , Qi Y , Cui Y , Ying J-M , et al. Hypermethylation of the 16q23. 1 tumor suppressor gene ADAMTS18 in clear cell renal cell carcinoma. International journal of molecular sciences. (2015) ;16: :1051–65. |
[86] | Yamada D , Kikuchi S , Williams YN , Sakurai-Yageta M , Masuda M , Maruyama T , et al. Promoter hypermethylation of the potential tumor suppressor DAL-1/4. 1B gene in renal clear cell carcinoma. International Journal of Cancer. (2006) ;118: :916–23. |
[87] | Yoo KH , Park Y-K , Chang S-G . DNA hypomethylation of interleukin 8 in clear cell renal cell carcinoma. Oncology letters. (2013) ;5: :39–42. |
[88] | Young AC , Craven RA , Cohen D , Taylor C , Booth C , Harnden P , et al. Analysis of VHL Gene Alterations and their Relationship to Clinical Parameters in Sporadic Conventional Renal Cell Carcinoma. Clinical Cancer Research. (2009) ;15: :7582–92. |
[89] | Zhao H , Leppert JT , Peehl DM . A Protective Role for Androgen Receptor in Clear Cell Renal Cell Carcinoma Based on Mining TCGA Data. PLoS One. (2016) ;11: :e0146505. |