Oscillation-Specific Nodal Differences in Parkinson’s Disease Patients with Anxiety
Abstract
Background:
Parkinson’s disease (PD) is a common neurodegenerative disorder that is predominantly known for its motor symptoms but is also accompanied by non-motor symptoms, including anxiety.
Objective:
The underlying neurobiological substrates and brain network changes associated with comorbid anxiety in PD require further exploration.
Methods:
An analysis of oscillation-specific nodal properties in patients with and without anxiety was conducted using resting-state functional magnetic resonance imaging (rs-fMRI) and graph theory. We used a band-pass filtering approach to differentiate oscillatory frequency bands for subsequent functional connectivity (FC) and graph analyses.
Results:
The study included 68 non-anxiety PD (naPD) patients, 62 anxiety PD (aPD) patients, and 64 healthy controls (NC). Analyses of nodal betweenness centrality (BC), degree centrality (DC), and efficiency were conducted across multiple frequency bands. The findings indicated no significant differences in BC among naPD, aPD, and NC within the 0.01–0.08 Hz frequency range. However, we observed a specific reduction in BC at narrower frequency ranges in aPD patients, as well as differing patterns of change in DC and efficiency, which are believed to reflect the neurophysiological bases of anxiety symptoms in PD.
Conclusions:
Differential oscillation-specific nodal characteristics have been identified in PD patients with anxiety, suggesting potential dysregulations in brain network dynamics. These findings emphasize the complexity of brain network alterations in anxiety-associated PD and identify oscillatory frequencies as potential biomarkers. The study highlights the importance of considering oscillatory frequency bands in the analysis of brain network changes.
INTRODUCTION
Parkinson’s disease (PD) is a progressive neurodegenerative disease that occurs in the elderly, with a higher incidence in males than in females [1]. Common motor symptoms amongst most PD patients include resting tremor, bradykinesia, and rigidity. In addition to motor symptoms, PD also causes non-motor symptoms (NMS) such as mood disorders, cognitive impairments, sleep disturbances, and autonomic dysfunction. Anxiety is among these NMS [2]. It has been shown to be a common exacerbating factor of PD, with a prevalence rate of 31% [3].
In PD with anxiety, resting-state functional magnetic resonance imaging (rs-fMRI) has become an active research field. Studies regarding brain networks have shown that compared to PD patients without anxiety, those with anxiety are characterized by changes in specific brain networks [4]. The commonly involved brain regions include the default mode network, salience network, and the frontal-striatal circuits, all of which are implicated in emotional processing and regulation [5]. Additionally, studies suggest that the degree of functional connectivity changes in certain brain regions in PD patients is correlated with the severity of anxiety symptoms. The limbic system plays a key role in mood regulation, and disruptions in that system can lead to anxiety [6]. Furthermore, interventional studies using rs-fMRI have started to explore how non-pharmacological interventions such as deep brain stimulation affect the functional connectivity patterns in patients with PD and anxiety [7]. Despite these advancements, challenges still persist in the field.
Several frequencies will resonate and oscillate simultaneously within neurons and networks due to their complex dynamics. Information can be carried across different dimensions by these different oscillations in brain networks [8, 9]. A wide range of power spectrums can be used by rs-fMRI to study dynamic brain networks, but oscillatory coupling is typically detected within a single frequency band [10]. Some studies have decomposed the fMRI oscillations into different frequency bands [11, 12]. Measurement of local spontaneous brain activity in patients with PD, however, revealed oscillation-specific functional abnormalities. In order to fully understand the underlying pathogenesis of PD, local measurements cannot fully capture the complexity of the human brain [13, 14]. Therefore, reverting the human brain to a large-scale network with multiple oscillatory frequencies may help to better understand PD, especially its comorbid psychiatric symptoms. Graph theory analysis models the human brain as a complex, large-scale network and provides a powerful mathematical framework for characterizing the topological structure of brain networks [15]. Some studies using this approach have found that the global network topology of patients with PD is disrupted in terms of integration and segregation [16]. Nevertheless, these studies only analyzed oscillations of the BOLD signal within a single frequency band while elucidating the pathogenic mechanisms of PD from the network perspective, and they neglected the unique information provided by multiple frequency bands [17, 18]. Consequently, nodal properties that may be affected by progressive oscillations in PD patients with anxiety have not yet been explored, suggesting that this may explain why some patients with PD experience anxiety in addition to their PD symptoms.
PD patients with and without anxiety were measured based on graph theory analysis within each oscillation frequency. In addition, the effect of different oscillation frequencies on the node characteristics is also investigated.
METHODS
Subjects
An informed consent form was signed by all PD patients and healthy control (HC), and all procedures were conducted in accordance with the Declaration of Helsinki and the ethical standards of the Institutional Research Board. In accordance with British Parkinson’s Disease Society criteria, senior neurosurgeons diagnosed PD [19]. Information regarding patient demographics (including age, sex, and education) and clinical assessments, such as Hoehn-Yahr stage (H-Y), duration of disease, levodopa and equivalent dose (LED). Hamilton Depression Rating Scale (HAMD) were employed to assess the patients’ depression state. The severity of anxiety is measured by 14 Hamilton Anxiety Assessment Scales (HAMA-14). In the control group, the above demographic and clinical information were collected. Clinical information and fMRI data were collected overnight (at least 12 hours) after medication discontinuation in patients with PD taking antiparkinsonian drugs. HAMA-14 scores were used to categorize PD patients into an anxiety group (aPD) and a non-anxiety group (naPD). Patients were divided into aPD group if their HAMA-14 score was more than 14, and naPD group if their HAMA-14 score was less than 14. Exclusion criteria were as follows: (1) schizophrenia, n = 1; (2) metal artifacts on MRI scans, n = 3; (3) underlying cognitive impairment according to MMSE scores (MMSE scores < 27), n = 1; (4) excessive head movement (>2 mm at transition, 2° rotation), n = 3; and (5) removal of more than 1/3 of the bad time points after scrubbing, n = 3.
The study was reviewed and approved by Ethics Committee of The First Affiliated Hospital of USTC (2022-RE-154).
MRI scanning
In the study, an eight-channel phased array head coil was used with a 3.0 T MRI scanner (GE Healthcare, Chicago, IL, USA) for patients with PD and neuropathy. 3D T1-weighted images were acquired using a sagittal fast gradient echo sequence. We acquired functional MRI images using the following SE-EPI sequence. Scanning parameters were as in the previous study [20].
fMRI data preprocessing
Data pre-processing was conducted with Resting-State fMRI Data Analysis Toolkit plus V1.27 (RESTplus V1.27, http://restfmri.net/forum/index.php), which is based on Statistical Parametric Mapping (SPM; https://www.fil.ion.ucl.ac.uk/spm/) [21]. Image data preprocessing was performed as in our previous study [20]. We used band-pass filtering as a tool for reducing non-neuronal contributions to BOLD fluctuations at the following frequencies: 0.01–0.08 Hz, 0.01–0.01 Hz, and 0.027–0.073 Hz, respectively.
Functional connectivity analysis
Functional connectivity (FC) analysis was performed using RESTplus v1.27 (http://www.restfmri.net/forum/restplus). We selected 58 regions of interest (ROIs) associated with the default mode network (DMN) from the Power 264 atlas to calculate ROI-level FC [22]. Pearson’s correlation analysis was used to calculate the FC values for the 58 regions of interest. Correlation coefficients were converted to z-scores using Fisher’s transformation of r-to-z coefficients. A 58×58 matrix was generated for each participant. The zFC matrices of the naPD, aPD, and HC groups were compared by a two-sample t-test. Bonferroni correction (p < 0.05) was applied when FC comparisons were made among the naPD, aPD, and HC groups. A table of DMN-related ROIs in the Power264 template against the AAL template is included in Supplementary Table 1.
Graph analysis
Graph analysis was performed using GRETNA 2.0. This study used 58 ROIs associated with the DMN from the power 264 atlas. Each ROI represents a node of the network. After extracting each node’s average time course, the Pearson correlation between their time courses was calculated to assess the resting-state functional connectivity between regions. Analyzing parametric data was improved using Fisher’s r-to-z transformation. Graph-theory-based network analysis results were obtained by first generating network matrices (58×58) in three frequency bands (0.01×0.08 Hz, 0.01×0.027 Hz, and 0.027×0.073 Hz) for each subject [23].
For each constructed matrix, a sparsity value was calculated by dividing the total number of edges by the maximum number of edges in the network prior to calculating topological node metrics. A sparsity interval of 5% has been chosen instead of one threshold since the topological attribute calculation is strongly influenced by network sparsity.
We calculated topological node metrics for each subject for the constructed functional networks. These metrics include node median centrality, node degree centrality, and node efficiency. To compare nodal metrics among naPD, aPD, and HC groups, a two-sample t-test was conducted. The Bonferroni correction (p < 1/58 = 0.01724) was applied to the p-value when comparing nodal metrics between groups.
Statistical analysis for demographic and clinical information
To determine whether the data were normally distributed, a one-sample Kolmogorov-Smirnov test was conducted. Differences in age, education, disease duration, UPDRS motor scores, and H-Y staging were compared between the full PD group and the normal control group, between the PD groups, and between the naPD group, aPD group, and control group using the independent samples t-test or ANOVA. Pearson’s chi-square test was used to compare the sex distribution between and among groups. For comparison of the differences in HAMA-14 scores between two or three groups, the Mann-Whitney U test or Kruskal-Wallis test was used due to non-normality in the data distribution. Pearson’s correlation coefficient was used to analyze the correlation between nodal characteristics and HAMA-14 scores.
RESULTS
Demographic and clinical information
The analysis included 68 patients with naPD, 62 patients with aPD, and 64 HC. There was a significant difference between the HC and PD groups in HAMA-14 scores, which is in line with the results of many epidemiological studies. With the exception of HAMA-14 scores, which were significantly lower in patients with naPD than those with aPD, other disease-related factors including H-Y stage, disease duration, and LED did not differ significantly between the groups. The specifications listed in Table 1 show the specified information.
Table 1
Demographic and clinical information of the participants
HC | naPD | aPD | p | |
No. | 64 | 68 | 62 | |
Age | 61.0±6.6 | 58.7±7.5 | 59.4±8.4 | 0.199 |
Duration (y) | – | 9.0±3.6 | 8.3±3.8 | 0.267 |
UPDRS-III drug off | – | 53.5±13.8 | 56.7±12.6 | 0.175 |
UPDRS-III drug on | 26.2±11.0 | 28.9±11.0 | 0.158 | |
HAMD | 16.92±4.32 | 17.29±4.74 | 16.28±5.05 | 0.072 |
HAMA-14 | 4.0±2.1 | 10.7±2.4 | 22.5±3.7 | <0.001 |
MMSE | 28.6±1.1 | 27.8±0.6 | 27.3±0.8 | 0.779 |
LED | 643.8±437.2 | 669.4±316.6 | 0.705 | |
Drug improvement rate | – | 0.5±0.1 | 0.5±0.1 | 0.200 |
Gender | 0.644 | |||
male | 30 (46.9%) | 36 (52.9%) | 34 (54.8%) | |
female | 34 (53.1%) | 32 (47.1%) | 28 (45.2%) | |
H-Y | 0.668 | |||
2.5 | – | 14 (22.6%) | 11 (16.1%) | |
3 | – | 29 (46.8%) | 39 (57.4%) | |
4 | – | 19 (30.6%) | 18 (26.5%) |
Oscillation-specific nodal alterations in PD with anxiety
For nodal betweenness centrality (BC) analysis (see Fig. 1; Supplementary Table 2), within the frequency range of 0.01–0.08 Hz, there were no significant differences identified between the naPD, aPD, and HC groups. Specifically within the 0.01–0.027 Hz range, the aPD group showed a decrease in BC within the right middle occipital gyrus compared to naPD. Additionally, the aPD group exhibited a reduced BC in the same right middle occipital gyrus and the right temporal pole, as well as an increased BC in the left paracentral lobule in comparison to the HC group. Compared to naPD patients, an increase in BC was observed in the left middle temporal gyrus in the frequency range of 0.027–0.073 Hz. Also, the aPD group presented with elevated BC in the left paracentral lobule compared with HC, while the naPD and HC groups did not show significant differences in BC.
Fig. 1
Oscillation-specific alterations of nodal betweenness centrality in PD with or without anxiety. Red nodes suggest an increase in betweenness centrality and blue nodes suggest a decrease in betweenness centrality.
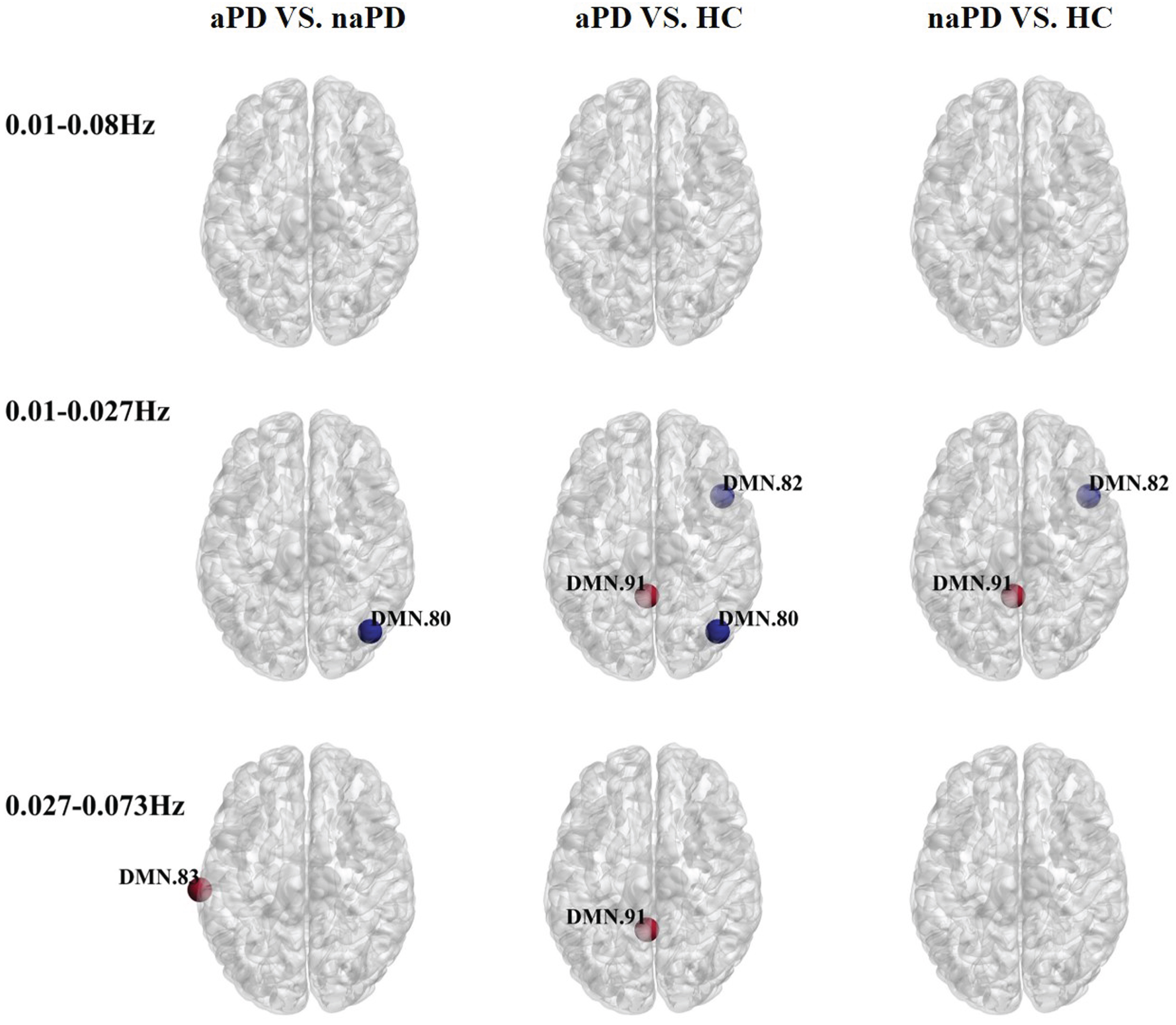
Regarding nodal degree centrality (DC) analysis (see Fig. 2; Supplementary Table 3), the aPD group had a higher DC in the left middle temporal gyrus within the 0.01–0.08 Hz frequency band. In comparison to HC, the aPD group had higher DC in the left middle temporal gyrus, left angular gyrus, right angular gyrus, and left paracentral lobule, along with a decreased DC in the right paracentral lobule. The naPD group exhibited an increased DC in the left angular gyrus and a decreased DC in the left middle temporal gyrus compared to HC. There were no significant DC differences between naPD and aPD within the 0.01–0.027 Hz band. In contrast to the HC group, the aPD group demonstrated increased DC in the left angular gyrus and Crus I of the cerebellar hemisphere. Crus I had an elevated DC in naPD compared to HC. Within the 0.027–0.073 Hz band, an increase in DC was noted in the left middle temporal gyrus for aPD patients in comparison to naPD. The aPD group showed increased DC in the left middle temporal gyrus and left paracentral lobule relative to HC, and naPD participants had increased DC in the left angular gyrus and right dorsolateral aspect of the suprafrontal gyrus compared to HC.
Fig. 2
Oscillation-specific alterations of nodal degree centrality in PD with or without anxiety. Red nodes suggest an increase in degree centrality and blue nodes suggest a decrease in degree centrality.
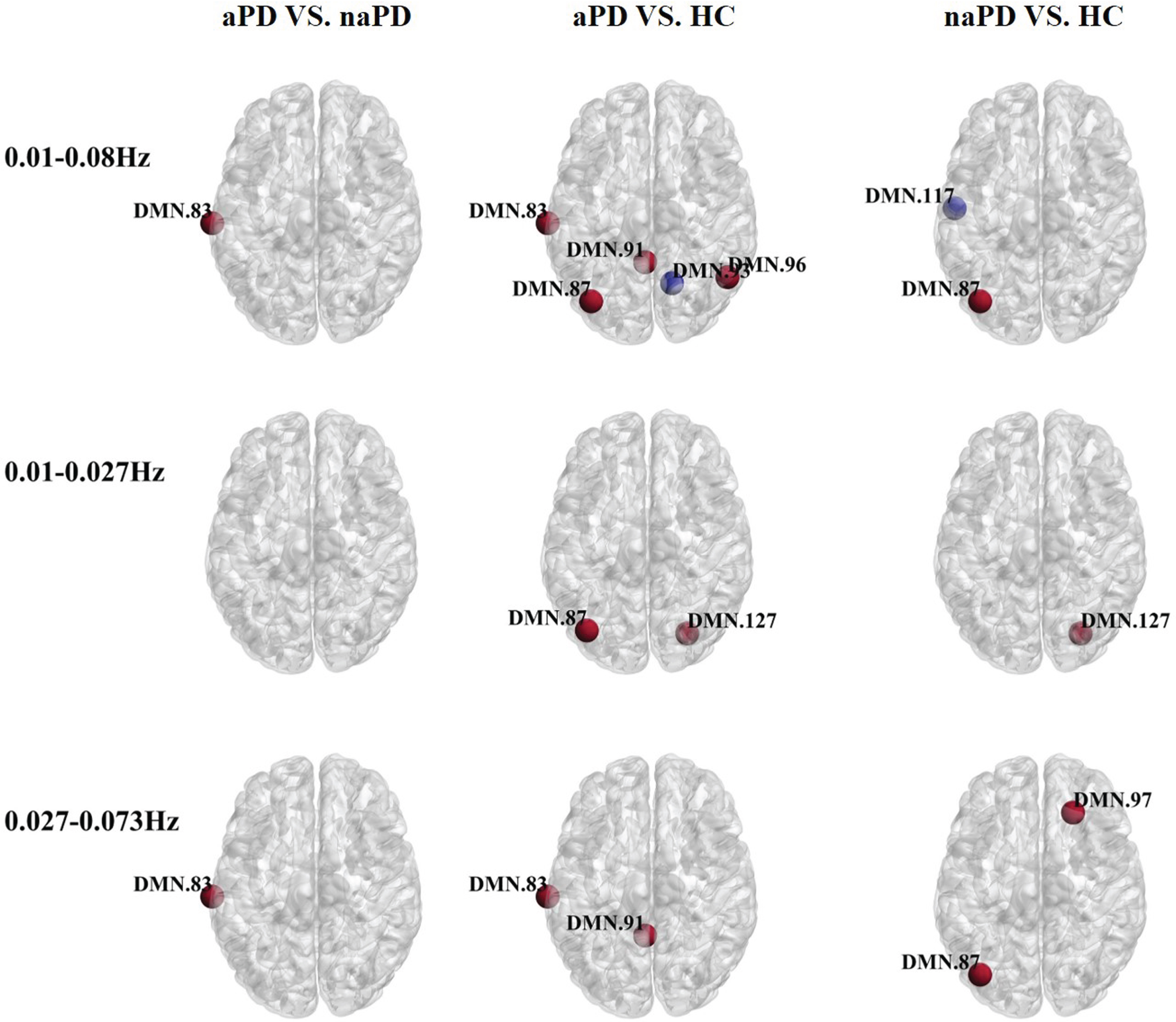
As for nodal efficiency (illustrated in Fig. 3; Supplementary Table 4), within the 0.01–0.08 Hz frequency range, the aPD group presented with enhanced efficiency in the left middle temporal gyrus compared to the naPD group. In comparing the aPD participants to the HC group, the left middle temporal gyrus, left angular gyrus, and left paracentral lobule displayed greater efficiency, while the right paracentral lobule was less efficient. The naPD group exhibited decreased efficiency in the left middle temporal gyrus in contrast with the HC group. Within the 0.01–0.027 Hz range, the left middle temporal gyrus efficiency was higher in the aPD group versus the naPD group. In comparison to HC, the aPD group displayed elevated efficiency in the left angular gyrus, while the naPD group showed lower efficiency in the left middle temporal gyrus. Within the 0.027–0.073 Hz band, the aPD group experienced higher efficiency in the left middle temporal gyrus and diminished efficiency in the left anterior cingulate gyrus compared to the naPD group. The aPD group also had increased efficiency in the left middle temporal gyrus and left paracentral lobule and decreased efficiency in the right paracentral lobule when compared to HC. The naPD group had lower efficiency in the left middle temporal gyrus than the HC group.
Fig. 3
Oscillation-specific alterations of nodal efficiency in PD with or without anxiety. Red nodes suggest an increase in efficiency and blue nodes suggest a decrease in efficiency.
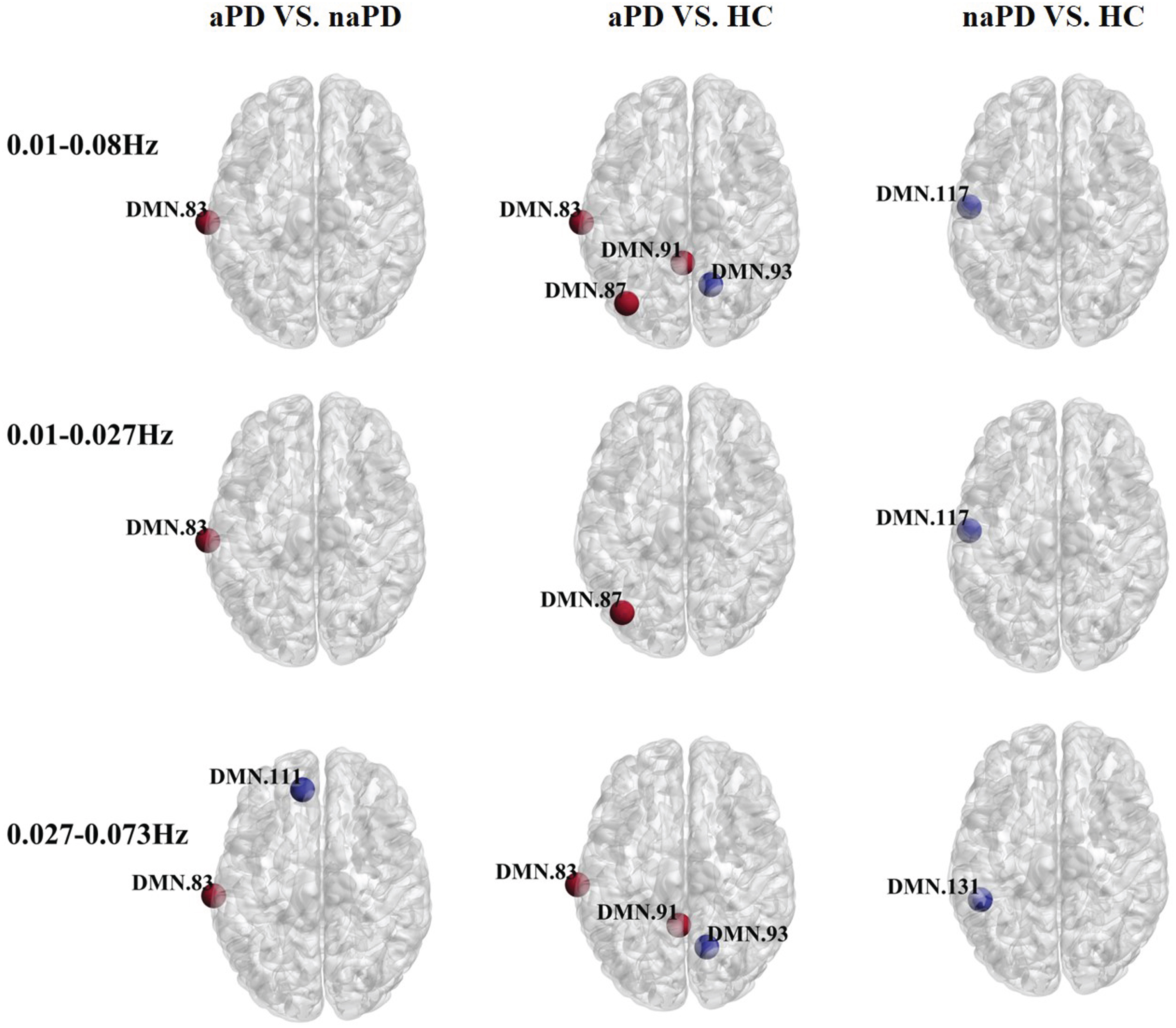
Correlation between node characteristics and anxiety in patients with aPD
We analyzed the characteristics of nodes that showed significant differences between aPD patients and naPD patients in relation to the patients’ HAMA-14 scores. We found that the nodal BC and DC of the left middle temporal gyrus (DMN83) within the 0.027–0.073 Hz frequency band are significantly negatively correlated with the HAMA-14 scores. Similarly, the DC of DMN83 within the 0.01–0.08 Hz range also shows a significant negative correlation with HAMA-14 scores. The nodal efficiency of DMN83 is negatively correlated with HAMA-14 scores within both the 0.01–0.027 Hz and 0.027–0.073 Hz ranges, but shows no significant correlation within the 0.01–0.08 Hz frequency range. The nodal efficiency of the left anterior cingulate gyrus (DMN111) within the 0.027–0.073 Hz range is significantly positively correlated with HAMA-14 scores (see Fig. 4).
Fig. 4
Correlation between node characteristics and anxiety in patients with aPD.
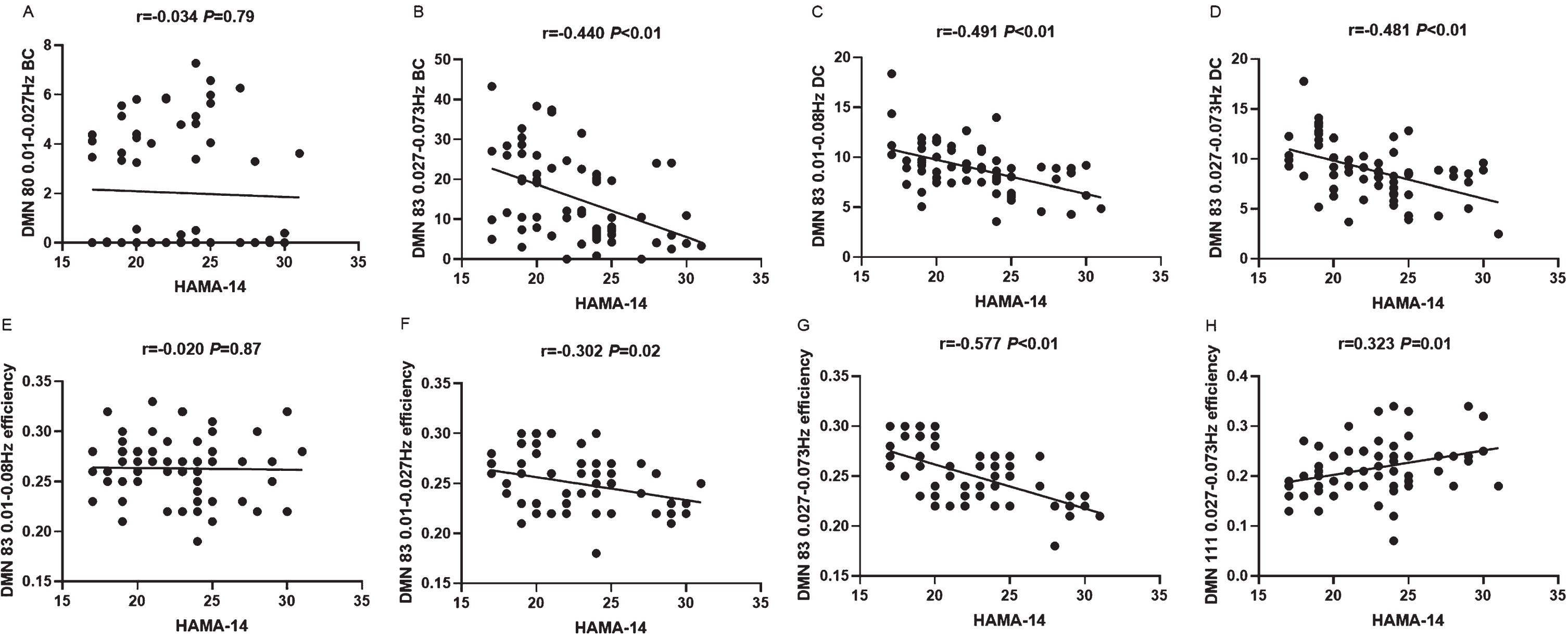
DISCUSSION
Research suggests that there may be specific oscillation-specific node differences in the brains of PD patients [24]. A study found increased functional connectivity between the limbic system (which is involved in emotion processing) and various cortical regions in anxious PD patients compared to PD patients without anxiety [25]. Another study using magnetoencephalography (MEG) found abnormal oscillatory power and functional connectivity in the frontal cortex of PD patients [26]. These findings suggest that there may be significant oscillation-specific node differences in the brains of PD patients with anxiety, which may account for the presence and severity of anxiety symptoms in these individuals.
In this study, we have garnered some interesting insights from our research into the oscillation-specific nodal properties of PD patients with anxiety. Through our analysis, we observed relatively preserved nodal properties in naPD patients. In contrast, more widespread abnormalities were observed in aPD patients, consistent across all three oscillatory frequencies and nodal property indicators. Additionally, nodal specificity also exhibited different changes across various frequency ranges.
Our results for BC in differing frequency ranges (0.01–0.08 Hz, 0.01–0.027 Hz, and 0.027–0.073 Hz) suggest that both anxiety status in PD and the analyzed frequency band influence nodal centrality. Specifically, in the 0.01–0.027 Hz range, the reduction in BC in the right middle occipital gyrus in aPD compared to naPD could reflect an interruption in integration within the visual processing areas, which is indirectly related to anxiety [27]. Additionally, BC changes in the right middle occipital gyrus, right temporal pole, and left paracentral lobule of the aPD group may be indicative of dysfunction in multimodal integration and motor planning regions associated with anxiety [28]. In addition, a previous graph theory study on PD patients found that BC of the left paracentral lobule was altered in PD patients with mild cognitive impairment, which may also illustrate the coupling association between anxiety and cognitive impairment, but further analysis is still needed [29]. In the higher frequency range (0.027–0.073 Hz), increased BC in the left middle temporal gyrus in aPD patients compared to naPD may indicate a reorganization or compensatory mechanism linked to the emotional and cognitive aspects of anxiety in PD [30]. The middle temporal gyrus is involved in multiple cognitive processes, including memory, language, and socio-emotional processing. An increase in BC may indicate that these functional areas are particularly active in processing anxiety-related information, requiring additional neural resources to handle complex emotional and cognitive information related to anxiety. The middle temporal gyrus is involved in multiple cognitive processes, including memory, language, and socio-emotional processing [31]. An increase in BC may indicate that these functional areas are particularly active in processing anxiety-related information, requiring additional neural resources to handle complex emotional and cognitive information related to anxiety.
Our DC analysis shows that compared to naPD and HC, the aPD group had broader changes in centrality, indicating a possible over-connectivity related to enhanced anxiety-related processing. The reduced DC in the right paracentral lobule in aPD patients compared to HC might suggest decreased motor and sensory integration capability, potentially contributing to the affective components of anxiety.
The patterns of change in nodal efficiency that we observed reinforce the evidence that functional integration and segregation in brain networks have been altered in PD with comorbid anxiety. Increased nodal efficiency in regions associated with emotion processing (such as the left middle temporal gyrus) in aPD compared to naPD could reflect an adaptive response to anxiety. Based on further correlational analysis, we found that the increase in nodal efficiency within the left middle temporal gyrus in the frequency range of 0.0027–0.0073 Hz is negatively correlated with anxiety scores, then this change can be considered adaptive. In this case, the brain network’s adjustment might help individuals to more effectively process emotional stimuli, reduce feelings of anxiety, and improve quality of life. An adaptive response means that despite the presence of neurodegenerative changes due to the disease, the brain is still able to maintain or achieve functional balance by enhancing certain neural pathways. On the other hand, reduced efficiency in areas like the right paracentral lobule may suggest a decreased functionality in sensorimotor areas, which could also be related to increased anxiety symptoms.
The different patterns of nodal centrality and efficiency changes across oscillatory frequencies we observed indicate that anxiety comorbid with PD is a multifaceted phenomenon in brain network dynamics. This raises the possibility that different aspects of the anxiety symptomatology in PD are represented differently across oscillatory frequencies, in line with an increasing body of literature that suggests frequency-specific roles in brain function and dysfunction. Physiological functions are dependent on oscillation-specific power distributions, but the complexity of neuronal properties and cellular structures contributes to oscillation power [32]. Node properties in large-scale networks facilitate the assessment of large network integration [33, 34].
Our findings regarding oscillation-specific differences in PD with anxiety hold potential clinical significance. Targeted interventions, such as neuromodulation, might be optimized by considering the individual’s unique pattern of brain network changes across different frequency bands. This specificity could guide more individualized and effective treatment approaches in the future. Moreover, identifying connectivity patterns associated with anxiety in PD could help with early diagnosis and potentially monitor treatment responses.
Of course, our study is not without limitations. Although we have demonstrated associations between altered nodal properties and comorbid anxiety in PD, causality cannot be established due to the cross-sectional nature of the study. Longitudinal research would provide a better understanding of the evolving changes in brain network dynamics associated with the progression of anxiety symptoms in PD. Additionally, our research prompts further exploration into the molecular and physiological underpinnings of these oscillation-specific brain network changes. This could involve multimodal imaging studies combining rs-fMRI with other techniques such as PET or EEG, thus offering a more detailed picture of the interplay between brain connectivity, neurochemical changes, and clinical symptoms in PD with anxiety.
In summary, our study enhances the understanding of the neurobiological substrates of anxiety in PD, presenting oscillatory frequency bands as an important consideration in the analysis of brain network alterations. As rs-fMRI becomes increasingly accessible for clinical research, our findings may have implications for the future of personalized medicine, where the modulation of specific frequency-dependent network patterns could be a target for novel therapeutic strategies.
Conclusion
Our study has initiated an exploration into the oscillation-specific nodal properties associated with anxiety in PD. We have observed distinct nodal attribute patterns within various frequency bands, offering new insights that could pave the way for understanding and conceptualizing anxiety in PD from a neurobiological standpoint. Our research garners evidence for the potential of oscillation frequencies to serve as biomarkers for the neurophysiological changes related to anxiety in PD patients. In the future, interventions may be optimized by personalizing them according to each individual’s specific brain network alterations, which are delineated by oscillation patterns. This precision in tailoring could enhance the effectiveness of therapeutic strategies, such as neuromodulation. Such an individualized approach would also facilitate the early detection of the development of anxiety in PD patients and improve monitoring of treatment responses, significantly impacting the quality of life and disease management for those affected.
ACKNOWLEDGMENTS
We would like to thank all authors for this study.
FUNDING
This paper is supported by the Joint Fund for Medical Artificial Intelligence (No.: MAI2023Q023), Doctoral Research Fund of the First Affiliated Hospital of USTC (No.: RC2021121) and Excellent Scientific research and innovation Team Project in Anhui Province (2023AH010080).
CONFLICT OF INTEREST
The authors have no conflict of interest to report.
DATA AVAILABILITY
Raw data have been presented in the Supplementary Material. Please contact the corresponding author to share the original image data after explaining the purpose.
SUPPLEMENTARY MATERIAL
[1] The supplementary material is available in the electronic version of this article: https://dx.doi.org/10.3233/JPD-240055.
REFERENCES
[1] | Tolosa E , Wenning G , Poewe W ((2006) ) The diagnosis of Parkinson’s disease. Lancet Neurol 5: , 75–86. |
[2] | Chang B , Mei J , Ni C , Xiong C , Chen P , Jiang M , Niu C ((2023) ) Development and validation of a prediction model for anxiety improvement after deep brain stimulation for Parkinson disease. Brain Sci 13: , 219. |
[3] | Ray S , Agarwal P ((2020) ) Depression and anxiety in Parkinson disease. Clin Geriatr Med 36: , 93–104. |
[4] | Perepezko K , Naaz F , Wagandt C , Dissanayaka N , Mari Z , Nanavati J , Bakker A , Pontone G ((2021) ) Anxiety in Parkinson’s disease: A systematic review of neuroimaging studies. J Neuropsychiatry Clin Neurosci 33: , 280–294. |
[5] | Kwon OY , Kam SC , Choi JH , Do JM , Hyun JS ((2011) ) Effects of sertraline on brain current source of the high beta frequency band: Analysis of electroencephalography during audiovisual erotic stimulation in males with premature ejaculation. Int J Impot Res 23: , 213–219. |
[6] | Hillyer A , Sharma M , Kuurstra A , Rosehart H , Menon R , Morrow S ((2023) ) Association between limbic system lesions and anxiety in persons with multiple sclerosis. Mult Scler Relat Disord 79: , 105021. |
[7] | Chang B , Mei J , Ni C , Niu C ((2023) ) Functional connectivity and anxiety improvement after subthalamic nucleus deep brain stimulation in Parkinson’s disease. Clin Interv Aging 18: , 1437–1445. |
[8] | Buzsáki G , Draguhn A ((2004) ) Neuronal oscillations in cortical networks. Science 304: , 1926–1929. |
[9] | Gonzalez-Burgos G , Cho R , Lewis D ((2015) ) Alterations in cortical network oscillations and parvalbumin neurons in schizophrenia. Biol Psychiatry 77: , 1031–1040. |
[10] | Guan X , Zeng Q , Guo T , Wang J , Xuan M , Gu Q , Wang T , Huang P , Xu X , Zhang M ((2017) ) Disrupted functional connectivity of basal ganglia across tremor-dominant and Akinetic/rigid dominant Parkinson’s disease. Front Aging Neurosci 9: , 360. |
[11] | Song X , Hu X , Zhou S , Xu Y , Zhang Y , Yuan Y , Liu Y , Zhu H , Liu W , Gao J ((2015) ) Association of specific frequency bands of functional MRI signal oscillations with motor symptoms and depression in Parkinson’s disease. Sci Rep 5: , 16376. |
[12] | Hou Y , Wu X , Hallett M , Chan P , Wu T ((2014) ) Frequency-dependent neural activity in Parkinson’s disease. Hum Brain Mapp 35: , 5815–5833. |
[13] | Rubinov M , Sporns O ((2010) ) Complex network measures of brain connectivity: Uses and interpretations. Neuroimage 52: , 1059–1069. |
[14] | Leitgeb E , Śterk M , Petrijan T , Gradiśnik P , Gosak M ((2020) ) The brain as a complex network: Assessment of EEG-based functional connectivity patterns in patients with childhood absence epilepsy. Epileptic Disord 22: , 519–530. |
[15] | Wang J , Wang X , Xia M , Liao X , Evans A , He Y ((2015) ) GRETNA: A graph theoretical network analysis toolbox for imaging connectomics. Front Hum Neurosci 9: , 386. |
[16] | Liao X , Vasilakos A , He Y ((2017) ) Small-world human brain networks: Perspectives and challenges. Neurosci Biobehav Rev 77: , 286–300. |
[17] | Han Y , Wang J , Zhao Z , Min B , Lu J , Li K , He Y , Jia J ((2011) ) Frequency-dependent changes in the amplitude of low-frequency fluctuations in amnestic mild cognitive impairment: A resting-state fMRI study. Neuroimage 55: , 287–295. |
[18] | Hong J , Muller-Oehring E , Pfefferbaum A , Sullivan E , Kwon D , Schulte T ((2018) ) Aberrant blood-oxygen-level-dependent signal oscillations across frequency bands characterize the alcoholic brain. Addict Biol 23: , 824–835. |
[19] | Li X , Zhou J , He R , Lian J , Jia J , Hsu C , Yuan S , Chen Z ((2023) ) A study on the effects of the Qihuang Needle therapy on patients with Parkinson’s disease. Front Neurol 13: , 1022057. |
[20] | Chang B , Xiong C , Ni C , Chen P , Jiang M , Mei J , Niu C ((2023) ) Prediction of STN-DBS for Parkinson’s disease by uric acid-related brain function connectivity: A machine learning study based on resting state function MRI. Front Aging Neurosci 15: , 1105107. |
[21] | Jia X , Wang J , Sun H , Zhang H , Liao W , Wang Z , Yan C , Song X , Zang Y ((2019) ) RESTplus: An improved toolkit for resting-state functional magnetic resonance imaging data processing. Sci Bull (Beijing) 64: , 953–954. |
[22] | Dretsch M , Rangaprakash D , Katz J , Daniel T , Goodman A , Denney T , Deshpande G ((2019) ) Strength and temporal variance of the default mode network to investigate chronic mild traumatic brain injury in service members with psychological trauma. J Exp Neurosci 13: , 1179069519833966. |
[23] | Zhang Y , Mao Z , Pan L , Ling Z , Liu X , Zhang J , Yu X ((2019) ) Frequency-specific alterations in cortical rhythms and functional connectivity in trigeminal neuralgia. Brain Imaging Behav 13: , 1497–1509. |
[24] | Guan X , Guo T , Zeng Q , Wang J , Zhou C , Liu C , Wei H , Zhang Y , Xuan M , Gu Q , Xu X , Huang P , Pu J , Zhang B , Zhang M ((2019) ) Oscillation-specific nodal alterations in early to middle stages Parkinson’s disease. Transl Neurodegener 8: , 36. |
[25] | Carey G , Lopes R , Viard R , Betrouni N , Kuchcinski G , Devignes Q , Defebvre L , Leentjens A , Dujardin K ((2020) ) Anxiety in Parkinson’s disease is associated with changes in the brain fear circuit. Parkinsonism Relat Disord 80: , 89–97. |
[26] | Disbrow E , Glassy N , Dressler E , Russo K , Franz E , Turner R , Ventura M , Hinkley L , Zweig R , Nagarajan S , Ledbetter C , Sigvardt K ((2022) ) Cortical oscillatory dysfunction in Parkinson disease during movement activation and inhibition. PLoS One 17: , e0257711. |
[27] | Frick A , Engman J , Alaie I , Björkstrand J , Faria V , Gingnell M , Wallenquist U , Agren T , Wahlstedt K , Larsson E , Morell A , Fredrikson M , Furmark T ((2014) ) Enlargement of visual processing regions in social anxiety disorder is related to symptom severity. Neurosci Lett 583: , 114–119. |
[28] | Killgore W , Schwab Z , Kipman M , Deldonno S , Weber M ((2013) ) Insomnia-related complaints correlate with functional connectivity between sensory-motor regions. Neuroreport 24: , 233–240. |
[29] | Díez-Cirarda M , Ibarretxe-Bilbao N , Peña J , Ojeda N ((2018) ) Neurorehabilitation in Parkinson’s disease: A critical review of cognitive rehabilitation effects on cognition and brain. Neural Plast 2018: , 2651918. |
[30] | Marwood L , Wise T , Perkins A , Cleare A ((2018) ) Meta-analyses of the neural mechanisms and predictors of response to psychotherapy in depression and anxiety. Neurosci Biobehav Rev 95: , 61–72. |
[31] | Scott H , Wimmer K , Pasternak T , Snyder A ((2023) ) Altered task demands lead to a division of labour for sensory and cognitive processing in the middle temporal area. Eur J Neurosci 57: , 1561–1576. |
[32] | Baria A , Baliki M , Parrish T , Apkarian A ((2011) ) Anatomical and functional assemblies of brain BOLD oscillations. J Neurosci 31: , 7910–7919. |
[33] | Bassett D , Bullmore E ((2017) ) Small-world brain networks revisited. Neuroscientist 23: , 499–516. |
[34] | Lin L , Fu Z , Jin C , Tian M , Wu S ((2018) ) Small-world indices via network efficiency for brain networks from diffusion MRI. Exp Brain Res 236: , 2677–2689. |