Identifying Subtle Motor Deficits Before Parkinson’s Disease is Diagnosed: What to Look for?
Abstract
Motor deficits typical of Parkinson’s disease (PD), such as gait and balance disturbances, tremor, reduced arm swing and finger movement, and voice and breathing changes, are believed to manifest several years prior to clinical diagnosis. Here we describe the evidence for the presence and progression of motor deficits in this pre-diagnostic phase in order to provide suggestions for the design of future observational studies for an effective, quantitatively oriented investigation. On the one hand, these future studies must detect these motor deficits in as large (potentially, population-based) cohorts as possible with high sensitivity and specificity. On the other hand, they must describe the progression of these motor deficits in the pre-diagnostic phase as accurately as possible, to support the testing of the effect of pharmacological and non-pharmacological interventions. Digital technologies and artificial intelligence can substantially accelerate this process.
INTRODUCTION
Timely identification of persons in their early stages of a neurodegenerative condition, such as Parkinson’s disease (PD), is crucial to initiate early treatment, potentially slowing disease progression [1]. There is growing evidence that motor deficits begin to appear in PD as early as two to perhaps ten years before clinical diagnosis is possible [2–7], and that these deficits do also progress at follow-up [6, 8–12]. This phase is hereinafter referred to as the pre-diagnostic (motor) phase, which is congruent with the term prodromal (motor) phase used by some other authors. The ability to capture subtle motor deficits and their progression has improved tremendously in recent years with advances in digital technology and new analytical methods that allow quantification and reliable recording over long periods of time [13–16].
Motor deficits in the pre-diagnostic phase of Parkinson’s disease
Postuma and colleagues were among the first that reported on the temporal appearance of motor deficits in 20 patients with REM sleep behavior disorder (RBD) who later developed clinically established PD [6]. Voice and face akinesia appeared nearly 9 years prior to clinical diagnosis followed by finger tapping deficits (8 years), mobility deficits (6 years), and rigidity and limb bradykinesia (4 years). The authors reported that these signs continued to deteriorate during the observation period [6].
Alteration of gait is a core feature of most PD patients at diagnosis [17]. In a longitudinal study on 680 healthy older adults, walking under supervised conditions was examined using a wearable sensor placed on the lower back. During the 7-year follow up period, 16 participants were diagnosed with clinically established PD. The study showed lower rhythm, higher variability, and higher asymmetry of steps in the latter participants, 4.5 years before diagnosis [12]. Gait speed and step length were the parameters of progression during the observation period [12]. Baseline gait data were comparable with another study investigating patients with high risk for PD (LRRK2-G2019S mutation carriers). The study showed increased stride time variability in carriers compared to age-matched non-carriers [18]. As the latter parameter is a marker for dynamic balance [19], it is possible that balance is also affected in this PD phase. This hypothesis is supported by another study which analyzed the medical records of around 8,000 patients before they were diagnosed with PD from the Health Improvement Network UK primary care database. This study found that balance problems were observed around 4 years before PD diagnosis was possible [2]. A cross-sectional, sensor-based study describing reduced static balance parameters in association with risk markers for PD [20] also points in this direction.
Arm swing asymmetry was observed in LRRK2-G2019S mutation carriers with and without clinically established PD [21]. The mutation is associated with a substantially increased risk of developing PD. Arm swing asymmetry, variability and decreased axial rotation smoothness were apparent in non-manifesting carriers but continued to decline in carriers after PD diagnosis [21]. Arm swing changes observed in diagnosed PD patients without relevant gait deficits suggest that this deficit may indeed have high potential as a motor marker in pre-diagnostic PD, especially as a progression marker [22]. A recent study [5], using accelerometry data from the wrist collected in the usual environment of UK Biobank participants for a week, observed the predictive ability of lowered acceleration of the wrist in identifying the pre-diagnostic motor phase of PD. When compared to genetic, lifestyle, blood biochemistry or other ‘common’ pre-diagnostic PD markers, these measures showed superior performance in distinguishing 113 pre-diagnostic PD patients up to 7 years before diagnosis from matched controls [5].
Another promising motor marker in the pre-diagnostic phase of PD may be finger tapping deficits. PD patients have been shown to have lower typing frequency and slower typing velocity than controls and display a sequence effect [23]. These measures may be related to the development of general bradykinesia, also reflected by altered handwriting [10]. In conjunction with previous RBD reports [6], a recent study showed that patients with RBD differed from controls in finger tapping amplitude and velocity decrement, with the latter parameter showing a significant relationship with the Movement Disorders Society –Unified Parkinson’s Disease Rating Scale, similar to PD patients [24].
Another study using an app-based approach in a large cohort of RBD patients demonstrated the presence of motor deficits including tremor, tapping speed, gait changes and voice [25]. Analyses of video recordings reported changes in speech variability as early as five years before PD diagnosis [26]. Smartphone speech data of people at high risk for PD, diagnosed PD patients and controls confirmed these findings [27]. Acoustic measurements derived from spontaneous speech, including fundamental frequency variability, pause interval duration, and speech timing rate, proved highly sensitive, effectively distinguishing between PD and control groups.
The restriction of respiratory function (reflected by breathing changes) could potentially also be counted among the interesting markers not only for clinically established [28] but also for pre-diagnostic PD. A recently published study using an AI-based algorithm not only demonstrated the high discriminative power of nocturnal respiratory signals between large cohorts of PD patients and controls but also provided evidence that these changes may occur even before a PD diagnosis is possible [29].
Taken together, there is emerging evidence for the occurrence of motor deficits in a pre-diagnostic phase of PD. However, they do not yet play a relevant role in the definition of this phase [30]. Why has it not been possible to date to define effective motor markers for this PD phase?
GAPS NOT YET ADEQUATELY ADDRESSED FOR ACCURATE DESCRIPTION OF MOTOR DEFICITS IN THE PRE-DIAGNOSTIC PD PHASE
There are several reasons why existing knowledge of the pre-diagnostic motor phase of PD is currently insufficient to 1) define with a high degree of certainty individuals who are in this phase across populations, and 2) to define progression trajectories that allow the success of, e.g., neuromodulatory agents to be measured. These deficits may be (mainly) due to:
The small size of the study cohorts that were examined in previous studies
Most studies currently available have examined one to several dozen participants. These small sample sizes are due to the difficulties in finding individuals in this phase. Conversely, they almost certainly cannot cover the diversity of the disease. In addition, although there is currently no convincing evidence that subtypes of PD already exist in the pre-diagnostic disease phase, it is likely that the course of the disease and its phenotypic manifestation already differ in this phase, reflecting differences in genetic, environmental, behavioral, and habitual characteristics between individuals [31, 32]. The recent paper by [5], examining data collected in the UK Brain Biobank is an example of the potential of utilizing existing population-based cohorts to explore motor deficits in the pre-diagnostic phase of PD. These types of studies are important because they aim to identify PD-specific deficits while taking into account comorbidities in the general population, which ultimately leads to higher accuracy in detection [32, 33]. However, rigorous verification is needed [32, 33].
The localization, complexity, and progression of motor deficits in the pre-diagnostic phase of PD, how they differ from age-related changes and how they are compensated
It is not yet entirely clear which motor deficits (tend to) occur first in this disease, how specific are they, in which sequence they may occur, how they progress, and which motor deficit poses the greatest risk for the future development of clinically established PD. For example, we know from early “post-diagnostic” PD that there is often a laterality of the cardinal symptoms [23], even with dissociation across these symptoms [34]. To what extent this already exists in the pre-diagnostic phase, and whether it persists consistently remains to be clarified. Moreover, several imaging studies have shown that compensatory mechanisms for motor deficits are particularly active in the early stages of neurodegenerative diseases, including PD [35, 36]. This may mask some deficits, particularly in a supervised assessment context [20, 37, 38].
The lack of evidence that motor deficits in pre-diagnostic PD can (also) be detected in everyday life
If we assume that, in principle, population-wide screening in the home environment is needed to detect a relevant proportion of people in the pre-diagnostic phase of PD, we need to ensure that these assessments are valid. Although there is some evidence that motor deficits in the pre-diagnostic PD phase are evident not only in the doctor’s office but also in the home environment of those affected [4], there is a significant lack of studies that support this on a quantitative and objective level. This is even more important as the presentation of motor deficits in PD may differ depending on whether they are measured in a usual environment or the clinic [39, 40].
A lack of knowledge about the context in which movements are performed in everyday life
Measurement of movement in everyday life has become straightforward (e.g., step counting, total energy expenditure, sleep patterns). However, all our movements take place within a context, such as where the person is, who they are interacting with, what food they have eaten (and how), and what the weather conditions are, and these contexts influence the movements [39, 41]. Interestingly, dopaminergic deficiency has been shown to be associated with deficits in the selection of context-adapted movement [42]. This association suggests that context factors should be collected particularly in PD-related studies. However, these context factors have been difficult or even impossible to collect so far. This may be associated with unacceptable aspects on the part of the study participants (e.g., filling in diaries) and data protection issues (e.g., GPS data).
Technology-based measurements that build only on clinically informed concepts of (motor) deficits
PD-related deficits have been primarily defined by medical professionals and patients. This is not, at least in some constellations, the language and granularity with which digital technology and new analytical methods such as artificial intelligence operate. The latter have no ability or “interest” to consider interpretative and comprehensive aspects of disease-related deficits, the former do.
It is therefore necessary for the quantitative assessment of deficits, also in the pre-diagnostic phase of PD, to critically examine the “composition” of these deficits and, if possible, to disaggregate and operationalize them so that the assessment is adapted to the capabilities of the new technology [16].
Assessment technologies do not fit into the lives of those assessed
When patients use medical devices at home, the technologies need to fit into their lives. It was recently reported that low effort of use, low disruption to their daily lives and good support are important enablers for the success of such evaluation strategies [43–45].
Furthermore, the definition of a subtle PD-specific motor deficit may be complicated by the fact that motor and non-motor deficits can overlap, even in the pre-diagnostic disease phase. Examples are deficits in salivation, speech, and facial expression [10]. In addition, although mild parkinsonian signs are associated with an increased risk of future clinically established PD, they are also more likely to occur in an aging population and may therefore reflect multiple pathologies [46].
PROPOSED NEXT STEPS IN DETECTION, QUANTIFICATION, AND FOLLOW-UP OF PRE-DIAGNOSTIC MOTOR DEFICITS IN PD
We posit that for the successful detection, quantification, and follow-up evaluation of pre-diagnostic motor deficits in PD, the following priorities need to be addressed:
Prospective studies are always superior to retrospective analyses, as the latter may have limitations that prevent meaningful interpretation of the results. In addition, it is essential to define a priori the concept of interest and the context of use in such studies (see Fig. 1A for an overview of the number of studies already available investigating pre-diagnostic motor deficits). In the development of the study design, it should be consistently ensured that existing knowledge from clinical and laboratory-based studies is implemented. Building on this, consideration needs to be given to which digital technologies have the highest potential to achieve an accurate answer to the study hypothesis (and not vice versa [47]). The definition of specific aspects of disease-related deficits should take into account the impressive possibilities offered by digital technologies. This has already been taken up for some deficits. For example, it is feasible to distinguish between static [48] and dynamic balance [19], and it is widely accepted that stride time variability is much more closely associated with dynamic imbalance than other gait parameters [49, 50]. For bradykinesia, a new framework has recently been proposed that distinguishes between bradykinesia, hypokinesia, sequence effect, hesitations/halts, akinesia, and oligokinesia in the context of reduced movement [51], making the feature much more amenable to detection with new technology.
Fig. 1
A) Number of currently available studies for the assessment of motor deficits in the (possible) pre-diagnostic phase of Parkinson’s disease (PD). The following search strategy was used in PubMed: “digital assessment”, “mobile health technology”, “gait”, “voice”, “balance”, “postural instability”, “bradykinesia”, “tremor” AND “prodromal Parkinson’s disease”, “idiopathic REM sleep behavior disorders”, “Parkinson’s disease”. B) Motor features appear about 2–10 years before the diagnosis of PD. To identify pre-diagnostic PD motor deficits, they must show a deviation from “normal” (i.e., no pre-diagnostic PD phase). This deviation should be measured in as large cohorts as possible, preferably population-based. For this purpose, it would be advisable to use markers that can be collected with the simplest possible methods and potentially in the home environment. If this defines a cohort that is (for a relevant part) in a pre-diagnostic PD phase, more complex methods can be used to determine the progression of motor features in this phase of PD, as the cohort is now smaller. Sup, studies using supervised, task-based assessments in the clinic; UnSup, studies using unsupervised assessment strategies, mostly in the home environment.
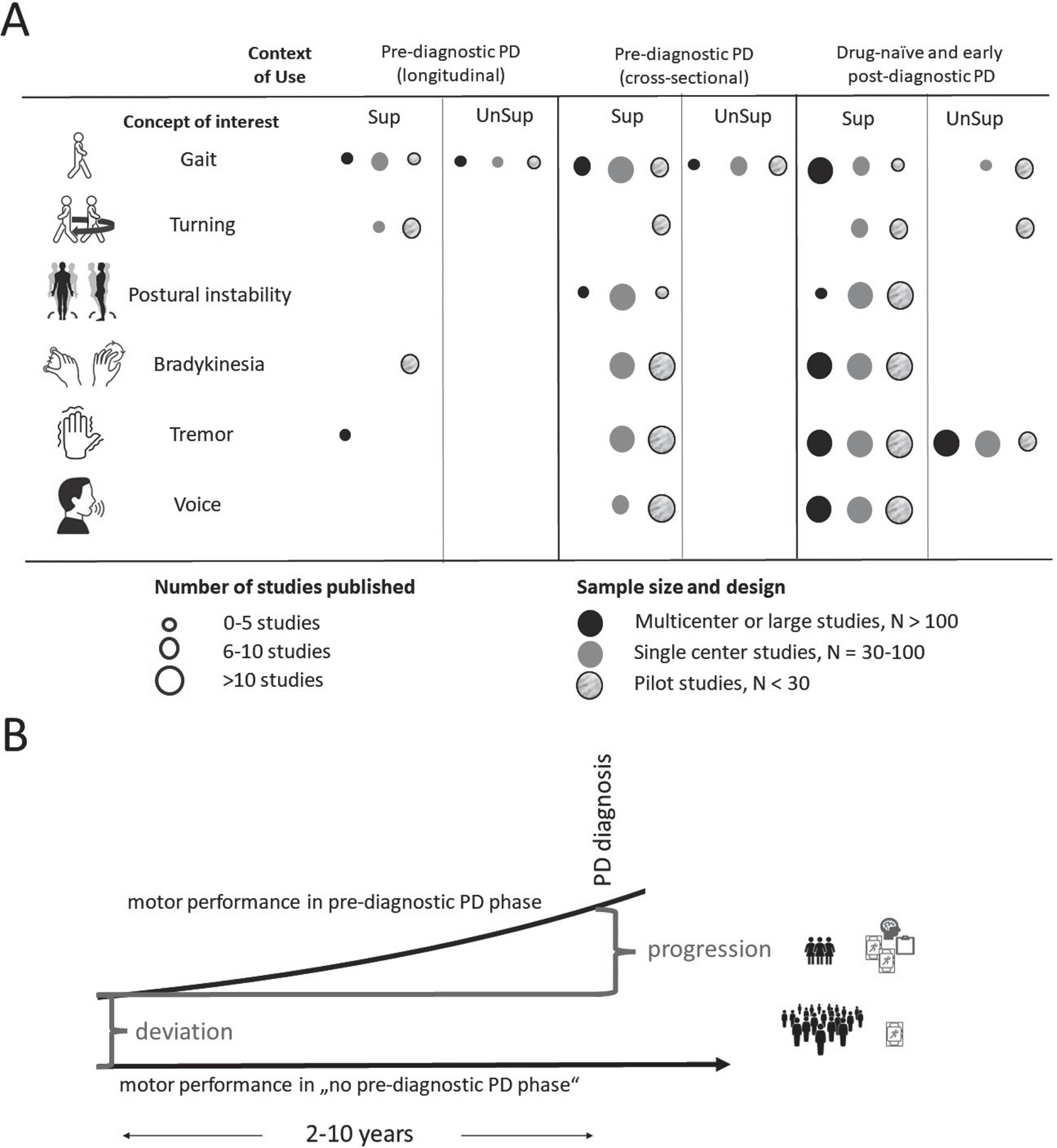
Clinical and laboratory studies should continue to invest in this area. They provide unique information about the characteristics of these features, especially when challenging experimental conditions are used that can unmask subtle motor deficits. These types of studies can anchor clinical evaluation and perform technical and clinical validation of the measurement tools and parameters of interest, ensuring that they are fit for purpose, for example as identification or progression markers. Recent work has brought new options that offer validation also in the home environment [52]. This opens up the possibility of collecting data in everyday life settings and in large cohorts.
For the identification of motor deficits in the pre-diagnostic PD phase, we propose to prioritize measurement in everyday life [53]. We follow here the idea that, for feasibility and economic reasons [54], population-wide assessment without a clinical visit should ultimately be the goal for this purpose (Fig. 1B). Consequently, research should focus on motor deficits that are detectable in the person’s usual ‘being’. It is very likely that this is possible and effective. For example, the Rotterdam study, comparing 109 people in a pre-diagnostic PD phase with 1090 controls, showed that the former had a continuous decline in instrumented activities of daily living (such as the ability to use the telephone, which was also shown for early “post-diagnostic” PD [55, 56], do laundry, shop, prepare food, do household chores, use transport, be responsible for own medication and be able to manage finances), with trajectories that differed from those of the controls from about 6 years before diagnosis [4]. The study team should always bear in mind that assessment strategies need to be somehow integrated into different aspects of the study participants’ daily lives without being too burdensome and intruding on their privacy. It is also important to remember that decline in activities of daily living and physical activity are not specific to PD, and any changes observed should take into account aging and co-morbidities as covariates [57, 58].
Based on the data currently available, changes in dexterity, voice, arm swing, gait, balance, and breathing (at least at night) appear to be the most promising parameters for detecting people at a pre-diagnostic phase of PD. The widespread use of consumer digital devices such as smartphones and smartwatches in the population makes it potentially possible to collect such parameters on a large scale and unobtrusively. The UK Brain Biobank study using a wrist-worn accelerometer over 7 days in pre-diagnostic PD patients in the home setting has demonstrated the effectiveness of this approach [5]. Other screening technologies are currently being developed by several groups, focusing on deficits in dexterity, gait and voice [60], and tremor [59]. These technology-enabled options with artificial intelligence can be used to remotely assess patients’ conditions in their natural home environment, facilitating a more comprehensive assessment and empowering patients to monitor their disease.
To develop progression markers in this pre-diagnostic phase, longitudinal cohort studies are needed that examine different disease trajectories to identify the weight of progression of different motor deficits (Fig. 1B). Network analysis is an interesting approach in the field of digital devices that can potentially help here. We understand this as the analysis of data obtained from multiple (similar or different) sensors, e.g., worn on the body, that record physiological processes and movement/mobility. Examples can be the calculation of interlimb coordination [61, 62], which has shown very high potential for measuring the progression of Duchenne muscular atrophy [63] and Friedreich’ ataxia [64]. Digital data measuring mobility and autonomic aspects (e.g., heartbeat) have been used to detect fatigue [65] and freezing of gait in PD [66], with promising results.
When designing prospective studies in the field of pre-diagnostic PD, new ways of collecting contextual factors must be explored. Digital diaries, for example, can be used for this purpose. It is also expected that in the near future it will be possible to extract contextual information from the digital tools that study participants wear on their bodies to record mobility and physiological parameters. Interesting options arise here through (additional) recording of environmental factors (such as daylight) and through more restrictive data extraction approaches, e.g., from GPS data (one possibility is the extraction of relative instead of absolute coordinates).
Approaches to data analysis should be able to deal with large amounts of data and have a ‘learning’ potential. Inspiration comes here, e.g., from the evaluation of pathological image data with the help of a multimodal artificial intelligence with both image and text understanding, which manages to classify pathological images with high accuracy using a data set that includes pathological images with text [67]. A similar approach is conceivable for artificial intelligence that analyses voice recordings and videos of a person’s movements. For example, it could be used to analyze a person who regularly gives speeches on his or her birthday or takes part in the annual city run, and the software would receive audio and video recordings that could be used to more precisely identify a pathology. Data should also be made available as a repository, e.g., for subsequent alternative and meta-analyses [68, 69]. We urge the field to foster open access data in a pre-competitive space to allow for faster progress of the field.
CONCLUSION
In conclusion, detection and monitoring of motor deficits in the pre-diagnostic phase of PD are crucial for the provision of effective treatment and understanding disease progression. Recent advances in digital technology have greatly improved our ability to capture subtle motor deficits and track their progression over time. Promising markers may be extracted from voice, arm swing, gait, balance, and breathing. However, there are still several challenges to address in future studies, including the need for larger and more diverse study cohorts that may focus on either identification or evaluation of progression in this phase, understanding the sequence of motor deficits, and validating assessments in everyday life settings. The future lies in prospective studies, advanced data analysis, and the integration of digital technology to identify and monitor motor deficits in the pre-diagnostic PD phase accurately.
ACKNOWLEDGMENTS
The authors have no acknowledgments to report.
FUNDING
The authors have no funding to report.
CONFLICT OF INTEREST
The authors have no conflict of interest to report.
REFERENCES
[1] | Noyce AJ , Rees RN , Acharya AP , Schrag A (2018) An early diagnosis is not the same as a timely diagnosis of Parkinson’s disease. F1000Res 7, F1000 Faculty Rev-1106. |
[2] | Schrag A , Horsfall L , Walters K , Noyce A , Petersen I ((2015) ) Prediagnostic presentations of Parkinson’s disease in primary care: A case-control study. Lancet Neurol 14: , 57–64. |
[3] | Heinzel S , Berg D , Gasser T , Chen H , Yao C , Postuma RB ((2019) ) Update of the MDS research criteria for prodromal Parkinson’s disease. Mov Disord 34: , 1464–1470. |
[4] | Darweesh SKL , Verlinden VJA , Stricker BH , Hofman A , Koudstaal PJ , Ikram MA ((2017) ) Trajectories of prediagnostic functioning in Parkinson’s disease. Brain 140: , 429–441. |
[5] | Schalkamp A-K , Peall KJ , Harrison NA , Sandor C ((2023) ) Wearable movement-tracking data identify Parkinson’s disease years before clinical diagnosis. Nat Med 29: , 2048–2056. |
[6] | Postuma RB , Lang AE , Gagnon JF , Pelletier A , Montplaisir JY ((2012) ) How does parkinsonism start? Prodromal parkinsonism motor changes in idiopathic REM sleep behaviour disorder. Brain 135: , 1860–1870. |
[7] | Simonet C , Mahlknecht P , Marini K , Seppi K , Gill A , Bestwick JP , Lees AJ , Giovannoni G , Schrag A , Noyce AJ ((2023) ) The emergence and progression of motor dysfunction in individuals at risk of Parkinson’s disease. Mov Disord 38: , 1636–1644. |
[8] | Joza S , Hu MT , Jung K-Y , Kunz D , Stefani A , Dušek P , Terzaghi M , Arnaldi D , Videnovic A , Schiess MC , Hermann W , Lee J-Y , Ferini-Strambi L , Lewis SJG , Leclair-Visonneau L , Oertel WH , Antelmi E , Sixel-Döring F , Cochen De Cock V , Liguori C , Liu J , Provini F , Puligheddu M , Nicoletti A , Bassetti CLA , Bušková J , Dauvilliers Y , Ferri R , Montplaisir JY , Lawton M , Kim H-J , Bes F , Högl B , Šonka K , Fiamingo G , Mattioli P , Lavadia ML , Suescun J , Woo KA , Marelli S , Martens KE , Janzen A , Plazzi G , Mollenhauer B , Fernandes M , Li Y , Cortelli P , Figorilli M , Cicero CE , Schaefer C , Guiraud L , Lanza G , Gagnon J-F , Sunwoo J-S , Ibrahim A , Girtler N , Trenkwalder C , Baldelli L , Pelletier A , Postuma RB ((2023) ) Progression of clinical markers in prodromal Parkinson’s disease and dementia with Lewy bodies: A multicentre study. Brain 146: , 3258–3272. |
[9] | Postuma RB , Iranzo A , Hu M , Högl B , Boeve BF , Manni R , Oertel WH , Arnulf I , Ferini-Strambi L , Puligheddu M , Antelmi E , Cochen De Cock V , Arnaldi D , Mollenhauer B , Videnovic A , Sonka K , Jung KY , Kunz D , Dauvilliers Y , Provini F , Lewis SJ , Buskova J , Pavlova M , Heidbreder A , Montplaisir JY , Santamaria J , Barber TR , Stefani A , Louis SEK , Terzaghi M , Janzen A , Leu-Semenescu S , Plazzi G , Nobili F , Sixel-Doering F , Dusek P , Bes F , Cortelli P , Ehgoetz Martens K , Gagnon JF , Gaig C , Zucconi M , Trenkwalder C , Gan-Or Z , Lo C , Rolinski M , Mahlknecht P , Holzknecht E , Boeve AR , Teigen LN , Toscano G , Mayer G , Morbelli S , Dawson B , Pelletier A ((2019) ) Risk and predictors of dementia and parkinsonism in idiopathic REM sleep behaviour disorder: A multicentre study. Brain 142: , 744–759. |
[10] | Fereshtehnejad SM , Yao C , Pelletier A , Montplaisir JY , Gagnon JF , Postuma RB ((2019) ) Evolution of prodromal Parkinson’s disease and dementia with Lewy bodies: A prospective study. Brain 142: , 2051–2067. |
[11] | Alotaibi F , Pelletier A , Gagnon JF , Montplaisir JY , Postuma RB ((2019) ) Prodromal marker progression in idiopathic rapid eye movement sleep behavior disorder: Sample size for clinical trials. Mov Disord 34: , 1914–1919. |
[12] | Del Din S , Elshehabi M , Galna B , Hobert MA , Warmerdam E , Suenkel U , Brockmann K , Metzger F , Hansen C , Berg D , Rochester L , Maetzler W ((2019) ) Gait analysis with wearables predicts conversion to Parkinson disease. Ann Neurol 86: , 357–367. |
[13] | Sánchez-Ferro Á , Elshehabi M , Godinho C , Salkovic D , Hobert MA , Domingos J , van Uem JMT , Ferreira JJ , Maetzler W ((2016) ) New methods for the assessment of Parkinson’s disease (2005 to 2015): A systematic review. Mov Disord 31: , 1283–1292. |
[14] | Bohlke K , Redfern MS , Rosso AL , Sejdic E ((2023) ) Accelerometry applications and methods to assess standing balance in older adults and mobility-limited patient populations: A narrative review. Aging Clin Exp Res 35: , 1991–2007. |
[15] | Guo CC , Chiesa PA , de Moor C , Fazeli MS , Schofield T , Hofer K , Belachew S , Scotland A ((2022) ) Digital devices for assessing motor functions in mobility-impaired and healthy populations: Systematic literature review. J Med Internet Res 24: , e37683. |
[16] | Chandrabhatla AS , Pomeraniec IJ , Ksendzovsky A ((2022) ) Co-evolution of machine learning and digital technologies to improve monitoring of Parkinson’s disease motor symptoms. NPJ Digit Med 5: , 32. |
[17] | Mirelman A , Bonato P , Camicioli R , Ellis TD , Giladi N , Hamilton JL , Hass CJ , Hausdorff JM , Pelosin E , Almeida QJ ((2019) ) Gait impairments in Parkinson’s disease. Lancet Neurol 18: , 697–708. |
[18] | Mirelman A , Gurevich T , Giladi N , Bar-Shira A , Orr-Urtreger A , Hausdorff JM ((2011) ) Gait alterations in healthy carriers of the LRRK2 G2019S mutation. Ann Neurol 69: , 193–197. |
[19] | Peterson DS , Van Liew C , Stuart S , Carlson-Kuhta P , Horak FB , Mancini M ((2020) ) Relating Parkinson freezing and balance domains: A structural equation modeling approach. Parkinsonism Relat Disord 79: , 73–78. |
[20] | Maetzler W , Mancini M , Liepelt-Scarfone I , Müller K , Becker C , van Lummel RC , Ainsworth E , Hobert M , Streffer J , Berg D , Chiari L ((2012) ) Impaired trunk stability in individuals at high risk for Parkinson’s disease. PLoS One 7: , e32240. |
[21] | Mirelman A , Bernad-Elazari H , Thaler A , Giladi-Yacobi E , Gurevich T , Gana-Weisz M , Saunders-Pullman R , Raymond D , Doan N , Bressman SB , Marder KS , Alcalay RN , Rao AK , Berg D , Brockmann K , Aasly J , Waro BJ , Tolosa E , Vilas D , Pont-Sunyer C , Orr-Urtreger A , Hausdorff JM , Giladi N ((2016) ) Arm swing as a potential new prodromal marker of Parkinson’s disease. Mov Disord 31: , 1527–1534. |
[22] | Mainka S , Lauermann M , Ebersbach G ((2023) ) Arm swing deviations in patients with Parkinson’s disease at different gait velocities. J Neural Transm 130: , 655–661. |
[23] | Panyakaew P , Duangjino K , Kerddonfag A , Ploensin T , Piromsopa K , Kongkamol C , Bhidayasiri R ((2023) ) Exploring the complex phenotypes of impaired finger dexterity in mild-to-moderate stage Parkinson’s disease: A time-series analysis. J Parkinsons Dis 13: , 977–990. |
[24] | Krupička R , Krýže P , Net’uková S , Duspivová T , Klempíŕ O , Szabó Z , Dušek P , Šonka K , Rusz J , Růžička E ((2020) ) Instrumental analysis of finger tapping reveals a novel early biomarker of parkinsonism in idiopathic rapid eye movement sleep behaviour disorder. Sleep Med 75: , 45–49. |
[25] | Arora S , Baig F , Lo C , Barber TR , Lawton MA , Zhan A , Rolinski M , Ruffmann C , Klein JC , Rumbold J , Louvel A , Zaiwalla Z , Lennox G , Quinnell T , Dennis G , Wade-Martins R , Ben-Shlomo Y , Little MA , Hu MT ((2018) ) Smartphone motor testing to distinguish idiopathic REM sleep behavior disorder, controls, and PD. Neurology 91: , E1528–E1538. |
[26] | Harel B , Cannizzaro M , Snyder PJ ((2004) ) Variability in fundamental frequency during speech in prodromal and incipient Parkinson’s disease: A longitudinal case study. Brain Cogn 56: , 24–29. |
[27] | Rusz J , Hlavnicka J , Tykalova T , Novotny M , Dusek P , Sonka K , Ruzicka E ((2018) ) Smartphone allows capture of speech abnormalities associated with high risk of developing Parkinson’s disease. IEEE Trans Neural Syst Rehabil Eng 26: , 1495–1507. |
[28] | Baille G , De Jesus AM , Perez T , Devos D , Dujardin K , Charley CM , Defebvre L , Moreau C ((2016) ) Ventilatory dysfunction in Parkinson’s disease. J Parkinsons Dis 6: , 463–471. |
[29] | Yang Y , Yuan Y , Zhang G , Wang H , Chen YC , Liu Y , Tarolli CG , Crepeau D , Bukartyk J , Junna MR , Videnovic A , Ellis TD , Lipford MC , Dorsey R , Katabi D ((2022) ) Artificial intelligence-enabled detection and assessment of Parkinson’s disease using nocturnal breathing signals. Nat Med 28: , 2207–2215. |
[30] | Berg D , Adler CH , Bloem BR , Chan P , Gasser T , Goetz CG , Halliday G , Lang AE , Lewis S , Li Y , Liepelt-Scarfone I , Litvan I , Marek K , Maetzler C , Mi T , Obeso J , Oertel W , Olanow CW , Poewe W , Rios-Romenets S , Schäffer E , Seppi K , Heim B , Slow E , Stern M , Bledsoe IO , Deuschl G , Postuma RB ((2018) ) Movement disorder society criteria for clinically established early Parkinson’s disease. Mov Disord 33: , 1643–1646. |
[31] | Liu SY , Zheng Z , Gu ZQ , Wang CD , Tang BS , Xu YM , Ma JH , Zhou YT , Feng T , Chen S Di , Chan P , Xu Y , Liu C , Wang Z , Wang P , Hu G , Wang W , Zhang B , Liang Z , Xie A , Luo B , Lü W , Sun S , Shao M , Liu Z , Liu Y , Ye Q , Chen H , Wan X , Sheng X ((2018) ) Prevalence of pre-diagnostic symptoms did not differ between LRRK2-related, GBA-related and idiopathic patients with Parkinson’s disease. Parkinsonism Relat Disord 57: , 72–76. |
[32] | Berg D , Borghammer P , Fereshtehnejad SM , Heinzel S , Horsager J , Schaeffer E , Postuma RB ((2021) ) Prodromal Parkinson disease subtypes - key to understanding heterogeneity. Nat Rev Neurol 17: , 349–361. |
[33] | Mirelman A , Rochester L , Simuni T , Hausdoff JM ((2023) ) Digital mobility measures to predict Parkinson’s disease. Lancet Neurol 22: , 1098–1100. |
[34] | Koh SB , Kwon DY , Seo WK , Kim JH , Kim JH , Lee SH , Oh K , Kim BJ , Park KW ((2010) ) Dissociation of cardinal motor signs in Parkinson’s disease patients. Eur Neurol 63: , 307–310. |
[35] | Caminiti SP , Pilotto A , Premi E , Galli A , Ferrari E , Gipponi S , Cottini E , Paghera B , Perani D , Padovani A ((2023) ) Dopaminergic connectivity reconfiguration in the dementia with Lewy bodies continuum. Parkinsonism Relat Disord 108: , 105288. |
[36] | Mak MKY , Cheung V , Ma S , Lu ZL , Wang D , Lou W , Shi L , Mok VCT , Chu WCW , Hallett M ((2016) ) Increased cognitive control during execution of finger tap movement in people with Parkinson’s disease. J Parkinsons Dis 6: , 639–650. |
[37] | Pilotto A , Zatti C , Hansen C , Rizzardi A , Catania M , Romijnders R , Purin L , Pasolini M , Galbiati A , Ferini-Strambi L , Berg D , Maetzler W , Padovani A ((2023) ) Turning alterations detected by mobile health technology in idiopathic REM sleep behavior disorder.. Research Square https://doi.org/10.21203/rs.3.rs-3076494/v1 [Preprint]. Posted online June 27, 2023. |
[38] | Maetzler W , Nieuwhof F , Hasmann SE , Bloem BR ((2013) ) Emerging therapies for gait disability and balance impairment: Promises and pitfalls. Mov Disord 28: , 1576–1586. |
[39] | Warmerdam E , Romijnders R , Hansen C , Elshehabi M , Zimmermann M , Metzger FG , von Thaler A-K , Berg D , Schmidt G , Maetzler W ((2021) ) Arm swing responsiveness to dopaminergic medication in Parkinson’s disease depends on task complexity. NPJ Parkinsons Dis 7: , 89. |
[40] | Warmerdam E , Hausdorff JM , Atrsaei A , Zhou Y , Mirelman A , Aminian K , Espay AJ , Hansen C , Evers LJW , Keller A , Lamoth C , Pilotto A , Rochester L , Schmidt G , Bloem BR , Maetzler W ((2020) ) Long-term unsupervised mobility assessment in movement disorders. Lancet Neurol 19: , 462–470. |
[41] | Lee YY , Tai CH , Fisher BE ((2022) ) Training in varying environmental contexts facilitates transfer of improved gait performance to new contexts for individuals with Parkinson disease: A randomized controlled trial. Arch Phys Med Rehabil 103: , 1917–1923. |
[42] | Pessiglione M , Guehl D , Agid Y , Hirsch EC , Féger J , Tremblay L ((2003) ) Impairment of context-adapted movement selection in a primate model of presymptomatic Parkinson’s disease. Brain 126: , 1392–1408. |
[43] | Mammen JR , Speck RM , Stebbins GT , Müller MLTM , Yang PT , Campbell M , Cosman J , Crawford JE , Dam T , Hellsten J , Jensen-Roberts S , Kostrzebski M , Simuni T , Barowicz KW , Cedarbaum JM , Dorsey ER , Stephenson D , Adams JL ((2023) ) Relative meaningfulness and impacts of symptoms in people with early-stage Parkinson’s disease. J Parkinsons Dis 13: , 619–632. |
[44] | Mathur S ((2021) ) Counting what counts: How to reach outcomes that truly matter to Parkinson’s patients. Mov Disord 36: , 1290–1292. |
[45] | Graeber J , Warmerdam E , Aufenberg S , Bull C , Davies K , Dixon J , Emmert K , Judd C , Maetzler C , Reilmann R , Ng WF , Macrae V , Maetzler W , Kaduszkiewicz H ((2023) ) Technology acceptance of digital devices for home use: Qualitative results of a mixed methods study. Digit Health 9: , 9. |
[46] | Buchanan SM , Richards M , Schott JM , Schrag A ((2021) ) Mild parkinsonian signs: A systematic review of clinical, imaging, and pathological associations. Mov Disord 36: , 2481–2493. |
[47] | Espay AJ , Hausdorff JM , Sánchez-Ferro Á , Klucken J , Merola A , Bonato P , Paul SS , Horak FB , Vizcarra JA , Mestre TA , Reilmann R , Nieuwboer A , Dorsey ER , Rochester L , Bloem BR , Maetzler W ((2019) ) A roadmap for implementation of patient-centered digital outcome measures in Parkinson’s disease obtained using mobile health technologies. Mov Disord 34: , 657–663. |
[48] | Hansen C , Beckbauer M , Romijnders R , Warmerdam E , Welzel J , Geritz J , Emmert K , Maetzler W ((2021) ) Reliability of imu-derived static balance parameters in neurological diseases. Int J Environ Res Public Health 18: , 3644. |
[49] | Delval A , Betrouni N , Tard C , Devos D , Dujardin K , Defebvre L , Labidi J , Moreau C ((2021) ) Do kinematic gait parameters help to discriminate between fallers and non-fallers with Parkinson’s disease? Clin Neurophysiol 132: , 536–541. |
[50] | Sidoroff V , Raccagni C , Kaindlstorfer C , Eschlboeck S , Fanciulli A , Granata R , Eskofier B , Seppi K , Poewe W , Willeit J , Kiechl S , Mahlknecht P , Stockner H , Marini K , Schorr O , Rungger G , Klucken J , Wenning G , Gaßner H ((2021) ) Characterization of gait variability in multiple system atrophy and Parkinson’s disease. J Neurol 268: , 1770–1779. |
[51] | Bologna M , Espay AJ , Fasano A , Paparella G , Hallett M , Berardelli A ((2023) ) Redefining bradykinesia. Mov Disord 38: , 551–557. |
[52] | Salis F , Bertuletti S , Bonci T , Caruso M , Scott K , Alcock L , Buckley E , Gazit E , Hansen C , Schwickert L , Aminian K , Becker C , Brown P , Carsin A-E , Caulfield B , Chiari L , D’Ascanio I , Del Din S , Eskofier BM , Garcia-Aymerich J , Hausdorff JM , Hume EC , Kirk C , Kluge F , Koch S , Kuederle A , Maetzler W , Micó-Amigo EM , Mueller A , Neatrour I , Paraschiv-Ionescu A , Palmerini L , Yarnall AJ , Rochester L , Sharrack B , Singleton D , Vereijken B , Vogiatzis I , Della Croce U , Mazzà C , Cereatti A , For The Mobilise-D Consortium ((2023) ) A multi-sensor wearable system for the assessment of diseased gait in real-world conditions. Front Bioeng Biotechnol 11: , 1143248. |
[53] | Maetzler W , Rochester L , Bhidayasiri R , Espay AJ , Sánchez-Ferro A , van Uem JMT ((2021) ) Modernizing daily function assessment in Parkinson’s disease using capacity, perception, and performance measures. Mov Disord 36: , 76–82. |
[54] | Feigin VL , Nichols E , Alam T , Bannick MS , Beghi E , Blake N , Culpepper WJ , Dorsey ER , ...Zodpey S , Naghavi M , Murray CJL , Vos T ((2019) ) Global, regional, and national burden of neurological disorders, 1990-2016: A systematic analysis for the Global Burden of Disease Study 2016. Lancet Neurol 18: , 459–480. |
[55] | Surangsrirat D , Sri-iesaranusorn P , Chaiyaroj A , Vateekul P , Bhidayasiri R ((2022) ) Parkinson’s disease severity clustering based on tapping activity on mobile device. Sci Rep 12: , 3142. |
[56] | Iakovakis D , Chaudhuri KR , Klingelhoefer L , Bostantjopoulou S , Katsarou Z , Trivedi D , Reichmann H , Hadjidimitriou S , Charisis V , Hadjileontiadis LJ ((2020) ) Screening of Parkinsonian subtle fine-motor impairment from touchscreen typing via deep learning. Sci Rep 10: , 12623. |
[57] | Leroux A , Xu S , Kundu P , Muschelli J , Smirnova E , Chatterjee N , Crainiceanu C ((2021) ) Quantifying the predictive performance of objectively measured physical activity on mortality in the UK Biobank. J Gerontol A Biol Sci Med Sci 76: , 1486–1494. |
[58] | Buchman AS , Wang T , Oveisgharan S , Zammit AR , Yu L , Li P , Hu K , Hausdorff JM , Lim ASP , Bennett DA ((2023) ) Correlates of person-specific rates of change in sensor-derived physical activity metrics of daily living in the Rush Memory and Aging Project. Sensors (Basel) 23: , 4152. |
[59] | Yuan W , Beaulieu-Jones B , Krolewski R , Palmer N , Veyrat-Follet C , Frau F , Cohen C , Bozzi S , Cogswell M , Kumar D , Coulouvrat C , Leroy B , Fischer TZ , Sardi SP , Chandross KJ , Rubin LL , Wills AM , Kohane I , Lipnick SL ((2021) ) Accelerating diagnosis of Parkinson’s disease through risk prediction. BMC Neurol 21: , 201. |
[60] | Pah ND , Motin MA , Kumar DK ((2022) ) Phonemes based detection of Parkinson’s disease for telehealth applications. Sci Rep 12: , 9687. |
[61] | Hernandez V , Dadkhah D , Babakeshizadeh V , Kulić D ((2021) ) Lower body kinematics estimation from wearable sensors for walking and running: A deep learning approach. Gait Posture 83: , 185–193. |
[62] | Hansen C , Chebil B , Cockroft J , Bianchini E , Romijnders R , Maetzler W ((2023) ) Changes in coordination and its variability with an increase in functional performance of the lower extremities. Biosensors (Basel) 13: , 156. |
[63] | Ricotti V , Kadirvelu B , Selby V , Festenstein R , Mercuri E , Voit T , Faisal AA ((2023) ) Wearable full-body motion tracking of activities of daily living predicts disease trajectory in Duchenne muscular dystrophy. Nat Med 29: , 95–103. |
[64] | Kadirvelu B , Gavriel C , Nageshwaran S , Chan JPK , Nethisinghe S , Athanasopoulos S , Ricotti V , Voit T , Giunti P , Festenstein R , Faisal AA ((2023) ) A wearable motion capture suit and machine learning predict disease progression in Friedreich’s ataxia. Nat Med 29: , 86–94. |
[65] | Antikainen E , Njoum H , Kudelka J , Branco D , Rehman RZU , Macrae V , Davies K , Hildesheim H , Emmert K , Reilmann R , Janneke van der Woude C , Maetzler W , Ng WF , O’Donnell P , Van Gassen G , Baribaud F , Pandis I , Manyakov N V. , van Gils M , Ahmaniemi T , Chatterjee M ((2022) ) Assessing fatigue and sleep in chronic diseases using physiological signals from wearables: A pilot study. Front Physiol 13: , 968185. |
[66] | Cockx H , Nonnekes J , Bloem B , van Wezel R , Cameron I , Wang Y ((2023) ) Dealing with the heterogeneous presentations of freezing of gait: How reliable are the freezing index and heart rate for freezing detection? J Neuroeng Rehabil 20: , 53. |
[67] | Huang Z , Bianchi F , Yuksekgonul M , Montine TJ , Zou J ((2023) ) A visual-language foundation model for pathology image analysis using medical Twitter. Nat Med 29: , 2307–2316. |
[68] | Hill DL , Stephenson D , Brayanov J , Claes K , Badawy R , Sardar S , Fisher K , Lee SJ , Bannon A , Roussos G , Kangarloo T , Terebaite V , Müller MLTM , Bhatnagar R , Adams JL , Dorsey ER , Cosman J ((2022) ) Metadata framework to support deployment of digital health technologies in clinical trials in Parkinson’s disease. Sensors 22: , 2136. |
[69] | Badawy R , Hameed F , Bataille L , Little MA , Claes K , Saria S , Cedarbaum JM , Stephenson D , Neville J , Maetzler W , Espay AJ , Bloem BR , Simuni T , Karlin DR ((2019) ) Metadata concepts for advancing the use of digital health technologies in clinical research. Digit Biomark 3: , 116–132. |