A Retrospective Comparison of Multiple Approaches to Anatomically Informed Contact Selection in Subthalamic Deep Brain Stimulation for Parkinson’s Disease
Abstract
Background:
Conventional deep brain stimulation (DBS) programming via trial-and-error warrants improvement to ensure swift achievement of optimal outcomes. The definition of a sweet spot for subthalamic DBS in Parkinson’s disease (PD-STN-DBS) may offer such advancement.
Objective:
This investigation examines the association of long-term motor outcomes with contact selection during monopolar review and different strategies for anatomically informed contact selection in a retrospective real-life cohort of PD-STN-DBS.
Methods:
We compared contact selection based on a monopolar review (MPR) to multiple anatomically informed contact selection strategies in a cohort of 28 PD patients with STN-DBS. We employed a commercial software package for contact selection based on visual assessment of individual anatomy following two predefined strategies and two algorithmic approaches with automatic targeting of either the sensorimotor STN or our previously published sweet spot. Similarity indices between chronic stimulation and contact selection strategies were correlated to motor outcomes at 12 months follow-up.
Results:
Lateralized motor outcomes of chronic DBS were correlated to the similarity between chronic stimulation and visual contact selection targeting the dorsal part of the posterior STN (rho = 0.36, p = 0.007). Similar relationships could not be established for MPR or any of the other investigated strategies.
Conclusions:
Our data demonstrates that a visual contact selection following a predefined strategy can be linked to beneficial long-term motor outcomes in PD-STN-DBS. Since similar correlations could not be observed for the other approaches to anatomically informed contact selection, we conclude that clear definitions and prospective validation of any approach to imaging-based DBS-programming is warranted.
INTRODUCTION
High-frequency deep brain stimulation (DBS) of the subthalamic nucleus (STN) is a well-established treatment option for advanced Parkinson’s disease (PD), improving motor and non-motor symptoms, reducing medication intake, and alleviating fluctuations in treatment response [1, 2]. While most studies have confirmed beneficial effects on motor outcomes, considerable interindividual variability is observed [3]. Spatial selectivity of the electric field, i.e., lead position and contact selection, is among the most critical factors for therapeutic success [4, 5].
Especially since the advent of directional leads, contact selection can be employed for selective current distribution to improve stimulation response [6–8]. Consequently, the practice of meticulously testing acute stimulation effects for each contact to determine optimal stimulation response, the monopolar review (MPR), has become even more tedious and time-consuming. The number of possible contact combinations presents a complex challenge to comprehensive assessments. Besides, a valuable MPR requires specialized expertise and involves subjective assessments of often fluctuating symptoms. Ambiguous results regularly necessitate repeated reprogramming sessions and extended observation periods [9–11].
Imaging-based lead localization and electric field modeling provide approximations of the directly affected brain area, i.e., the volume of tissue activation (VTA) [12]. Group analyses of stimulation effects and corresponding VTAs have been used to establish probabilistic maps identifying the optimal DBS target areas or ‘sweet spots’ [13]. Converging evidence suggests that DBS involving the posterodorsal STN facilitates the most effective suppression of motor symptoms with minimal side effects [14–17]. This region contains the STN motor region and the entry zone of relevant fiber tracts [18–22]. Furthermore, the region corresponds to the distribution of pathological beta-activity within the STN [23]. Notably, the role of the white matter adjacent to the STN is debated [24].
With a growing body of commercial and scientific software packages, VTA visualization is becoming increasingly available to DBS clinicians paving the way for broad implementation of anatomically informed DBS programming [11, 25–28]. Previous studies reported a dramatic reduction of time requirements for achieving non-inferior motor outcomes for PD-STN-DBS relative to MPR [9, 10, 26, 29]. However, the programming approaches across publications were relatively ill-defined about the dorsolateral STN and, thus, remain open to interpretation.
Anatomically informed DBS programming holds the potential for increased efficacy in terms of time consumption and individual outcomes, as well as a more objective foundation for treatment decisions. Still, there is a lack of real-life evidence, systematic evaluations of long-term outcomes, a unified approach, and evidence-based guidelines.
In this study, we retrospectively analyzed standardized assessments of our PD-STN-DBS cohort, investigating the association of long-term motor outcomes with different contact selection strategies comparing MPR, two strategies for a visual assessment of individual anatomy, and two algorithmically optimized strategies targeting the motor STN or our previously published sweet spot.
MATERIALS AND METHODS
Study cohort and long-term assessment
In our clinical database of STN-DBS-PD patients, we identified all patients who had received a standardized MPR, adequate imaging quality in preoperative MRI, and postoperative CT imaging, a preoperative Unified Parkinson’s Disease Rating Scale motor examination (UPDRS III) after overnight cessation of dopaminergic drugs (MED OFF) and after a levodopa challenge (MED ON), and a postoperative UPDRS III assessment with active DBS after medication cessation (STIM ON/MED OFF) 12 months after DBS implantation. As per clinical routine at our center, chronic stimulation parameters were initially based upon intraoperative assessments and subsequently underwent several weeks of refinement in an external rehabilitation facility. After a reevaluation based upon a standardized monopolar review at the 3-month mark, parameters were tailored to the patients’ needs during routine follow-up visits, including a mandatory assessment at 6 months. It is important to note that the DBS team conducted any adjustments without specific knowledge of each patient’s anatomical details. Sufficient data were available for 28 subjects. All assessments are a standardized part of our clinical routine.
The institutional review board of the University of Cologne waived the need for ethics approval and the need to obtain consent for the collection, analysis and publication of the retrospectively obtained and anonymized data for this non-interventional study (23-1211-retro, June 2, 2023).
Before surgery, all subjects were clinically diagnosed with PD in alignment with current MDS criteria and demonstrated a significant preoperative levodopa response while lacking severe cognitive impairment, impeding neuropsychiatric issues, significant brain atrophy, or white matter disease [30]. A local expert panel had approved eligibility for DBS surgery, and all subjects had received directional leads (Vercise Cartesia™ Directional Lead, Boston Scientific Corp., Valencia, California, USA). Descriptive clinical data of the study population are provided in Supplementary Table 1.
Motor outcomes
Motor symptoms were quantified based on the sum of lateralized items of the UPDRS part III (items 20–26). Stimulation (STIM ON/MED OFF) and levodopa (MED ON) responses were assessed as percentage change compared to the baseline MED OFF assessment. For further evaluation of individual treatment success, a response ratio (stimulation response/levodopa response) was derived analogous to Nickl et al. [5].
Contact selection based on a monopolar review
A standardized MPR was obtained per clinical routine at least three months after surgery in MED OFF. After baseline evaluation of motor symptoms (rigidity, tremor, bradykinesia) in the STIM OFF condition, each symptom was assessed during monopolar stimulation (2 mA, 60μs, 130 Hz). For directional levels, additional assessments were performed in omnidirectional configuration, resulting in ten conditions per hemisphere. Symptom severity was assessed analogous to the respective UPDRS III items (0–4 points). The contact sequence was randomized to minimize fatigue and distraction bias. Side effect thresholds were examined in MED ON to minimize exertional burden and determined by incremental increases of the stimulation amplitude (60μs, 130 Hz). The contact providing the most effective symptom suppression across symptoms was determined by comparing the overall reduction of symptom scores, defining MPR-based contact selection (MPR). In ambiguous cases, contacts with higher side effect thresholds were prioritized. If unambiguous contact selection was impossible, further analysis was omitted (n = 2 hemispheres).
Anatomically informed contact selection based on visual assessment
Patient-specific anatomy and lead positions were visualized using GUIDE™ XT (Boston Scientific Corp., USA). Sufficient image quality was visually confirmed. Preoperative isometric T1- and T2-weighted MRI (1.0 mm3 voxel size, 3T Ingenia, Achieva, 1.5T Ingenia, Philips Healthcare, The Netherlands) were coregistered with a postoperative high-resolution CT scan (IQon Spectral CT, iCT 256, Brilliance 256, Philips Healthcare, The Netherlands). Automatic STN segmentation as implemented in GUIDE™ XT was visually verified and manually refined based on T2 contrast. Automatic detection of lead positions and orientation based on CT artifacts was visually confirmed. If necessary, automatic reconstruction was corrected manually. If an algorithmic determination of lead orientation failed, orientation was estimated manually based on the prominence of the hyperdense marker signal.
After reconstruction, two users (CH and GB) with extensive experience in clinical DBS care independently performed anatomically informed contact selection based on visual assessment. While GB had significant prior experience in the clinical use of GUIDE™ XT, CH was introduced to the software during this investigation, received a brief informal training by GB, and was considered a novice user.
Both users assessed individual anatomy based on VTA visualization in GUIDE™ XT with parameters set to 2.0 mA, 60μs, and 130 Hz (analogous to MPR) to approximate the location and directionality of the respective stimulation volumes. The programming approach, in general, was informed by the sweet spot literature at large and prior experiences with the clinical implementation of GUIDE™ XT [14–16, 31]. To explore the unresolved issue of to what extent the white matter dorsal to the STN should purposefully be targeted, two different approaches were defined and are detailed below.
Omnidirectional settings were selected if a lead was positioned close to the visual center of the posterior third of the STN. Otherwise, the maximal directional focus was applied and faced toward the posterior third of the STN. Contact levels close to the dorsal border of the STN were selected. With the first approach, the dorsal border of the VTA was aligned with the dorsal border of the STN, focusing on the dorsal motor STN (VISUAL-A), while with the second approach, approximately 10–25% of the simulated VTA was placed dorsal to the STN emulating the distribution of our previously published sweet spot (VISUAL-B) [15]. If a ring contact was the closest to the dorsal border of the STN with a lead positioned off-center to the posterior third of the STN, directional contacts on the neighboring level could be used to facilitate directional stimulation at the user’s discretion.
Representative screenshots of the user interface (and an exemplary application of VISUAL-B) are provided in Supplementary Figure 1.
Anatomically informed contact selection based on algorithmic optimization
Based on reconstructions of individual anatomy and VTA modeling in the Lead-DBS toolbox, which are described in detail below, algorithmic computation (MATLAB function fmincon) was used to determine the (multi-) contact selection with optimized targeting of our probabilistic sweet spot (ALGO-sweetspot) by maximizing the sum of probability weighted sweet spot voxels within a VTA at 2 mA, 60μs, 130 Hz [15, 32]. Analogous computations were performed to determine the (multi-) contact selections with maximum overlap with the motor STN as defined by the DISTAL atlas (ALGO-mSTN) [18].
Comparison of chronic stimulation settings and contact selection strategies
Since GUIDE™ XT does not allow for quantitative and comparative analyses of stimulation fields, the Lead-DBS toolbox was used to further analyze the previously determined contact selections [27]. Visual quality control was performed for each processing step. Preoperative MRI were coregistered using statistical parametrical mapping (SPM, version 12), and postoperative CT images were coregistered using a two-stage linear registration (rigid followed by affine) as implemented in Advanced Normalization Tools (ANTs) [33, 34]. If the methods mentioned above resulted in suboptimal results, linear transformation as implemented in FSL (FLIRT, FMRIB Software Library, version 6.0) was used [35, 36]. Normalization into the Montreal Neurological Institute space (MNI 2009b, nonlinear, asymmetric) was performed by a symmetric diffeomorphic registration approach (SyN-ANTS). In the case of suboptimal results, a non-linear transformation approach utilizing tissue segmentation implemented in SPM12 (SEGMENT) was employed [34, 37, 38]. DBS electrode localizations were corrected for a brain shift by the Lead-DBS brain shift correction module with the “coarse mask” setting. Lead trajectories were identified with the PaCER algorithm. Lead rotation was determined using DiODe [39–41].
VTA calculations were performed in patient space in isometric 0.2 mm resolution. A precomputed electrical field model integrated into the Lead-DBS toolbox (FastField [42]) was used to calculate binary VTAs (thresholded at 0.2 V/mm) for the chronic stimulation settings. For comparison, representative VTAs were calculated for all contact selections with identical parameters (2 mA, 60μs, 130 Hz). Atlas segmentation of the motor STN (DISTAL [18]) and our previously published sweet spot for PD-STN-DBS [15], which are integrated into the Lead-DBS toolbox, were transformed from MNI space into patient space using nonlinear normalization matrices obtained during Lead-DBS processing.
The similarity between VTAs was quantified via the Dice coefficient and calculated as the ratio of the twofold overlap volume to the sum of the respective VTA volumes [43]. Motor STN or sweet spot coverages were quantified as percentage overlap of VTAs with the binarized target volume. We then investigated the relationship between the motor outcome of chronic VTAs and their similarity with VTAs from the different contact selection strategies using correlation analyses (Fig. 1).
Fig. 1
Schematic overview of the applied methodology. Lateralized long-term motor outcomes were correlated with various contact selection strategies via quantitative assessment of similarity between chronic stimulation settings and the contact selection based on each strategy.
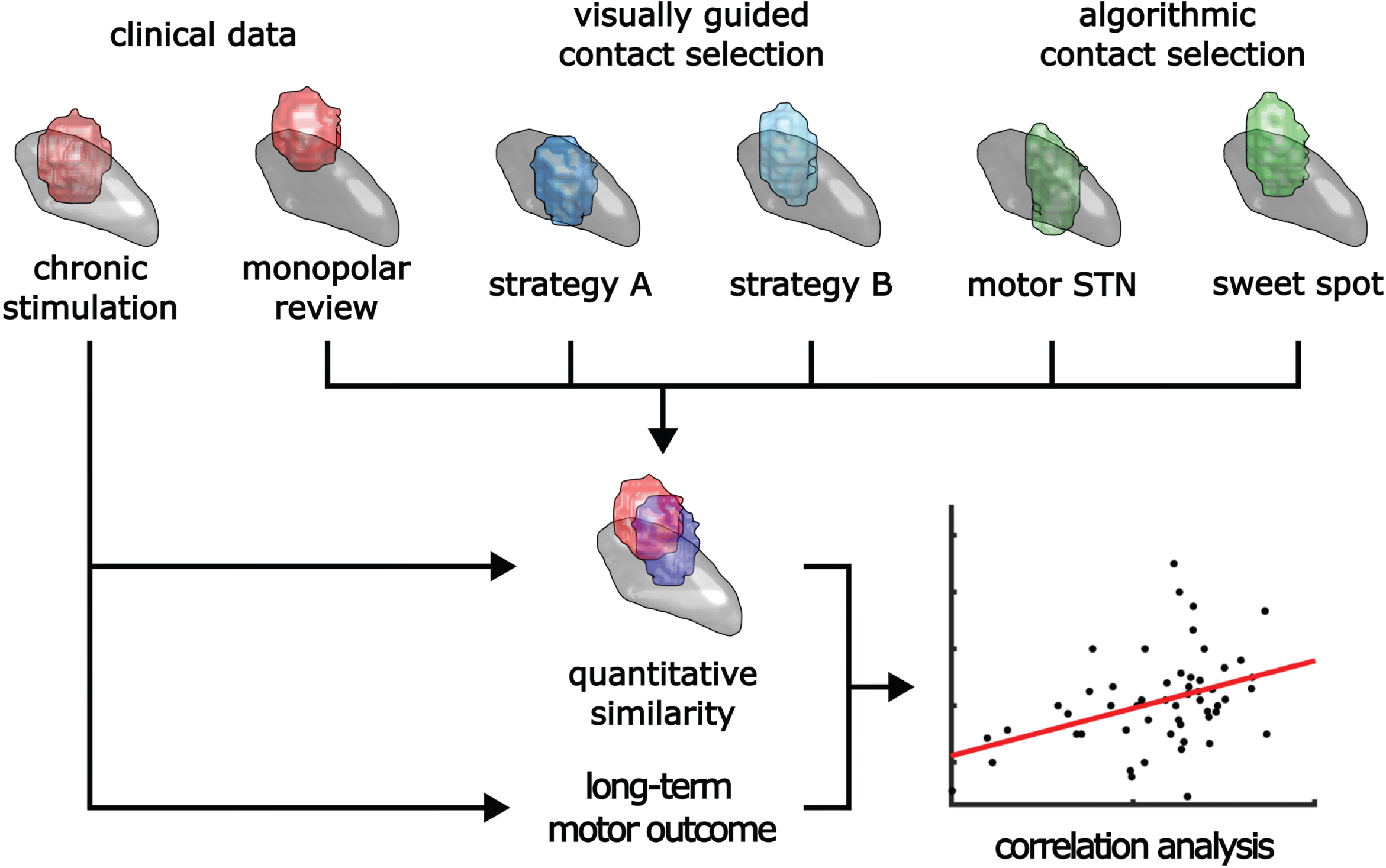
Neuroanatomical context
To evaluate neuroanatomical context, voxel-based n-maps for chronic stimulation and each contact selection strategy were calculated in isometric 0.2 mm resolution after transfer of individual VTAs to the right hemisphere of the MNI template space using nonlinear normalization matrices obtained during Lead-DBS processing. After summation of the respective volumes, each voxel indicated how many of the respective VTA included the respective voxel. A core region was defined at a threshold of n > 50% of all VTAs.
Statistical analysis
All results are reported as mean±standard deviation. Data were tested for normality with the Shapiro-Wilk test. Whenever necessary, nonparametric statistics were applied. p-values less than 0.05 were considered statistically significant. p-values corrected for multiple testing were calculated according to the Holm-Bonferroni method and are indicated as pcorr. All computations and statistical analyses were performed using MATLAB (version R2022b, The MathWorks Inc., USA).
RESULTS
Motor outcome
A full analysis could be completed for 54 hemispheres. Presurgical levodopa response (MED OFF vs. MED ON) demonstrated a UPDRS III hemiscore reduction of 6.0±3.0 points. The DBS response reduced the UPDRS III hemiscore by 5.9±4.9 points at the 12-months follow-up (Fig. 3). Even though DBS responses matched the presurgical levodopa effect on average, we observed considerable interindividual variability (Supplementary Table 2).
Anatomically informed contact selection based on visual assessment
The mean processing time of the expert user to prepare the GUIDE™ XT session and determine a first visual contact selection for each subject was 13.6±3.3 min. Subsequent assessments for contact selection (without redundant preprocessing) were performed in 2.16±0.59 min by the expert user and 2.07±0.29 min by the novice user. Comparing VTAs among users demonstrated high interrater reliability for both visual strategies (median Dice coefficient of 0.90 (IQR 0.86 –0.95)).
In our further analysis, we thoroughly examined the assessments of both users individually and found no significant discrepancies in the aspects we investigated. For clarity, we present our findings utilizing the expert assessments unless explicitly stated otherwise.
Comparison of contact selections to chronic stimulation settings
Dice coefficients between chronic stimulation and contact selections did not differ among contact selection strategies (ANOVA, F(4,265) = 2.05, p = 0.09, Fig. 2A). While the individual difference between MPR and the other contact selection strategies in relation to chronic stimulation was relatively small on average (mean Δ Dice coefficient = 0.07±0.27) and did not differ among groups (ANOVA, F(3,212) = 0.57, p = 0.63) a wide range of different similarities was found (Fig. 2B).
Fig. 2
Comparative analysis of contact selection strategies in relation to chronic stimulation settings, motor STN and the utilized sweet spot. A) Comparative analysis did not reveal any trend of preferential similarity between the volume of tissue activation (VTA) of chronic stimulation and VTAs of respective contact selection methods. Dice coefficients (DC) of VTAs of chronic stimulation and either monopolar review-based contact selection (MPR), visual contact selection within the dorsal motor STN (VISUAL-A), visual contact selection including the adjacent white matter (VISUAL-B), or automated contact selection with optimization of the coverage of either the motor STN (ALGO-mSTN) or the sweet spot (ALGO-sweetspot) showed similar distributions among contact selection strategies. B) Comparison of DCs of the VTA of chronic stimulation and MPR to the DCs of VISUAL-A and the chronic stimulation settings on the individual level demonstrates a wide range of different similarities. C) The percentage coverage of the motor STN was significantly higher with VTAs of contact selection strategies focusing on the motor STN (VISUAL-A/ALGO-mSTN), than with the strategies informed by the utilized sweet spot (VISUAL-B/ALGO-sweetspot) [15]. ALGO-mSTN also showed significantly higher values than contact selections based on monopolar reviews (MPR). D) Among automated contact selections ALGO-sweetspot percentage sweet spot coverage was significantly higher, while VISUAL-A and VISUAL-B did not differ significantly. With MPR, sweet spot coverage was lower than VISUAL-A, VISUAL-B, and ALGO-sweetspot. We did not find a significant difference between visual and automatic approaches for both target structures. (ANOVA, post-hoc Tukey’s test, *p < 0.05, **p < 0.01, ***p < 0.001).
![Comparative analysis of contact selection strategies in relation to chronic stimulation settings, motor STN and the utilized sweet spot. A) Comparative analysis did not reveal any trend of preferential similarity between the volume of tissue activation (VTA) of chronic stimulation and VTAs of respective contact selection methods. Dice coefficients (DC) of VTAs of chronic stimulation and either monopolar review-based contact selection (MPR), visual contact selection within the dorsal motor STN (VISUAL-A), visual contact selection including the adjacent white matter (VISUAL-B), or automated contact selection with optimization of the coverage of either the motor STN (ALGO-mSTN) or the sweet spot (ALGO-sweetspot) showed similar distributions among contact selection strategies. B) Comparison of DCs of the VTA of chronic stimulation and MPR to the DCs of VISUAL-A and the chronic stimulation settings on the individual level demonstrates a wide range of different similarities. C) The percentage coverage of the motor STN was significantly higher with VTAs of contact selection strategies focusing on the motor STN (VISUAL-A/ALGO-mSTN), than with the strategies informed by the utilized sweet spot (VISUAL-B/ALGO-sweetspot) [15]. ALGO-mSTN also showed significantly higher values than contact selections based on monopolar reviews (MPR). D) Among automated contact selections ALGO-sweetspot percentage sweet spot coverage was significantly higher, while VISUAL-A and VISUAL-B did not differ significantly. With MPR, sweet spot coverage was lower than VISUAL-A, VISUAL-B, and ALGO-sweetspot. We did not find a significant difference between visual and automatic approaches for both target structures. (ANOVA, post-hoc Tukey’s test, *p < 0.05, **p < 0.01, ***p < 0.001).](https://ip.ios.semcs.net:443/media/jpd/2024/14-3/jpd-14-3-jpd230200/jpd-14-jpd230200-g002.jpg)
Correlation between similarity indices and motor outcomes
Long-term motor outcomes were significantly correlated to the similarity of chronic stimulation and VISUAL-A with higher similarity indices being associated with greater motor improvement (Δ UPDRS III hemiscore [%]: Spearman’s rho = 0.36, p = 0.007, pcorr = 0.04; response ratio (STIM/LD): Spearman’s rho = 0.38, p = 0.005, pcorr = 0.02, Fig. 3, Supplementary Figure 2). In other words, long-term UPDRS III improvement was greater, when chronic stimulation settings matched VISUAL-A settings better. We did not observe a significant correlation for VISUAL-B and algorithmic contact selection (ALGO-mSTN/ALGO-sweetspot) (Fig. 3). Importantly, we also did not observe any significant association of motor outcome to MPR (Δ UPDRS III hemiscore [%]: Spearman’s rho = 0.19, p = 0.18, Fig. 3; response ratio (STIM/LD): Spearman’s rho = 0.02, p = 0.87, Supplementary Figure 2). Symptom-specific subanalysis for akinetic-rigid and tremor subscores did not reveal any significant correlations (Supplementary Figure 3).
Fig. 3
Lateralized motor outcomes in correlation to contact selection strategies. A) Compared to preoperative baseline (MED OFF), UPDRS III hemiscore significantly improved in MED OFF/STIM ON 12 months after surgery. ***p< 0.001 (paired t-test). B-F) Lateralized motor outcomes were significantly correlated to the dice coefficients (DC) between chronic stimulation and visual contact selection focussed on the dorsal motor STN (VISUAL-A). DCs of the contact selection based on the monopolar review (MPR), an alternative visual contact selection strategy involving the dorsal adjacent white matter (VISUAL-B), algorithmically optimized coverage of the motor STN (ALGO-mSTN), or the sweet spot (ALGO-sweetspot) were not correlated to changes in UPDRS III hemiscore [15]. In the case of statistical significance, a least-squares regression line (red) was added as a visual reference.
![Lateralized motor outcomes in correlation to contact selection strategies. A) Compared to preoperative baseline (MED OFF), UPDRS III hemiscore significantly improved in MED OFF/STIM ON 12 months after surgery. ***p< 0.001 (paired t-test). B-F) Lateralized motor outcomes were significantly correlated to the dice coefficients (DC) between chronic stimulation and visual contact selection focussed on the dorsal motor STN (VISUAL-A). DCs of the contact selection based on the monopolar review (MPR), an alternative visual contact selection strategy involving the dorsal adjacent white matter (VISUAL-B), algorithmically optimized coverage of the motor STN (ALGO-mSTN), or the sweet spot (ALGO-sweetspot) were not correlated to changes in UPDRS III hemiscore [15]. In the case of statistical significance, a least-squares regression line (red) was added as a visual reference.](https://ip.ios.semcs.net:443/media/jpd/2024/14-3/jpd-14-3-jpd230200/jpd-14-jpd230200-g003.jpg)
Neuroanatomical context
Comparison of motor STN coverage among strategies did reveal a significant group effect (ANOVA, F(4,265) = 8.82, p < 0.001, Fig. 2C). Tukey’s post-hoc test showed VISUAL-A and ALGO-mSTN covered significantly higher percentages of the motor STN than VISUAL-B and ALGO-sweetspot. ALGO-mSTN also showed significantly higher values than MPR.
Similarly, sweet spot coverage differed among contact selection strategies (ANOVA, F(4,265) = 21.86, p < 0.001, Fig. 2D). Tukey’s post hoc test showed significantly higher values for ALGO-sweetspot, VISUAL-A and VISUAL-B than ALGO-mSTN and MPR. We did not find any significant differences among ALGO-mSTN and MPR or ALGO-sweetspot, VISUAL-A, and VISUAL-B.
Voxel-based n-maps of contact selection strategies in common space revealed core regions (n > 50%) at the border of the motor and associative region of the STN (Fig. 4). Chronic and MPR VTAs were distributed over a larger area with relatively small core regions, while visual and algorithmic contact selection strategies resulted in more uniform and focused VTA distributions (Fig. 4, Supplementary Figure 4). VISUAL-A, VISUAL-B, and ALGO-sweetspot core regions were located at the dorsal border of the STN. Among these VISUAL-B demonstrated the most pronounced extranuclear involvement, while VISUAL-A showed the greatest involvement of the motor STN (Fig. 4C, D, F). The involvement of the motor STN was maximal for ALGO-mSTN, resulting in the most ventral core region with a minimal extranuclear compartment (Fig. 4E).
Fig. 4
Voxel-based n-maps for chronic stimulation and each contact selection strategy. Anterior (1) and lateral (2) view of the right STN in common space (L: lateral, M: medial, P: posterior, A: anterior). Green volumes indicate a core region that is part of the volume of tissue activation (VTA) in ≥50% of hemispheres in chronic stimulation (A) or the calculated VTAs of different contact selections (B–F). Grey volumes indicate all voxels that are part of the VTA for at least 1 hemisphere. Pie charts (3) display the proportions of the core regions that are either part of the motor (red), associative (blue), or limbic (yellow) partition of the STN or extend into the extranuclear compartment (white).
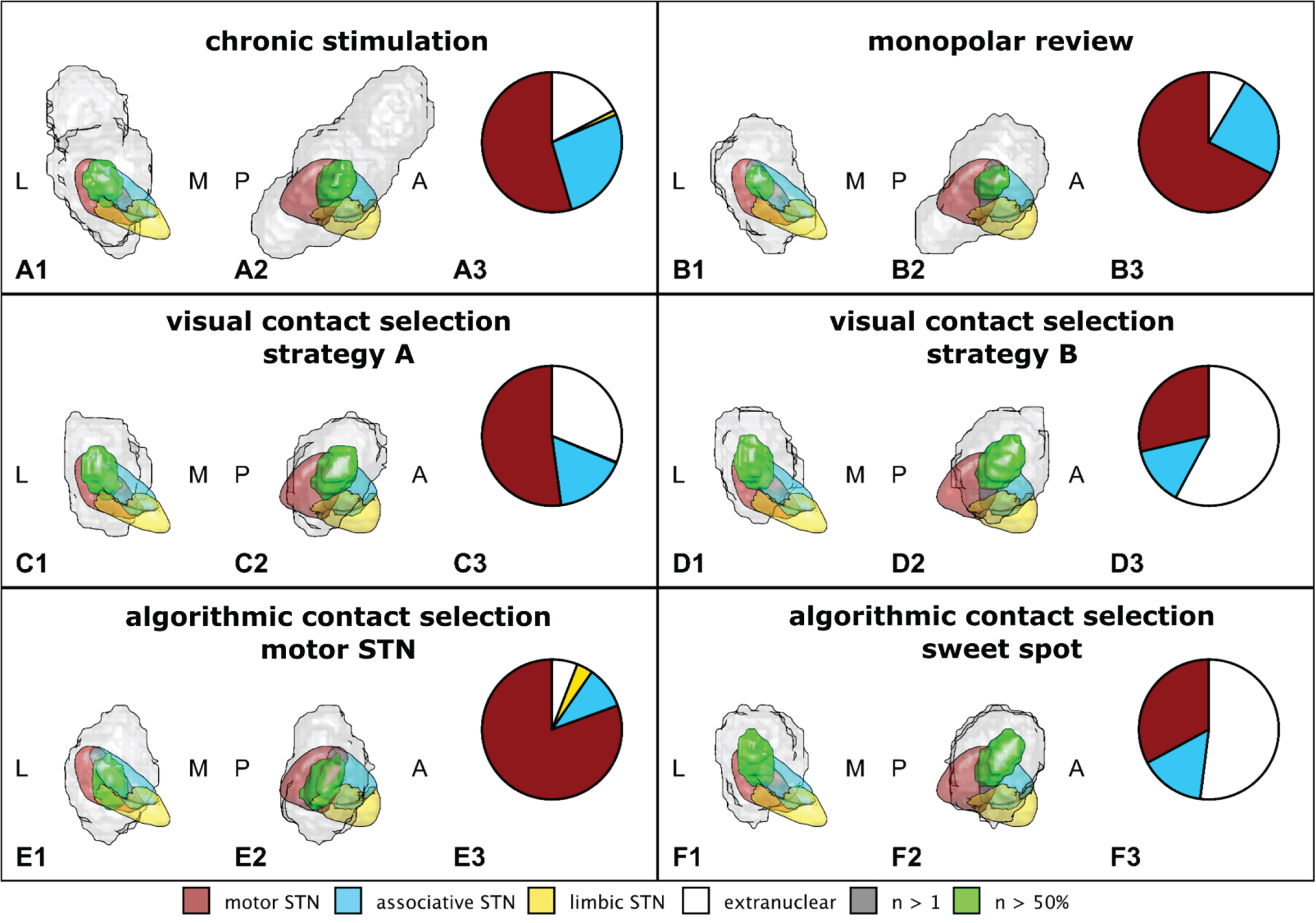
DISCUSSION
This retrospective analysis demonstrates that anatomically informed contact selections based on visual assessment following a predefined approach targeting the dorsal motor STN can be linked to beneficial long-term motor outcomes after STN-DBS in PD. Put differently: In cases with better outcome, clinical contact selection was more in line with VISUAL-A selections. Similar relationships could not be established for three other approaches to anatomically informed contact selection and for conventional contact selections based on monopolar review. These findings implicate a significant impact of the specific method used in applying anatomical information to DBS treatment for individual patients.
Given the varying definitions of the sweet spot across the literature, we defined two strategies for visual contact selection that differed in terms of how much of the dorsal white matter should be involved [24]. As only the strategy aligning the superior aspect of the VTA with the dorsal border of the STN in subject space was associated with a beneficial motor outcome, we conclude that minuscule differences in the strategy can be critical to the success of anatomically informed contact selection. This becomes especially relevant if technical advancements such as new imaging protocols (FGATIR, QSM, etc.) or techniques like diffusion-based tractography are implemented and might change our definition of relevant anatomical landmarks [44, 45].
Notably, algorithmically optimized targeting of neither the motor STN nor a probabilistic sweet spot correlated with beneficial motor outcomes. While the sweet spot literature already suggested that the entire motor STN does not suffice as a target definition, the missing link between the sweet spot and motor outcome was unexpected [14, 15, 28]. We agree with Jaradat et al., who hypothesized that non-linear transformation from MNI to subject space might introduce detrimental inaccuracies, diminishing the predictive value of targets defined in a common space on a single subject level [46]. Correspondingly, we found that even though the dorsal border of the STN limited our VISUAL-A approach based on individual T2-contrast in subject space, the core region of the n-map in MNI space widely surpassed the dorsal border of the atlas STN with about 30% located in the extranuclear compartment. Previous studies comparing the results of monopolar reviews and visual contact selection already reported higher levels of agreement for a visual approach compared to automatic sweet spot targeting, which might implicate a corrective impact of human interpretation during visual assessment [46, 47]. We conclude that further development beyond the applied methodology is necessary to translate insights from probabilistic sweet spots to the individual patient.
Remarkably, long-term motor outcomes were not linked to MPR, the conventional approach to DBS contact selection. Waldthaler et al. reported superior symptom control for MPR compared to visual contact selection in the acute setting for 92% of cases [9]. However, long-term stimulation settings in our cohort often differed remarkably from MPR results, indicating suboptimal initial DBS response led to reprogramming efforts. Similarly, Jaradat et al. reported that agreement between the best level during MPR and the utilized level after 12 months was as low as 58% for their patients. While expertly executed MPR under controlled conditions has been considered the gold standard and utilized as a benchmark for optimal DBS programming, our results indicate that MPR might hold limited predictive value for long-term outcomes in real-life conditions [44, 45].
Putting contact selection strategies into a neuroanatomical context, we observed considerably less consistency among MPR VTAs. VISUAL-A VTAs resulted in similar coverage of the motor STN compared to ALGO-mSTN, which was optimized in that regard, but converged more dorsally around the dorsolateral border of the STN (Fig. 4C, E). Combined with the significant correlation of VISUAL-A to beneficial motor outcomes, this finding provides further evidence that the sweet spot for PD-STN-DBS supersedes the proper motor STN.
ALGO-sweetspot and VISUAL-B converged even more dorsally than VISUAL-A, resulting in significantly less involvement of the motor STN. As only VISUAL-A was linked to beneficial motor outcomes, we assume that the pronounced extranuclear involvement of VISUAL-B and ALGO-sweetspot might have exceeded beneficial margins and resulted in inadequate, i.e., suboptimal targeting of the proper STN.
In line with previous publications, we also conclude patient-specific visualization allows for quick assessment of local anatomy enabling visual contact selection with a high inter-user reliability [9, 10, 26].
Limitations
As MPR was performed at constant amplitudes, we decided to forego estimation of stimulation amplitude with GUIDE™ XT to enable direct comparison among contact selection strategies. Even though this decision limits the scope of our investigation, we omitted extrapolation of stimulation amplitudes based on VTA following the arguments of Lange et al. [10]. As a binarized model, VTA visualization oversimplifies the complex reality of DBS. Local voltage gradients are expected to be continuous and heterogeneous affecting neuron populations differently depending on myelinization and fiber orientation [48, 49]. Correspondingly, Pavese et al. reported frequent subsequent adjustments of amplitude suggestions based on VTA modeling, and Nordenström et al. reported that clinical effect thresholds did not correlate well with predictions based on VTA modelling [46, 47]. While probabilistic sweet spot mapping allows for the deduction of the desired position of the electric field, it does not provide sufficient information regarding the optimal extent of the electrical field, respectively, the necessary current in individual patients.
Finally, the results of a retrospective analysis of single-center data without targeted intervention must be interpreted carefully and only allow for limited conclusions. None of the anatomically informed contact selections were directly applied to our patients. However, our findings correspond well with the observations of Lee et al., who already reported significant improvement in motor symptoms and further reduction of levodopa intake in a large cohort of clinically established omnidirectional PD-STN-DBS by anatomically informed reprogramming using far less sophisticated methods of visualization and a less precise target definition [50]. Additional investigations are warranted to clarify the optimal approach to establish definite recommendations for clinical practice.
Conclusion and perspective
There is an urgent need to validate DBS programming approaches that curtail the lengthy and often ambiguous process of repeated clinical evaluation and reprogramming. Our data provides further evidence that visual DBS programming can be considered a feasible approach to PD-STN-DBS, which holds the potential to further improve clinical care beyond currently established practice. However, as our results clearly demonstrate, possible success depends on a clearly defined strategy. Likewise, ill-defined strategies will lead to suboptimal results. Thus, all visual DBS programming strategies, as well as emerging computational approaches, need to be validated before implementation [51].
Looking ahead, our study findings suggest that for initial programming, a validated approach to visual DBS programming might become more effective than a conventional monopolar review in future clinical applications. Moreover, the results imply that image-guided reprogramming may benefit patients with chronic DBS settings that deviate significantly from visually implied contact selections. We intend to evaluate these hypotheses through future prospective studies.
AUTHOR CONTRIBUTIONS
Brandt GA: Conceptualization, methodology, software, data curation, formal analysis, visualization, writing – original draft. Stopic V: Investigation, resources, data curation, writing – review & editing. Hennen C: Investigation, writing – review & editing. Strelow JN: Investigation, writing – review & editing. Petry-Schmelzer JN: Investigation, methodology, writing – review & editing. Baldermann JC: writing – review & editing. Visser-Vandewalle V: Resources, writing – review & editing. Fink GR: Resources, writing – review & editing. Barbe MT: Conceptualization, resources, supervision, writing – review & editing. Dembek TA: Conceptualization, methodology, software, formal analysis, supervision, writing – review & editing.
ACKNOWLEDGMENTS
We acknowledge support for the article processing charge from the DFG (German Research Foundation 491454339).
FUNDING
The authors have no funding to report.
CONFLICT OF INTEREST
Brandt GA: GAB does not declare any conflict of interest. Stopic V: VS was funded by the Advanced Cologne Clinician Scientist program of the Medical Faculty of the University of Cologne, unrelated to this project. Hennen C: CH was supported by the German Research Foundation (DFG, Project Number 502436811), unrelated to this project. Strelow JN: JNS has received funding for an investigator-initiated trial (IIT; Reference number: ERP-2021-12740) and received speaker honoraria from Medtronic GmbH. Petry-Schmelzer JN: JNPS was supported by the Cologne Clinician Scientist Program (CCSP)/Faculty of Medicine/University of Cologne, funded by the German Research Foundation (DFG, FI 773/15-1) unrelated to this project. Baldermann JC: JCB was funded by the Else Kröner-Fresenius-Stiftung (grant number 2022_EKES.23) and receives funding from the German Research Foundation (CRC-1451, Project 431549029-C07). Visser-Vandewalle V: VVV received financial support for contributions to congresses and advisory boards from Boston Scientific, Medtronic and LivaNova. Fink GR: GRF serves as an editorial board member of Cortex, Neurological Research and Practice, NeuroImage: Clinical, Zeitschrift für Neuropsychologie, DGNeurologie, and Info Neurologie & Psychiatrie; receives royalties from the publication of the books Funktionelle MRT in Psychiatrie und Neurologie, Neurologische Differentialdiagnose, and SOP Neurologie; receives royalties from the publication of the neuropsychological tests KAS and Köpps; received honoraria for speaking engagements from Deutsche Gesellschaft für Neurologie (DGN) and Forum für medizinische Fortbildung FomF GmbH. Barbe MT: MTB received speaker’s honoraria from Medtronic, Boston Scientific, Abbott (formerly St Jude), GE Medical, UCB, Apothekerverband Köln eV, and Bial; research funding from the Felgenhauer-Stiftung, Forschungspool Klinische Studien (University of Cologne), Horizon 2020 (Gondola), Medtronic (ODIS), and Boston Scientific; and advisory honoraria for the Institut für Qualitaet und Wirtschaftlichkeit im Gesundheitswesen. Dembek TA: TAD’s work was supported by the Cologne Clinician Scientist Program (CCSP)/Faculty of Medicine/University of Cologne, funded by the German Research Foundation (DFG, FI 773/15-1). He additionally received speaker honoraria from Boston Scientific and Medtronic unrelated to this work.
DATA AVAILABILITY
The data presented in this study are available upon request from the corresponding author. The data are not publicly available for privacy reasons.
SUPPLEMENTARY MATERIAL
[1] The supplementary material is available in the electronic version of this article: https://dx.doi.org/10.3233/JPD-230200.
REFERENCES
[1] | Krack P , Batir A , Van Blercom N , Chabardes S , Fraix V , Ardouin C , Koudsie A , Limousin PD , Benazzouz A , LeBas JF , Benabid A-L , Pollak P ((2003) ) Five-year follow-up of bilateral stimulation of the subthalamic nucleus in advanced Parkinson’s disease. N Engl J Med 349: , 1925–1934. |
[2] | Schuepbach WMM , Rau J , Knudsen K , Volkmann J , Krack P , Timmermann L , Hälbig TD , Hesekamp H , Navarro SM , Meier N , Falk D , Mehdorn M , Paschen S , Maarouf M , Barbe MT , Fink GR , Kupsch A , Gruber D , Schneider G-H , Seigneuret E , Kistner A , Chaynes P , Ory-Magne F , Brefel Courbon C , Vesper J , Schnitzler A , Wojtecki L , Houeto J-L , Bataille B , Maltête D , Damier P , Raoul S , Sixel-Doering F , Hellwig D , Gharabaghi A , Krüger R , Pinsker MO , Amtage F , Régis J-M , Witjas T , Thobois S , Mertens P , Kloss M , Hartmann A , Oertel WH , Post B , Speelman H , Agid Y , Schade-Brittinger C , Deuschl G , EARLYSTIM Study Group ((2013) ) Neurostimulation for Parkinson’s disease with early motor complications. N Engl J Med 368: , 610–622. |
[3] | Kleiner-Fisman G , Herzog J , Fisman DN , Tamma F , Lyons KE , Pahwa R , Lang AE , Deuschl G ((2006) ) Subthalamic nucleus deep brain stimulation: Summary and meta-analysis of outcomes.. Mov Disord 21: , S290-S304. |
[4] | Bari AA , Fasano A , Munhoz RP , Lozano AM ((2015) ) Improving outcomes of subthalamic nucleus deep brain stimulation in Parkinson’s disease. Expert Rev Neurother 15: , 1151–1160. |
[5] | Nickl RC , Reich MM , Pozzi NG , Fricke P , Lange F , Roothans J , Volkmann J , Matthies C ((2019) ) Rescuing suboptimal outcomes of subthalamic deep brain stimulation in parkinson disease by surgical lead revision.. Neurosurgery 85: , E314–E321. |
[6] | Steigerwald F , Müller L , Johannes S , Matthies C , Volkmann J ((2016) ) Directional deep brain stimulation of the subthalamic nucleus: A pilot study using a novel neurostimulation device. Mov Disord 31: , 1240–1243. |
[7] | Dembek TA , Reker P , Visser-Vandewalle V , Wirths J , Treuer H , Klehr M , Roediger J , Dafsari HS , Barbe MT , Timmermann L ((2017) ) Directional DBS increases side-effect thresholds-A prospective, double-blind trial. Mov Disord 32: , 1380–1388. |
[8] | Alonso-Frech F , Fernandez-Garcia C , Gómez-Mayordomo V , Monje MHG , Delgado-Suarez C , Villanueva-Iza C , Catalan-Alonso MJ ((2021) ) Non-motor adverse effects avoided by directional stimulation in Parkinson’s disease: A case report. Front Neurol 12: , 786166. |
[9] | Waldthaler J , Bopp M , Kühn N , Bacara B , Keuler M , Gjorgjevski M , Carl B , Timmermann L , Nimsky C , Pedrosa DJ ((2021) ) Imaging-based programming of subthalamic nucleus deep brain stimulation in Parkinson’s disease. Brain Stimulat 14: , 1109–1117. |
[10] | Lange F , Steigerwald F , Malzacher T , Brandt GA , Odorfer TM , Roothans J , Reich MM , Fricke P , Volkmann J , Matthies C , Capetian PD ((2021) ) Reduced programming time and strong symptom control even in chronic course through imaging-based DBS programming. Front Neurol 12: , 785529. |
[11] | Picillo M , Lozano AM , Kou N , Puppi Munhoz R , Fasano A ((2016) ) Programming deep brain stimulation for Parkinson’s disease: The Toronto Western Hospital Algorithms. Brain Stimulat 9: , 425–437. |
[12] | Chaturvedi A , Butson CR , Lempka SF , Cooper SE , McIntyre CC ((2010) ) Patient-specific models of deep brain stimulation: Influence of field model complexity on neural activation predictions. Brain Stimulat 3: , 65–77. |
[13] | Dembek TA , Baldermann JC , Petry-Schmelzer J-N , Jergas H , Treuer H , Visser-Vandewalle V , Dafsari HS , Barbe MT ((2022) ) Sweetspot mapping in deep brain stimulation: Strengths and limitations of current approaches. Neuromodulation 25: , 877–887. |
[14] | Akram H , Sotiropoulos SN , Jbabdi S , Georgiev D , Mahlknecht P , Hyam J , Foltynie T , Limousin P , De Vita E , Jahanshahi M , Hariz M , Ashburner J , Behrens T , Zrinzo L ((2017) ) Subthalamic deep brain stimulation sweet spots and hyperdirect cortical connectivity in Parkinson’s disease. NeuroImage 158: , 332–345. |
[15] | Dembek TA , Roediger J , Horn A , Reker P , Oehrn C , Dafsari HS , Li N , Kühn AA , Fink GR , Visser-Vandewalle V , Barbe MT , Timmermann L ((2019) ) Probabilistic sweet spots predict motor outcome for deep brain stimulation in Parkinson disease. Ann Neurol 86: , 527–538. |
[16] | Butson CR , Cooper SE , Henderson JM , Wolgamuth B , McIntyre CC ((2011) ) Probabilistic analysis of activation volumes generated during deep brain stimulation. Neuroimage 54: , 2096–2104. |
[17] | Hamel W , Köppen JA , Alesch F , Antonini A , Barcia JA , Bergman H , Chabardes S , Contarino MF , Cornu P , Demmel W , Deuschl G , Fasano A , Kühn AA , Limousin P , McIntyre CC , Mehdorn HM , Pilleri M , Pollak P , Rodríguez-Oroz MC , Rumià J , Samuel M , Timmermann L , Valldeoriola F , Vesper J , Visser-Vandewalle V , Volkmann J , Lozano AM ((2017) ) Targeting of the subthalamic nucleus for deep brain stimulation: A survey among Parkinson disease specialists. World Neurosurg 99: , 41–46. |
[18] | Ewert S , Plettig P , Li N , Chakravarty MM , Collins DL , Herrington TM , Kühn AA , Horn A ((2018) ) Toward defining deep brain stimulation targets in MNI space: A subcortical atlas based on multimodal MRI, histology and structural connectivity. Neuroimage 170: , 271–282. |
[19] | Haynes WIA , Haber SN ((2013) ) The organization of prefrontal-subthalamic inputs in primates provides an anatomical substrate for both functional specificity and integration: Implications for Basal Ganglia models and deep brain stimulation. J Neurosci 33: , 4804–4814. |
[20] | Lambert C , Zrinzo L , Nagy Z , Lutti A , Hariz M , Foltynie T , Draganski B , Ashburner J , Frackowiak R ((2012) ) Confirmation of functional zones within the human subthalamic nucleus: Patterns of connectivity and sub-parcellation using diffusion weighted imaging. NeuroImage 60: , 83–94. |
[21] | Avecillas-Chasin JM , Alonso-Frech F , Nombela C , Villanueva C , Barcia JA ((2019) ) Stimulation of the tractography-defined subthalamic nucleus regions correlates with clinical outcomes. Neurosurgery 85: , E294–E303. |
[22] | Petersen MV , Lund TE , Sunde N , Frandsen J , Rosendal F , Juul N , Østergaard K ((2017) ) Probabilistic versus deterministic tractography for delineation of the cortico-subthalamic hyperdirect pathway in patients with Parkinson disease selected for deep brain stimulation. J Neurosurg 126: , 1657–1668. |
[23] | Horn A , Neumann W-J , Degen K , Schneider G-H , Kühn AA ((2017) ) Toward an electrophysiological “sweet spot” for deep brain stimulation in the subthalamic nucleus. Hum Brain Mapp 38: , 3377–3390. |
[24] | de Roquemaurel A , Wirth T , Vijiaratnam N , Ferreira F , Zrinzo L , Akram H , Foltynie T , Limousin P ((2021) ) Stimulation sweet spot in subthalamic deep brain stimulation –myth or reality? A critical review of literature. Stereotact Funct Neurosurg 99: , 425–442. |
[25] | Pourfar MH , Mogilner AY , Farris S , Giroux M , Gillego M , Zhao Y , Blum D , Bokil H , Pierre MC ((2015) ) Model-based deep brain stimulation programming for Parkinson’s disease: The GUIDE Pilot Study. Stereotact Funct Neurosurg 93: , 231–239. |
[26] | Pavese N , Tai YF , Yousif N , Nandi D , Bain PG ((2020) ) Traditional trial and error versus neuroanatomic 3-dimensional image software-assisted deep brain stimulation programming in patients with Parkinson disease. World Neurosurg 134: , e98–e102. |
[27] | Horn A , Li N , Dembek TA , Kappel A , Boulay C , Ewert S , Tietze A , Husch A , Perera T , Neumann W-J , Reisert M , Si H , Oostenveld R , Rorden C , Yeh F-C , Fang Q , Herrington TM , Vorwerk J , Kühn AA ((2019) ) Lead-DBS v2: Towards a comprehensive pipeline for deep brain stimulation imaging. Neuroimage 184: , 293–316. |
[28] | Nguyen TAK , Nowacki A , Debove I , Petermann K , Tinkhauser G , Wiest R , Schüpbach M , Krack P , Pollo C ((2019) ) Directional stimulation of subthalamic nucleus sweet spot predicts clinical efficacy: Proof of concept. Brain Stimulat 12: , 1127–1134. |
[29] | Duffley G , Szabo A , Lutz BJ , Mahoney-Rafferty EC , Hess CW , Ramirez-Zamora A , Zeilman P , Foote KD , Chiu S , Pourfar MH , Goas Cnp C , Wood JL , Haq IU , Siddiqui MS , Afshari M , Heiry M , Choi J , Volz M , Ostrem JL , San Luciano M , Niemann N , Billnitzer A , Savitt D , Tarakad A , Jimenez-Shahed J , Aquino CC , Okun MS , Butson CR ((2023) ) Interactive mobile application for Parkinson’s disease deep brain stimulation (MAP DBS): An open-label, multicenter, randomized, controlled clinical trial. Parkinsonism Relat Disord 109: , 105346. |
[30] | Postuma RB , Berg D , Stern M , Poewe W , Olanow CW , Oertel W , Obeso J , Marek K , Litvan I , Lang AE , Halliday G , Goetz CG , Gasser T , Dubois B , Chan P , Bloem BR , Adler CH , Deuschl G ((2015) ) MDS clinical diagnostic criteria for Parkinson’s disease. Mov Disord Soc 30: , 1591–1601. |
[31] | Wodarg F , Herzog J , Reese R , Falk D , Pinsker MO , Steigerwald F , Jansen O , Deuschl G , Mehdorn HM , Volkmann J ((2012) ) Stimulation site within the MRI-defined STN predicts postoperative motor outcome. Mov Disord 27: , 874–879. |
[32] | Byrd RH , Gilbert JC , Nocedal J ((2000) ) A trust region method based on interior point techniques for nonlinear programming. Math Program 89: , 149–185. |
[33] | Ashburner J , Friston K (2007) CHAPTER 4 –Rigid Body Registration. In Statistical Parametric Mapping, Friston K, Ashburner J, Kiebel S, Nichols T, PennyW, eds. Academic Press, London, pp. 49-62. |
[34] | Avants BB , Tustison NJ , Song G , Cook PA , Klein A , Gee JC ((2011) ) A reproducible evaluation of ANTs similarity metric performance in brain image registration. Neuroimage 54: , 2033–2044. |
[35] | Jenkinson M , Smith S ((2001) ) A global optimisation method for robust affine registration of brain images. Med Image Anal 5: , 143–156. |
[36] | Jenkinson M , Bannister P , Brady M , Smith S ((2002) ) Improved optimization for the robust and accurate linear registration and motion correction of brain images. Neuroimage 17: , 825–841. |
[37] | Fonov V , Evans A , McKinstry R , Almli C , Collins D ((2009) ) Unbiased nonlinear average age-appropriate brain templates from birth to adulthood. Neuroimage 47: , S102. |
[38] | Ashburner J , Friston KJ ((2005) ) Unified segmentation. Neuroimage 26: , 839–851. |
[39] | Husch A , Petersen V. M , Gemmar P , Goncalves J , Hertel F ((2018) ) PaCER –A fully automated method for electrode trajectory and contact reconstruction in deep brain stimulation. Neuroimage Clin 17: , 80–89. |
[40] | Hellerbach A , Dembek TA , Hoevels M , Holz JA , Gierich A , Luyken K , Barbe MT , Wirths J , Visser-Vandewalle V , Treuer H ((2018) ) DiODe: Directional orientation detection of segmented deep brain stimulation leads: A sequential algorithm based on CT imaging. Stereotact Funct Neurosurg 96: , 335–341. |
[41] | Dembek TA , Hellerbach A , Jergas H , Eichner M , Wirths J , Dafsari HS , Barbe MT , Hunsche S , Visser-Vandewalle V , Treuer H ((2021) ) DiODe v2: Unambiguous and fully-automated detection of directional DBS lead orientation. Brain Sci 11: , 1450. |
[42] | Baniasadi M , Proverbio D , Goncalves J , Hertel F , Husch A ((2020) ) FastField: An open-source toolbox for efficient approximation of deep brain stimulation electric fields. Neuroimage 223: , 117330. |
[43] | Dice LR ((1945) ) Measures of the amount of ecologic association between species. Ecology 26: , 297–302. |
[44] | Sudhyadhom A , Haq IU , Foote KD , Okun MS , Bova FJ ((2009) ) A high resolution and high contrast MRI for differentiation of subcortical structures for DBS targeting: The Fast Gray Matter Acquisition T1 Inversion Recovery (FGATIR).T44-T. Neuroimage 47: , 52. |
[45] | Alkemade A , de Hollander G , Keuken MC , Schäfer A , Ott DVM , Schwarz J , Weise D , Kotz SA , Forstmann BU ((2017) ) Comparison of T2*-weighted and QSM contrasts in Parkinson’s disease to visualize the STN with MRI. PLoS One 12: , e0176130. |
[46] | Jaradat A , Nowacki A , Montalbetti M , Debove I , Petermann K , Schlaeppi J-A , Lachenmayer L , Tinkhauser G , Krack P , Nguyen T-AK , Pollo C ((2023) ) Probabilistic subthalamic nucleus stimulation sweet spot integration into a commercial deep brain stimulation programming software can predict effective stimulation parameters. Neuromodulation 26: , 348–355. |
[47] | Nordenström S , Petermann K , Debove I , Nowacki A , Krack P , Pollo C , Nguyen TAK ((2022) ) Programming of subthalamic nucleus deep brain stimulation for Parkinson’s disease with sweet spot-guided parameter suggestions. Front Hum Neurosci 16: , 925283. |
[48] | Hamani C , Florence G , Heinsen H , Plantinga BR , Temel Y , Uludag K , Alho E , Teixeira MJ , Amaro E , Fonoff ET ((2017) ) Subthalamic nucleus deep brain stimulation: Basic concepts and novel perspectives.ENEURO.0140-17. eNeuro 4: ,ENEURO.0140-17.2017. |
[49] | Duffley G , Anderson DN , Vorwerk J , Dorval AD , Butson CR ((2019) ) Evaluation of methodologies for computing the deep brain stimulation volume of tissue activated. J Neural Eng 16: , 066024. |
[50] | Lee J-Y , Jeon BS , Paek SH , Lim YH , Kim M-R , Kim C ((2010) ) Reprogramming guided by the fused images of MRI and CT in subthalamic nucleus stimulation in Parkinson disease. Clin Neurol Neurosurg 112: , 47–53. |
[51] | Roediger J , Dembek TA , Achtzehn J , Busch JL , Krämer A-P , Faust K , Schneider G-H , Krause P , Horn A , Kühn AA ((2023) ) Automated deep brain stimulation programming based on electrode location: A randomised, crossover trial using a data-driven algorithm. Lancet Digit Health 5: , e59–e70. |