Investigating the Shared Genetic Etiology Between Parkinson’s Disease and Depression
Abstract
Background:
Depression is a common symptom in Parkinson’s disease (PD), resulting from underlying neuropathological processes and psychological factors. However, the extent to which shared genetic risk factors contribute to the relationship between depression and PD is poorly understood.
Objective:
To examine the effects of common genetic variants influencing the etiology of PD and depression risk at the genome-wide and local genomic regional level.
Methods:
We comprehensively investigated the genetic relationship between PD and depression using genome-wide association studies data. First, we estimated the genetic correlation at the genome-wide level using linkage-disequilibrium score regression, followed by local genetic correlation analysis using the GWAS-pairwise method and functional annotation to identify genes that may jointly influence the risk for both traits. Also, we performed Latent Causal Variable, Latent Heritable Confounder Mendelian Randomization, and traditional Mendelian Randomization analyses to investigate the potential causal relationship.
Results:
Although the genetic correlation between PD and depression was not statistically significant at the genome-wide level, GWAS-pairwise analyses identified 16 genomic segments associated with PD and depression, implicating nine genes. Further analyses revealed distinct patterns within individual genes, suggesting an intricate pattern. These genes involve various biological processes, including neurotransmitter regulation, senescence, and nucleo-cytoplasmic transport mechanisms. We did not observe genetic evidence of causality between PD and depression.
Conclusions:
Our findings did not support a genome-wide genetic correlation or a causal association between both conditions. However, we identified genomic segments but identified genomic segments linked to distinct biological pathways influencing their etiology.Further research is needed to understand their functional consequences.
INTRODUCTION
Parkinson’s disease (PD) is a common neurodegenerative disorder affecting 2–3% of the population over 60 years old [1]. It is characterized by a range of motor symptoms, such as bradykinesia, tremor, and rigidity [2, 3], as well as non-motor symptoms [4], such as loss of sense of smell, drooling, gastrointestinal problems, and mood disorders, including anxiety and depression [5].
Depression is among the most common non-motor symptoms of PD, with a reported prevalence of 32.6 to 41% among individuals with PD [6–9]; however, estimates vary greatly across studies due to differences in the technical definitions of depression and assessment tools [10]. Depression is a mood disorder characterized by persistent low mood or loss of interest, and symptoms include sleep disturbance, significant weight change, and increased suicidality [11, 12]. Furthermore, depression has been shown to have a detrimental effect on the quality of life of people with PD, worsening motor outcomes, having a more negative perception of health status, and reduced engagement in treatment [13, 14].
The high prevalence of depression among individuals with PD is likely due to a combination of psychological factors and underlying neuropathological processes. From a psychological perspective, a diagnosis of PD can have an impact on patients and their families, who may face significant lifestyle adjustments to manage PD’s symptoms, potentially leading to psychological distress and increasing risk of depression [15, 16]. On the other hand, some of the most prominent pathological alterations leading to neurological manifestations of PD, such as the degeneration of dopaminergic neurons in the substantia nigra and the accumulation of Lewy bodies, especially in subcortical brain regions, have also been associated, to a lesser extent, with depression [9, 17]. Similarly, the loss of adrenergic and serotonergic neurons may contribute to the development of depression symptoms [18–20].
The etiology of both PD and depression has been studied at the genetic level, with both disorders showing a moderate heritability; estimates are 34% for PD [21] and 38% for depression [22]. Furthermore, genome-wide association studies (GWAS) have identified 90 and 178 genomic risk loci for PD [23] and depression [24], respectively. Notably, a previous study suggested a shared familial susceptibility to PD with depression [25].
Although PD and depression have been observed as co-occurring disorders, there is a dearth of research investigating the shared genetic factors that might underlie their co-occurrence. In the present study, we examine the shared genetic factors that influence the etiology of PD and depression at the genome-wide and regional genomic levels. In addition, we investigate potential causal genetic effects between these conditions. This study aims to elucidate the genetic relationship between PD and depression and highlight specific segments of the genome that could be of interest to follow-up in future molecular studies.
MATERIALS AND METHODS
GWAS summary statistics data for PD and depression
We used summary statistics from a GWAS meta-analysis for PD, which included data from several sources, including the International Parkinson’s Disease Genomics Consortium (IPDGC), 23andMe, Inc., and the UK Biobank. A fixed-effects meta-analysis was performed using METAL [26]. The data included a total sample size of 1,474,097 participants of European ancestry, of which approximately 37.7 K were cases, 18.6 K were UK Biobank proxy cases (having a first-degree relative with PD), and 1,417,791 were controls.
More information about the GWAS meta-analysis is available in the corresponding publication [23]. Summary-level data from the 23andMe cohort was obtained through the corresponding application procedure (https://research.23andme.com/dataset-access/) and Institutional Data Transfer Agreement. Additionally, we obtained a version of this summary statistics without 23andMe, Inc., and the UK Biobank from corresponding authors [23].
For our analysis, the GWAS summary statistics for depression were provided by the Psychiatric Genomics Consortium (PGC). The summary statistics were estimated through an inverse-variance weighted meta-analysis of European ancestry individuals from the UK Biobank, the PGC, and 23andMe. The total sample included 807,553 individuals (246,363 cases and 561,190 controls). Full details of the GWAS are available in the corresponding publication [27] and through the PGC website (https://www.med.unc.edu/pgc/download-results/), excluding the 23andMe sample. Additionally, summary data for the 23andMe cohort was obtained through the relevant application process, following a Data Transfer Agreement (https://research.23andme.com/dataset-access/).
Genome-wide and local genomic regional level analyses
To measure the genetic correlation between PD and depression at the whole-genome level, we employed the Linkage-disequilibrium score regression method (LDSC), which is robust and can account for potential sample overlap [28]. Additionally, we used the GWAS pairwise (GWAS-PW) method [29] to further investigate the genetic overlap between PD and depression by identifying specific segments of the genome shared across these two phenotypes.
The GWAS-PW method divides the genome into approximately 1,703 independent genomic segments, and for each segment, it estimates the posterior probability of association (PPA) for four models. These models include (i) the genomic segment is uniquely associated with PD, (ii) the genomic segment is uniquely associated with depression, (iii) the genomic segment is associated with both traits via the same genetic variants, and (iv) the genomic segment is associated with both phenotypes through different genetic variants. In this study, we focused on genomic segments for which model three (i.e., shared causal genetic variants) had a PPA > 0.5 [30].
Functional annotation
We used the FUMA (Functional Mapping and Annotation) [31] online platform, version 1.4.1, for the entire genome to perform functional annotation. We then filtered functional data for the specific genomic segments identified through GWAS-PW. We used Multi-marker Analysis of GenoMic Annotation (MAGMA) [32] online platform version 1.3.7 to conduct gene-based tests and map protein-coding genes to the genomic segments of interest. To correct for multiple comparisons, we applied a Bonferroni correction with a significance level of 0.05 divided by the total number of genes in shared regions.
Comparison of SNP effect directions and regional visualization
To explore the correlation between effect sizes and directions derived from the initial GWAS summary statistics for PD and depression, we computed the Pearson correlation coefficient, considering only variants with a significance threshold of p < 0.05. In addition, we generated beta-beta plots to provide a visual representation of these associations. These analyses were carried out specifically for the individual genes mapped to protein-coding genes in the genomic segments influencing the etiology of PD and depression [33].
After performing functional annotation, we observed that most genes associated with PD and depression were located on chromosome 22. To investigate the genomic region further, we created a regional plot employing the topr tool version 1.1.8 [34]. LocusZoom [35] website (https://my.locuszoom.org/) was utilized to generate regional plots and investigate linkage disequilibrium (LD) patterns between the most significant SNPs for each GWAS in the region.
Latent causal variable
We performed Latent Causal Variable (LCV) analysis to assess whether horizontal pleiotropy or potential causal genetic effects (i.e., vertical pleiotropy) [36] could drive the association between PD and depression. Briefly, this method models a latent variable L as a mediator of the genetic correlation between the phenotypes under study, in this case, PD and depression. Furthermore, by comparing the correlation between each phenotype with the latent variable, the LCV method estimates the genetic causal proportion (GCP) parameter. The GCP represents the proportion of the genetic correlation likely to be explained by vertical pleiotropy. Therefore, it ranges from –1 to 1, where a GCP = 0 suggests the absence of potential genetic causality.
In contrast, |GCP| = 1 suggests the full identification of vertical pleiotropic effects supporting a potential causal association. Some of the hallmarks of the LCV method include being less susceptible to bias by horizontal pleiotropic effects and being robust to sample overlap [36–38]. Further details regarding this method and how to interpret findings are available elsewhere [36, 39, 40].
Latent heritable confounder mendelian randomization
We performed Latent Heritable Confounder Mendelian Randomization (LHC-MR) analyses to assess bidirectional potential causal genetic effects between PD and depression regardless of sample overlap, given that the GWAS summary statistics for both traits include samples from 23andMe and the UK Biobank cohorts. Unlike traditional MR methods, LHC-MR uses the full set of GWAS summary statistics to investigate potential causal associations between two complex traits, improving statistical power to estimate bi-directional causal genetic effects, direct heritabilities, and confounder effects while accounting for sample overlap. Notably, LHC-MR can outperform several traditional MR methods [41]. Full details for the LHC-MR method are described elsewhere [41].
Sensitivity analyses
Mendelian randomization
Mendelian randomization (MR) is used to infer causal genetic effects between an exposure and an outcome phenotype [37, 42] based on three assumptions: genetic variants are only associated with the outcome via exposure, not confounded, and robustly associated with the exposure phenotype, independent of the outcome [43, 44]. To ensure no sample overlap between PD and Depression GWAS, we employed a version of the PD GWAS without participants in the 23andMe and UK Biobank cohorts. Therefore, we were only able to investigate potential causal genetic effects using PD as the exposure and depression as the outcome due to the low number of instrumental variables available.
The study used a modified version of the PD GWAS without participants from the 23andMe and UK Biobank cohorts to avoid sample overlap between PD and Depression GWAS. This allowed for the investigation of potential causal genetic effects using PD as the exposure and depression as the outcome due to limited instrumental variables.
We conducted MR analyses using R-4.0.2 [45, 46] and three libraries: MR-Base, MRInstruments, and TwoSampleMR. Specifically, we used five MR methods to investigate potential causal genetic effects between PD and depression. These methods included inverse variance weighted (IVW), MR Egger (MR-Egger), weighted median method, simple mode MR, and the weighted mode method. The IVW method estimates the magnitude of the potential causal genetic effects as the average effect between the instrumental variable and the outcome, divided by the effect between the instrumental variable and the exposure [47]. The MR-Egger method is used to conduct a weighted linear regression in which the intercept can vary freely, and the slope indicates the potential causal genetic effect free from horizontal pleiotropic effects [47, 48]. In addition, the weighted median method estimates putative causal genetic effects while adjusting for up to half of the instrumental variables with horizontal pleiotropic effects. The weighted mode method weights the number of instrumental variables in each cluster through the inverse variance of the effect on the outcome phenotype [47], and the simple mode MR clusters instrumental variables based on similarity with the putative causal effect estimates [47]. We also used the MR-PRESSO (Mendelian Randomization Pleiotropy RESidual Sum and Outlier) method to evaluate the potential horizontal pleiotropic outlier SNPs between PD and depression [49].
RESULTS
Genetic correlation and functional annotation
The genetic correlation between PD and depression was examined using LD score regression. Whole-genome analysis did not reveal a significant correlation between the two traits (rG = 0.03, p = 0.26).
We conducted a pairwise GWAS analysis to investigate the relationship between PD and depression at the local genetic level. We identified 16 genomic segments associated with both phenotypes through the same genetic variants (Supplementary Table 1). We mapped these genomic regions to protein-coding genes using MAGMA (Supplementary Table 2). The results showed that nine genes were associated with PD and depression (Table 1). These included RBMS1, PCLO, ASXL3, EP300, L3MBTL2, CHADL, RANGAP1, ZC3H7B, and TEF.
Table1
Genes in genomic segments identified via pairwise GWAS are associated with both Parkinson’s disease (PD) and depression
Chromosome | Gene | Biological relevance | PD gene-based test p | Depression gene-based test p |
2 | RBMS1 | •Encodes a protein involved in DNA replication, gene transcription, and cell apoptosis [68]. | 3.85E–06 | 1.60E–04 |
•It has been linked to cellular senescence and aging [69] and implicated in neocortex development [68]. | ||||
7 | PCLO | •Involved in disrupted presynaptic vesicle trafficking [70]. | 6.39E–05 | 1.28E–12 |
•Previously associated with depression [70]. | ||||
18 | ASXL3 | •Involved in gene transcription via lipogenesis or inhibiting histone deubiquitination [71]. | 9.21E–06 | 3.48E–05 |
•It is predominantly expressed in tissues such as the brain, spinal cord, kidney, liver, and bone marrow [72]. | ||||
•ASXL3 is a risk locus for PD [23]. | ||||
•Differentially expressed in major depressive disorder [73]. | ||||
22 | EP300 | •Histone acetyltransferase that regulates gene transcription processes [74,75]. | 8.47E–07 | 8.35E–06 |
•Promising gene target for molecular docking in depression therapy [76]. | ||||
22 | L3MBTL2 | •Involved in disrupted dopamine metabolism [77]. | 8.42E–07 | 7.66E–05 |
•Potential important target for the study of PD biomarkers [77]. | ||||
22 | CHADL | •Modulation of chondrocyte differentiation and extracellular matrix formation [79]. | 1.18E–06 | 4.49E–05 |
•Underlying genetic factor between depression and osteoarthritis [80]. | ||||
22 | RANGAP1 | •Dysfunction of nucleo-cytoplasmic transport mechanisms [81,82], ultimately affecting dopaminergic neurons [65]. | 1.87E–07 | 1.09E–07 |
22 | ZC3H7B | •Predominantly known for being differentially expressed in cerebral cortex tissue, specifically in individuals with depression [73,83]. | 2.25E–07 | 7.57E–07 |
•Putatively involved in translation regulation [84]. | ||||
22 | TEF | •Involved in the regulation of circadian rhythm [85]. | 1.57E–05 | 2.19E–04 |
•TEF Polymorphism is associated with sleep disturbances and predicted deterioration in sleep quality in PD patients [86]. |
Heterogeneity in effects for selected SNPs and regional visualization
We evaluated the correlation between effect sizes and directions from the original GWAS analyses by computing the Pearson correlation coefficient for nominally significant SNPs within previously identified gene loci and generating beta-beta plots (Supplementary Figure 1). The SNPs showed a weak correlation (r = 0.2, p < 0.001). However, distinct patterns emerged when examining SNPs located within individual genes, such as positive correlations in genes identified as RBMS1 (r = 0.4, p < 0.001), PCLO (r = 0.4, p < 0.001), and ASXL3 (r = 0.99, p < 0.001) and negative correlations for SNPs located in genes in chromosome 22, EP300 (r = –0.9, p < 0.001), L3MBTL2 (r = 0.89, p < 0.001), CHADL (r = 0.91, p < 0.001), RANGAP1 (r = 0.45, p < 0.001), ZC3H7B (r = 0.98, p < 0.001), and TEF (r = 0.91, p < 0.001).
We used regional association plots to explore further the genomic region on chromosome 22 that harbored six of the nine genes within genomic segments associated with PD and depression (±500 kb). The top associated SNPs in each GWAS dataset (rs5751084 for PD and rs5995992 for depression) were located within different LD blocks (Fig. 1, Supplementary Figure 2). The regional plots that show the LD structure of the segment (Supplementary Figure 2) suggest that there are two LD blocks in the chr22 : 41452876-41829000 region, defined using a criterion of r2 > 0.6. The LD r-squared between the two top SNPs (rs5751084 and rs5995992) was 0.19 in the CEU population and 0.20 in the GBR population (according to LDlink: https://ldlink.nih.gov/).
Fig. 1
Regional plot for the chr22 : 41452876-41829000 region in GWAS summary statistics showing SNPs associated with Parkinson’s disease (PD) in blue and depression in yellow. Top variants within the region for each dataset are annotated by their rs number, and vertical gray dotted lines highlight their positions within the genes shown in the lower panel, which only includes protein-coding genes. The horizontal red dashed line represents the common genome-wide significance threshold.
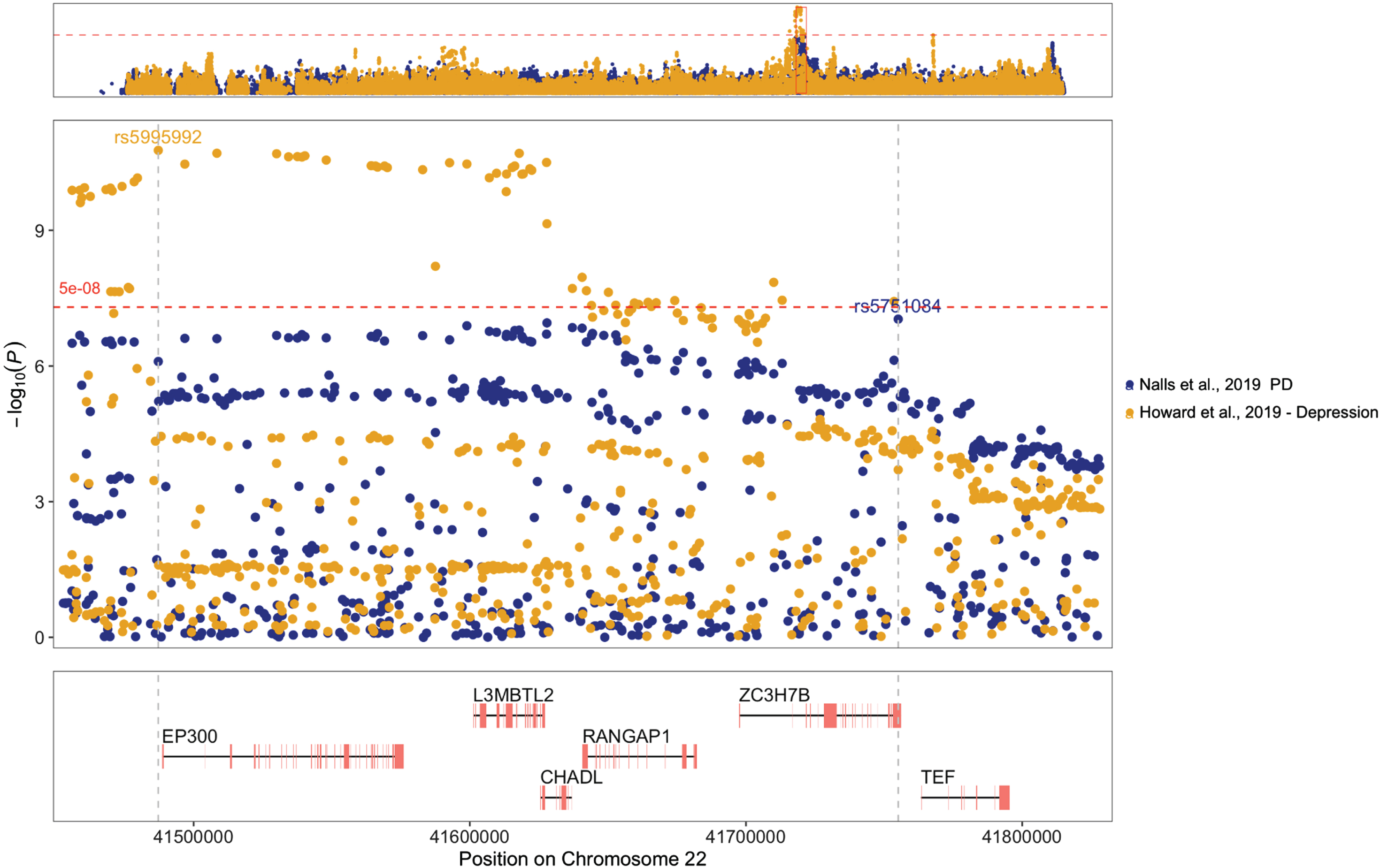
Potential causal genetic effects
We performed LCV and LHC-MR analyses to investigate possible causal genetic effects between PD and depression. We did not observe evidence of genetic causality between these phenotypes with the LCV (GCP = 0, SE = 0.58, p = 0.89). Furthermore, the examination of bidirectional causal effects with the LHC-MR method did not provide evidence of potential causal genetic effects of PD on depression risk (Beta = 0.16, SE = 0.10, p = 0.11) or of depression on PD risk (Beta = 0.01, SE = 0.03, p = 0.70). The heritability, confounder, and polygenicity estimates from the HLC-MR method suggest that there is a genetic contribution to PD and depression, and that external (i.e., environmental) factors could also influence this relationship (Supplementary Table 3).
Sensitivity analyses
Mendelian randomization
To investigate potential causal genetic effects between PD and depression, we performed a Generalized Summary-data-based Mendelian Randomization analysis using GWAS summary statistics for PD that excluded the UK Biobank and 23andMe samples. We utilized several MR methods, including MR Egger, weighted median, inverse variance weighted, simple mode, and weighted mode, to examine the relationship between these two traits. We did not find any evidence suggesting that genetic liability to PD increases the likelihood of developing depression with any of the MR methods (Supplementary Figure 3 and Supplementary Table 4). Additionally, the instrumental variables used for the MR analysis did not show significant heterogeneous outlier SNPs (MR-PRESSO global test = 0.10), and the MR-Egger intercept did not indicate horizontal pleiotropy (Egger intercept = 0.0062, p = 0.37) (Supplementary Tables 5–7). However, it is important to note that with the MR Egger method, there is evidence of heterogeneity among the instrumental variables used, as indicated by the Q statistics, suggesting variability that could influence the MR estimates. This heterogeneity was not observed in the MR-PRESSO method. Furthermore, our inspection of the funnel plot symmetry did not reveal any evidence of unbalanced pleiotropy (Supplementary Figures 4 and 5).
DISCUSSION
The present study used LDSC, GWAS-PW, LCV, and MR approaches to explore the genetic overlap between PD and depression. Although our findings do not support a genome-wide genetic correlation or causal genetic effects between both disorders at the whole-genome level, we provide evidence for genomic segments and molecular pathways that influence the etiology of both PD and depression.
Our findings represent a paradox due to the high prevalence of depression among patients with PD [50]. They could suggest that genetic factors may not solely drive the epidemiological association between PD and depression. Alternatively, there are several possible explanations for our observations. First, significant local positive and negative genetic correlations in different genomic segments could cancel each other and result in a non-significant correlation at the whole-genome level. Second, the depression GWAS used here is unlikely to be enriched for PD, and the etiology of PD-related depression could differ from other forms of depression. Lastly, individuals might develop depression as a result of being diagnosed with PD or neurotransmission abnormalities [18, 51–53], brain circuit disturbances [54, 55], and the development of other non-motor symptoms related to PD’s prodromal phase [56], such as REM sleep behavior disorder, constipation and impairment of daily activities. Prodromal PD is characterized by the manifestation of non-motor symptoms years or even decades before the onset of motor symptoms [57]. Finally, the association between PD and depression could be partially explained by environmental factors.
Below, we discuss some of our findings at a local genetic level after performing functional annotation of genomic segments that influence depression and PD, providing meaningful insights into the biological pathways that could explain previous observations at the phenotypic level. Although further research is required to understand the co-occurrence between PD and depression, our findings could explain the underlying molecular pathways specific to depression in PD.
The relationship between PD and depression is complex. Although the severity of depression in PD appears to be greater than among patients living with other chronic medical illnesses [58], there is no consensus on whether PD-related depression substantially differs from major depressive disorder and other forms of depression [59]. Nonetheless, studies have suggested an increased risk of developing PD for patients with a previous diagnosis of depression [50, 60]. This observation is supported by further reports of a higher incidence rate of depressive symptoms among PD patients, particularly during the prodromal phase [60]. In addition, the onset of depressive symptoms among individuals with PD is observed between 2–10 [61] and up to 17 years [62] before PD diagnosis or the manifestation of predominant motor symptoms. Therefore, to understand the onset and development of depressive symptoms in preclinical PD, possible underlying mechanisms have been scrutinized, including alterations in the neural circuitry in the basal ganglia and the lateral habenula [63].
In the present study, we identified nine genes associated with the risk of developing PD and depression, six located on chromosome 22. Our study revealed that SNPs within genes located on chromosomes 2, 7, and 18 exhibited a consistently strong and statistically significant positive correlation in their effects. In contrast, SNPs in chromosome 22 showed a negative strong and significant correlation, suggesting a complex correlation pattern in which SNP effects tend to cancel each other out because they go in opposite directions (i.e., some increase risk while others decrease risk), resulting in no significant genetic correlation at the genome-wide level. In addition, regional analysis and LD observations indicated that SNPs associated with PD and depression located in chromosome 22 are in different LD blocks. These findings highlight the heterogeneous nature of PD and depression, suggesting that distinct genetic variants underlie the associations observed for each phenotype.
To different extents, these genes have been described in previous studies seeking to understand the etiology of PD, or depression. For instance, RANGAP1 and altered nucleo-cytoplasmic transport mechanisms have been associated with PD, depression, and other neuropsychiatric disorders [64–66]. In particular, the misvocalization of transcription factors, such as NF-κB has been observed to increase nuclear translocation in dopaminergic neurons in the brains of individuals with PD [65]. Similarly, pCREB cytoplasmic aggregates have been reported in the substantia nigra among PD patients, and these phenomena have been demonstrated to be specific to dopaminergic neurons [65]. Therefore, our results suggest that the dysfunction of nucleo-cytoplasmic transport mechanisms related to RANGAP1, might be involved to different extents in the etiology of both depression and PD due to shared signaling pathways, ultimately affecting dopaminergic neurons. Further details for the biological roles of genes identified in the present study are summarized in Table 1.
The limitations of the present study must be addressed. For instance, one of the pitfalls when surveying depression is the heterogeneity of its assessment, diagnosis, and/or classification [67]. Here, we leveraged GWAS summary data by Howard et al. (2019), including individuals of European ancestry with a broad definition of depression from UK Biobank, which includes self-reports of having received a clinical diagnosis or treatment for depression. Moreover, as we employed data from European-ancestry-only individuals, the generalizability of our findings must be addressed with caution until confirmed in samples from other populations and ethnicities.
Furthermore, the evaluation of the magnitude and direction of effect sizes was limited to genetic variants of nominal significance (p < 0.05) within the regions of interest. Only one of these variants (rs5995992) showed genome-wide significance (p < 5E–8) in the depression GWAS, while none were genome-wide significant in the PD GWAS. Ideally, having several genetic variants surviving the genome-wide threshold for both phenotypes would have given our observations more robustness. In addition, we recognize that MR methods assume the use of non-overlapping samples between the phenotypes under study. We note that we were unable to fulfil this assumption as both GWAS summary statistics for PD and depression include samples from the UK Biobank and 23andMe participants [23, 27].
In summary, we leveraged various methods to investigate the genetic overlap between PD and depression. While our findings do not support a genome-wide genetic correlation or direct causal link between these two conditions, they emphasize the complex nature of their association. Our results imply that shared genomic segments are linked to distinct genes, which participate in pathways that may contribute to the etiology of PD and depression. These findings provide insights into the complex genetic mechanisms underlying these disorders and suggest that genomic segments influencing both phenotypes may play a crucial role in understanding the pathogenesis of PD and depression.
ACKNOWLEDGMENTS
PRP is a doctoral student from Programa de Doctorado en Ciencias Biomédicas, Universidad Nacional Autónoma de México (UNAM). This work received support from Luis Aguilar, Alejandro León, and Jair García of the Laboratorio Nacional de Visualización Científica Avanzada. We also thank Carina Uribe Díaz, Alejandra Castillo Carbajal, and Christian Molina-Aguilar for their technical support. We would like to thank the research participants and employees of 23andMe, Inc. for making this work possible.
FUNDING
PRP’s receives a fellowship from the GP2 Trainee Network, part of the Global Parkinson’s Genetics Program, and funded by the Aligning Science Across Parkinson’s (ASAP) initiative.
LMGM’s support comes from a UQ Research Training Scholarship from The University of Queensland (UQ). MER is funded by Australia’s National Health and Medical Research Council (GNT1102821), the Rebecca L Cooper Medical Research Foundation (F20231230), and The Michael J. Fox Foundation and Shake It Up Australia Foundation (MJFF-021952).
BLM’s support is through the Australian National Health and Medical Research Council Investigator grant scheme (APP2017176). AMR was supported by CONACYT-FORDECYT-PRONACES grants no. [11311] and [6390], Programa de Apoyo a Proyectos de Investigación e Innovación Tecnológica–Universidad Nacional Autónoma de México (PAPIIT-UNAM) grants no. IA203021 and IN218023, and Chan Zuckerberg Initiative Ancestry Network (2021-240438).
CONFLICT OF INTEREST
The author have no conflict of interest to report.
DATA AVAILABILITY
Full GWAS summary statistics for PD and depression, including the 23andMe cohort, are available upon request through a Data Transfer Agreement and the appropriate application procedure (https://research.23andme.com/dataset-access/). Summary-level data for depression, excluding the 23andMe cohort, is publicly available on the Psychiatric Genomics Consortium website (https://www.med.unc.edu/pgc/shared-methods/open-source-philosophy/).
SUPPLEMENTARY MATERIAL
[1] The supplementary material is available in the electronic version of this article: https://dx.doi.org/10.3233/JPD-230176.
REFERENCES
[1] | Pringsheim T , Jette N , Frolkis A , Steeves TDL ((2014) ) The prevalence of Parkinson’s disease: A systematic review and meta-analysis. Mov Disord 29: , 1583–1590. |
[2] | Moustafa AA , Chakravarthy S , Phillips JR , Gupta A , Keri S , Polner B , Frank MJ , Jahanshahi M ((2016) ) Motor symptoms in Parkinson’s disease: A unified framework. Neurosci Biobehav Rev 68: , 727–740. |
[3] | Váradi C ((2020) ) Clinical features of Parkinson’s disease: The evolution of critical symptoms. Biology 9: , 103. |
[4] | Fereshtehnejad S-M , Shafieesabet M , Farhadi F , Hadizadeh H , Rahmani A , Naderi N , Khaefpanah D , Shahidi GA , Delbari A , Lökk J ((2015) ) Heterogeneous determinants of quality of life in different phenotypes of Parkinson’s disease. PLoS One 10: , e0137081. |
[5] | Aarsland D , Marsh L , Schrag A ((2009) ) Neuropsychiatric symptoms in Parkinson’s disease. Mov Disord 24: , 2175–2186. |
[6] | Cong S , Xiang C , Zhang S , Zhang T , Wang H , Cong S ((2022) ) Prevalence and clinical aspects of depression in Parkinson’s disease: A systematic review and meta-analysis of 129 studies. Neurosci Biobehav Rev 141: , 104749. |
[7] | Gustafsson H , Nordström A , Nordström P ((2015) ) Depression and subsequent risk of Parkinson disease: A nationwide cohort study. Neurology 84: , 2422–2429. |
[8] | Khedr EM , Abdelrahman AA , Elserogy Y , Zaki AF , Gamea A ((2020) ) Depression and anxiety among patients with Parkinson’s disease: Frequency, risk factors, and impact on quality of life. Egypt J Neurol Psychiatry Neurosurg 56: , 116. |
[9] | Aarsland D , Påhlhagen S , Ballard CG , Ehrt U , Svenningsson P ((2011) ) Depression in Parkinson disease— epidemiology, mechanisms and management. Nat Rev Neurol 8: , 35–47. |
[10] | Laux G ((2022) ) Parkinson and depression: Review and outlook. J Neural Transm 129: , 601–608. |
[11] | American Psychiatric Association (2022) Diagnostic and Statistical Manual of Mental Disorders: DSM-5-TR, American Psychiatric Association Publishing. |
[12] | Aman AM , García-Marín LM , Thorp JG , Campos AI , Cuellar-Partida G , Martin NG , Rentería ME ((2022) ) Phenome-wide screening of the putative causal determinants of depression using genetic data. Hum Mol Genet 31: , 2887–2898. |
[13] | Schrag A ((2006) ) Quality of life and depression in Parkinson’s disease. J Neurol Sci 248: , 151–157. |
[14] | Su W , Liu H , Jiang Y , Li S , Jin Y , Yan C , Chen H ((2021) ) Correlation between depression and quality of life in patients with Parkinson’s disease. Clin Neurol Neurosurg 202: , 106523. |
[15] | Chaudhuri KR , Healy DG , Schapira AHV ,((2006) ) National Institute for Clinical Excellence (2006) Non-motor symptoms of Parkinson–s disease: Diagnosis and management.. Lancet Neurol 5: , 235–245. |
[16] | Rod NH , Bordelon Y , Thompson A , Marcotte E , Ritz B ((2013) ) Major life events and development of major depression in Parkinson’s disease patients. Eur J Neurol 20: , 663–670. |
[17] | Tsopelas C , Stewart R , Savva GM , Brayne C , Ince P , Thomas A , Matthews FE , Neuropathological correlates of late-life depression in older peopleMedical Research Council Cognitive Function and Ageing Study (2011) Neuropathological correlates of late-life depression in older people.. Br J Psychiatry 198: , 109–114. |
[18] | Remy P , Doder M , Lees A , Turjanski N , Brooks D ((2005) ) Depression in Parkinson’s disease: Loss of dopamine and noradrenaline innervation in the limbic system. Brain 128: , 1314–1322. |
[19] | Frisina PG , Haroutunian V , Libow LS ((2009) ) The neuropathological basis for depression in Parkinson’s disease. Parkinsonism Relat Disord 15: , 144–148. |
[20] | Jørgensen LM , Henriksen T , Mardosiene S , Keller SH , Stenbæk DS , Hansen HD , Jespersen B , Thomsen C , Weikop P , Svarer C , Knudsen GM ((2021) ) Parkinson patients have a presynaptic serotonergic deficit: A dynamic deep brain stimulation PET study. J Cereb Blood Flow Metab 41: , 1954–1963. |
[21] | Wirdefeldt K , Gatz M , Reynolds CA , Prescott CA , Pedersen NL ((2011) ) Heritability of Parkinson disease in Swedish twins: A longitudinal study.. Neurobiol Aging 32: , 1–1923.e1-88. |
[22] | Kendler KS , Gatz M , Gardner CO , Pedersen NL ((2006) ) A Swedish national twin study of lifetime major depression. Am J Psychiatry 163: , 109–114. |
[23] | Nalls MA , Blauwendraat C , Vallerga CL , Heilbron K , Bandres-Ciga S , Chang D , Tan M , Kia DA , Noyce AJ , Xue A , Bras J , Young E , von Coelln R , Simón-Sánchez J , Schulte C , Sharma M , Krohn L , Pihlstrøm L , Siitonen A , Iwaki H , Leonard H , Faghri F , Gibbs JR , Hernandez DG , Scholz SW , Botia JA , Martinez M , Corvol J-C , Lesage S , Jankovic J , Shulman LM , Sutherland M , Tienari P , Majamaa K , Toft M , Andreassen OA , Bangale T , Brice A , Yang J , Gan-Or Z , Gasser T , Heutink P , Shulman JM , Wood NW , Hinds DA , Hardy JA , Morris HR , Gratten J , Visscher PM , Graham RR , Singleton AB ,23andMe Research Team, System Genomics of Parkinson’s Disease Consortium, International Parkinson’s Disease Genomics Consortium ((2019) ) Identification of novel risk loci, causal insights, and heritable risk for Parkinson’s disease: A meta-analysis of genome-wide association studies. Lancet Neurol 18: , 1091–1102. |
[24] | Levey DF , Stein MB , Wendt FR , Pathak GA , Zhou H , Aslan M , Quaden R , Harrington KM , Nuñez YZ , Overstreet C , Radhakrishnan K , Sanacora G , McIntosh AM , Shi J , Shringarpure SS , 23andMe Research Team Million Veteran Program. Concato J , Polimanti R , Gelernter J ((2021) ) Bi-ancestral depression GWAS in the Million Veteran Program and meta-analysis in >1.2 million individuals highlight new therapeutic directions. Nat Neurosci 24: , 954–963. |
[25] | Arabia G , Grossardt BR , Geda YE , Carlin JM , Bower JH , Ahlskog JE , Maraganore DM , Rocca WA ((2007) ) Increased risk of depressive and anxiety disorders in relatives of patients with Parkinson disease. Arch Gen Psychiatry 64: , 1385–1392. |
[26] | Willer CJ , Li Y , Abecasis GR ((2010) ) METAL: Fast and efficient meta-analysis of genomewide association scans. Bioinformatics 26: , 2190–2191. |
[27] | Howard DM , Adams MJ , Clarke T-K , Hafferty JD , Gibson J , Shirali M , Coleman JRI , Hagenaars SP , Ward J , Wigmore EM , Alloza C , Shen X , Barbu MC , Xu EY , Whalley HC , Marioni RE , Porteous DJ , Davies G , Deary IJ , Hemani G , Berger K , Teismann H , Rawal R , Arolt V , Baune BT , Dannlowski U , Domschke K , Tian C , Hinds DA , Trzaskowski M , Byrne EM , Ripke S , Smith DJ , Sullivan PF , Wray NR , Breen G , Lewis CM , McIntosh AM ((2019) ) Genome-wide meta-analysis of depression identifies 102 independent variants and highlights the importance of the prefrontal brain regions. Nat Neurosci 22: , 343–352. |
[28] | Bulik-Sullivan BK , Loh P-R , Finucane HK , Ripke S , Yang J , Patterson N , Daly MJ , Price AL , Neale BM ((2015) ) LD Score regression distinguishes confounding from polygenicity in genome-wide association studies. Nat Genet 47: , 291–295. |
[29] | Pickrell JK , Berisa T , Liu JZ , Ségurel L , Tung JY , Hinds DA ((2016) ) Detection and interpretation of shared genetic influences on 42 human traits. Nat Genet 48: , 709–717. |
[30] | Mitchell BL , Thorp JG , Evans DM , Nyholt DR , Martin NG , Lupton MK ((2020) ) Exploring the genetic relationship between hearing impairment and Alzheimer’s disease. Alzheimers Dement 12: , e12108. |
[31] | Watanabe K , Taskesen E , van Bochoven A , Posthuma D ((2017) ) Functional mapping and annotation of genetic associations with FUMA. Nat Commun 8: , 1826. |
[32] | de Leeuw CA , Mooij JM , Heskes T , Posthuma D ((2015) ) MAGMA: Generalized gene-set analysis of GWAS data. PLoS Comput Biol 11: , e1004219. |
[33] | Makarious MB (2023) GP2code/GP2-AFR-AACmetaGWAS: Initial Release, Zenodo. |
[34] | Juliusdottir T ((2023) ) topr: An R package for viewing and annotating genetic association results. BMC Bioinformatics 24: , 268. |
[35] | Boughton AP , Welch RP , Flickinger M , VandeHaar P , Taliun D , Abecasis GR , Boehnke M ((2021) ) LocusZoom.js: Interactive and embeddable visualization of genetic association study results. Bioinformatics 37: , 3017–3018. |
[36] | O’Connor LJ , Price AL ((2018) ) Distinguishing genetic correlation from causation across 52 diseases and complex traits. Nat Genet 50: , 1728–1734. |
[37] | García-Marín LM , Campos AI , Martin NG , Cuéllar-Partida G , Rentería ME ((2020) ) Inference of causal relationships between sleep-related traits and 1,527 phenotypes using genetic data. Sleep 44: , zsaa154. |
[38] | García-Marín LM , Campos AI , Martin NG , Cuéllar-Partida G , Rentería ME ((2021) ) Phenome-wide analysis highlights putative causal relationships between self-reported migraine and other complex traits. J Headache Pain 22: , 66. |
[39] | García-Marín LM , Campos AI , Kho P-F , Martin NG , Cuéllar-Partida G , Rentería ME ((2021) ) Phenome-wide screening of GWAS data reveals the complex causal architecture of obesity. Hum Genet 140: , 1253–1265. |
[40] | García-Marín LM , Campos AI , Cuéllar-Partida G , Medland SE , Kollins SH , Rentería ME ((2021) ) Large-scale genetic investigation reveals genetic liability to multiple complex traits influencing a higher risk of ADHD. Sci Rep 11: , 22628. |
[41] | Darrous L , Mounier N , Kutalik Z ((2021) ) Simultaneous estimation of bi-directional causal effects and heritable confounding from GWAS summary statistics. Nat Commun 12: , 7274. |
[42] | Siewert KM , Klarin D , Damrauer SM , Chang KM , Tsao PS , Assimes TL , Davey SG , Voight BF ((2020) ) Cross-trait analyses with migraine reveal widespread pleiotropy and suggest a vascular component to migraine headache. Int J Epidemiol 49: , 1022–1031. |
[43] | Zhu Z , Zheng Z , Zhang F , Wu Y , Trzaskowski M , Maier R , Robinson MR , McGrath JJ , Visscher PM , Wray NR , Yang J ((2018) ) Causal associations between risk factors and common diseases inferred from GWAS summary data. Nat Commun 9: , 224. |
[44] | Benn M , Nordestgaard BG ((2018) ) From genome-wide association studies to Mendelian randomization: Novel opportunities for understanding cardiovascular disease causality, pathogenesis, prevention, and treatment. Cardiovasc Res 114: , 1192–1208. |
[45] | Venables WN , Smith DM (2003) The R development core team. An Introduction to R, Version 1. |
[46] | R Core Team (2018). R: A language and environment for statistical computing. R Foundation for Statistical Computing, Vienna, Austria. Available online athttps://www.R-project.org. |
[47] | Hwang L-D , Lawlor DA , Freathy RM , Evans DM , Warrington NM ((2019) ) Using a two-sample Mendelian randomization design to investigate a possible causal effect of maternal lipid concentrations on offspring birth weight. Int J Epidemiol 48: , 1457–1467. |
[48] | Bowden J , Davey Smith G , Burgess S ((2015) ) Mendelian randomization with invalid instruments: Effect estimation and bias detection through Egger regression. Int J Epidemiol 44: , 512–525. |
[49] | Verbanck M , Chen C-Y , Neale B , Do R ((2018) ) Detection of widespread horizontal pleiotropy in causal relationships inferred from Mendelian randomization between complex traits and diseases. Nat Genet 50: , 693–698. |
[50] | Marsh L ((2013) ) Depression and Parkinson’s disease: Current knowledge. Curr Neurol Neurosci Rep 13: , 409. |
[51] | Liu K-C , Li J-Y , Tan H-H , Du C-X , Xie W , Zhang Y-M , Ma W-L , Zhang L ((2015) ) Serotonin6 receptors in the dorsal hippocampus regulate depressive-like behaviors in unilateral 6-hydroxydopamine-lesioned Parkinson’s rats. Neuropharmacology 95: , 290–298. |
[52] | Kamińska K , Lenda T , Konieczny J , Czarnecka A , Lorenc-Koci E ((2017) ) Depressive-like neurochemical and behavioral markers of Parkinson’s disease after 6-OHDA administered unilaterally to the rat medial forebrain bundle. Pharmacol Rep 69: , 985–994. |
[53] | Pagano G , Niccolini F , Fusar-Poli P , Politis M ((2017) ) Serotonin transporter in Parkinson’s disease: A meta-analysis of positron emission tomography studies. Ann Neurol 81: , 171–180. |
[54] | Qiu Y-H , Huang Z-H , Gao Y-Y , Feng S-J , Huang B , Wang W-Y , Xu Q-H , Zhao J-H , Zhang Y-H , Wang L-M , Nie K , Wang L-J ((2021) ) Alterations in intrinsic functional networks in Parkinson’s disease patients with depression: A resting-state functional magnetic resonance imaging study. CNS Neurosci Ther 27: , 289–298. |
[55] | Wang H , Chen H , Wu J , Tao L , Pang Y , Gu M , Lv F , Luo T , Cheng O , Sheng K , Luo J , Hu Y , Fang W ((2018) ) Altered resting-state voxel-level whole-brain functional connectivity in depressed Parkinson’s disease. Parkinsonism Relat Disord 50: , 74–80. |
[56] | Antar T , Morris HR , Faghri F , Leonard HL , Nalls MA , Singleton AB , Iwaki H ((2021) ) Longitudinal risk factors for developing depressive symptoms in Parkinson’s disease. J Neurol Sci 429: , 117615. |
[57] | Roos DS , Klein M , Deeg DJH , Doty RL , Berendse HW ((2022) ) Prevalence of prodromal symptoms of Parkinson’s disease in the late middle-aged population. J Parkinsons Dis 12: , 967. |
[58] | Arun MP , Bharath S , Pal PK , Singh G ((2011) ) Relationship of depression, disability, and quality of life in Parkinson’s disease: A hospital-based case-control study. Neurol India 59: , 185–189. |
[59] | Weintraub D , Mamikonyan E ((2019) ) The neuropsychiatry of Parkinson disease: A perfect storm. Am J Geriatr Psychiatry 27: , 998–1018. |
[60] | Bareeqa SB , Samar SS , Kamal S , Masood Y , Allahyar , Ahmed SI , Hayat G ((2022) ) Prodromal depression and subsequent risk of developing Parkinson’s disease: A systematic review with meta-analysis. Neurodegener Dis Manag 12: , 155–164. |
[61] | Schrag A , Horsfall L , Walters K , Noyce A , Petersen I ((2015) ) Prediagnostic presentations of Parkinson’s disease in primary care: A case-control study. Lancet Neurol 14: , 57–64. |
[62] | Seritan AL , Rienas C , Duong T , Delucchi K , Ostrem JL ((2019) ) Ages at onset of anxiety and depressive disorders in Parkinson’s disease. J Neuropsychiatry Clin Neurosci 31: , 346–352. |
[63] | Borgonovo J , Allende-Castro C , Laliena A , Guerrero N , Silva H , Concha ML ((2017) ) Changes in neural circuitry associated with depression at pre-clinical, pre-motor and early motor phases of Parkinson’s disease. Parkinsonism Relat Disord 35: , 17–24. |
[64] | Chen V , Moncalvo M , Tringali D , Tagliafierro L , Shriskanda A , Ilich E , Dong W , Kantor B , Chiba-Falek O ((2020) ) The mechanistic role of alpha-synuclein in the nucleus: Impaired nuclear function caused by familial Parkinson’s disease SNCA mutations. Hum Mol Genet 29: , 3107. |
[65] | Bitetto G , Di Fonzo A ((2020) ) Nucleo-cytoplasmic transport defects and protein aggregates in neurodegeneration. Transl Neurodegener 9: , 25. |
[66] | Champagne F ((2022) ) Differential DNA methylation and epigenetic age in postmortem brain tissue associated with depression and post-traumatic stress disorder. Biol Psychiatry 91: , S8. |
[67] | Allsopp K , Read J , Corcoran R , Kinderman P ((2019) ) Heterogeneity in psychiatric diagnostic classification. Psychiatry Res 279: , 15–22. |
[68] | Habib K , Bishayee K , Kang J , Sadra A , Huh S-O ((2022) ) RNA binding protein Rbms1 enables neuronal differentiation and radial migration during neocortical development by binding and stabilizing the RNA message for Efr3a. Mol Cells 45: , 588–602. |
[69] | Li S , Liu H , Bian S , Sha X , Li Y , Wang Y ((2020) ) The accelerated aging model reveals critical mechanisms of late-onset Parkinson’s disease. BioData Mining 13: , 4. |
[70] | Wray NR , Ripke S , Mattheisen M , Trzaskowski M , Byrne EM , Abdellaoui A , Adams MJ , Agerbo E , Air TM , Andlauer TMF , et al. ((2018) ) Genome-wide association analyses identify 44 risk variants and refine the genetic architecture of major depression. Nat Genet 50: , 668–681. |
[71] | Srivastava A , Ritesh KC , Tsan Y-C , Liao R , Su F , Cao X , Hannibal MC , Keegan CE , Chinnaiyan AM , Martin DM , Bielas SL ((2015) ) De novo dominant ASXL3 mutations alter H2A deubiquitination and transcription in Bainbridge-Ropers syndrome. Hum Mol Genet 25: , 597–608. |
[72] | Yang L , Guo B , Zhu W , Wang L , Han B , Che Y , Guo L ((2020) ) Bainbridge-ropers syndrome caused by loss-of-function variants in ASXL3: Clinical abnormalities, medical imaging features, and gene variation in infancy of case report. BMC Pediatr 20: , 287. |
[73] | Wu W , Howard D , Sibille E , French L ((2021) ) Differential and spatial expression meta-analysis of genes identified in genome-wide association studies of depression. Transl Psychiatry 11: , 8. |
[74] | Bartholdi D , Roelfsema JH , Papadia F , Breuning MH , Niedrist D , Hennekam RC , Schinzel A , Peters DJM ((2007) ) Genetic heterogeneity in Rubinstein-Taybi syndrome: Delineation of the phenotype of the first patients carrying mutations in EP300. J Med Genet 44: , 327–333. |
[75] | Menke LA , DDD study , Gardeitchik T , Hammond P , Heimdal KR , Houge G , Hufnagel SB , Ji J , Johansson S , Kant SG , Kinning E , Leon EL , Newbury-Ecob R , Paolacci S , Pfundt R , Ragge NK , Rinne T , Ruivenkamp C , Saitta SC , Sun Y , Tartaglia M , Terhal PA , van Essen AJ , Vigeland MD , Xiao B , Hennekam RC ((2018) ) Further delineation of an entity caused by CREBBP and EP300 mutations but not resembling Rubinstein-Taybi syndrome. Am J Med Genet A 176: , 862–876. |
[76] | Zhang X , Wang M , Qiao Y , Shan Z , Yang M , Li G , Xiao Y , Wei L , Bi H , Gao T ((2022) ) Exploring the mechanisms of action of for the treatment of depression using network pharmacology and molecular docking. Ann Transl Med 10: , 282. |
[77] | Gallagher CL , Bell B , Palotti M , Oh J , Christian BT , Okonkwo O , Sojkova J , Buyan-Dent L , Nickles RJ , Harding SJ , Stone CK , Johnson SC , Holden JE ((2015) ) Anterior cingulate dopamine turnover and behavior change in Parkinson’s disease. Brain Imaging Behav 9: , 821–827. |
[78] | Alexander L , Jelen LA , Mehta MA , Young AH ((2021) ) The anterior cingulate cortex as a key locus of ketamine’s antidepressant action. Neurosci Biobehav Rev 127: , 531–554. |
[79] | Tillgren V , Ho JCS , Önnerfjord P , Kalamajski S ((2015) ) The novel small leucine-rich protein chondroadherin-like (CHADL) is expressed in cartilage and modulates chondrocyte differentiation. J Biol Chem 290: , 918–925. |
[80] | Zhang F , Rao S , Baranova A ((2022) ) Shared genetic liability between major depressive disorder and osteoarthritis. Bone Joint Res 11: , 12. |
[81] | Bahrami S , Hindley G , Winsvold BS , O’Connell KS , Frei O , Shadrin A , Cheng W , Bettella F , Rødevand L , Odegaard KJ , Fan CC , Pirinen MJ , Hautakangas HM , HUNTAll-In Headache , Dale AM , Djurovic S , Smeland OB , Andreassen OA ((2022) ) Dissecting the shared genetic basis of migraine and mental disorders using novel statistical tools. Brain 145: , 142–153. |
[82] | Lange J , Wood-Kaczmar A , Ali A , Farag S , Ghosh R , Parker J , Casey C , Uno Y , Kunugi A , Ferretti P , Andre R , Tabrizi SJ ((2021) ) Mislocalization of nucleocytoplasmic transport proteins in human Huntington’s disease PSC-derived striatal neurons. Front Cell Neurosci 15: , 742763. |
[83] | Baird DA , Liu JZ , Zheng J , Sieberts SK , Perumal T , Elsworth B , Richardson TG , Chen C-Y , Carrasquillo MM , Allen M , Reddy JS , De Jager PL , Ertekin-Taner N , Mangravite LM , Logsdon B , Estrada K , Haycock PC , Hemani G , Runz H , Smith GD , Gaunt TR ,AMP-AD eQTL working group (2021) Identifying drug targets for neurological and psychiatric disease via genetics and the brain transcriptome. PLoS Genet 17: , e1009224. |
[84] | Vitour D , Lindenbaum P , Vende P , Becker MM , Poncet D ((2004) ) RoXaN, a novel cellular protein containing TPR, LD, and zinc finger motifs, forms a ternary complex with eukaryotic initiation factor 4G and rotavirus NSP3. J Virol 78: , 3851–3862. |
[85] | Takahashi JS ((2017) ) Transcriptional architecture of the mammalian circadian clock. Nat Rev Genet 18: , 164. |
[86] | Menza M , Dobkin RD , Marin H , Bienfait K ((2010) ) Sleep Disturbances in Parkinson’s Disease .Mov Disord 25: , S117. |