Diabetes and Parkinson’s Disease: Understanding Shared Molecular Mechanisms
Abstract
Aging is a major risk factor for Parkinson’s disease (PD). Genetic mutations account for a small percentage of cases and the majority appears to be sporadic, with yet unclear causes. However, various environmental factors have been linked to an increased risk of developing PD and, therefore, understanding the complex interplay between genetic and environmental factors is crucial for developing effective disease-modifying therapies. Several studies identified a connection between type 2 diabetes (T2DM) and PD. T2DM is characterized by insulin resistance and failure of β-cells to compensate, leading to hyperglycemia and serious comorbidities. Both PD and T2DM share misregulated processes, including mitochondrial dysfunction, oxidative stress, chronic inflammation, altered proteostasis, protein aggregation, and misregulation of glucose metabolism. Chronic or recurring hyperglycemia is a T2DM hallmark and can lead to increased methylglyoxal (MGO) production, which is responsible for protein glycation. Glycation of alpha-synuclein (aSyn), a central player in PD pathogenesis, accelerates the deleterious aSyn effects. Interestingly, MGO blood plasma levels and aSyn glycation are significantly elevated in T2DM patients, suggesting a molecular mechanism underlying the T2DM - PD link. Compared to high constant glucose levels, glycemic variability (fluctuations in blood glucose levels), can be more detrimental for diabetic patients, causing oxidative stress, inflammation, and endothelial damage. Accordingly, it is imperative for future research to prioritize the exploration of glucose variability’s influence on PD development and progression. This involves moving beyond the binary classification of patients as diabetic or non-diabetic, aiming to pave the way for the development of enhanced therapeutic interventions.
INTRODUCTION
Aging is the major known risk factor for Parkinson’s disease (PD) and, due to a generalized increase in lifespan, the prevalence of PD is drastically increasing [1]. Only 5–10% of PD cases are linked to mutations, in less than 10 genes [2]. These cases have the typical Mendelian inheritance pattern and are usually referred to as genetic PD. However, the majority of cases is sporadic and little is known about the interplay between environmental and genetic factors that trigger, facilitate and aggravate PD pathogenesis [3, 4]. A number of risk factors including rural living, farming, head injury, constipation and well-water consumption have been correlated with an increased risk to develop PD [5–7]. Therefore, deciphering the complex architecture of genetic and environmental factors that increase the risk for PD development is essential in order to enable the development of effective disease-modifying therapies.
TYPE 2 DIABETES MELLITUS: AN ESTABLISHED RISK FACTOR FOR PD
Diabetes is a widespread and highly heterogenous group of metabolic disorders. The most prominent types of diabetes are type 1 (T1DM) and type 2 diabetes (T2DM). T2DM is characterized by long-term insulin resistance and/or insulin action and the failure of β-cells to compensate for this. It constitutes 90–95% of all DM cases [8]. In contrast, T1DM is an autoimmune disease that involves the destruction of insulin-producing pancreatic β-cells. Hyperglycemia is a characteristic feature of both T1DM and T2DM. Strikingly, due to the tremendous impact of metabolic alterations at an organismal level, diabetes is associated with several comorbidities including retinopathy, neuropathy, hypertension and vascular complications [9–12].
Epidemiological studies recently provided unequivocal evidence for a connection between diabetes and PD. In fact, several prospective and retrospective studies have assessed the prevalence of T2DM in PD patients, the risk of developing PD in T2DM patients, and the impact of T2DM on PD severity and progression [13–20].
Nevertheless, a connection between T2DM and PD has been debated for several years. Interestingly, case-control studies showed no increased risk for developing PD in diabetic individuals [21]. However, case-control studies are more typically suffering from selection- and survival bias as well as reverse causality. In contrast, meta-analyses reported a 27–38% increase in risk of PD among diabetic patients and a 4% increase for prediabetic patients [21–25]. The odds ratio for T2DM patients to also develop PD is highest in studies involving older participants, suggesting also an age association [26]. In addition, clinical studies reveal that PD patients with comorbid T2DM frequently experience more severe forms of PD after T2DM onset [22, 27–29]. However, whether this association truly represents a causal link is still matter of debate.
WHAT ABOUT TYPE 1 DIABETES?
Since T1DM is far less frequent when compared to T2DM, data on the association between T1DM and PD are much sparser, and still controversial. For example, a GWAS study revealed an association between type 1 diabetes and PD [30]. Two epidemiological studies in Denmark and Sweden found no increased risk for PD in T1DM patients [31, 32]. In contrast, a study using data of 1.8 million individuals in Austria confirmed the relation between T1DM and PD (2.3, CI 1.9–2.7 for DM1) [33]. This suggests additional studies will be necessary to clarify a putative connection between T1DM and PD.
IMBALANCED FASTING GLUCOSE LEVELS IN DIABETES
Lifestyle and genetic susceptibility affect onset and pathology of both T2DM and PD. Strikingly, accumulating evidence suggests that the two diseases share pathological mechanisms and influence the onset and progression of each other.
Based on our understanding of various disease processes, and frequent comorbidities, it is clear that we need to engage multidisciplinary teams of physicians and healthcare providers in order to treat different conditions. This is particularly true in the context of PD and T2DM. Importantly, we propose to not simply use the binary discrimination diabetic/non-diabetic, as this may compromise our understanding of the underlying molecular mechanisms involved in T2DM and PD.
Traditionally, T2DM or pre-T2DM is diagnosed based on either glycated hemoglobin (HbA1c) or single-time-point measurements of blood glucose concentration. Glycated HbA1c levels allow us to evaluate the glycemic control of the previous 3 months. One-time measurements of capillary blood glucose levels provide information on the levels at the time of measurement. Importantly, advances in continuous glucose monitoring devices in the last years enable continuous measurements of blood glucose levels and trends, providing insight into an individual’s glycemic variability, i.e., swings in blood glucose levels throughout the day, with both hypoglycemic episodes and also peaks of high glucose levels, in the case of certain meals [34]. While a certain degree of glycemic variability is a normal physiological phenomenon, glycemic variability seems to be increased in prediabetic and diabetic individuals [35]. It is well established now that the blood sugar levels of many people are more dynamic and show higher peaks than expected. A study with 80 adults without diabetes diagnosis used continuous blood glucose monitoring and found that 9% of individuals experienced daily episodes with blood glucose levels in the diabetic range [36]. Another study characterized glucose fluctuations and distinguishes three types of “glucotype” with increasing variability (low, moderate, severe). Interestingly, 25% of normoglycemic individuals experienced severe glycemic variability with prediabetic and diabetic glucose levels [37].
GLYCEMIC VARIABILITY CAN HAVE MORE DELETERIOUS EFFECTS THAN CONSTANT HIGH GLUCOSE LEVELS
Apart from the harmful consequences of hyperglycemia for diabetic patients, it has been known for several years that strong fluctuations in glucose levels present an additional burden (see model in Fig. 2). Fluctuations in glucose over a period of 24 hours causes more endothelial damage than higher constant glucose levels [38]. Compared to constantly high glucose levels, glycemic variability leads to more oxidative stress, inflammation and apoptosis, and is linked to the development and severity of diabetic complications [39–41]. HbA1c levels do not provide information about glucose oscillations and individuals with very similar HbA1c can have significantly different diurnal glucose variation.
HYPERGLYCEMIA AND GLYCATION: ONE POSSIBLE CONNECTION BETWEEN PD AND DIABETES
Given the clinical associations between DM and PD, it is important to determine the molecular mechanisms underlying this link. Both PD and DM are chronic disorders that are mainly experienced by people in the second part of their lives. Furthermore, both conditions are of multifactorial origin, with a variety of genetic variants and environmental factors acting in combination. In addition, PD and DM share several misregulated processes and pathways, including mitochondrial dysfunction, oxidative stress, chronic inflammation, altered proteostasis, protein aggregation, and misregulation of glucose metabolism [42]. Interestingly, some mouse lines in use as DM models show pathological characteristics that resemble early PD features [43], and antidiabetic drugs are now being tested in PD [44].
Insulin, secreted by the pancreatic β cells, activates insulin receptor substrates via insulin receptors (IRs), and acts to maintain blood glucose levels stable. Since insulin does not increase cellular glucose uptake in the brain, the brain was long assumed to be glucose-insensitive. However, more recent studies revealed that insulin plays an important role in neuronal survival and brain function, and that several insulin receptors are expressed in various brain regions [45, 46]. Insulin resistance is a pathological condition in which cells fail to properly sense and respond to insulin. While insulin resistance is a central problem in DM, it has also been described in the brains of PD patients [47].
Both T2DM and PD are associated with the accumulation of misfolded proteins that form amyloid aggregates. The majority of DM patients display amyloids of “islet amyloid polypeptide” (IAPP) in the insulin-secreting pancreatic cells, causing cellular dysfunctions and ultimately death [48, 49]. In vitro experiments suggest that aSyn and IAPP can cross-seed each other aggregation and amyloid formation [50].
Mitochondrial dysfunction is an early event in PD pathogenesis, and a variety of familial PD-related genes are involved in mitochondrial function [51]. In addition, many PD-causing toxins, including those that are currently used to model parkinsonism in the laboratory, act on mitochondria [52].
While many pathological pathways have been connected to connect PD and DM, chronic or recurring hyperglycemia is a central hallmark of T2DM and is thought to account for most of the T2DM associated complications. Neurons are particularly sensitive to high glucose levels and hyperglycemia leads to a variety of direct neurotoxic effects [53]. In addition to its direct effects, higher glucose levels also lead to increased production of reactive oxoaldehydes including methylglyoxal (MGO), a glycolytic by-product that forms primarily from glyceraldehyde-3-phosphate and dihydroxyacetone phosphate (see Fig. 1) [54]. MGO is a dicarbonyl that reacts with lysine, arginine, the proteins N-terminus and thiol groups of cysteines in a series of chemical reactions known as glycation. While a plethora of sugars and metabolites resulting from sugar metabolism can cause glycation in physiological contexts, MGO is the most reactive molecule [55, 56]. In physiological glucose concentrations, cells are protected from MGO by the glyoxalase system. Glyoxalase 1 and 2 react with MGO in the presence of reduced glutathione, forming lactate [57]. Interestingly, MGO plasma levels are significantly elevated in T2DM patients [58]. Consistently, patients with diabetic nephropathy, a major diabetic complication, have twice as high MGO plasma levels as healthy controls [59–62].
Fig. 1
MGO is formed as a side-product of glycolysis. The spontaneous degradation of glyceraldehyde-3-phosphate (GA3P) and dihydroxyacetonephosphate (DHAP) generates MGO. The glyoxalase system, a set of enzymes, is responsible for detoxification of MGO, forming lactate.
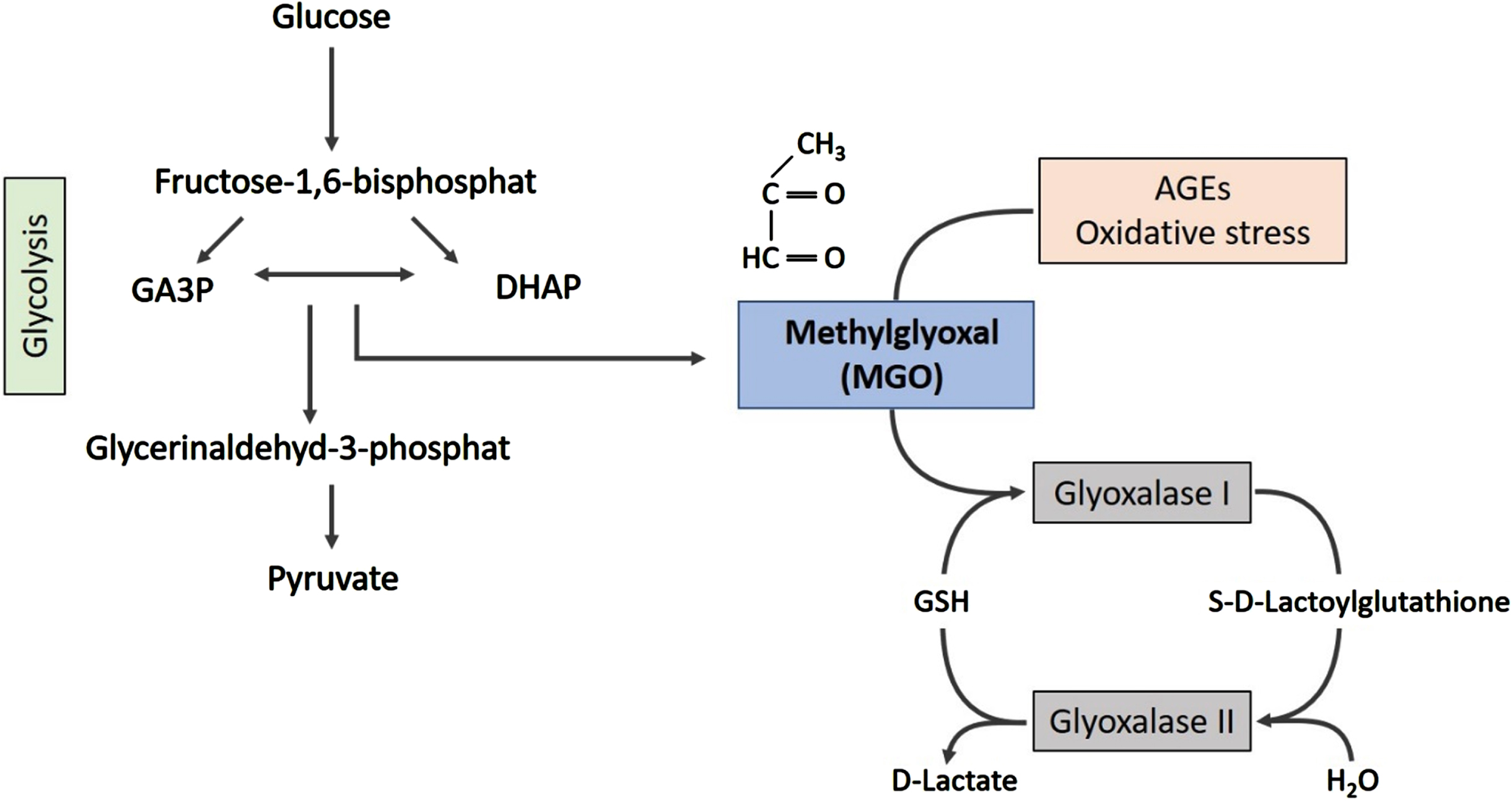
Fig. 2
Oscillations of glucose levels and diabetic complications. Strong oscillations of glucose levels—glycemic variability—throughout the day contribute to diabetic complications, and possibly increase susceptibility to PD.
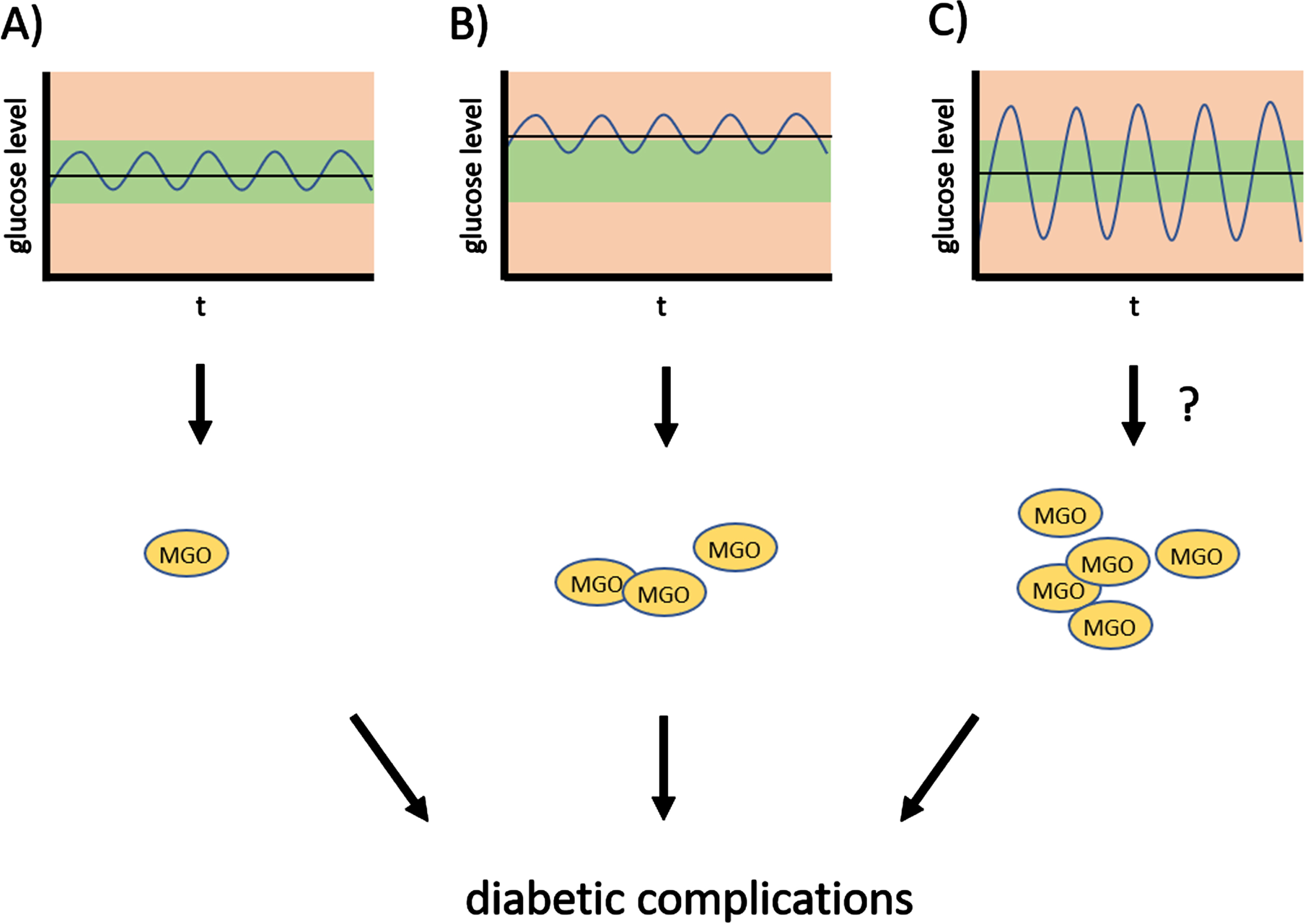
On average, MGO levels are elevated in diabetic individuals, and several studies suggest that MGO levels may discriminate patients with and without certain diabetes complications. Even though precise measurements of MGO are challenging, [63] diabetes-affected individuals with pain in the foot have higher MGO levels, compared to either healthy controls or diabetes-affected individuals with no pain [64]. Higher MGO levels correlate with cardiovascular damage in T1DM and T2DM patients [65]. Likewise, higher MGO levels correlate with diabetic polyneuropathy and nephropathy, both frequent T2DM complications [61, 66]. To date, no consensus on how glycemic variability should be measured and classified has been reached [67]. To our knowledge, so far only one study reported that increased long-term variability of fasting plasma glucose is associated with an increased risk to develop PD in non-diabetic individuals [68].
Accumulating evidence suggests now that glycation provides a molecular link between PD and T2DM [69]. Lewy bodies, the pathognomonic proteinaceous inclusions found in most post mortem PD brains, show elevated levels of AGEs [70]. In addition, it was shown that AGE levels are elevated in several brain regions of cases with PD-related changes [71]. Using an in vitro mouse model we showed that MGO can glycate alpha-synuclein (aSyn) in the brain [72]. Glycation leads to reduced membrane binding and clearance through a reduction in αSN ubiquitination and SUMOylation. Glycation of aSyn may lead to the loss of function of glyceraldehyde 3-phosphate dehydrogenase, an enzyme involved in glycolysis and implicated in neurodegenerative disorders [73]. Numerous studies have demonstrated that glycation of aSyn has significant impacts on the aggregation behavior of aSyn and reduces fibril formation [74–77]. Additionally, glycated aSyn shows minimal incorporation into fibrils [78].
Interestingly, glycation accelerates the formation of toxic aSyn oligomers, as observed in cell and animal models [74, 78]. Since aSyn is a long-lived protein, rich in lysine residues (15 residues), it is prone to glycation in various sites. Interestingly, we found that the aSyn N-Terminus is particularly susceptible to glycation [74]. Importantly, DJ-1, a protein with a proposed deglycase activity [79], is associated with a recessive form of PD, suggesting a loss of function might be at play, but additional studies will be necessary to establish this connection.
Thus, for all of the above, we posit that hyperglycemia-induced glycation is one of several mechanisms that may contribute to the increased risk of PD in DM patients.
DIABETES AND PD: CONNECTED BY TIME
The suggested effects induced by glycemic variability are generally all of relatively low intensity. However, it is important to note that PD is a progressive condition with a long pre-motor phase, with manifestations that tend not to be specific for PD. The pre-motor phase can last for several years, or even decades and, during this phase, dopaminergic cell loss is likely to progress until the midbrain circuits are no longer able to function properly, leading to the typical cardinal motor features of PD. Strikingly, it is estimated that, by the time a patient experiences the characteristic motor symptoms, 40% –60% of neurons in the substantia nigra have already been lost [80, 81]. Consequently, efficiently targeting neurodegeneration in the pre-motor phase is a major goal of ongoing research. Thus, although the pathological mechanisms associated with aSyn glycation may, alone, have only mild detrimental effects, we need to consider these can accumulate over several years and decades, thereby tipping the proteostasis network and, thereby, contribute to the pathology.
CONCLUSIONS
The frequency and severity of glycemic variation in healthy, pre-diabetic, and diabetic individuals argues for studies that not only distinguish patients based on mean glucose levels and/or diabetes diagnosis but, instead, relate blood glucose variability or glucotypes to PD. This research will advance the understanding of lifestyle-related factors that contribute to the development of PD, and how we might be able to intervene to reduce the risk. One challenge in PD care is that the typical motor features and, consequently, diagnosis, usually appear many years or even decades after disease onset. Therefore, novel approaches, including those using artificial intelligence to integrate different modalities of datasets that capture the biological complexity of PD, will enable the combined assessment of risk factors, including glucotypes, thereby facilitating the identification of individuals at high risk for PD development and providing strategies to slow down disease progression [82, 83].
ACKNOWLEDGMENTS
The authors have no acknowledgments to report.
FUNDING
T.F.O. is supported by the Deutsche Forschungsgemeinschaft (DFG, German Research Foundation) under Germany’s Excellence Strategy - EXC 2067/1- 390729940, by SFB1286 (B8). T. F. O. is supported by BMBF through the EU Joint Programme on Neurodegenerative Disease Research (JPND, www.jpnd.edu) project (OligoFit).
CONFLICT OF INTEREST
T.F.O. is an Editorial Board Member of this journal, but was not involved in the peer-review process nor had access to any information regarding its peer-review.
REFERENCES
[1] | Hou Y , Dan X , Babbar M , Wei Y , Hasselbalch SG , Croteau DL , Bohr VA ((2019) ) Ageing as a risk factor for neurodegenerative disease. Nat Rev Neurol 15: , 565–581. |
[2] | Hernandez DG , Reed X , Singleton AB ((2016) ) Genetics in Parkinson disease: Mendelian versus non-Mendelian inheritance. J Neurochem 139 Suppl 1: , 59–74. |
[3] | Bellou V , Belbasis L , Tzoulaki I , Evangelou E , Ioannidis JPA ((2016) ) Environmental risk factors and Parkinson’s disease: An umbrella review of meta-analyses. Parkinsonism Relat Disord 23: , 1–9. |
[4] | Johnson ME , Stecher B , Labrie V , Brundin L , Brundin P ((2019) ) Triggers, facilitators, and aggravators: Redefining Parkinson’s disease pathogenesis. Trends Neurosci 42: , 4–13. |
[5] | Fleming SM ((2017) ) Mechanisms of gene-environment interactions in Parkinson’s disease. Curr Environ Health Rep 4: , 192–199. |
[6] | Schaefers ATU , Teuchert-Noodt G ((2016) ) Developmental neuroplasticity and the origin of neurodegenerative diseases. World J Biol Psychiatry 17: , 587–599. |
[7] | Tanner CM , Kame F , Ross GW , Hoppin JA , Goldman SM , Korell M , Marras C , Bhudhikanok GS , Kasten M , Chade AR , Comyns K , Richards MB , Meng C , Priestley B , Fernandez HH , Cambi F , Umbach DM , Blair A , Sandler DP , Langston JW ((2011) ) Rotenone, paraquat, and Parkinson’s disease. Environ Health Perspect 119: , 866–872. |
[8] | ((2019) ) 2. Classification and diagnosis of diabetes: Standards of medical care in diabetes-2019. Diabetes Care 42: , S13–S28. |
[9] | Cole JB , Florez JC ((2020) ) Genetics of diabetes mellitus and diabetes complications. Nat Rev Nephrol 16: , 377–390. |
[10] | Gregg EW , Sattar N , Ali MK ((2016) ) The changing face of diabetes complications. Lancet Diabetes Endocrinol 4: , 537–547. |
[11] | Lotfy M , Adeghate J , Kalasz H , Singh J , Adeghate E ((2017) ) Chronic complications of diabetes mellitus: A mini review. Curr Diabetes Rev 13: , 3–10. |
[12] | Shah AD , Langenberg C , Rapsomaniki E , Denaxas S , Pujades-Rodriguez M , Gale CP , Deanfield J , Smeeth L , Timmis A , Hemingway H ((2015) ) Type 2 diabetes and incidence of cardiovascular diseases: A cohort study in 1·9 million people. Lancet Diabetes Endocrinol 3: , 105–113. |
[13] | Cereda E , Barichella M , Cassani E , Caccialanza R , Pezzoli G ((2012) ) Clinical features of Parkinson disease when onset of diabetes came first: A case-control study. Neurology 78: , 1507–1511. |
[14] | De Pablo-Fernandez E , Sierra-Hidalgo F , Benito-León J , Bermejo-Pareja F ((2017) ) Association between Parkinson’s disease anddiabetes: Data from NEDICES study. Acta Neurol Scand 136: , 732–736. |
[15] | Driver JA , Smith A , Buring JE , Gaziano JM , Kurth T , Logroscino G ((2008) ) Prospective cohort study of type 2 diabetes and the risk of Parkinson’s disease. Diabetes Care 31: , 2003–2005. |
[16] | Hu G , Jousilahti P , Bidel S , Antikainen R , Tuomilehto J ((2007) ) Type 2 diabetes and the risk of Parkinson’s disease. Diabetes Care 30: , 842–7. |
[17] | Schernhammer E , Hansen J , Rugbjerg K , Wermuth L , Ritz B ((2011) ) Diabetes and the risk of developing Parkinson’s disease in Denmark. Diabetes Care 34: , 1102–1108. |
[18] | Sun Y , Chang Y-H , Chen H-F , Su Y-H , Su H-F , Li C-Y ((2012) ) Risk of Parkinson disease onset in patients with diabetes: A 9-year population-based cohort study with age and sex stratifications. Diabetes Care 35: , 1047–1049. |
[19] | Xu Q , Park Y , Huang X , Hollenbeck A , Blair A , Schatzkin A , Chen H ((2011) ) Diabetes and risk of Parkinson’s disease. Diabetes Care 34: , 910–915. |
[20] | Yang Y-W , Hsieh T-F , Li C-I , Liu C-S , Lin W-Y , Chiang J-H , Li T-C , Lin C-C ((2017) ) Increased risk of Parkinson disease with diabetes mellitus in a population-based study. Medicine (Baltimore) 96: , e5921. |
[21] | Cereda E , Barichella M , Pedrolli C , Klersy C , Cassani E , Caccialanza R , Pezzoli G ((2011) ) Diabetes and risk of Parkinson’s disease: A systematic review and meta-analysis. Diabetes Care 34: , 2614–2623. |
[22] | Yue X , Li H , Yan H , Zhang P , Chang L , Li T ((2016) ) Risk of Parkinson disease in diabetes mellitus: An updated meta-analysis of population-based cohort studies. Medicine (Baltimore) 95: , e3549. |
[23] | Liu W , Tang J ((2021) ) Association between diabetes mellitus and risk of Parkinson’s disease: A prisma-compliant meta-analysis. Brain Behav 11: , e02082. |
[24] | Chohan H , Senkevich K , Patel RK , Bestwick JP , Jacobs BM , Bandres Ciga S , Gan-Or Z , Noyce AJ ((2021) ) Type 2 diabetes as a determinant of Parkinson’s disease risk and progression. Mov Disord 36: , 1420–1429. |
[25] | Aune D , Schlesinger S , Mahamat-Saleh Y , Zheng B , Udeh-Momoh CT , Middleton LT ((2023) ) Diabetes mellitus, prediabetes and the risk of Parkinson’s disease: A systematic review and meta-analysis of 15 cohort studies with 29.9 million participants and 86,345 cases. Eur J Epidemiol 38: , 591–604. |
[26] | Cheong JLY , de Pablo-Fernandez E , Foltynie T , Noyce AJ ((2020) ) The association between type 2 diabetes mellitus and Parkinson’s disease. J Parkinsons Dis 10: , 775–789. |
[27] | Biosa A , Outeiro TF , Bubacco L , Bisaglia M ((2018) ) Diabetes mellitus as a risk factor for Parkinson’s disease: A molecular point of view. Mol Neurobiol 55: , 8754–8763. |
[28] | Camargo Maluf F , Feder D , Alves De Siqueira Carvalho A ((2019) ) Analysis of the relationship between type II diabetes mellitus and Parkinson’s disease: A systematic review. Parkinsons Dis 2019: , 4951379. |
[29] | Komici K , Femminella GD , Bencivenga L , Rengo G , Pagano G ((2021) ) Diabetes mellitus and Parkinson’s disease: A systematic review and meta-analyses. J Parkinsons Dis 11: , 1585–1596. |
[30] | Witoelar A , Jansen IE , Wang Y , Desikan RS , Gibbs JR , Blauwendraat C , Thompson WK , Hernandez DG , Djurovic S , Schork AJ , Bettella F , Ellinghaus D , Franke A , Lie BA , McEvoy LK , Karlsen TH , Lesage S , Morris HR , Brice A , Wood NW , Heutink P , Hardy J , Singleton AB , Dale AM , Gasser T , Andreassen OA , Sharma M , International Parkinson’s Disease Genomics Consortium (IPDGC), North American Brain Expression Consortium (NABEC), and United Kingdom Brain Expression Consortium (UKBEC) Investigators ((2017) ) Genome-wide pleiotropy between Parkinson disease and autoimmune diseases. JAMA Neurol 74: , 780–792. |
[31] | Li X , Sundquist J , Sundquist K ((2012) ) Subsequent risks of Parkinson disease in patients with autoimmune and related disorders: A nationwide epidemiological study from Sweden. Neurodegener Dis 10: , 277–284. |
[32] | Rugbjerg K , Friis S , Ritz B , Schernhammer ES , Korbo L , Olsen JH ((2009) ) Autoimmune disease and risk for Parkinson disease: A population-based case-control study. Neurology 73: , 1462–1468. |
[33] | Klimek P , Kautzky-Willer A , Chmiel A , Schiller-Frühwirth I , Thurner S ((2015) ) Quantification of diabetes comorbidity risks across life using nation-wide big claims data. PLoS Comput Biol 11: , e1004125. |
[34] | Malandrucco I , Russo B , Picconi F , Menduni M , Frontoni S ((2020) ) Glycemic status assessment by the latest glucose monitoring technologies. Int J Mol Sci 21: , 8243. |
[35] | Wang C , Lv L , Yang Y , Chen D , Liu G , Chen L , Song Y , He L , Li X , Tian H , Jia W , Ran X ((2012) ) Glucose fluctuations in subjects with normal glucose tolerance, impaired glucose regulation and newly diagnosed type 2 diabetes mellitus. Clin Endocrinol (Oxf) 76: , 810–815. |
[36] | Borg R , Kuenen JC , Carstensen B , Zheng H , Nathan DM , Heine RJ , Nerup J , Borch-Johnsen K , Witte DR ((2010) ) Real-life glycaemic profiles in non-diabetic individuals with low fasting glucose and normal HbA1c: The A1C-Derived Average Glucose (ADAG) study. Diabetologia 53: , 1608–1611. |
[37] | Hall H , Perelman D , Breschi A , Limcaoco P , Kellogg R , McLaughlin T , Snyder M ((2018) ) Glucotypes reveal new patterns of glucose dysregulation. PLoS Biol 16: , e2005143. |
[38] | Ceriello A , Esposito K , Piconi L , Ihnat MA , Thorpe JE , Testa R , Boemi M , Giugliano D ((2008) ) Oscillating glucose is more deleterious to endothelial function and oxidative stress than mean glucose in normal and type 2 diabetic patients. Diabetes 57: , 1349–1354. |
[39] | Alfieri V , Myasoedova VA , Vinci MC , Rondinelli M , Songia P , Massaiu I , Cosentino N , Moschetta D , Valerio V , Ciccarelli M , Marenzi G , Genovese S , Poggio P ((2021) ) The role of glycemic variability in cardiovascular disorders. Int J Mol Sci 22: , 8393. |
[40] | Jung HS ((2015) ) Clinical implications of glucose variability: Chronic complications of diabetes. Endocrinol Metab (Seoul) 30: , 167–174. |
[41] | Watt C , Sanchez-Rangel E , Hwang JJ ((2020) ) Glycemic variability and CNS inflammation: Reviewing the connection. Nutrients 12: , 3906. |
[42] | Yu H , Sun T , He X , Wang Z , Zhao K , An J , Wen L , Li JY , Li W , Feng J ((2022) ) Association between Parkinson’s disease and diabetes mellitus: From epidemiology, pathophysiology and prevention to treatment. Aging Dis 13: , 1591. |
[43] | de Bem AF , Krolow R , Farias HR , de Rezende VL , Gelain DP , Moreira JCF , Duarte JM das N , de Oliveira J ((2021) ) Animal models of metabolic disorders in the study of neurodegenerative diseases: An overview. Front Neurosci 14: , 604150. |
[44] | König A , Vicente Miranda H , Outeiro TF ((2018) ) Alpha-synucleinglycation and the action of anti-diabetic agents in Parkinson’s disease. J Parkinsons Dis 8: , 33–43. |
[45] | Sabari SS , Balasubramani K , Iyer M , Sureshbabu HW , Venkatesan D , Gopalakrishnan AV , Narayanaswamy A , Senthil Kumar N , Vellingiri B ((2023) ) Type 2 diabetes (T2DM) and Parkinson’s disease (PD): A mechanistic approach. Mol Neurobiol 60: , 4547–4573. |
[46] | Chiu SL , Cline HT ((2010) ) Insulin receptor signaling in the development of neuronal structure and function. Neural Dev 5: , 7. |
[47] | Morris JK , Vidoni ED , Perea RD , Rada R , Johnson DK , Lyons K , Pahwa R , Burns JM , Honea RA ((2014) ) Insulin resistance and gray matter volume in neurodegenerative disease. Neuroscience 270: , 139–147. |
[48] | Jaikaran ETAS , Nilsson MR , Clark A ((2004) ) Pancreatic beta-cell granule peptides form heteromolecular complexes which inhibit islet amyloid polypeptide fibril formation. Biochem J 377: , 709–716. |
[49] | Wang Y , Bergström J , Ingelsson M , Westermark GT ((2023) ) Studieson alpha-synuclein and islet amyloid polypeptide interaction. Front Mol Biosci 10: , 1080112. |
[50] | Pagano G , Polychronis S , Wilson H , Giordano B , Ferrara N , Niccolini F , Politis M ((2018) ) Diabetes mellitus and Parkinson disease. Neurology 90: , e1654–e1662. |
[51] | Larsen SB , Hanss Z , Krüger R ((2018) ) The genetic architecture of mitochondrial dysfunction in Parkinson’s disease. Cell Tissue Res 373: , 21–37. |
[52] | Prasad EM , Hung SY ((2020) ) Behavioral tests in neurotoxin-induced animal models of Parkinson’s disease. Antioxidants (Basel) 9: , 1007. |
[53] | Tomlinson DR , Gardiner NJ ((2008) ) Glucose neurotoxicity. Nat Rev Neurosci 9: , 36–45. |
[54] | Kalapos MP ((1999) ) Methylglyoxal in living organisms - Chemistry, biochemistry, toxicology and biological implications. Toxicol Lett 110: , 145–175. |
[55] | Lo TWC , Westwood ME , McLellan AC , Selwood T , Thornalley PJ ((1994) ) Binding and modification of proteins by methylglyoxal under physiological conditions. J Biol Chem 269: , 32299–32305. |
[56] | Thornalley PJ ((2005) ) Dicarbonyl intermediates in the maillard reaction. Ann N Y Acad Sci 1043: , 111–117. |
[57] | Allaman I , Bélanger M , Magistretti PJ ((2015) ) Methylglyoxal, the dark side of glycolysis. Front Neurosci 9: , 23. |
[58] | Reyaz A , Alam S , Chandra K , Kohli S , Agarwal S ((2020) ) Methylglyoxal and soluble RAGE in type 2 diabetes mellitus: Association with oxidative stress. J Diabetes Metab Disord 19: , 515–521. |
[59] | Beisswenger PJ ((2014) ) Methylglyoxal in diabetes: Link to treatment, glycaemic control and biomarkers of complications. Biochem Soc Trans 42: , 450–456. |
[60] | Beisswenger PJ , Howell SK , Touchette AD , Lal S , Szwergold BS ((1999) ) Metformin reduces systemic methylglyoxal levels in type 2 diabetes. Diabetes 48: , 198–202. |
[61] | Lu J , Randell E , Han YC , Adeli K , Krahn J , Meng QH ((2011) ) Increased plasma methylglyoxal level, inflammation, and vascular endothelial dysfunction in diabetic nephropathy. Clin Biochem 44: , 307–311. |
[62] | McLellan AC , Thornalley PJ , Benn J , Sonksen PH ((1994) ) Glyoxalase system in clinical diabetes mellitus and correlation with diabetic complications. Clin Sci (Lond) 87: , 21–29. |
[63] | Rabbani N , Thornalley PJ ((2014) ) Measurement of methylglyoxal by stable isotopic dilution analysis LC-MS/MS with corroborative prediction in physiological samples. Nat Protoc 9: , 1969–1979. |
[64] | Bierhaus A , Fleming T , Stoyanov S , Leffler A , Babes A , Neacsu C , Sauer SK , Eberhardt M , Schnölzer M , Lasischka F , Neuhuber WL , Kichko TI , Konrade I , Elvert R , Mier W , Pirags V , Lukic IK , Morcos M , Dehmer T , Rabbani N , Thornalley PJ , Edelstein D , Nau C , Forbes J , Humpert PM , Schwaninger M , Ziegler D , Stern DM , Cooper ME , Haberkorn U , Brownlee M , Reeh PW , Nawroth PP ((2012) ) Methylglyoxal modificationof Nav1.8 facilitates nociceptive neuron firing and causeshyperalgesia in diabetic neuropathy. Nat Med 18: , 926–933. |
[65] | Kilhovd BK , Giardino I , Torjesen PA , Birkeland KI , Berg TJ , Thornalley PJ , Brownlee M , Hanssen KF ((2003) ) Increased serum levels of the specific AGE-compound methylglyoxal-derived hydroimidazolone in patients with type 2 diabetes. Metabolism 52: , 163–167. |
[66] | Andersen ST , Witte DR , Dalsgaard EM , Andersen H , Nawroth P , Fleming T , Jensen TM , Finnerup NB , Jensen TS , Lauritzen T , Feldman EL , Callaghan BC , Charles M ((2018) ) Risk factors for incident diabetic polyneuropathy in a cohort with screen-detected type 2 diabetes followed for 13 years: ADDITION-Denmark. Diabetes Care 41: , 1068–1075. |
[67] | Suh S , Kim JH ((2015) ) Glycemic variability: How do we measure it and why is it important? Diabetes Metab J 39: , 273–282. |
[68] | Chung HS , Lee JS , Kim JA , Roh E , Lee YB , Hong SH , Yu JH , Kim NH , Yoo HJ , Seo JA , Kim SG , Baik SH , Choi KM ((2021) ) Fasting plasma glucose variability in midlife and risk of Parkinson’s disease: A nationwide population-based study. Diabetes Metab 47: , 101195. |
[69] | Konig A , Miranda HV , Outeiro TF ((2018) ) Alpha-synuclein glycation and the action of anti-diabetic agents in Parkinson’s disease. J Parkinsons Dis 8: , 33–43. |
[70] | Castellani R , Smith MA , Richey PL , Perry G ((1996) ) Glycoxidation and oxidative stress in Parkinson disease and diffuse Lewy body disease. Brain Res 737: , 195–200. |
[71] | Dalfó E , Portero-Otín M , Ayala V , Martínez A , Pamplona R , Ferrer I ((2005) ) Evidence of oxidative stress in the neocortex inincidental Lewy body disease. J Neuropathol Exp Neurol 64: , 816–30. |
[72] | Miranda HV , Cássio R , Correia-Guedes L , Gomes MA , Chegão A , Miranda E , Soares T , Coelho M , Rosa MM , Ferreira JJ , Outeiro TF ((2017) ) Posttranslational modifications of blood-derived alpha-synuclein as biochemical markers for Parkinson’s disease. Sci Rep 7: , 13713. |
[73] | Semenyuk P , Barinova K , Muronetz V ((2019) ) Glycation of α-synuclein amplifies the binding withglyceraldehyde-3-phosphate dehydrogenase. Int J Biol Macromol 127: , 278–285. |
[74] | Vicente Miranda H , Szegö ÉM , Oliveira LMA , Breda C , Darendelioglu E , de Oliveira RM , Ferreira DG , Gomes MA , Rott R , Oliveira M , Munari F , Enguita FJ , Simões T , Rodrigues EF , Heinrich M , Martins IC , Zamolo I , Riess O , Cordeiro C , Ponces-Freire A , Lashuel HA , Santos NC , Lopes LV , Xiang W , Jovin TM , Penque D , Engelender S , Zweckstetter M , Klucken J , Giorgini F , Quintas A , Outeiro TF ((2017) ) Glycation potentiates α-synuclein-associated neurodegeneration in synucleinopathies. Brain 140: , 1399–1419. |
[75] | Lee D , Park CW , Paik SR , Choi KY ((2009) ) The modification of α-synuclein by dicarbonyl compounds inhibits its fibril-forming process. Biochim Biophys Acta 1794: , 421–430. |
[76] | Barinova K , Serebryakova M , Sheval E , Schmalhausen E , Muronetz V ((2019) ) Modification by glyceraldehyde-3-phosphate prevents amyloid transformation of alpha-synuclein. Biochim Biophys Acta Proteins Proteom 1867: , 396–404. |
[77] | Shaikh S , Nicholson LFB ((2008) ) Advanced glycation end products induce} cross-linking of alpha-synuclein and accelerate the process of intracellular inclusion body formation. J Neurosci Res 86: , 2071–2082. |
[78] | Farzadfard A , König A , Petersen SV , Nielsen J , Vasili E , Dominguez-Meijide A , Buell AK , Outeiro TF , Otzen DE ((2022) ) Glycationmodulates alpha-synuclein fibrillization kinetics: A sweet spot forinhibition. J Biol Chem 298: , 101848. |
[79] | Richarme G , Liu C , Mihoub M , Abdallah J , Leger T , Joly N , Liebart J-C , Jurkunas UV , Nadal M , Bouloc P , Dairou J , Lamouri A ((2017) ) Guanine glycation repair by DJ-1/Park7 and its bacterial homologs. Science 357: , 208–211. |
[80] | Fearnley JM , Lees AJ ((1991) ) Ageing and Parkinson’s disease: Substantia nigra regional selectivity. Brain 114: , 2283–2301. |
[81] | Greffard S , Verny M , Bonnet AM , Beinis JY , Gallinari C , Meaume S , Piette F , Hauw JJ , Duyckaerts C ((2006) ) Motor score of the Unified Parkinson Disease Rating Scale as a good predictor of Lewy body-associated neuronal loss in the substantia nigra. Arch Neurol 63: , 584–588. |
[82] | Hansson O ((2021) ) Biomarkers for neurodegenerative diseases. Nat Med 27: , 954–963. |
[83] | Yang Y , Yuan Y , Zhang G , Wang H , Chen YC , Liu Y , Tarolli CG , Crepeau D , Bukartyk J , Junna MR , Videnovic A , Ellis TD , Lipford MC , Dorsey R , Katabi D ((2022) ) Artificial intelligence-enabled detection and assessment of Parkinson’s disease using nocturnal breathing signals. Nat Med 28: , 2207–2215. |