Nomogram for Early Prediction of Parkinson’s Disease Based on microRNA Profiles and Clinical Variables
Abstract
Background:
Few efficient and simple models for the early prediction of Parkinson’s disease (PD) exists.
Objective:
To develop and validate a novel nomogram for early identification of PD by incorporating microRNA (miRNA) expression profiles and clinical indicators.
Methods:
Expression levels of blood-based miRNAs and clinical variables from 1,284 individuals were downloaded from the Parkinson’s Progression Marker Initiative database on June 1, 2022. Initially, the generalized estimating equation was used to screen candidate biomarkers of PD progression in the discovery phase. Then, the elastic net model was utilized for variable selection and a logistics regression model was constructed to establish a nomogram. Additionally, the receiver operating characteristic (ROC) curves, decision curve analysis (DCA), and calibration curves were utilized to evaluate the performance of the nomogram.
Results:
An accurate and externally validated nomogram was constructed for predicting prodromal and early PD. The nomogram is easy to utilize in a clinical setting since it consists of age, gender, education level, and transcriptional score (calculated by 10 miRNA profiles). Compared with the independent clinical model or 10 miRNA panel separately, the nomogram was reliable and satisfactory because the area under the ROC curve achieved 0.72 (95% confidence interval, 0.68-0.77) and obtained a superior clinical net benefit in DCA based on external datasets. Moreover, calibration curves also revealed its excellent prediction power.
Conclusion:
The constructed nomogram has potential for large-scale early screening of PD based upon its utility and precision.
INTRODUCTION
Parkinson’s disease (PD) is a neurodegenerative illness that particularly affects older populations and poses a significant health burden with a doubling in prevalence, mortality and disability-adjusted life-years from 1990 to 2016 [1]. Recently, the prevalence of PD is rising faster than any other neurological disorder; therefore, PD has become an urgent public health issue [2]. The clinical features of PD worsen with time, often showing several non-motor symptoms (e.g., rapid eye movement sleep disorder, anosmia, constipation) early and then gradually progressing to severe and irreversible postural instability and cognitive impairment. The misfolded and aggregation of alpha-synuclein (SNCA) in the degenerative progression of PD are involved with the onset of motor and non-motor symptoms. PD is not curable; therefore, the prevention or delay of disease progression at an early stage (prodromal and early PD) is imperative. Because prodromal and early PD has an inconspicuous clinical presentation, it is necessary to obtain novel biomarkers for early identification. Recent studies suggest [3, 4] that miRNAs can be used as potential biomarkers for early diagnosis of PD, and a recent meta-analysis [5] indicates that blood miRNAs can serve as diagnostic biomarkers of PD by quantitative assessment of published miRNA expression data. The stable and abundant concentration of miRNA in blood and the development of ultrasensitive assays enable both accurate and precise measurement of miRNA expression. Increasingly, RNA databases also provide efficient annotation of miRNA targets [6]. Multiple studies demonstrate the downregulation/upregulations of miRNAs accompanied by the progression of PD [7–10]; however, the inconsistent findings among various studies have impeded the utilization of miRNA as an early diagnostic biomarker. Additionally, studies have revealed that many important demographic characteristics are associated with PD progression, including age and gender [11]. Clinical information can be obtained easily during routine follow-up, whereas the prediction power is controversial perhaps due to population heterogeneity in terms of inherent racial and ethnic disparities [12]. Taking into consideration the established evidence, we speculated that the combination of miRNA expression profiles and clinical variables may achieve a good value for the early prediction of PD.
A nomogram is a representative graphical calculating tool that has been widely used in clinical practice since it is easy to conduct and can be utilized for repeat assessments during clinical follow-ups [13]. Although many clinical models have been formulated for early identifying PD patients, none can be used widely among the general population. The possible reasons are due to the lack of effective external validation and inconvenience [14] as well as low accuracy. Furthermore, nearly all existing models rely on a very limited sample size of cross-sectional design while lacking robust support of evidence originating from the large longitudinal cohort. Therefore, there is an unmet need to formulate a risk clinical model for the early prediction of PD in usage. The Parkinson’s Progression Marker Initiative (PPMI, https://www.ppmi-info.org/) is a multi-center large-scale cohort study that began in 2010 and was aimed to identify biomarkers for early diagnosis and monitoring of therapy through enrolling newly diagnosed PD patients and matched healthy controls. The study samples come from 33 clinical sites that cover the United States, Europe, Western Asia (Israel), and the Western Pacific Region (Australia), which should make the findings generalized and reliable. Several studies have applied the clinical factors and genetic information [15] from the PPMI to predict motor progression and cognitive impairment of newly diagnosed PD, but none combined clinical features and miRNA as predictors for prodromal and early PD.
To fill this gap, this study aimed to develop and independently externally validate a nomogram by integrating a miRNA panel and several easily obtained clinical variables using PPMI. We hypothesized that the addition of a blood-based miRNA expression profile to clinical variables may contribute significantly to improved early prediction of PD.
MATERIALS AND METHODS
Study design and participants
The current study is based on the PPMI cohort established in 2010. The subjects include newly diagnosed PD patients, participants in the prodromal stage, and healthy controls from 33 clinical sites in the United States, Europe, Israel, and Australia. The clinical variables and blood samples were obtained at the study baseline and during four clinical follow-up visits during the next three years (6, 12, 24, and 36 months). Blood samples were obtained by venous draw during periodic clinical visits and small non-coding RNA sequencing was performed in batches. Detailed information regarding quality control and sample preparation of blood samples was provided in a previous study [16]. All microRNA (miRNA) expression levels were counted as normalized read counts, reads per million (RPM), and mapped to miRbase-v22. All datasets were downloaded from the PPMI database on June 1, 2022. The included criteria of participants were as follows: 1) Newly diagnosed PD patients in Hoehn and Yahr (HY) stage 1 or 2; 2) Participants in the prodromal stage; 3) Healthy controls; 4) Participants who underwent blood RNA sequencing. The excluded criteria were as follows: 1) Participants who had missing values of the Movement Disorder Society-Sponsored Revision of the Unified Parkinson’s Disease Rating Scale (MDS-UPDRS) total score or HY stage in the baseline; and 2) The specific miRNA will be deleted if in > 50% of blood samples the miRNAs are not expressed or expressed at levels too low to measure (RPM = 0). The determination of the cut-off value (50%) was mainly based on the distribution of the histogram (Supplementary Figure 1) for counts of blood samples with the abundance of miRNAs equal to 0 (RPM = 0) as well as consideration of corresponding standards in tissue /disease/cell lines samples demonstrating that about 70% of known miRNAs are expressed at a low level or not expressed (RPM < 1) [17].
A total of 1,284 subjects (706 men and 578 women) aged 19 to 92 years met the inclusion criteria and unmet excluded criteria and were admitted into the formal analysis. All participants were randomly divided into discovery datasets (n = 23) and external testing datasets (n = 10) based on the 33 clinical sites via simple sampling. In the discovery phase, a total number of 794 subjects contributed to 2,211 blood samples at 3 years of follow-up. To train and evaluate an accurate PD model, the discovery datasets were further randomly split into training and separate internal validation datasets at a ratio of 7 : 3 without replacement. Moreover, the established nomogram model was independently validated in the testing phase (n = 490). The complete study design is shown in Fig. 1.
Fig. 1
Flowchart of the study design. The nomogram was developed and validated in three phases, including biomarker screening, model construction, and external validation. For the biomarker screening phase, GEE model1ξ used the HY stages as an outcome indicator, while GEE model2ɛ used MDS-UPDRS as an outcome.
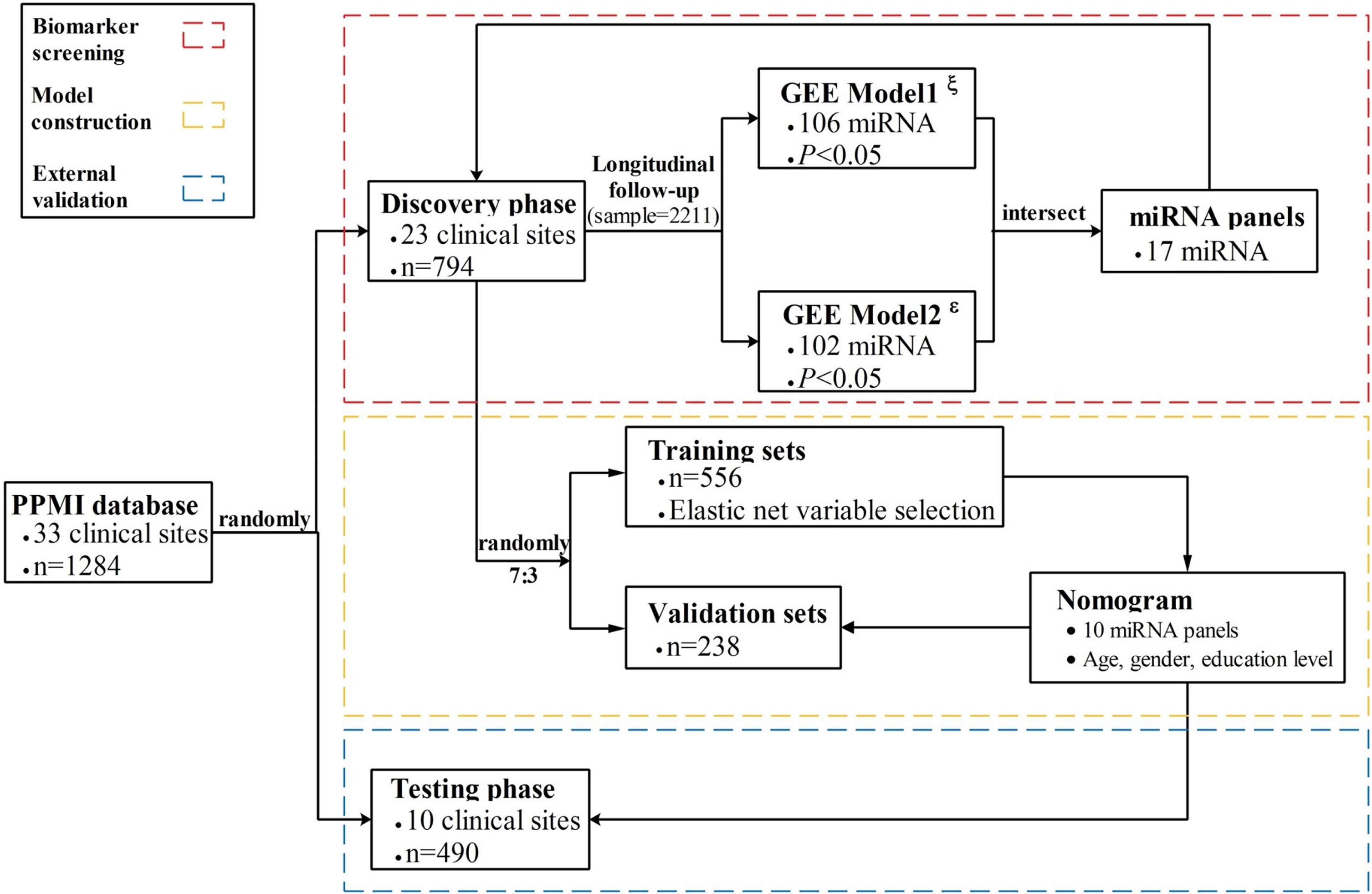
Predictors and outcomes definition
To screen candidate progressive biomarkers of PD, we defined the widely used two approaches to assess the progression of PD, including the HY stage and MDS-UPDRS. The HY stage describes 5 stages in the progression of PD, which were still recognized as an important reference standard for disability and impairment measures, although it put much attention to PD symptoms, for instance, postural instability [18]. The MDS-UPDRS refers to using four parts of scores reflecting the major symptoms of PD and monitoring the disease progression [19]. Emerging evidence suggests that PD severity, which was measured by HY stages, is associated with the differences defined by the MDS-UPDRS in all aspects of PD [20]. This study combined MDS-UPDRS and HY stages as outcomes, which contributed to improving the precision of the association analysis between miRNA expression and PD progression. To better assess the relationship between miRNA expression and PD progression risks, this study included healthy controls in the association analysis. The MDS-UPDRS total score and HY Stage of the control group were obtained from the PPMI database based on the assessment of both motor and non-motor symptoms associated with PD.
Furthermore, data on demographics and years of education were available in PPMI. The miRNA expression profiles of all participants cover 2,656 miRNAs, and the MDS-UPDRS total score was the addition of UPDRS part I, II to III. The case group was defined as newly diagnosed PD in HY stage 1 or 2 and participants in the prodromal stage, whereas all healthy participants were determined as the control group in this study.
Statistical analysis
Data on continuous variables were described as median (P25, P75), while category variables were represented as frequency (proportional). Chi-square test and Kruskal-Wallis test were utilized to compare the difference in baseline characteristics among training, validating and testing datasets. Initially, generalized estimating equation (GEE) models were used to examine the associations between miRNA expression levels and disease progression of PD which was reflected by the HY stages (GEE model1, GEE1) and MDS-UPDRS total score (GEE model2, GEE2). The sample size at the study baseline and additional 4 clinical visits are listed as follows: 794, 316, 432, 384, and 285. GEE is a quasi-likelihood estimating process that can give unbiased results under some missing data assumptions and analyze both discrete and continuous outcome variables. Finally, a total of 106 miRNAs were identified in GEE1 and 102 in GEE2 (p < 0.05). To screen the most relevant miRNAs of PD progression, we obtained intersect miRNAs (n = 17) between 106 miRNAs in GEE1 and 102 miRNAs in GEE2. Then, the randomly divided training datasets (n = 556) were used to develop a nomogram, while the remaining datasets (n = 238) further validated the model performance. Since several studies [21, 22] have demonstrated that elastic net generally outperforms both lasso and ridge regression by balancing between lasso and ridge penalties, the elastic net regression model was applied to select predictors associated with early PD by comprehensively considering the Akaike information criterion (AIC) and the sample-size adjusted AIC (AICC). Moreover, the lasso regression model was additionally performed to evaluate the variables selection approach of this study.
In clinical studies, especially those conducted across multiple centers, it can be challenging to gather complete data on participants at every site. Therefore, we utilized random sampling for validation (split sample validation) instead of full validation using a different study. Although training and validation sets were not selected by some characteristic, such as patient enrollment/diagnosis time, or geographic region, we still yielded valid results via random sampling. Moreover, we implemented the elastic net regression model in training datasets for variable selection by cross-validation (8-fold), which improves the stability of the selection of predictors and the quality of predictors [23].
Finally, the best nomogram consisting of a 10-miRNA panel, age, gender, and education level was established by the logistics regression model. The receiver operating characteristic (ROC) and decision curve analysis (DCA) curves were applied to evaluate the prediction accuracy and clinical net benefit of the nomogram. Calibration curves were drawn to determine the consistency between the observed and predicted risks of the nomogram. Additionally, the testing datasets were used to validate the efficacy and generalization of the nomogram by integrating ROC, DCA, and calibration curves. Waterfall plots were used to depict the subjects with the application of the nomogram for comparison risk scores between healthy control and cases.
Table 1
Baseline characteristics in the training, validation, and testing datasets
Variables | Training (n = 556) | Validation (n = 238) | Testing (n = 490) | p |
Age, y | 62.9 (54.7,69.9) | 61.2 (54.1,69.7) | 62.0 (51.8,68.5) | 0.122 |
MDS-UPDRS total score | 18.0 (5.0,34.0) | 16.0 (5.0,32.0) | 15.0 (5.0,32.0) | 0.533 |
Gender | 0.348 | |||
Man | 297 (53.4) | 127 (53.4) | 282 (57.6) | |
Woman | 259 (46.6) | 111 (46.6) | 208 (42.4) | |
Education level | 0.715 | |||
Less than 12, y | 49 (8.8) | 15 (6.3) | 36 (7.3) | |
12–16, y | 262 (47.1) | 116 (48.7) | 216 (44.1) | |
Greater than 16, y | 221 (39.7) | 97 (40.8) | 216 (44.1) | |
Missing | 24 (4.3) | 10 (4.2) | 22 (4.5) | |
Hoehn and Yahr staging | 0.293 | |||
0 | 252 (45.3) | 113 (47.5) | 252 (51.4) | |
1 | 106 (19.1) | 45 (18.9) | 93 (19.0) | |
2 | 198 (35.6) | 80 (33.6) | 145 (29.6) |
The Hoehn and Yahr staging (HY Stage) 0 indicates no signs of PD symptoms, whereas the HY Stage 1 and 2 mean that tremor, rigidity, reduced arm swing, and slowness are present on one or both sides of the body.
Target genes of the 10 miRNAs were obtained from miRTarBase. Enrichment function analysis was additionally performed on the combined target genes regulated by the obtained 10 miRNAs. The GO analysis was used to identify potential biological evidence of the target gene with PD in three ontologies: biological process (BP), cellular component (CC), and molecular function (MF). KEGG enrichment analysis was performed to examine computationally predicted biological pathways of target genes. All bioinformatics analyses were performed by the R package “clusterProfiler”, and a ‘simplify’ function was used to eliminate redundant GO terms in the analysis based on their semantic similarities (>0.7) [25]. The adjusted p-value was calculated by the Benjamini and Hochberg (BH) approaches. All tests were two-sided and a p value less than 0.05 was recognized as statistically significant. The data management and statistical analyses in this study were completed by SAS 9.4 (Copyright 2002–2012 by SAS Institute Inc., Cary, NC, USA) and R-studio 2021.09.1 (Copyright 2009–2019 RStudio, Inc.). All sampling was conducted utilizing PROC SURVEYSELECT (SEED = 123) in SAS 9.4. The source code and part data were deposited at https://github.com/XiangqingHou/Blood-based-miRNA-and-its-nomogram-for-early-prediction-of-PD.
RESULTS
The characteristics of study populations
A total of 1,284 participants were included in the formal analysis, and the baseline median (P25, P75) age was 62 (54, 69) years. The ratio of males to females was approximately 120%. In this study, 55.4% of subjects (n = 711) were classified as case groups which included early PD and prodromal cases, while the remaining were defined as healthy controls (n = 573). A table comparing PD cases, prodromal cases, and controls is shown in Supplementary Table 1. We obtained the MDS-UPDRS total score and HY stage and then integrated the 2 methods to assess the disease progression of PD. The MDS-UPDRS total median (P25, P75) score was 31 (21, 42) among the case group, which was significantly larger than healthy controls (p < 0.001). At baseline, the numbers of subjects in HY stages 0, 1, and 2 were 617, 244, and 423, respectively. According to the study design (Fig. 1), we assigned the 33 clinical sites as numerical labels and then used simple sampling randomly selecting 23 sites labeled as discovery datasets and the remaining (n = 10) as external testing datasets. Then, the discovery datasets were randomly split into a training and another separate validation set at a ratio of 7 : 3 without replacement. Finally, all participants were assigned to training (n = 556), validation (n = 238), and external testing sets (n = 490) separately. The comparison of baseline clinical indicators among the three datasets (Table 1) suggests there is no significant difference in all variables (p > 0.05), which suggests well-balanced comparability. In the discovery phase, 794 subjects contributed to 2,211 blood samples at 5 longitudinal follow-ups over 3 years.
Biomarker screening of miRNA profiles
To screen reliable candidate miRNAs which were associated with the progression of PD, we conducted a comprehensive integrative analysis using 2 GEE models which applied MDS-UPDRS total score and HY stages as outcomes separately. The GEE model is very suitable for modeling longitudinal samples, which enabled our findings to be robust and reliable. Finally, we obtained 17 miRNAs from 2,656 miRNAs that were annotated by miRbase-v22 from PPMI. Additionally, we also implemented the elastic net regression model in training datasets for variable selection by cross-validation (8-fold). Finally, we obtained 10 miRNAs to calculate the transcriptional score since the model with the 10-miRNA panel has the lowest AIC and AICC. The 10-miRNA panel included miR-4301, miR-190a-5p, miR-22-3p, miR-3200-5p, miR-3613-5p, miR-423-5p, miR-4433b-5p, miR-4677-5p, miR-548b-5p, and miR-654-5p. (Supplementary Figure 2). Moreover, the results (Supplementary Figure 3) clearly reveal that the best LASSO model consists of 13 predictors (10 miRNAs, age, sex, and education level), which is consistent with the variable selection of the elastic net model. Additionally, the box plots for the expression level of the 10 identified miRNAs based on control (healthy participants) and case groups in the training, validation and testing datasets separately can be observed in Supplementary Figure 4.
Development and construction of a nomogram
The logistics regression model was utilized to establish a nomogram using 10 obtained miRNAs, age, gender, and education level (Fig. 2). Based on the coefficients of the regression model, the transcriptional score was defined as follows and the abundance of miRNAs was measured with normalized expression units (RPM, reads per million mapped reads):
Fig. 2
Nomogram to predict the risk of PD. Shown are clinical predictors and transcriptional scores as well as their corresponding nomogram points. The total points indicate the addition of the scores for each variable, then a vertical line can be drawn to determine the risk of PD according to the total points.
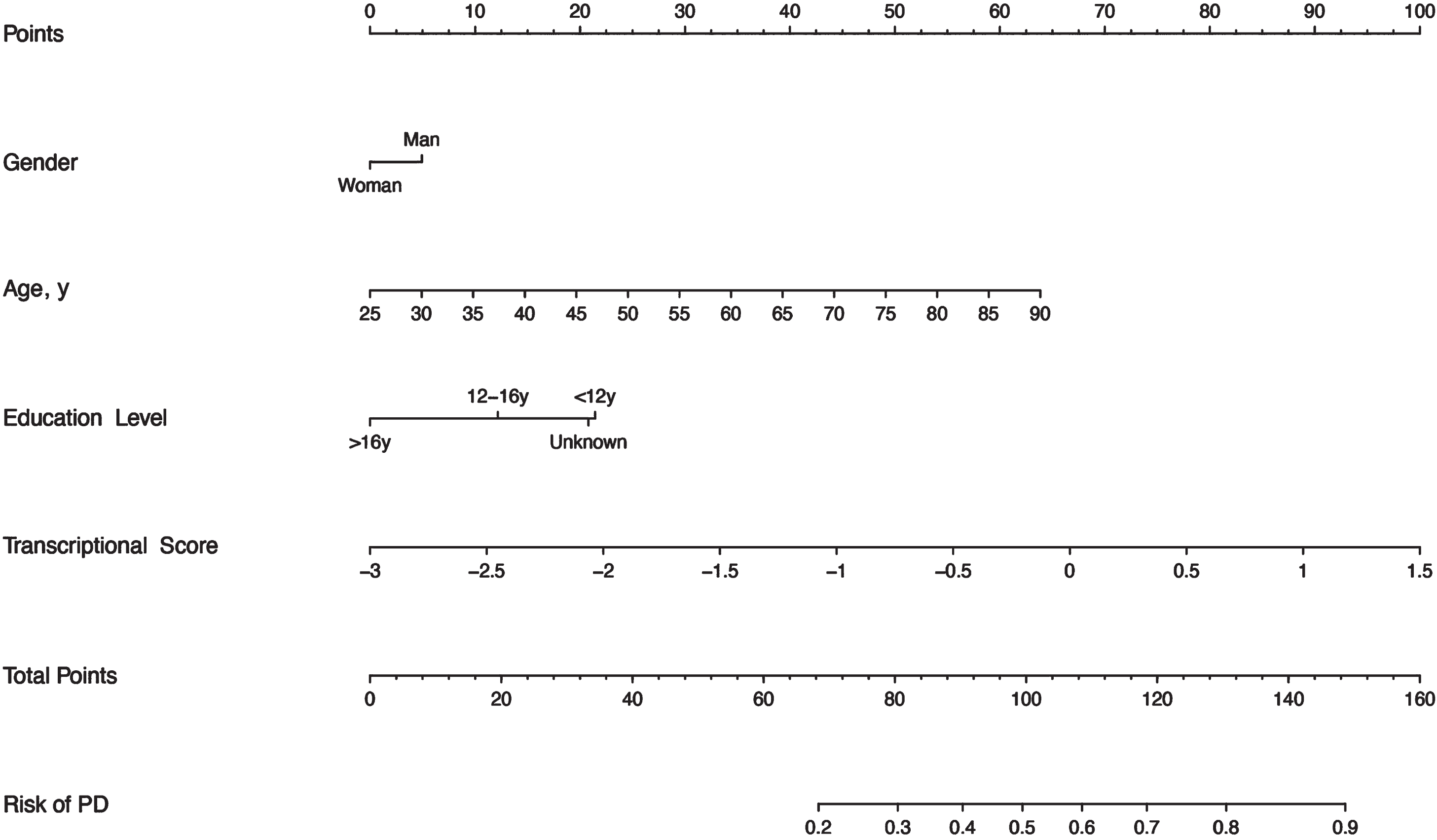
This nomogram is convenient to use in the clinic. For instance, we take as an example a man aged 55 years with about 15 years of education, with a transcriptional score of the miRNAs profile equal to 1. Based on the nomogram, we can obtain the corresponding score of about 5, 29, 13, and 88, respectively. Then, the total score is 135 (5 + 29 + 13 + 88), which can indicate the risk of PD is approaching 0.8 to 0.9.
Validation and evaluation of the nomogram
To comprehensively validate and evaluate the prediction performance of the nomogram and further investigate whether it performs better than the individual clinical model and 10 miRNA panel individually, we analyzed and compared the ROC, DCA, and calibration curves of the various models in this study and concluded that for the ROC curves (Fig. 3), the nomogram displayed overall satisfactory and stable predictive performance since the AUC (95% CI) achieved 0.70 (0.65–0.74), 0.74 (0.68–0.80), and 0.72 (0.68–0.77) in training, validation, and testing datasets, separately. Additionally, the nomogram performs statistically better (p < 0.05) than the individual clinical model or 10 miRNAs panel in all three datasets (Supplementary Table 2). For the DCA curves (Fig. 4), the results suggest that the nomogram overall can achieve a higher clinical net benefit (NB) than other intervention strategies in all three datasets. Moreover, compared with others, the nomogram can obtain the largest NB when the threshold probability (TP) was between 50% to 75%. In contrast, in Supplementary Figure 5, the nomogram presented a higher net reduction (NR) than treating all strategies and significantly reduced the unnecessary interventions when the TP was greater than 15%. For the calibration curves (Fig. 5), the nomogram displayed a good predictive consistency between observed and predicted PD risk. The calibration curve achieved approximately ideal agreement between observed outcomes and predictions and was shown with a 45° line in the testing datasets, which further confirmed the prediction accuracy and robustness of the nomogram.
Fig. 3
ROC curves for comparisons of the nomogram, clinical, and miRNA panel in the training, validation, and testing datasets. The labels show the AUC and its 95% confidence interval in the nomogram (blue line), miRNA panel (red line), and clinical model (green line). The clinical model consists of age, gender, and education level, while the miRNA panel includes 10 miRNAs.
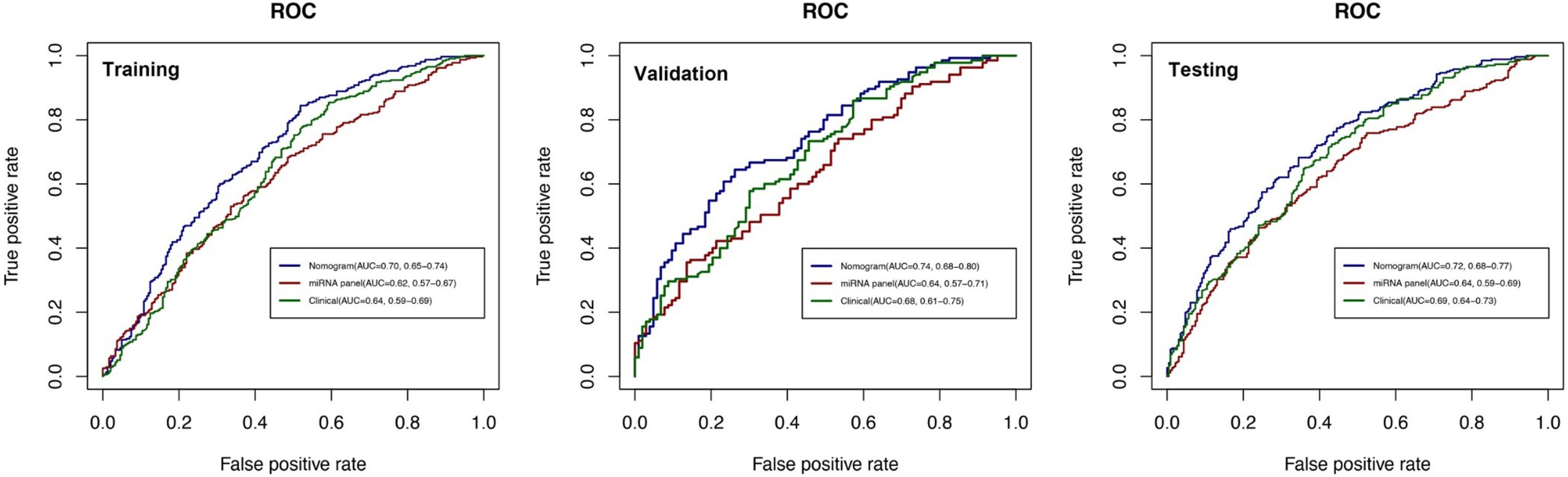
Fig. 4
DCA curves for comparisons of the nomogram, clinical and miRNA panel in training, validation, and testing datasets. Shown are the threshold probability (TP) on the x-axis and the net benefit on the y-axis. Assuming the TP is predetermined, the models which are close to the top regions can obtain the maximum net benefit compared with treating all or treating none or other interventions. The clinical model consists of age, gender, and education level, while the miRNA panel includes 10 miRNAs.
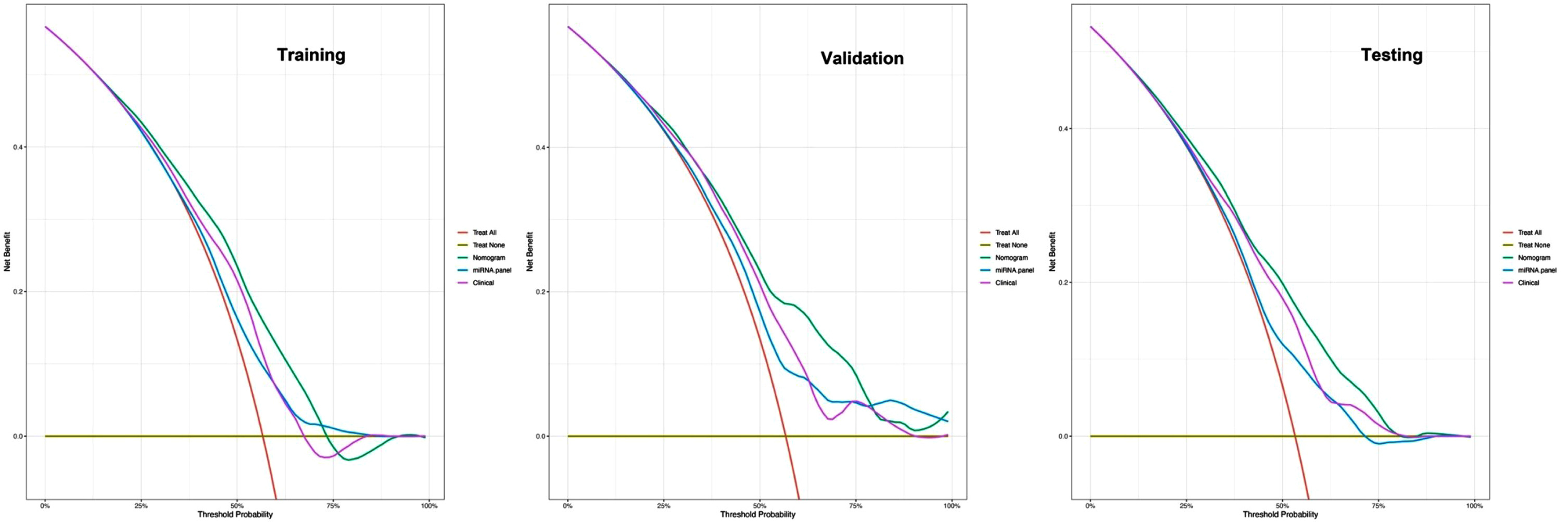
Fig. 5
Calibration curves of the nomogram in the training, validation and testing datasets. Shown are the nomogram predicted probability (PP) for early PD on the x-axis and the observed probabilities (OP) on the y-axis. The “Ideal” grey dotted line represents the PP and OP are totally anastomoses. The “Apparent” (red line) represents the comparison of the PP and OP of the nomogram, while the “Bias-corrected” (green line) indicates an adjusted calibration curve across predicted values by 1,000 bootstrapped samples.
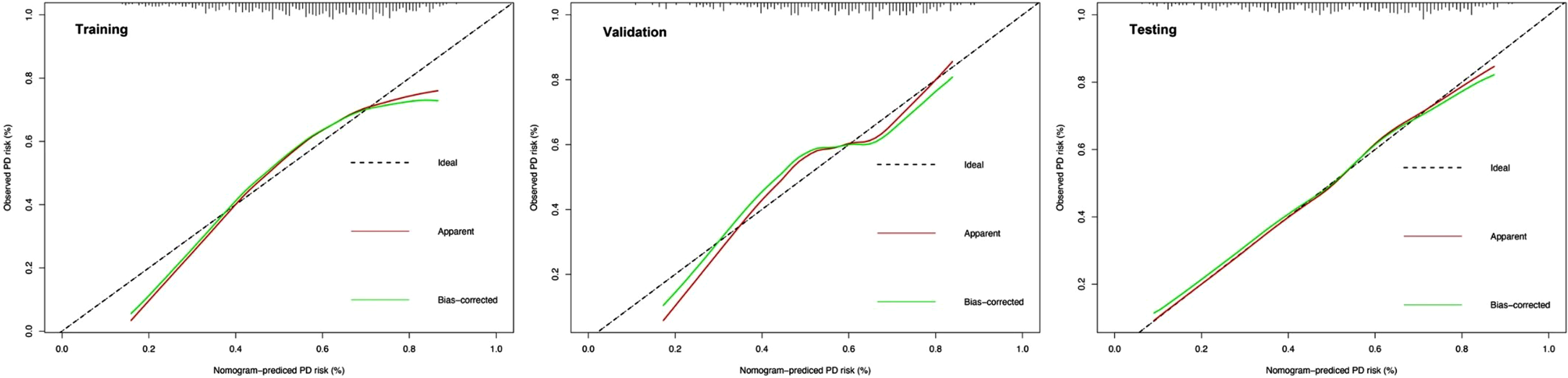
In the training set, the total nomogram scores calculated by the nomogram were categorized into two risk groups, low-risk (<42.1) and high-risk (≥42.1), following the cut-off points detected by the ROC analysis. The waterfall plot for the distribution of nomogram scores between healthy control and cases (prodromal and early PD) of individuals in the training, validation and testing cohort separately can be observed in Supplementary Figure 6.
Prediction of miRNA targets and over-representation analysis
To better interpret the biological correlations between the obtained 10 miRNAs and the risk of PD, we obtained 1,084 predicted target genes that may be regulated by the 10 miRNAs. Over-representation analysis of the target genes reported a significant enrichment for response to oxygen levels, decreased oxygen levels, hypoxia, etc. that were associated with oxidative stress in biological processes terms. Additionally, significant enriched GO terms for protein kinase activity or kinase regulator activity can be identified in BP, CC, and MF ontologies (Supplementary Figure 7). Interestingly, the KEGG analysis not only identified cellular senescence enrichment pathways implicated with DNA damage, oxidative stress and neuroinflammation, but also depicted several cancer-related enrichment pathways, for instance, the glioma and p53 signaling pathways (Supplementary Figure 8).
DISCUSSION
Recently, prediction models through simple tests have been widely applied in many diseases such as cardiovascular disease by detecting blood pressure and glycated hemoglobin [26]. However, no such simple clinical model has been developed for predicting PD at the early stage (prodromal and early PD). To address this issue, this study aimed to develop and validate an easy-to-use clinical tool for the early screening of high-risk PD patients among the general population based on the PPMI database. Our constructed and validated nomogram outperforms an individual clinical model or 10 miRNA panel separately, which confirms the improved prediction efficacy for prodromal and early PD when blood-based miRNA expression data are combined with clinical variables. Blood-based miRNAs are believed to be an ideal biomarker of PD because of their cost-effective and non-invasiveness properties [27]. A recent study [9] identified a 6-serum extracellular vesicle-derived miRNA panel for early prediction of PD and the model achieved a satisfactory prediction power. However, in that study, the limited sample size and all samples obtained from a cross-sectional design reduced the reliability of the predictors which can be observed by wider confidence intervals of AUC in the ROC curve. Another similar study [28] integrated a blood-based gene expression classifier and DNA methylation data to predict the occurrence of PD. Although the predictive performance of the top 21 hypo-up gene and top 33 hypo-up gene methylation classifier is overall satisfactory, it lacks the simplicity of use and independent external validation. Khoo et al. [29] demonstrated the feasibility of a plasma-based circulating miRNA panel as diagnosis biomarkers via Real-Time Quantitative Reverse Transcription PCR (qRT-PCR); however, their findings lacked adequate evaluations for the predictive performance of the miRNA panel, for example, the discrimination, calibration, and clinical effectiveness of the predictive model should be well considered and reported [30]. In comparison to these, our study has several strengths. First, this is the first to develop a simple nomogram for early prediction of PD by integrating miRNA expression levels and clinical variables based on a large longitudinal multi-center study. Second, we applied many statistical methods and separate testing datasets to comprehensively evaluate the model performance. Third, the nomogram is very convenient for clinical usage and repeat assessments of individuals.
Recently epidemiologic evidence [31] suggests that nearly 0.1 and 0.2% of the populations around the world are affected by PD and the prevalence is increasing with age as well as affecting approximately 1% of the population over 60 years of age. We can take as an example, a hypothetical country of 100 million people, and 10 million greater than 60 years of age. We define a benchmark that the general population with a probability of early PD greater than 75% should be directed towards clinical interventions, while the specific probability threshold was reduced to 15% among those over 60. In this case, our nomogram yielded a net reduction (NR)all=24% and NR > 60,years = 1%, respectively (Supplementary Figure 5). That means compared to the strategies of treating all, the nomogram could reduce unnecessary interventions of 24 million among the general population and 1 million among those over 60, separately [32]. Therefore, we propose using this nomogram as a large-scale population screening tool for early PD which can obtain a maximum benefit at a low cost.
To comprehensively investigate the associations of the 10-miRNA panel and their 1,084 potential downstream target genes with the pathogenesis of PD, we further performed miRNA target enrichment analysis. Our findings revealed that several GO categories are significantly implicated with oxidative stress and the ubiquitin-proteasome system. More specifically, it has been shown that oxidative stress and the ubiquitin-proteasome system are the key molecular pathogenic mechanisms of PD [33]. The GO categories concerning several protein kinase complex activities, for instance, serine/threonine kinase [34] and mitogen-activated protein kinase [35], which have been confirmed implicated with the pathobiology of PD were also revealed by significant enrichment of the miRNA targets. Serine/threonine kinases play a role in the regulation of blood and immune cell populations and meet the metabolic demands of participating in an immune response by mediating TCR signaling [36]. Mitogen-activated protein kinase also acts as an integration point for extracellular signals and regulates immune cell defense [37]. Our findings also revealed several cancer-related enrichment pathways of the target genes. We speculate this is due to PD and some cancers, for instance, glioma, sharing several coincident biological mechanisms in the development of disease [38].
Currently, the diagnosis of PD mostly depends on clinical symptoms; therefore, the misdiagnosis rate of PD is still high because of indistinguishable symptoms between atypical parkinsonian disorders and PD, especially at the early stage of PD. According to Occam’s Razor [39], the best model is the one that covers fewer variables and can achieve optimal prediction performance simultaneously; therefore, we utilized only three important demographic characteristics (age, gender, and education level) which can be easily obtained at the early stage of PD. It has been well known that the risk of PD increases rapidly with age, and the age-standardized prevalence ratio of males to females is about 1.5 in 2016 according to Global Burden Disease data [1]. Several studies have revealed that demographic characteristics can be good predictors of early PD, although their predictive power is controversial. To improve the model’s performance, we first combined miRNA profiles with the clinical model to develop a novel nomogram for the early prediction of PD. The results suggested that compared with the individual miRNA panels and clinical model, our nomogram has a superior predictive power since it displays significantly higher AUC of ROC than the two individual models separately in the validation datasets (0.74 vs. 0.64, p = 0.002; 0.74 vs. 0.68, p = 0.004). Additionally, we comprehensively balanced the underfitting and overfitting of the nomogram via cross-validation.
Several research limitations should be noted. We speculate that there is still much room for improvement in the predictive power of our nomogram via identifying novel miRNAs or clinical features and utilizing more machine learning (ML) methods. A recent similar study [14] used PPMI datasets but with more complicated ML methods and all available variables to predict PD risk, and their model has shown a high AUC prediction. Compared with Makarious et al.’s research [14], our study has several outstanding features: 1) We utilized the GEE model to analyze longitudinal RNA-sequencing data in the biomarker screening phase, which makes our results more reliable and stable. However, Makarious and his colleagues only obtained blood samples at baseline and used the logistic regression model to perform differential expression between cases and controls; 2) This study utilized non-coding miRNA as predictors because of their availability in body fluids and enrichment in serum extracellular vesicles; therefore, miRNAs can penetrate the blood-brain barrier [40] based on their small molecular weight ( 22 nucleotides) and stability when circulating between cells. Moreover, miRNAs can significantly increase blood–brain barrier permeability by regulating biological processes such as apoptosis and inflammation; 3) Our nomogram is very suitable for utilization in the clinic since it is easy to use for doctors and to explain to patients. On the contrary, Makarious’s model used complex ML methods to integrate genetics, clinic-demographic, and transcriptomics data which requires highly technical and complicated tests at a cost of more resources. It has been well known that an AUC greater than 0.7 may be taken as an acceptable clinical usage cut-off value [41], particularly based on the evidence from a large-scale longitudinal cohort. In this case, our model can provide satisfactory cost-effective prediction performance now and may present a good reference model for further similar studies. Besides, more biological evidence of the obtained miRNAs as early biomarkers of PD may be further provided by experiments. The enrolled participants only come from PPMI, and all samples were assayed on a next-generation sequencing platform. This might limit the generalizability of our findings to other independent populations using different technologies.
In conclusion, we developed and validated an effective and simple nomogram for the early identification of PD. This nomogram enables early diagnosis, and monitoring of disease progression in individuals as well as may guide limited clinical interventions.
ACKNOWLEDGMENTS
We would like to acknowledge the Parkinson’s Progression Markers Initiative (PPMI) as the source of all data in the study. PPMI – a public-private partnership – is funded by the Michael J. Fox Foundation for Parkinson’s Research funding partners 4D Pharma, Abbvie, Acurex Therapeutics, Allergan, Amathus Therapeutics, ASAP, Avid Radiopharmaceuticals, Bial Biotech, Biogen, BioLegend, Bristol-Myers Squibb, Calico, Celgene, Dacapo Brain Science, Denali, The Edmond J. Safra Foundaiton, GE Healthcare, Genentech, GlaxoSmithKline, Golub Capital, Handl Therapeutics, Insitro, Janssen Neuroscience, Lilly, Lundbeck, Merck, Meso Scale Discovery, Neurocrine Biosciences, Pfizer, Piramal, Prevail, Roche, Sanofi Genzyme, Servier, Takeda, Teva, UCB, Verily, and Voyager Therapeutics.
FUNDING
This work was supported by the Faculty of Health Sciences, University of Macau [grant number MYRG2020-00213-FHS] to G.W. The funders had no roles in study design, data collection, data analysis and interpretation as well as the writing of the report. XQ.H. and G.W. had full access to the data in the study and had final responsibility for the decision to submit it for publication.
CONFLICT OF INTEREST
The authors have no conflict of interest to report.
DATA AVAILABILITY
Data used in the preparation of this article were obtained from the Parkinson’s Progression Markers Initiative (PPMI) database (https://www.ppmi-info.org/data). For up-to-date information on the study, visit https://www.ppmi-info.org.
SUPPLEMENTARY MATERIAL
[1] The supplementary material is available in the electronic version of this article: https://dx.doi.org/10.3233/JPD-225080.
REFERENCES
[1] | GBD 2016 Parkinson’s Disease Collaborators ((2018) ) Global, regional, and national burden of Parkinson’s disease, 1990–2016: A systematic analysis for the Global Burden of Disease Study 2016. Lancet Neurol 17: , 939–953. |
[2] | The Lancet Neurology ((2022) ) Parkinson’s disease needs an urgent public health response. Lancet Neurol 21: , 759. |
[3] | Kuo MC , Liu SC , Hsu YF , Wu RM ((2021) ) The role of noncoding RNAs in Parkinson’s disease: Biomarkers and associations with pathogenic pathways. J Biomed Sci 28: , 78. |
[4] | Manna I , Quattrone A , De Benedittis S , Iaccino E , Quattrone A ((2021) ) Roles of non-coding RNAs as novel diagnostic biomarkers in Parkinson’s disease. J Parkinsons Dis 11: , 1475–1489. |
[5] | Schulz J , Takousis P , Wohlers I , Itua IOG , Dobricic V , Rücker G , Binder H , Middleton L , Ioannidis JPA , Perneczky R , Bertram L , Lill CM ((2019) ) Meta-analyses identify differentially expressed micrornas in Parkinson’s disease. Ann Neurol 85: , 835–851. |
[6] | Kozomara A , Birgaoanu M , Griffiths-Jones S ((2019) ) miRBase: From microRNA sequences to function. Nucleic Acids Res 47: , D155–D162. |
[7] | Behbahanipour M , Peymani M , Salari M , Hashemi MS , Nasr-Esfahani MH , Ghaedi K ((2019) ) Expression profiling of blood microRNAs. 885, 361, and 17 in the patients with the Parkinson’s disease: Integrating interaction data to uncover the possible triggering age-related mechanisms. Sci Rep 9: , 13759. |
[8] | Dong H , Wang C , Lu S , Yu C , Huang L , Feng W , Xu H , Chen X , Zen K , Yan Q , Liu W , Zhang C , Zhang CY ((2016) ) A panel of four decreased serum microRNAs as a novel biomarker for early Parkinson’s disease. Biomarkers 21: , 129–137. |
[9] | He S , Huang L , Shao C , Nie T , Xia L , Cui B , Lu F , Zhu L , Chen B , Yang Q ((2021) ) Several miRNAs derived from serum extracellular vesicles are potential biomarkers for early diagnosis and progression of Parkinson’s disease. Transl Neurodegener 10: , 25. |
[10] | Fazeli S , Motovali-Bashi M , Peymani M , Hashemi MS , Etemadifar M , Nasr-Esfahani MH , Ghaedi K ((2020) ) A compound downregulation of SRRM2 and miR-27a-3p with upregulation of miR-27b-3p in PBMCs of Parkinson’s patients is associated with the early stage onset of disease. PLoS One 15: , e0240855. |
[11] | Schrag A , Siddiqui UF , Anastasiou Z , Weintraub D , Schott JM ((2017) ) Clinical variables and biomarkers in prediction of cognitive impairment in patients with newly diagnosed Parkinson’s disease: A cohort study. Lancet Neurol 16: , 66–75. |
[12] | Dahodwala N , Siderowf A , Xie M , Noll E , Stern M , Mandell DS ((2009) ) Racial differences in the diagnosis of Parkinson’s disease. Mov Disord 24: , 1200–1205. |
[13] | Hou X , Wang D , Zuo J , Li J , Wang T , Guo C , Peng F , Su D , Zhao L , Ye Z ((2019) ) Development and validation of a prognostic nomogram for HIV/AIDS patients who underwent antiretroviral therapy: Data from a China population-based cohort. EBioMedicine 48: , 414–424. |
[14] | Makarious MB , Leonard HL , Vitale D , Iwaki H , Sargent L , Dadu A , Violich I , Hutchins E , Saffo D , Bandres-Ciga S , Kim JJ , Song Y , Maleknia M , Bookman M , Nojopranoto W , Campbell RH , Hashemi SH , Botia JA , Carter JF , Craig DW , Van Keuren-Jensen K , Morris HR , Hardy JA , Blauwendraat C , Singleton AB , Faghri F , Nalls MA ((2022) ) Multi-modality machine learning predicting Parkinson’s disease. NPJ Parkinsons Dis 8: , 35. |
[15] | Latourelle JC , Beste MT , Hadzi TC , Miller RE , Oppenheim JN , Valko MP , Wuest DM , Church BW , Khalil IG , Hayete B , Venuto CS ((2017) ) Large-scale identification of clinical and genetic predictors of motor progression in patients with newly diagnosed Parkinson’s disease: A longitudinal cohort study and validation. Lancet Neurol 16: , 908–916. |
[16] | Kern F , Fehlmann T , Violich I , Alsop E , Hutchins E , Kahraman M , Grammes NL , Guimarães P , Backes C , Poston KL , Casey B , Balling R , Geffers L , Krüger R , Galasko D , Mollenhauer B , Meese E , Wyss-Coray T , Craig DW , Van Keuren-Jensen K , Keller A ((2021) ) Deep sequencing of snoRNAs reverals hallmarks and regulatory modules of the transcriptome during Parkinson’s disease progression. Nat Aging 1: , 309–322. |
[17] | Gong J , Wu YL , Zhang XT , Liao YF , Sibanda VL , Liu W , Guo AY ((2014) ) Comprehensive analysis of human small RNA sequencing data provides insights into expression profiles and miRNA editing. RNA Biol 11: , 1375–1385. |
[18] | Hoehn MM , Yahr MD ((1967) ) Parkinsonism: Onset, progression, and mortality. Neurology 17: , 427–442. |
[19] | Goetz CG , Tilley BC , Shaftman SR , Stebbins GT , Fahn S , Martinez-Martin P , Poewe W , Sampaio C , Stern MB , Dodel R , Dubois B , Holloway R , Jankovic J , Kulisevsky J , Lang AE , Lees A , Leurgans S , LeWitt PA , Nyenhuis D , Olanow CW , Rascol O , Schrag A , Teresi JA , van Hilten JJ , LaPelle N , Movement Disorder Society UPDRS Revision Task Force ((2008) ) Movement Disorder Society-sponsored revision of the Unified Parkinson’s Disease Rating Scale (MDS-UPDRS): Scale presentation and clinimetric testing results. Mov Disord 23: , 2129–2170. |
[20] | Skorvanek M , Martinez-Martin P , Kovacs N , Rodriguez-Violante M , Corvol JC , Taba P , Seppi K , Levin O , Schrag A , Foltynie T , Alvarez-Sanchez M , Arakaki T , Aschermann Z , Aviles-Olmos I , Benchetrit E , Benoit C , Bergareche-Yarza A , Cervantes-Arriaga A , Chade A , Cormier F , Datieva V , Gallagher DA , Garretto N , Gdovinova Z , Gershanik O , Grofik M , Han V , Huang J , Kadastik-Eerme L , Kurtis MM , Mangone G , Martinez-Castrillo JC , Mendoza-Rodriguez A , Minar M , Moore HP , Muldmaa M , Mueller C , Pinter B , Poewe W , Rallmann K , Reiter E , Rodriguez-Blazquez C , Singer C , Tilley BC , Valkovic P , Goetz CG , Stebbins GT ((2017) ) Differences in MDS-UPDRS scores based on Hoehn and Yahr stage and disease duration. Mov Disord Clin Pract 4: , 536–544. |
[21] | Zou H , Hastie T ((2005) ) Regularization and variable selection via the elastic net. J R Stat Soc Series B Stat Methodol 67: , 301–320. |
[22] | Sirimongkolkasem T , Drikvandi R ((2019) ) On regularisation methods for analysis of high dimensional data. Ann Data Sci 6: , 737–763. |
[23] | Steyerberg EW , Vergouwe Y ((2014) ) Towards better clinical prediction models: Seven steps for development and an ABCD for validation. Eur Heart J 35: , 1925–1931. |
[24] | Huang HY , Lin YC , Cui S , Huang Y , Tang Y , Xu J , Bao J , Li Y , Wen J , Zuo H , Wang W , Li J , Ni J , Ruan Y , Li L , Chen Y , Xie Y , Zhu Z , Cai X , Chen X , Yao L , Chen Y , Luo Y , LuXu S , Luo M , Chiu CM , Ma K , Zhu L , Cheng GJ , Bai C , Chiang YC , Wang L , Wei F , Lee TY , Huang HD ((2022) ) miRTarBase update 2022: An informative resource for experimentally validated miRNA-target interactions. Nucleic Acids Res 50: , D222–D230. |
[25] | Wu T , Hu E , Xu S , Chen M , Guo P , Dai Z , Feng T , Zhou L , Tang W , Zhan L , Fu X , Liu S , Bo X , Yu G ((2021) ) clusterProfiler 4.0: A universal enrichment tool for interpreting omics data. Innovation (Camb) 2: , 100141. |
[26] | Angelantonio ED , Gao P , Khan H , Butterworth AS ((2014) ) Glycated hemoglobin measurement and prediciton of cardiovascular disease. JAMA 311: , 1225–1233. |
[27] | Roser AE , Gomes LC , Schunemann J , Maass F , Lingor P ((2018) ) Circulating miRNAs as diagnostic biomarkers for Parkinson’s disease. Front Neurosci 12: , 625. |
[28] | Wang C , Chen L , Yang Y , Zhang M , Wong G ((2019) ) Identification of potential blood biomarkers for Parkinson’s disease by gene expression and DNA methylation data integration analysis. Clin Epigenetics 11: , 24. |
[29] | Khoo SK , Petillo D , Kang UJ , Resau JH , Berryhill B , Linder J , Forsgren L , Neuman LA , Tan AC ((2012) ) Plasma-based circulating MicroRNA biomarkers for Parkinson’s disease. J Parkinsons Dis 2: , 321–331. |
[30] | Zhou ZR , Wang WW , Li Y , Jin KR , Wang XY , Wang ZW , Chen YS , Wang SJ , Hu J , Zhang HN , Huang P , Zhao GZ , Chen XX , Li B , Zhang TS ((2019) ) In-depth mining of clinical data: The construction of clinical prediction model with R. Ann Transl Med 7: , 796. |
[31] | Tysnes OB , Storstein A ((2017) ) Epidemiology of Parkinson’s disease. J Neural Transm (Vienna) 124: , 901–905. |
[32] | Chen JJ , Shen SP , Li Y , Fan JJ , Xiong SY , Xu JT , Zhu CX , Lin LJ , Dong XS , Duan WW , Zhao Y , Qian X , Liu ZH , Wei YY , Christiani DC , Zhang RY , Chen F ((2022) ) APOLLO: An accurate and independently validated prediction model of lower-grade gliomas overall survival and a comparative study of model performance. EBioMedicine 79: , 104007. |
[33] | Jankovic J , Tan EK ((2020) ) Parkinson’s disease: Etiopathogenesis and treatment. J Neurol Neurosurg Psychiatry 91: , 795–808. |
[34] | Mehdi SJ , Rosas-Hernandez H , Cuevas E , Lantz SM , Barger SW , Sarkar S , Paule MG , Ali SF , Imam SZ ((2016) ) Protein kinases and Parkinson’s disease. Int J Mol Sci 17: , 1585. |
[35] | Bohush A , Niewiadomska G , Filipek A ((2018) ) Role of mitogen activated protein kinase signaling in Parkinson’s disease. Int J Mol Sci 19: , 2973. |
[36] | Navarro MN , Cantrell DA ((2019) ) Serine-threonine kinases in TCR signaling. Nat Immunol 15: , 808–814. |
[37] | Soares-Silva M , Diniz FF , Gomes GN , Bahia D ((2016) ) The mitogen-activated protein kinase (MAPK) pathway: Role in immune evasion by trypanosomatids. Front Microbiol 7: , 183. |
[38] | Mencke P , Hanss Z , Boussaad I , Sugier PE , Elbaz A , Kruger R ((2020) ) Bidirectional relation between Parkinson’s disease and glioblastoma multiforme. Front Neurol 11: , 898. |
[39] | Van Den Berg HA ((2018) ) Occam’s razor: From Ockham’s via moderna to modern data science. Sci Prog 101: , 261–272. |
[40] | Ma F , Zhang X , Yin KJ ((2020) ) MicroRNAs in central nervous system diseases: A prospective role in regulating blood-brain barrier integrity. Exp Neurol 323: , 113094. |
[41] | Mandrekar JN ((2010) ) Receiver operating characteristic curve in diagnostic test assessment. J Thorac Oncol 5: , 1315–1316. |