Axial Impairment Following Deep Brain Stimulation in Parkinson’s Disease: A Surgicogenomic Approach
Abstract
Background:
Postoperative outcome following deep brain stimulation (DBS) of the subthalamic nucleus is variable, particularly with respect to axial motor improvement. We hypothesized a genetic underpinning to the response to surgical intervention, termed “surgicogenomics”.
Objective:
We aimed to identify genetic variants associated with clinical heterogeneity in DBS outcome of Parkinson’s disease (PD) patients that could then be applied clinically to target selection leading to improved surgical outcome.
Methods:
Retrospective clinical data was extracted from 150 patient’s charts. Each individual was genotyped using the genome-wide NeuroX array tailored to study neurologic diseases. Genetic data were clustered based on surgical outcome assessed by comparing pre- and post-operative scores of levodopa equivalent daily dose and axial impairment at one and five years post-surgery. Allele frequencies were compared between patients with excellent vs. moderate/poor outcomes grouped using a priori defined cut-offs. We analyzed common variants, burden of rare coding variants, and PD polygenic risk score.
Results:
NeuroX identified 2,917 polymorphic markers at 113 genes mapped to known PD loci. The gene-burden analyses of 202 rare nonsynonymous variants suggested a nominal association of axial impairment with 14 genes (most consistent with CRHR1, IP6K2, and PRSS3). The strongest association with surgical outcome was detected between a reduction in levodopa equivalent daily dose and common variations tagging two linkage disequilibrium blocks with SH3GL2.
Conclusion:
Once validated in independent populations, our findings may be implemented to improve patient selection for DBS in PD.
INTRODUCTION
Deep brain stimulation (DBS) of the subthalamic nucleus (STN) is an increasingly common treatment for severe motor fluctuations and dyskinesias in Parkinson’s disease (PD) [1]. STN-DBS provides excellent control of appendicular cardinal signs of PD (e.g., tremor or rigidity); however, postoperative outcome can be variable in terms of axial motor symptoms. In fact, speech, gait and balance can worsen post STN-DBS due to the complex interplay of modifiable (e.g., medication/parameter adjustments) and unmodifiable (e.g., disease progression) factors.
The majority of DBS centers use the same criteria for patient selection (e.g., levodopa responsiveness) [2]. Yet, some studies report excellent control of gait [3], while others report gait impairment after DBS in ∼13%of patients [4]. Over a third of DBS failures can be ascribed to inappropriate indication for surgery [5], and a consensus review has suggested that careful patient selection is key to improving outcome [6]. While some predictors of poor axial outcome are starting to become clear, there is no consensus on the impact of age or disease duration. Furthermore, a limited number of inconclusive studies were seeking long-term predictors of surgical success [7]. Levodopa responsiveness has long been considered a valuable predictor of short-term outcome [8], but longer follow-up studies suggest otherwise [9]. Overall, there is considerable heterogeneity in both an individual’s PD phenotype and their response to DBS.
PD-related genetic loci undoubtedly play an imp-ortant role in phenotypic heterogeneity, including disease progression, development of motor complications, cognitive decline, and psychiatric disturbances [10, 11]. Furthermore, there is heterogeneity in the time to develop postural instability among monogenic forms of parkinsonism (e.g., progression-free survival from postural instability 10 years after disease onset was 97%in ATP13A2 and 50%in SNCA carriers) [12].
Pharmacogenomics established that genes play an important role in the response to pharmaceutical intervention. Here, we test the hypothesis that there is a genetic underpinning to a patient’s response to surgical intervention, which we have termed “surgicogenomics”. Specifically, we sought to identify genetic predictors of the development of axial signs post STN-DBS in PD. A precedent for the concept of “surgicogenomics” in STN-DBS in PD is supported by a meta-analysis that found carriers of mutations in LRRK2, GBA, and PRKN had different postoperative outcomes with respect to levodopa equivalent daily dose, activities of daily living, motor complications and cognitive functions [13]).
Specifically, our recent review found evidence of a good short-term outcome following STN-DBS in patients with pathogenic mutations in PRKN, LRRK2, and GBA. However, GBA-carriers developed earlier cognitive impairment and had a lesser reduction in medication, demonstrating that genetic background can influence response to STN-DBS [14]. Evidence in monogenic forms of PD gathered by systematic reviews have obvious limitations. Only one study tried to establish the predictive value of a few variants in two genes, including variants tagging a PD-associated SNCA haplotype [15].
In this study of 150 deeply phenotyped individuals, we aimed to identify loci associated with outcome after up to five years post STN-DBS, using the genome-wide NeuroX array tailored to study neurologic diseases [16].
MATERIALS AND METHODS
Participants
This study was performed in accordance with University Health Network Research Ethics Board approved protocol (UHN-REB 08-0615-AE). Info-rmed consent was obtained from patients who had undergone STN-DBS under the care of the Edmund J. Safra program in PD at Toronto Western Hospital. We recruited all consecutive patients fulfilling the inclusion criteria (e.g., diagnosis of PD, bilateral stimulation of STN, absence of post-surgical complications, or electrode misplacement) with satisfactory clinical information and adequate follow-up. All patients were operated at Toronto Western Hospital by the same neurosurgical team, in the same operating room, and under the supervision of one of three neurosurgeons (MH, SKK, or AML) in the period 1997–2014. All included subjects met the UK brain bank criteria for PD [17]. To minimize the variability introduced by the inherent error of surgical techniques, using neuroimaging techniques, we confirmed adequate electrode position using activation volume overlap with the STN and/or adjacent Zona Incerta. These data were available for 129 of 150 enrolled participants. Only 2 of 129 individuals were excluded due to poorly placed electrodes, as evidenced by a lack of overlap with either the STN or Zona Incerta. 21 participants lacking in electrode placement data remained in the cohort, as based on our observed 1.6%rate of poor placement, less than one of these 21 participants might be expected to have a poorly placed electrode. Thus, our final, cohort comprised 148 participants, the demographics of which are summarized in Table 1.
Table 1
Dataset characteristics
Cohort characteristics (N = 148) | Mean±SD (Min–Max) | Missing Data (N) |
Male/Female (N) | 108/40 | 0 |
Age at onset (y) | 45±8 (21–63) | 0 |
Age at surgery (y) | 58±7 (35–73) | 0 |
disease duration at surgery (y) | 11±5 (4–29) | 0 |
1-year follow-up (months) | 11±3 (4–21) | 0 |
5-year follow-up (months) | 60±7 (36–88) | 0 |
Pre-op UPDRS-III OFF L-dopa | 39±12 (11–86) | 0 |
Pre-op UPDRS-III ON L-dopa | 15±8 (3–50) | 2 |
L-dopa responsiveness (%) | 62±22 (–109–95) | 2 |
1-year UPDRS-III Total OFF L-dopa ON DBS | 21±10.1 (2–55)* (p < 0.05 vs. Pre-op UPDRS-III OFF L-dopa) | 1 |
DBS efficacy (1-year UPDRS-III Total OFF L-dopa ON DBS vs. Pre-op UPDRS-III Total OFF L-dopa) (%) | 42±29 (–82–93) | 1 |
5-year UPDRS-III Total OFF L-dopa ON DBS | 26±10.9 (3–55) *(p < 0.05 vs. Pre-op UPDRS-III OFF L-dopa) | 89 |
DBS efficacy (5-year UPDRS-III Total OFF L-dopa ON DBS vs. Pre-op UPDRS-III Total OFF L-dopa) (%) | 34±31 (-67–87) | 89 |
Pre-op LEDD | 1489±715 (0–3776) | 1 |
1-year LEDD | 798±512 (0–3750) *(p < 0.05 vs. Pre-op LEDD) | 4 |
1-year LEDD reduction (vs. Pre-op LEDD) (%) | 48±72(–775.0%–100.0) | 4 |
5-year LEDD | 863±595 (0–4200) *(p < 0.05 vs. Pre-op LEDD) | 1 |
5-year LEDD reduction (vs. Pre-op LEDD) (%) | 41109.6±(–1150.0%–100.0) | 0 |
STN-DBS outcome clusters (N) | ||
ST Axial UPDRS subscore (Excellent vs. moderate/poor) | 46 vs. 102 | 0 |
LT Axial UPDRS subscore (Excellent vs. moderate/poor) | 20 vs. 124 | 4 |
ST LEDD (high vs. moderate/low LEDD reduction) | 59 vs. 85 | 4 |
LT LEDD (high vs. moderate/low LEDD reduction) | 60 vs. 87 | 1 |
STN, subthalamic nucleus; DBS, deep brain stimulation; LEDD, levodopa equivalent daily dose (mg/day); UPDRS-III, motor section (part III) of the Unified Parkinson’s Disease Rating Scale; ST, short-term (∼1 year); LT, long-term (∼5 years).
Clinical data
Patients were assessed according to a standardized protocol in place at Toronto Western Hospital [18–20]. Briefly, this is inspired by the Core assessment program for surgical interventional therapies in Parkinson’s disease (CAPSIT-PD) criteria [2]). Patients were assessed in the morning at 9 am in the ‘practically defined off state’ (e.g., at least 12 h from the last dopaminergic agent, usually the night before) and video-evaluated using the Unified Parkinson’s Disease Rating Scale (UPDRS). For the on-medication condition, patients were challenged with the same dose used in the pre-operative phase (total levodopa equivalent doses of the first morning intake +20%, administered as crushed tablets of levodopa/carbidopa dissolved in carbonated water). Retrospective clinical data was extracted from patient charts, by investigators blinded to the genetic data, at two time points post STN-DBS: short-term (ST) follow up was ∼1 year post STN-DBS (11.4±3.0 months) and long-term (LT) follow up was ∼5 years post STN-DBS (60.5±7.3 months). The clinical data collected included Movement Disorders Society (UPDRS) parts I-IV [21] and levodopa equivalent daily dose (LEDD), which was calculated using standardized methods [22] (Table 1). DBS efficacy was calculated by comparing the percentage change in the UPDRS-III subtotal in the off-medication state pre surgery, with the UPDRS-III subtotal off medication with stimulation on post-surgery. To cluster genetic data, surgical outcome, assessed by comparing pre- and post-operative scores at both ST and LT follow up, were used to create four subgroups (Table 1), cut-offs for which were identified a priori based on the longstanding clinical experience of our center.
The percentage change of LEDD at baseline before STN-DBS surgery was calculated at ST and LT follow up. Compared to baseline, STN-DBS patients were subdivided into two groups: those with a high reduction (> 50%) in LEDD and those with a moderate/low reduction in LEDD (≤50%) (Table 1).
The UPDRS axial subscore was generated using a standardized calculation [23], namely the sum of items 13 (falling), 14 (freezing), and 25 (walking) of the UPDRS part II and items 29 (gait) and 30 (postural stability) of the UPDRS part III. The percentage change in the axial subscore in the off-medication state at baseline before STN-DBS surgery, compared to the off-medication on stimulation state at both ST and LT follow-up was calculated. Based on percentage change in axial subscore, STN-DBS patients were subdivided into two groups: those with an excellent outcome (> 70%reduction in axial subscore compared to baseline) and those with a moderate/poor outcome (≤70%reduction in axial subscore compared to baseline) (Table 1).
In total, our study focused on four different clusters of STN-DBS outcome: ST Axial subscore, LT Axial subscore, ST LEDD and LT LEDD (Table 1). For each of these outcomes, cluster scores were missing for only 2–7 patients. Other traditional outcome measures, such as total UPDRS, were not included in our study due to a lack of available data.
Analyses of NeuroX markers
Genomic DNA was isolated from blood using a QIAGEN kit and genotyped on the NeuroX array (Illumina Inc.) at the Clinical Genomics Centre (Toronto, Canada). NeuroX has the standard exome content of ∼240,000 variants, as well as ∼24,000 custom variants related to neurologic diseases, including ∼1,000 known mutations causing neurodegenerative diseases and ∼10,000 single nucleotide polymorphisms (SNPs) tagging significant loci detected by genome-wide association studies (GWASs). Genotype data obtained by NeuroX was loaded to Gen-omeStudio (Illumina Inc.), which confirmed a call rate of > 0.96 for all samples, and GenTrain score of > 0.35 for all SNPs. NeuroX markers with GenTrain scores between 0.35–0.70 were visually inspected and those with a cluster separation score < 0.2 were removed [24]. ANNOVAR was used for the functional annotation of NeuroX markers including their associated protein changes and the potential impact of variants on protein function [25], as well as to obtain the frequency of the NeuroX markers in the Genome Aggregation Database (gnomAD v.1). For the association study, we extracted NeuroX markers mapped to both Mendelian PD genes and PD loci implicated by the most recent GWAS [26]. In total, we analyzed 113 PD-related genes (Supplementary Table 1).
NeuroX genotypes were converted to PLINK in-put files to perform chi-square association tests and obtain p-values (adjusted for multiple testing). To evaluate the potential impact of genetic variants on STN-DBS outcome, allele frequencies were com-pared between patients with excellent vs. moderate/poor outcomes (as defined above). Variants with nominal associations in different outcome clusters (p < 0.05) were subjected to the Tagger function of the Haploview program (aggressive tagging; using 2- and 3-marker haplotypes) based on genotype data from the study participants. Tagging SNPs were further analyzed for association with the four outcome clusters.
For each STN-DBS outcome cluster, we assessed the burden of rare coding variants with minor allele frequencies < 0.01 in the gnomAD exome subset (v1) (https://gnomad.broadinstitute.org/). We used the Sequencing Kernel Association Test (SKAT) package, including three tests: 1) SKAT (most powerful when most variants in the target region are non-causal or the effects of causal variants are in different directions); 2) burden (more suitable when most variants have effects on the phenotype in the same direction); and 3) SKATO optimized for both scenarios [27]. The combined p-values for all rare variants of each gene are reported.
Polygenic risk score (PRS) was calculated for each participant with PRSice v 2.2.11, without linkage disequilibrium (LD) clumping or P thresholding. We used the summary statistics of 1,805 SNPs from the latest PD GWAS, which were shown to best differentiate patients and controls [26]. Using principal component analysis with HapMap 3, we restricted this analysis to individuals of European ancestry (n = 124), since the original PRS was calculated for the European population. To determine the genotype of missing variants, we performed imputation with the Michigan Imputation Server on the Haplotype Reference Consortium (Version r1.1 2016) using Minimac4 and Eagle v2.4. Out of the 1,805 SNPs, only 1,667 variants were available with an imputation quality (r2) above 0.8.
RESULTS
Clinical data are summarized in Table 1. Levodopa responsiveness pre-operatively was 62±22%. One year after surgery, STN-DBS efficacy and medication reduction were calculated to be 42%and 48%, respectively (both p < 0.0001 vs. pre-operative values).
Annotation of the NeuroX data revealed 5,128 markers located at 113 genes mapped to known PD loci (Supplementary Table 1). Their genotypes were analyzed among the 150 PD patients who had undergone STN-DBS. In total, 2,917 of these markers were polymorphic in our PD cohort, including 373 coding variants, 202 of which were rare nonsynonymous variants with a minor allele frequency < 0.01 in the gnomAD exome database. Notably, 15 of them (Table 2) had combined annotation dependent depletion scores > 30 (representing the top 0.1%of deleterious variants in the human genome), including a pathogenic mutation in LRRK2 (p.G2019S).
Table 2
Characteristics of patients carrying nonsynonymous heterozygous variants with a CADD score > 30 or a stop gain variant
Gene | Variant | SNP ID | CADD | Minor allele frequency | Patient characteristics | STN-DBS outcome | ||||||
PD cohort | gnom | ID | sex | age at | ST Axial | LT Axial | ST LEDD | LT LEDD | ||||
AD-exome | onset | |||||||||||
LRRK2 | p.G2019S | rs34637584 | 35 | 0.003 | 0.0005 | 10114 | F | 47 | E | E | E | E |
VPS13C | p.R3564H | rs116228685 | 35 | 0.003 | 0.0003 | 10538 | M | 52 | M | M | M | M |
DNAH17 | p.G4044S | rs199692490 | 35 | 0.003 | 0.0004 | 10239 | M | 39 | E | E | E | E |
UBAP2 | p.R174Q | rs79607078 | 34 | 0.003 | 0.0043 | 10478 | M | 42 | E | na | M | M |
HIP1R | p.A911T | rs141813189 | 34 | 0.003 | 0.0012 | 10537 | M | 37 | M | E | M | M |
NEK1 | p.R261H | rs200161705 | 34 | 0.007 | 0.0024 | 10446 | M | 51 | M | M | M | M |
10739 | M | 33 | M | M | M | M | ||||||
PRKN | p.R126W | rs34424986 | 34 | 0.007 | 0.0019 | 10487 | M | 48 | M | M | M | M |
10550 | M | 44 | M | M | E | E | ||||||
CNTN1 | p.R966C | rs150734960 | 34 | 0.007 | 0.0008 | 10446 | M | 51 | M | M | M | M |
10792 | M | 34 | M | M | M | E | ||||||
HIP1R | p.R564W | rs140743610 | 33 | 0.003 | 0.001 | 10681 | M | 47 | M | M | E | M |
SIPA1L2 | p.P714S | rs200216436 | 32 | 0.003 | 0.0003 | 10715 | M | 33 | M | M | E | M |
SH3GL2 | p.A221T | rs760865937 | 32 | 0.003 | 0.0002 | 10487 | M | 48 | M | M | M | M |
BRIP1 | p.R264W | rs28997569 | 32 | 0.003 | 0.0008 | 10313 | M | 30 | M | M | M | M |
KCNIP3 | p.R39H | rs35516857 | 32 | 0.01 | 0.0022 | 10487 | M | 48 | M | M | M | M |
10442 | F | 45 | E | M | E | E | ||||||
10698 | F | 46 | M | M | M | M | ||||||
DNAH17 | p.F2520V | rs200203879 | 32 | 0.003 | 0.0025 | 10316 | F | 61 | E | M | E | E |
RIT2 | p.R182H | rs148544378 | 31 | 0.01 | 0.009 | 10434 | M | 43 | M | M | M | M |
10338 | M | 55 | M | M | E | M | ||||||
10595 | M | 34 | E | E | E | E | ||||||
VPS13C | p.R3609X | rs138846118 | 49 | 0.003 | 0.0002 | 10413 | M | 51 | M | M | E | E |
CADD, combined annotation dependent depletion; PD, Parkinson’s disease; MAF, Minor allele frequency; Sex, F –female, M –male; STN, subthalamic nucleus; DBS, deep brain stimulation; ST, short-term (∼1 year); LT, long-term (∼5 years); Axial, Axial subscore of the Unified Parkinson’s Disease Rating Scale; LEDD, levodopa equivalent daily dose (mg/day); STN-DBS outcome, E –excellent, M –moderate/poor; na, not available.
Using the SKAT package, we investigated the gene-by-gene joint burden of rare variants on STN-DBS outcome. Nominally significant findings were detected for 14 genes (Table 3), none remained significant after correction for multiple testing. However, three genes showed nominally significant results by all three SKAT tests, including CRHR1 (NM_001145146.2) and IP6K2 (NM_001005909.2) for the ST Axial subscore; as well as PRSS3 for the LT Axial subscore.
Table 3
Results of the joint burden analysis of rare variants on STN-DBS outcome. The Burden, SKATO, and SKAT test of the SKAT package were performed for the four DBS outcome groups (ST Axial subscore, LT Axial subscore, ST LEDD, and LT LEDD). Three genes (CRHR1, IP6K2, PRSS3) showed nominally significant p-values for the excellent outcome by all 3 tests (bold-typed)
Gene | Burden | SKATO | SKAT | |||||||||
ST LEDD | ST Axial | LT LEDD | LT Axial | ST LEDD | ST Axial | LT LEDD | LT Axial | ST LEDD | ST Axial | LT LEDD | LT Axial | |
CHD9 | 0.040 | 0.820 | 0.200 | 0.520 | 0.063 | 0.730 | 0.300 | 0.520 | 0.140 | 0.560 | 0.630 | 0.520 |
CRHR1 | 0.070 | 0.015 | 0.370 | 0.089 | 0.067 | 0.015 | 0.370 | 0.090 | 0.070 | 0.015 | 0.370 | 0.090 |
HIP1R | 0.630 | 0.460 | 0.230 | 0.150 | 0.750 | 0.660 | 0.370 | 0.080 | 0.567 | 0.480 | 0.400 | 0.044 |
IP6K2 | 0.230 | 0.009 | 0.350 | 0.480 | 0.240 | 0.009 | 0.350 | 0.480 | 0.240 | 0.009 | 0.350 | 0.480 |
ITGA8 | 0.790 | 0.920 | 0.035 | 0.480 | 0.580 | 0.520 | 0.060 | 0.650 | 0.420 | 0.370 | 0.220 | 0.920 |
KCNS3 | 0.230 | 0.033 | 0.240 | 0.570 | 0.330 | 0.050 | 0.330 | 0.700 | 0.500 | 0.100 | 0.500 | 0.850 |
LRRK2 | 0.270 | 0.160 | 0.130 | 0.140 | 0.420 | 0.260 | 0.210 | 0.060 | 0.450 | 0.420 | 0.320 | 0.036 |
MAP4K4 | 0.240 | 0.350 | 0.783 | 0.140 | 0.330 | 0.460 | 0.460 | 0.060 | 0.500 | 0.640 | 0.340 | 0.043 |
MED12L | 0.240 | 0.550 | 0.783 | 0.140 | 0.330 | 0.350 | 0.460 | 0.060 | 0.500 | 0.260 | 0.340 | 0.043 |
PAM | 0.440 | 0.830 | 0.673 | 0.220 | 0.570 | 0.054 | 0.850 | 0.340 | 0.390 | 0.034 | 0.780 | 0.900 |
PRSS3 | 0.150 | 0.920 | 0.150 | 0.008 | 0.150 | 0.920 | 0.150 | 0.008 | 0.150 | 0.920 | 0.150 | 0.008 |
RIMS1 | 0.090 | 0.550 | 0.086 | 0.140 | 0.130 | 0.350 | 0.130 | 0.060 | 0.230 | 0.260 | 0.230 | 0.043 |
SMPD1 | 0.690 | 0.051 | 0.710 | 0.160 | 0.560 | 0.087 | 0.660 | 0.090 | 0.380 | 0.130 | 0.460 | 0.044 |
TMEM175 | 0.240 | 0.033 | 0.240 | 0.140 | 0.330 | 0.050 | 0.330 | 0.060 | 0.500 | 0.100 | 0.510 | 0.043 |
DBS, deep brain stimulation; STN, subthalamic nucleus; Axial, Axial subscore of the Unified Parkinson’s Disease Rating Scale; LEDD, levodopa equivalent daily dose (mg/day); ST, short-term (∼1 year); LT, long-term (∼5 years).
The chi-square association test between each pol-ymorphic marker and the four outcome clusters identified 590 nominally significant signals (Fig. 1A, Supplementary Table 2), none of which survived Bonferroni correction (p < 0.00002). The strongest association was detected between the LT LEDD outcome and an intronic SNP (rs10810812) in SH3GL2 (p = 0.00028), as well as between ST LEDD outcome and 13 SNPs located in a ∼150 Kb genomic region within intron 1 of SH3GL2 (Chr9:17586101-17735083) (p = 0.001). Investigation of the LD-structure of the SH3GL2 locus (Supplementary Figure 1) showed that the region tagged by 13 SNPs belongs to a single LD-block, which includes the GWAS-significant SNP rs10756907 [26]. In contrast, rs10810812 belongs to a different LD-block, containing another GWAS-significant SNP rs13294100 [26]. To identify independent signals, all nominally significant variations were analyzed using the Haploview Tagger function, which revealed 153 variants tagging separate blocks (100%of alleles at r2 > = 0.8 were captured with a mean max r2 of 0.96). Among them, rs10810812 in SH3GL2 showed a significant association of the G-allele with a high LT LEDD outcome even after Bonferroni correction (adjusted p = 0.043) (Fig. 1B).
Fig. 1
Manhattan plot. A) The association study between the four STN-DBS outcome clusters and 2917 polymorphic NeuroX markers (mapped to known PD loci) identified 590 nominally significant signals. The strongest nominal association (uncorrected p = 0.00028) was detected between the LT LEDD outcome and rs10810812 in SH3GL2, as well as between the ST LEDD outcome and 13 SNPs located in a ∼150Kb genomic region (Chr9:17586101-17735083). B) To reveal the independent association signals, 153 tagging variants selected by Haploview were tested for association with STN-DBS outcome clusters. Among these tagging variants, the rs10810812 SNP in SH3GL2 showed a significant association with LT LEDD outcome after Bonferroni correction (adjusted p = 0.043). DBS, deep brain stimulation; LEDD, levodopa equivalent daily dose (mg/day); LT, long-term (∼5 years); ST, short-term (~1 year); STN, subthalamic nucleus.
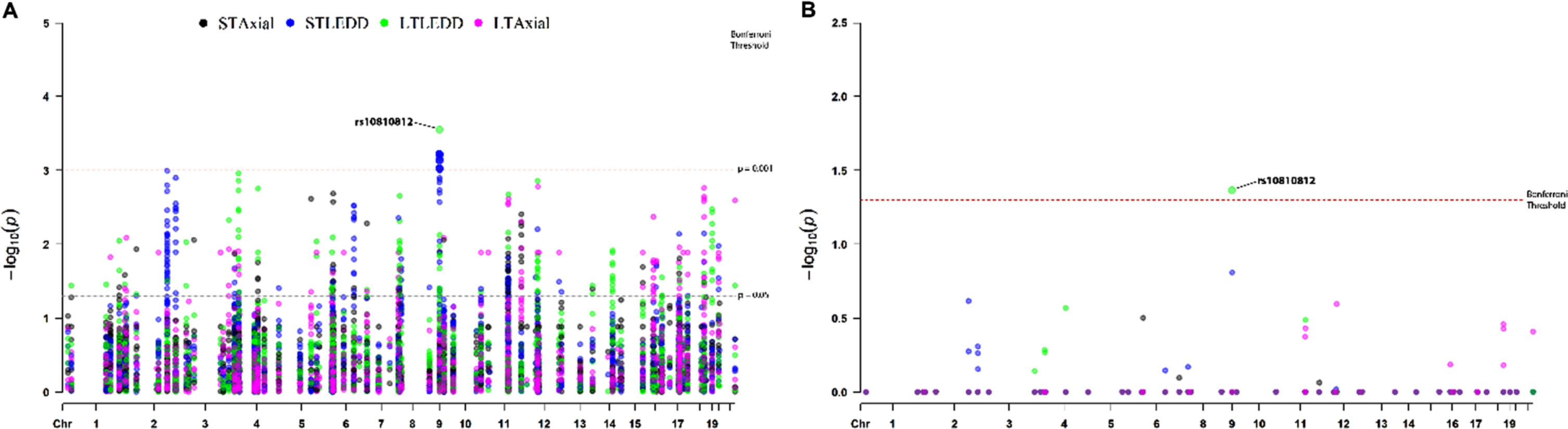
To test for associations between PRS and the four outcome clusters, we used a logistic regression approach with and without adjustment for sex, age at surgery, and disease duration at the time of surgery. No statistically significant associations were found (Table 4).
Table 4
Results of the association study between polygenic risk score and the four outcome clusters, including results adjusted for sex, age at surgery, and disease duration at surgery
Adjusted values | ||||||
Outcome | Beta | SE | P | Beta | SE | P |
ST LEDD | 0.04 | 0.19 | 0.82 | –0.02 | 0.20 | 0.93 |
ST Axial | –0.24 | 0.20 | 0.22 | –0.31 | 0.21 | 0.13 |
LT LEDD | –0.07 | 0.19 | 0.72 | –0.09 | 0.19 | 0.63 |
LT Axial | 0.14 | 0.24 | 0.56 | 0.14 | 0.25 | 0.59 |
Axial, axial subscore of the Unified Parkinson’s Disease Rating Scale; LEDD, levodopa equivalent daily dose (mg/day); ST, short-term (∼1 year); LT, long-term (∼5 years); SE, standard error.
DISCUSSION
Demand for DBS in PD has increased exponentially in recent years, and this trend is likely to con-tinue with the suggestion that patients should undergo surgery sooner [28]. However, three decades after its first clinical application, the selection of DBS candidates is still far from optimal as it remains reliant upon clinical features that lack the specificity and granularity to capture the highly heterogeneous neurobiological underpinnings of PD [29]. This failure is particularly relevant to two aspects, first the ability of the brain to tolerate the procedure (e.g., clinical deterioration following surgery); and second a lack of accurate predictors of long-term outcome. For example, the assumption that patients over 70 should not undergo STN-DBS, in light of the reported post-surgical axial motor deterioration [30], has been challenged by successful procedures in elderly patients, indicating that age per se is not a reliable proxy of brain frailty. As for long-term outcome, recent—not yet replicated—data point to a role for frontal cognitive impairment, indicating that a more widespread pathology at baseline might predict a faster disease progression [31]. Reliable indicators of surgical outcome would dramatically improve the application of this increasingly popular treatment for PD.
Based on the limited data seen in monogenetic forms of dystonia and PD [32], it has been suggested that genetic factors might be taken into account to select DBS candidates. So far, only a single study has attempted to establish the link between STN-DBS outcome and a few variants in two PD genes. It reported that in a cohort of 85 patients a more favorable motor outcome two years post-surgery was associated with two SNPs tagging a PD-related haplotype at the 3’ untranslated region of SNCA, strongly supporting the evaluation of genetic biomarkers in surgical cohorts [15]. Importantly, in our cohort, we found that both SNPs were nominally associated with an improved ST Axial subscore (p = 0.018 for rs356219 and p = 0.029 for rs356220).
Our study aimed to correlate NeuroX variants with detailed post-operative clinical data in our deeply phenotyped cohort, to identify loci associated with STN-DBS outcome in the hope that eventually such knowledge could be applied clinically to target patient selection for STN-DBS, leading to improved surgical outcome. Importantly, patients included in this study are representative of the typical population undergoing STN-DBS and likewise, the effect of surgery on motor signs and LEDD reduction is in keeping with the extensive literature published to date [33, 34]. Our analysis suggested three nominally significant candidate genes (CRHR1, IP6K2, and PRSS3) that may influence the development of axial symptoms post STN-DBS and one gene (SH3GL2) linked to a reduction of dopamine replacement therapy post STN-DBS.
CRHR1 was associated with axial symptoms one year post DBS. It encodes a corticotropin-releasing hormone receptor that has previously been associated with PD in two large GWASs [26, 35]. CRHR1 is widely expressed in the brain and its stimulation in the pituitary gland leads to the release of glucocorticoids [36]. There is growing appreciation for the role of glucocorticoids and their receptors in PD, with increased signaling worsening symptoms and enhancing neurodegeneration in experimental models of PD [37].
Our analysis also suggests an association between axial symptoms one year post DBS and IP6K2, which was previously linked to PD in two GWASs [26, 38]. IP6K2 encodes Inositol hexakisphosphate kinase 2 that has been shown to mediate apoptosis [39]. IP6K2 is abundantly expressed in the brain and has been implicated in the neurodegenerative process of several diseases. In amyotrophic lateral sclerosis, IP6K2 has been shown to promote cell death associated with TDP-43 aggregation [40]. Similarly, activation of IP6K2 has been associated with pathogenicity in Huntington’s disease [39]. As both CRHR1 and IP6K2 are implicated in neurodegenerative processes, it is possible that they influence the development of axial symptoms post DBS by an effect on disease progression.
Our data suggested that axial symptoms 5 years post DBS were linked to PRSS3, encoding the try-psinogen protease serine 3, which is highly expressed in both the pancreas and the brain. PRSS3 was identified by GWAS through quantitative trait locus mapping (nominating the gene under the disease linked LD-block based on the functional data) [26]. Also, four PRSS3 variants were noted in a South African PD population using exome sequencing [41]. Little is known about trypsinogens and PD; however, trypsin-2 mRNA has been found in PD substantia nigra where it has been associated with levodopa-induced dyskinesia and psychosis [42]. Thus, it is plausible that trypsinogen signaling might impact PD-related symptoms.
A tempting but speculative view concerns the role of these three genes in inflammatory and apoptotic responses of the brain, an area receiving increasing attention in PD [43]. Interestingly, recent animal data indicate that DBS may act as a regulator of the inflammatory response in PD states, attenuating classical activation of astrocytes and cytokine induction [44], thus confirming the hypothesis of a preliminary study in PD patients undergoing DBS [45]. On the other hand, autopsy data have shown inflammatory responses around the electrodes of DBS patients [46] and systemic inflammation has been reported to impair brain’s ability to tolerate a DBS procedure [47]. Taken together, these notions might indicate that inflammatory response may be associated with the post-surgical decline of some PD patients undergoing STN-DBS, possibly indicating that CRHR1, IP6K2, and PRSS3 are involved in such a response.
Finally, our study points to an association between LEDD post STN-DBS and common SNPs in SH3GL2 encoding endophilin A1, a known risk factor for PD. The observed association with endophilin A1 could stem from its contribution to synaptic vesicle exocytosis influencing vulnerability of dopaminergic neurons in PD [38, 48]. Alternatively, since LEDD reduction is a proxy of overall DBS outcome, SH3GL2 might have a more general role in surgical efficacy.
Baseline clinical characteristics for patients with and without the observed rare variants in IP6K2, PRSS3, and CRHR1 and between patients with AA vs. GG genotype of rs10810812 in SH3GL2, were not significantly different, other than one nominally significant result that was observed for older age of onset in IP6K2-carriers (Supplementary Table 3). Thus, based on the available clinical data, these identified variants do not appear to have different baseline clinical characteristics that could help with patient selection. Indeed, this finding emphasizes the potential for genetic testing to detect possible markers to inform patient selection.
We appreciate that the conclusions drawn from our study are subject to limitations. First, although one of the largest DBS cohort studies to date, we recognize for a genetic cohort the sample size is relatively small. Second, the use of arbitrary cut-offs to categorize patients has obvious limitations that we tried to minimize with their a priori defini-tion. In spite of these limitations, our study of 150 STN-DBS patients evaluated at a single center with a standardized battery up to 5 years after surgery replicated the findings of two SNCA SNPs (rs356219 and rs356220) previously associated with neurostimulation outcome [15]. In addition, high effect sizes were observed for several nominally significant variants. For example, rs35507033 in LRRK2 for ST LEDD outcome, rs75638861 for ST Axial subscore, and several variants for LT Axial subscore outcome (e.g., rs184013125, rs142022985, rs117922937, rs146051626). Although our findings should be considered preliminary in nature, we hope our work encourages future studies to validate our findings in independent cohorts. Such future experiments might also address epigenetic modifications that could dictate surgical outcomes and investigate the effects of DBS itself on DNA methylation and the brain transcriptome. Finally, NeuroX is a commonly used array in genetic investigation of neurodegenerative diseases; however, it was designed prior to the recent GWASs that identified novel PD loci. Therefore, future studies could have more power using the more updated platform designed with a focus on neurodegenerative disorders (e.g., Illumina’s Neuro Booster).
CONCLUSIONS
Our study has enhanced understanding of the mechanisms responsible for the successes of DBS and gene-phenotype correlations in PD and adds to growing calls to evaluate genetic biomarkers in surgical cohorts. We emphasize the potential for genetic testing to detect markers to inform patient selection. To this end, standardized assessment of our future surgical population will represent an invaluable tool. Although outside the scope of the present study, it is critical that these findings are validated in independent populations to pave the way for the implementation of new recommendations with respect to patient selection and/or target selection (e.g., STN vs. globus pallidus interna) for DBS in PD.
ACKNOWLEDGMENTS
The authors would like to acknowledge the patients who participated in this research, as well as the McLaughlin and Blidner Family Foundations and funder of the Chair in Neuromodulation and Multi-Disciplinary Care at the University of Toronto and University Health Network for their generous support. This study was funded by the McLaughlin, Blidner Family Foundations, and the Canadian Consortium on Neurodegeneration in Aging.
CONFLICT OF INTEREST
NPV, AF, and ER received a grant from the McLaughlin Foundation to support this work. AF rec-eived research support and/or speaker honoraria from Abbott, Brainlab, Boston Scientific, and Medtronic; he is a consultant for Abbott, Boston Scientific, Cere Gate, INBRAIN Neuroelectronics, and Medtronic.
SUPPLEMENTARY MATERIAL
[1] The supplementary material is available in the electronic version of this article: https://dx.doi.org/10.3233/JPD-212730.
REFERENCES
[1] | Fasano A , Daniele A , Albanese A ((2012) ) Treatment of motor and non-motor features of Parkinson’s disease with deep brain stimulation. Lancet Neurol 11: , 429–442. |
[2] | Defer GL , Widner H , Marié RM , Rémy P , Levivier M ((1999) ) Core assessment program for surgical interventional therapies in Parkinson’s disease (CAPSIT-PD). Mov Disord 14: , 572–584. |
[3] | Barbe MT , Tonder L , Krack P , Debû B , Schüpbach M , Paschen S , Dembek TA , Kühn AA , Fraix V , Brefel-Courbon C , Wojtecki L , Maltête D , Damier P , Sixel-Döring F , Weiss D , Pinsker M , Witjas T , Thobois S , Schade-Brittinger C , Rau J , Houeto JL , Hartmann A , Timmermann L , Schnitzler A , Stoker V , Vidailhet M , Deuschl G ((2020) ) Deep brain stimulation for freezing of gait in Parkinson’s disease with early motor complications. Mov Disord 35: , 82–90. |
[4] | Sharma VD , Lyons KE , Nazzaro JM , Pahwa R ((2019) ) Deep brain stimulation of the subthalamic nucleus in Parkinson’s disease patients over 75 years of age. J Neurol Sci 399: , 57–60. |
[5] | Okun MS , Tagliati M , Pourfar M , Fernandez HH , Rodriguez RL , Alterman RL , Foote KD ((2005) ) Management of referred deep brain stimulation failures: A retrospective analysis from 2 movement disorders centers. Arch Neurol 62: , 1250–1255. |
[6] | Bronstein JM , Tagliati M , Alterman RL , Lozano AM , Volkmann J , Stefani A , Horak FB , Okun MS , Foote KD , Krack P , Pahwa R , Henderson JM , Hariz MI , Bakay RA , Rezai A , Marks WJ Jr., Moro E , Vitek JL , Weaver FM , Gross RE , DeLong MR ((2011) ) Deep brain stimulation for Parkinson disease: An expert consensus and review of key issues. Arch Neurol 68: , 165. |
[7] | Fasano A , Romito LM , Daniele A , Piano C , Zinno M , Bentivoglio AR , Albanese A ((2010) ) Motor and cognitive outcome in patients with Parkinson’s disease 8 years after subthalamic implants. Brain 133: , 2664–2676. |
[8] | Kleiner-Fisman G , Herzog J , Fisman DN , Tamma F , Lyons KE , Pahwa R , Lang AE , Deuschl G ((2006) ) Subthalamic nucleus deep brain stimulation: Summary and meta-analysis of outcomes. Mov Disord 21 Suppl 14: , S290–304. |
[9] | Piboolnurak P , Lang AE , Lozano AM , Miyasaki JM , Saint-Cyr JA , Poon YY , Hutchison WD , Dostrovsky JO , Moro E ((2007) ) Levodopa response in long-term bilateral subthalamic stimulation for Parkinson’s disease. Mov Disord 22: , 990–997. |
[10] | Puschmann A ((2013) ) Monogenic Parkinson’s disease and parkinsonism: Clinical phenotypes and frequencies of known mutations. Parkinsonism Relat Disord 19: , 407–415. |
[11] | Fagan ES , Pihlstrøm L ((2017) ) Genetic risk factors for cognitive decline in Parkinson’s disease: A review of the literature. Eur J Neurol 24: , 561–e520. |
[12] | Marsili L , Vizcarra JA , Sturchio A , Dwivedi AK , Keeling EG , Patel D , Mishra M , Farooqi A , Merola A , Fasano A , Mata IF , Kauffman MA , Espay AJ (2020) When does postural instability appear in monogenic parkinsonisms? An individual-patient meta-analysis. J Neurol. |
[13] | Artusi CA , Dwivedi AK , Romagnolo A , Pal G , Kauffman M , Mata I , Patel D , Vizcarra JA , Duker A , Marsili L , Cheeran B , Woo D , Contarino MF , Verhagen L , Lopiano L , Espay AJ , Fasano A , Merola A ((2019) ) Association of subthalamic deep brain stimulation with motor, functional, and pharmacologic outcomes in patients with monogenic Parkinson disease: A systematic review and meta-analysis. JAMA Netw Open 2: , e187800. |
[14] | de Oliveira LM , Barbosa ER , Aquino CC , Munhoz RP , Fasano A , Cury RG ((2019) ) Deep brain stimulation in patients with mutations in Parkinson’s disease-related genes: A systematic review. Mov Disord Clin Pract 6: , 359–368. |
[15] | Weiss D , Herrmann S , Wang L , Schulte C , Brockmann K , Plewnia C , Gasser T , Sharma M , Gharabaghi A , Krüger R ((2016) ) Alpha-synuclein gene variants may predict neurostimulation outcome. Mov Disord 31: , 601–603. |
[16] | Nalls MA , Bras J , Hernandez DG , Keller MF , Majounie E , Renton AE , Saad M , Jansen I , Guerreiro R , Lubbe S , Plagnol V , Gibbs JR , Schulte C , Pankratz N , Sutherland M , Bertram L , Lill CM , DeStefano AL , Faroud T , Eriksson N , Tung JY , Edsall C , Nichols N , Brooks J , Arepalli S , Pliner H , Letson C , Heutink P , Martinez M , Gasser T , Traynor BJ , Wood N , Hardy J , Singleton AB ((2015) ) NeuroX, a fast and efficient genotyping platform for investigation of neurodegenerative diseases. Neurobiol Aging 36: , 1605.e1607–1612. |
[17] | Hughes AJ , Daniel SE , Kilford L , Lees AJ ((1992) ) Accuracy of clinical diagnosis of idiopathic Parkinson’s disease: A clinico-pathological study of 100 cases. J Neurol Neurosurg Psychiatry 55: , 181–184. |
[18] | Honey CR , Hamani C , Kalia SK , Sankar T , Picillo M , Munhoz RP , Fasano A , Panisset M ((2017) ) Deep brain stimulation target selection for Parkinson’s disease. Can J Neurol Sci 44: , 3–8. |
[19] | Munhoz RP , Picillo M , Fox SH , Bruno V , Panisset M , Honey CR , Fasano A ((2016) ) Eligibility criteria for deep brain stimulation in Parkinson’s disease, tremor, and dystonia. Can J Neurol Sci 43: , 462–471. |
[20] | Panisset M , Picillo M , Jodoin N , Jodoin YY , Valencia-Mizrachi A , Fasano A , Munhoz R , Honey CR , ((2017) ) Establishing a standard of care for deep brain stimulation centers in Canada. Can J Neurol Sci 44: , 132–138. |
[21] | Fahn S , Elton RL ((1987) ) Unified Parkinsons Disease Rating Scale, MacMillan Health Care Information, New Jersey. |
[22] | Tomlinson CL , Stowe R , Patel S , Rick C , Gray R , Clarke CE ((2010) ) Systematic review of levodopa dose equivalency reporting in Parkinson’s disease. Mov Disord 25: , 2649–2653. |
[23] | Bloem BR , Marinus J , Almeida Q , Dibble L , Nieuwboer A , Post B , Ruzicka E , Goetz C , Stebbins G , Martinez-Martin P , Schrag A ((2016) ) Measurement instruments to assess posture, gait, and balance in Parkinson’s disease: Critique and recommendations. Mov Disord 31: , 1342–1355. |
[24] | Guo Y , He J , Zhao S , Wu H , Zhong X , Sheng Q , Samuels DC , Shyr Y , Long J ((2014) ) Illumina human exome genotyping array clustering and quality control. Nat Protoc 9: , 2643–2662. |
[25] | Ghani M , Lang AE , Zinman L , Nacmias B , Sorbi S , Bessi V , Tedde A , Tartaglia MC , Surace EI , Sato C , Moreno D , Xi Z , Hung R , Nalls MA , Singleton A , St George-Hyslop P , Rogaeva E ((2015) ) Mutation analysis of patients with neurodegenerative disorders using NeuroX array. Neurobiol Aging 36: , 545.e549–514. |
[26] | Nalls MA , Blauwendraat C , Vallerga CL , Heilbron K , Bandres-Ciga S , Chang D , Tan M , Kia DA , Noyce AJ , Xue A , Bras J , Young E , von Coelln R , Simón-Sánchez J , Schulte C , Sharma M , Krohn L , Pihlstrøm L , Siitonen A , Iwaki H , Leonard H , Faghri F , Gibbs JR , Hernandez DG , Scholz SW , Botia JA , Martinez M , Corvol JC , Lesage S , Jankovic J , Shulman LM , Sutherland M , Tienari P , Majamaa K , Toft M , Andreassen OA , Bangale T , Brice A , Yang J , Gan-Or Z , Gasser T , Heutink P , Shulman JM , Wood NW , Hinds DA , Hardy JA , Morris HR , Gratten J , Visscher PM , Graham RR , Singleton AB ((2019) ) Identification of novel risk loci, causal insights, and heritable risk for Parkinson’s disease: A meta-analysis of genome-wide association studies. Lancet Neurol 18: , 1091–1102. |
[27] | Lee S , Wu MC , Lin X ((2012) ) Optimal tests for rare variant effects in sequencing association studies. Biostatistics 13: , 762–775. |
[28] | Kalakoti P , Ahmed O , Bollam P , Missios S , Wilden J , Nanda A ((2015) ) Predictors of unfavorable outcomes following deep brain stimulation for movement disorders and the effect of hospital case volume on outcomes: An analysis of 33, 642 patients across 234 US hospitals using the National (Nationwide) Inpatient Sample from 2002 to 2011. Neurosurg Focus 38: , E4. |
[29] | Geraedts VJ , Feleus S , Marinus J , van Hilten JJ , Contarino MF ((2020) ) What predicts quality of life after subthalamic deep brain stimulation in Parkinson’s disease? A systematic review. Eur J Neurol 27: , 419–428. |
[30] | Russmann H , Ghika J , Villemure JG , Robert B , Bogousslavsky J , Burkhard PR , Vingerhoets FJ ((2004) ) Subthalamic nucleus deep brain stimulation in Parkinson disease patients over age 70 years. Neurology 63: , 1952–1954. |
[31] | Cavallieri F , Fraix V , Bove F , Mulas D , Tondelli M , Castrioto A , Krack P , Meoni S , Schmitt E , Lhommée E , Bichon A , Pélissier P , Chevrier E , Kistner A , Seigneuret E , Chabardès S , Moro E ((2021) ) Predictors of long-term outcome of subthalamic stimulation in Parkinson disease. Ann Neurol 89: , 587–597. |
[32] | Panov F , Tagliati M , Ozelius LJ , Fuchs T , Gologorsky Y , Cheung T , Avshalumov M , Bressman SB , Saunders-Pullman R , Weisz D , Alterman RL ((2012) ) Pallidal deep brain stimulation for DYT6 dystonia. J Neurol Neurosurg Psychiatry 83: , 182–187. |
[33] | Krack P , Volkmann J , Tinkhauser G , Deuschl G ((2019) ) Deep brain stimulation in movement disorders: From experimental surgery to evidence-based therapy. Mov Disord 34: , 1795–1810. |
[34] | Ligaard J , Sannæs J , Pihlstrøm L ((2019) ) Deep brain stimulation and genetic variability in Parkinson’s disease: A review of the literature. NPJ Parkinsons Dis 5: , 18. |
[35] | Bandres-Ciga S , Ahmed S , Sabir MS , Blauwendraat C , Adarmes-Gómez AD , Bernal-Bernal I , Bonilla-Toribio M , Buiza-Rueda D , Carrillo F , Carrión-Claro M , Gómez-Garre P , Jesús S , Labrador-Espinosa MA , Macias D , Méndez-Del-Barrio C , Periñán-Tocino T , Tejera-Parrado C , Vargas-González L , Diez-Fairen M , Alvarez I , Tartari JP , Buongiorno M , Aguilar M , Gorostidi A , Bergareche JA , Mondragon E , Vinagre-Aragon A , Croitoru I , Ruiz-Martínez J , Dols-Icardo O , Kulisevsky J , Marín-Lahoz J , Pagonabarraga J , Pascual-Sedano B , Ezquerra M , Cámara A , Compta Y , Fernández M , Fernández-Santiago R , Muñoz E , Tolosa E , Valldeoriola F , Gonzalez-Aramburu I , Sanchez Rodriguez A , Sierra M , Menéndez-González M , Blazquez M , Garcia C , Suarez-San Martin E , García-Ruiz P , Martínez-Castrillo JC , Vela-Desojo L , Ruz C , Barrero FJ , Escamilla-Sevilla F , Mínguez-Castellanos A , Cerdan D , Tabernero C , Gomez Heredia MJ , Perez Errazquin F , Romero-Acebal M , Feliz C , Lopez-Sendon JL , Mata M , Martínez Torres I , Kim JJ , Dalgard CL , Brooks J , Saez-Atienzar S , Gibbs JR , Jorda R , Botia JA , Bonet-Ponce L , Morrison KE , Clarke C , Tan M , Morris H , Edsall C , Hernandez D , Simon-Sanchez J , Nalls MA , Scholz SW , Jimenez-Escrig A , Duarte J , Vives F , Duran R , Hoenicka J , Alvarez V , Infante J , Marti MJ , Clarimón J , López de Munain A , Pastor P , Mir P , Singleton A ((2019) ) The genetic architecture of Parkinson disease in Spain: Characterizing population-specific risk, differential haplotype structures, and providing etiologic insight. Mov Disord 34: , 1851–1863. |
[36] | Hillhouse EW , Grammatopoulos DK ((2006) ) The molecular mechanisms underlying the regulation of the biological activity of corticotropin-releasing hormone receptors: Implications for physiology and pathophysiology. Endocr Rev 27: , 260–286. |
[37] | Vyas S , Maatouk L ((2013) ) Contribution of glucocorticoids and glucocorticoid receptors to the regulation of neurodegenerative processes. CNS Neurol Disord Drug Targets 12: , 1175–1193. |
[38] | Chang D , Nalls MA , Hallgrímsdóttir IB , Hunkapiller J , van der Brug M , Cai F , Kerchner GA , Ayalon G , Bingol B , Sheng M , Hinds D , Behrens TW , Singleton AB , Bhangale TR , Graham RR ((2017) ) A meta-analysis of genome-wide association studies identifies 17 new Parkinson’s disease risk loci. Nat Genet 49: , 1511–1516. |
[39] | Nagata E , Saiardi A , Tsukamoto H , Okada Y , Itoh Y , Satoh T , Itoh J , Margolis RL , Takizawa S , Sawa A , Takagi S ((2011) ) Inositol hexakisphosphate kinases induce cell death in Huntington disease. J Biol Chem 286: , 26680–26686. |
[40] | Nagata E , Nonaka T , Moriya Y , Fujii N , Okada Y , Tsukamoto H , Itoh J , Okada C , Satoh T , Arai T , Hasegawa M , Takizawa S ((2016) ) Inositol hexakisphosphate kinase 2 promotes cell death in cells with cytoplasmic TDP-43 aggregation. Mol Neurobiol 53: , 5377–5383. |
[41] | Glanzmann B ((2016) ) Identification of novel Parkinson’s disease genes in the South African population, using a whole exome sequencing approach. Thesis, Faculty of Medicine and Health Sciences, Department of Biomedical Sciences, Stellenbosch University, p. 196. |
[42] | Hurley MJ , Durrenberger PF , Gentleman SM , Walls AF , Dexter DT ((2015) ) Altered expression of brain proteinase-activated receptor-2, trypsin-2 and serpin proteinase inhibitors in Parkinson’s disease. J Mol Neurosci 57: , 48–62. |
[43] | Benoit SM , Xu H , Schmid S , Alexandrova R , Kaur G , Thiruvahindrapuram B , Pereira SL , Jog M , Hebb MO ((2020) ) Expanding the search for genetic biomarkers of Parkinson’s disease into the living brain. Neurobiol Dis 140: , 104872. |
[44] | Campos ACP , Kikuchi DS , Paschoa AFN , Kuroki MA , Fonoff ET , Hamani C , Pagano RL , Hernandes MS ((2020) ) Unraveling the role of astrocytes in subthalamic nucleus deep brain stimulation in a Parkinson’s disease rat model. Cell Mol Neurobiol 40: , 939–954. |
[45] | Pan H , Zhao Y , Zhai Z , Zheng J , Zhou Y , Zhai Q , Cao X , Tian J , Zhao L ((2018) ) Role of plasminogen activator inhibitor-1 in the diagnosis and prognosis of patients with Parkinson’s disease. Exp Ther Med 15: , 5517–5522. |
[46] | Kronenbuerger M , Nolte KW , Coenen VA , Burgunder JM , Krauss JK , Weis J ((2015) ) Brain alterations with deep brain stimulation: New insight from a neuropathological case series. Mov Disord 30: , 1125–1130. |
[47] | Wang XQ , Zhuang HX , Zhang LX , Chen X , Niu CS , Zhao M ((2019) ) Nomogram for predicting postoperative delirium after deep brain stimulation surgery for Parkinson’s disease. World Neurosurg 130: , e551–e557. |
[48] | Nguyen M , Wong YC , Ysselstein D , Severino A , Krainc D ((2019) ) Synaptic, mitochondrial, and lysosomal dysfunction in Parkinson’s disease. Trends Neurosci 42: , 140–149. |