The Home-Based Sleep Laboratory
Abstract
Sleep disturbances are prevalent in neurodegenerative diseases in general, and in Parkinson’s disease (PD) in particular. Recent evidence points to the clinical value of sleep in disease progression and improving quality of life. Therefore, monitoring sleep quality in an ongoing manner at the convenience of one’s home has the potential to improve clinical research and to contribute to significantly better personalized treatment. Further, precise mapping of sleep patterns of each patient can contribute to a better understanding of the disease, its progression and the appropriate medical treatment. Here we review selective, state-of-the-art, home-based devices for assessing sleep and sleep related disorders. We highlight the large potential as well as the main challenges. In particular, we discuss medical validity, standardization and regulatory concerns that currently impede widespread clinical adoption of existing devices. Finally, we propose a roadmap with the technological and scientific steps that are required to impact PD research and treatment.
INTRODUCTION
Sleep disturbances are one of the most common non-motor symptoms in Parkinson’s disease (PD), with an estimated prevalence as high as 40– 90% [1, 2]. Sleep duration, sleep fragmentation, Rapid Eye Movement (REM) sleep behavior disorder, and sleep-disordered breathing, have all been associated with an increased risk of neurodegeneration, and are an independent risk for cognitive decline and dementia in PD [1]. Periodic limb movements of sleep and restless legs syndrome are also very common in PD. In conjunction, sleep abnormalities, in particular REM sleep without atonia, are an important bio-marker for disease and disease progression [3]. Indeed, the etiology of impaired sleep in PD is multifactorial and is likely an interaction between internal and external factors, such as primary sleep disorders [4], primary neurodegeneration [5– 7], medication side effects [5– 8], environmental conditions, and genetic factors [9]. Each factor can contribute alone or as a modifier, resulting in variability and poor diagnosis and treatment [8, 9].
Laboratory video polysomnography (vPSG) is the gold-standard for assessing sleep physiology in health and disease. PSG is most useful for diagnosis and treatment of obstructive sleep apnea (OSA), narcolepsy, REM sleep behavior disorder, and non-REM parasomnias [10]. Despite the clear utility of PSG in clinical sleep medicine, high cost and inconvenience have motivated the development of alternative home-based systems [11, 12].
Both the wellness and the clinical markets share an interest in “real-world” and home-based sleep monitoring (HBSM). HBSM can provide longitudinal assessment, avoiding the biggest PSG limitations: The atypical sleeping environment and the single-night snapshot. Measuring multiple nights in one’s own environment can provide insight into sleep dynamics. Such data can prove invaluable for the discovery of new intrinsic sleep patterns, revealing frequency and severity of observed sleep disorders or the impact of various factors (e.g., exercise, food, caffeine, alcohol, medication, and stress). The present paper reviews some of the recent developments in HBSM and provides a ‘road map’ for the implementation of such devices into clinical sleep assessment that can be used to enhance early diagnostics, improve PD treatment and incorporate into clinical trials.
WEARABLE TECHNOLOGIES
Sleep monitoring based on movement
Wearable sensors for sleep monitoring received increased attention in recent years. Most available systems utilize movement sensors and monitor blood saturation, heart rate and/or respiratory rate. A widely adopted system is the Actigraphy, a watch-like device containing accelerometers. Actigraphy has been used to study sleep– wake patterns for at least 30 years [13– 18] with a correlation of 90% with PSG in normal subjects, and an endorsement from the American Academy of Sleep Medicine for the assessment of sleep/wake activity in adults [19– 21]. However, actigraphy may be less accurate in specific subpopulations, such as those with PD or the elderly, due to reduced movement, as quiet wakefulness may be scored as sleep [22].
Inertial sensors can also provide information on position and nocturnal movements, to inform on duration of night rest [23], sleep interruptions and nocturnal hypokinesia in PD patients [17, 24, 25]. Some commercially available wrist devices (e.g., Fitbit, LARK, Garmin, etc.) also state that they can provide sleep quality, yet there are only a handful of validation studies on the accuracy of these devices compared to PSG. Meltzer et al. [26] found that Fitbit and Actigraph consistently misidentified sleep/wake states compared to PSG. In another study, Fitbit Charge 2™ showed high sensitivity for detecting sleep/wake, with 0.81 accuracy in detecting N1+N2 sleep (“light sleep”), but only 0.49 accuracy in detecting N3 sleep (“deep sleep”), and in general overestimated PSG in total sleep time by 9min, light sleep by 34min, and underestimated deep sleep by 24min [27]. These validation studies were conducted on healthy young adults and have not been explored as of yet in patients with PD [28], which likely further impact accuracy.
Sleep monitoring based on autonomic function
A decrease in heart rate (HR) and increases in vagal mediated heart rate variability (HRV) correlate with different sleep stages, arousals and EEG activity. Similarly, respiratory rate (RR) changes in different sleep stages, with non-REM sleep (in particular, deep sleep) characterized by more stable and regular respiratory amplitude and frequency, while RR and SpO2 monitoring have been reported useful for the assessment of OSA.
Several systems have been explored for unobtrusive autonomic monitoring [29– 31]. These include garments with embedded electrodes, belts and wrist devices. Using cardiorespiratory and movement signals showed moderate to high accuracy in discriminating sleep stages (ranging from 69% : wake-REM-N1N2-N3 to 92% : sleep/wake) in healthy adults [32], and were useful for the assessment of severe sleep apnea [29, 33]. However, artifacts in ECG signals can originate from movements, technical failures in cumbersome systems or ectopic beats (e.g., arrhythmia), which can result in spurious quantifications of signals (e.g., missing or double beats) and, thus, lead to biased estimations of HR and HRV. Certain medications (e.g., b-blockers) or cardiac denervation, common in PD, can further impact these metrics, potentially invalidating their physiologic meaning. Similarly, respiratory home-based analysis can be underestimated in PD patients, especially in those with greater motor dysfunction [31].
NONCONTACT: NEARABLE DEVICES
‘Nearables’ are remote sensors that do not directly contact the body but have been used for assessment of behavior and function. ‘Sleep score’ and ‘Sleep Cycle’ are examples of applications that use a mobile phone built-in accelerometer to monitor movement when the phone is placed near one’s pillow. The applications report on sleep time and sleep phases. Although none of the apps offer raw data and supporting validation studies are not yet available, their affordability and accessibility is a clear advantage.
Several commercially available products use piezoelectric or electrodes (e.g., EarlySense, Emfit Besd sensor) placed under the mattress to estimate respiration, HR and movement [34, 35]. Examination in healthy adults showed moderate agreement (71%) with PSG [34]. The distinction between wake and REM sleep were most often misclassified [34]. A recent study comparing a consumer monitoring device based on ballistocardiography (Beddit Sleep-Tracker) and PSG, showed extremely poor agreement for NREM classifications (kappa=0.095, p<0.001) [35]. These findings suggest poor compatibility with clinical sleep assessment.
Video-based sleep analysis in the home can provide information on sleep efficiency and nocturnal movements. New emerging three-dimensional video analysis shows promise in distinguishing REM sleep behavior disorder (RBD) from other motor activities during sleep, as examined in a PSG lab [36]. However, the utility of such an approach should be further explored in the home, taking into account also ethical concerns about privacy and data security of the recordings.
Radiofrequency devices use changes in radio waves reflection to derive the position of individuals [37, 38]. Such systems provide unlimited data from multiple nights, therefore variance over time can be investigated without compromising privacy. A recent study used both radiofrequency home monitoring (SMHOME), as well as PSG (with both systems) to monitor OSA [37]. The SMHOME classified 92% of cases correctly and showed high agreement with PSG for detecting breathing problems. As expected, night after night, variability was high. Only one study was found using a radiofrequency device in patients with PD (Emerald) [38]. Data on time in bed/per day and number and duration of nightly awakenings showed high variance across and within individuals. More research is needed to fully evaluate the benefits in PD.
BRAIN SIGNAL TECHNOLOGIES
The development of ambulatory technologies capable of monitoring brain activity during sleep longitudinally is critical for advancing sleep science. Systems utilizing movement sensors or autonomic measures are important indirect measures, but they are not sufficient for distinguishing sleep stages that are necessary to diagnose NREM parasomnias or RBD [12, 39].
Several systems incorporating EEG sensors were recently described. Shambroom et al. [40] reported on an automated wireless system based on a headband with three silver-coated fabric sensors. Mikkelsen et al. [41] used ear electrodes to demonstrate automatic sleep EEG classification. Recent work [42] explored the accuracy of the Dreem headband, a dry EEG device in detecting sleep stages. Accuracy was overall high (83.5±6.4%) and comparable to that of experts scoring PSG (86.4±8.0%) [42]. However, the device does not record EOG or EMG, lowering its precision in detecting, for example, REM sleep without atonia.
A promising approach towards ambulatory electrophysiology is flexible electrode arrays. In particular, electrodes printed on soft substrates are marked by their conformity with the skin, are lightweight, ease of placement on the skin, and user comfort. The X-trodes home PSG system [43] is a wireless wearable system which provides a sleep-specific electrode array including two surface EMG sensors on the chin, two EOG electrodes positioned in the proximity of the right eye, and four forehead EEG electrodes placed on the forehead. The system was recently tested in both the laboratory and home setting, showing high accuracy compared to video recording EEG assessment (89– 92%) [43], providing a potentially suitable home-based PSG over multiple nights. However, this should be further explored in patients with PD.
DISCUSSION AND FUTURE DIRECTION
Understanding the link between PD and sleep goes well beyond academic curiosity. If poor sleep quality is a cause and an early indication for neural and motor decline in PD, it is critically important to provide patients, and those that belong to risk groups, with timely information about their sleep quality to initiate treatment or life-style changes. There is still a debate whether sleep disturbances are primary or secondary to PD pathology. Nevertheless, it is important to understand whether their severity is associated with disease progression and whether behavioral modification or therapeutic adjustment can improve a patient’s quality of life and potentially impact disease progression. Furthermore, additional detailed knowledge on sleep and sleep architecture in PD, from multiple nights in the person’s natural environment, can contribute to revealing new biomarkers.
Despite the availability of some devices, those are not validated against the current gold-standard and are based on small studies on healthy adults. The impact of the disease on the measurements may be dramatic negating proper use. Moreover, many of the systems evaluate a specific physiological function (i.e., movement, HR, RR). Such data have merit for specific evaluation such as OSA, hypokinesia or sleep interruptions, but does not provide the full scope of an uninterrupted night’s sleep. Medical validity, standardization and regulation are key to the adoption of digital tools into clinical practice. Possible steps for such implementation are described in Fig. 1. The first stage is concerned with system engineering and validation. A HBSM must accommodate several critical requirements: it must collect medically meaningful data of the highest quality and reliability. Monitoring sleep must take place in a minimally obtrusive manner, enabling subjects to use it routinely without affecting their sleep patterns. A home-based system has to be also easy to operate, otherwise adoption will be limited and will not help resolve the missing data challenge. This first stage must also focus on improving the fidelity of the collected data (i.e., reducing noise sources and artifacts), and the introduction of automated data analysis. The development of real time and cloud-based analysis would expand the clinical utility. With such automation, physicians could obtain a sleep report before meeting the patient.
Fig. 1
Roadmap for sleep staging at home for PD Care.
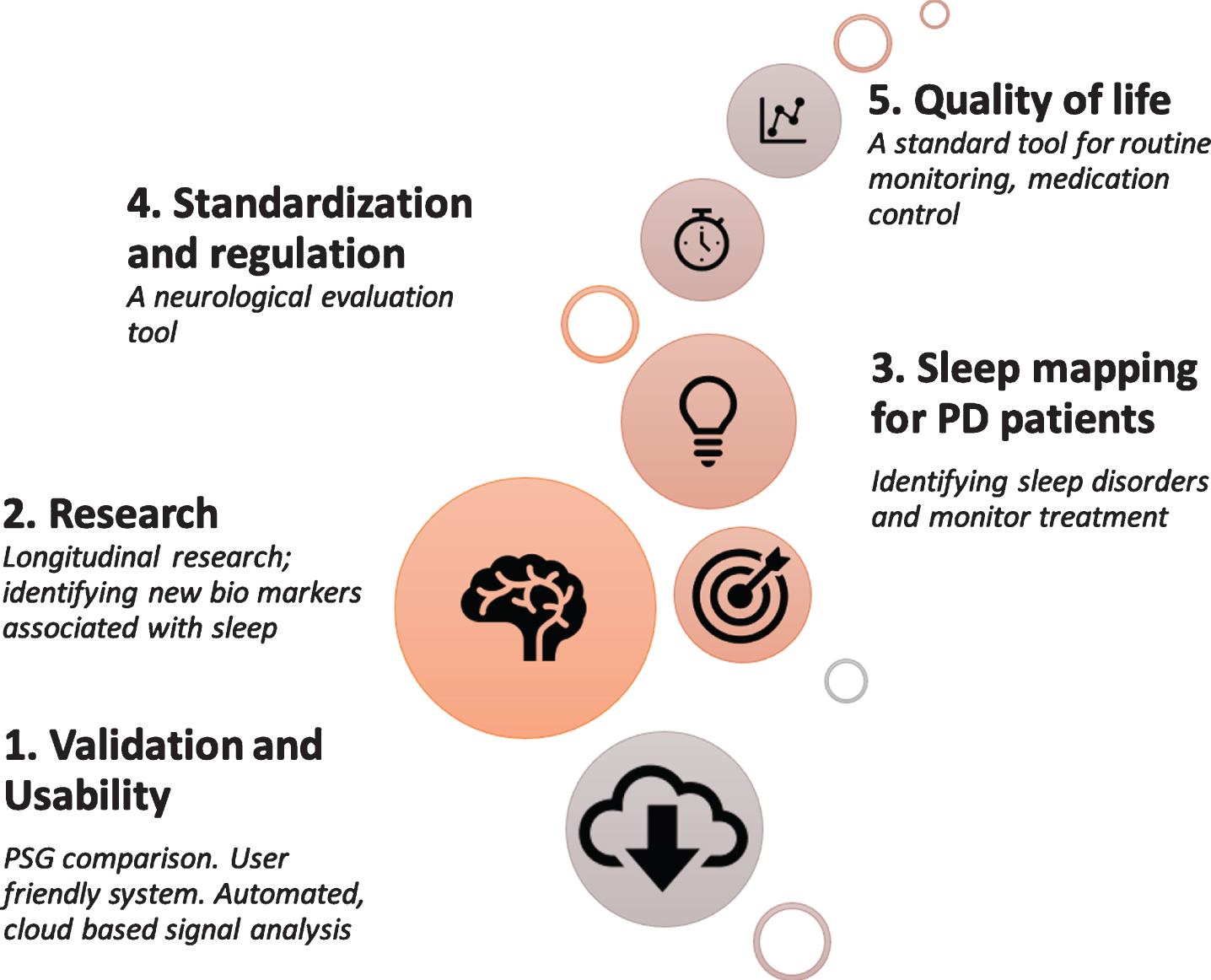
A HBSM has to be carefully and rigorously validated against the gold standard. Although PSG has limitations, it is imperative to understand whether and under which circumstances HBSM can be trusted and how to interpret collected data. It is important to remember that multifaceted configurations with numerous sensors attached to multiple body locations are useful for research purposes but are unlikely to be used in large clinical trials due to relatively high costs and increased patient burden. Clearly, there is a trade-off between maximizing data power and minimizing the effort involved in obtaining it. Nevertheless, data obtained in the home should encompass as many and as similar outputs as the gold standard (e.g., HR, RR, EEG, and movement).
Once a technology has achieved these steps, the exact manner by which it can affect PD care has to be investigated. Most of what we know about sleep in PD is based on patient self-reports, therefore the validity of what we know about frequency and severity is limited. The next stage is therefore to revisit existing studies, focusing on sleep staging as a tool for helping PD patients. In particular, longitudinal research may help identify new bio markers associated with sleep (Fig.1.2). This step should also focus on characterizing disease progression and severity. Next, sleep mapping of PD patients may help identify sleep disorders and monitor possible treatment, medication efficacy and behavioral factors (Fig.1.3). Addressing regulation requirements and standardization is critical for future clinical adaptation and inclusion into clinical trials and clinical practice (Fig.1.4). Only then, HBSM can be used as a standard tool for routine monitoring and medication control to overall impact patient quality of life (Fig.1.5).
Digital technology helps shifting healthcare from reactive to preventive and predictive care. Within this shift, there is a growing demand to make sleep assessment possible using home-based systems. Recent developments offer new and exciting opportunities, but there is still a long process to ensure clinical use. The recent global situation, under the new COVID-19 reality, further highlights the dire need for a shift towards home-based data collection and telemedicine.
ACKNOWLEDGMENTS
The authors thank F. Fahoum, M. de Vos, L. Inzelberg, Z. Peremen, and Y. Nir for many fruitful discussions.
CONFLICT OF INTEREST
YH declares financial interest in X-trodes Ltd., which holds the licensing rights of the skin technology cited in this paper. The authors have no other relevant affiliations or financial involvement with any organization or entity with a financial interest in, or financial conflict with the subject matter or materials discussed in the manuscript, apart from those disclosed.
REFERENCES
[1] | Postuma RB ((2014) ) Prodromal Parkinson’s disease - Using REM sleep behavior disorder as a window. Parkinsonism Relat Disord 20: (Suppl 1), S1–4. |
[2] | Paparrigopoulos TJ ((2005) ) REM sleep behaviour disorder: Clinical profiles and pathophysiology. Int Rev Psychiatry 17: , 293–300. |
[3] | Cesari M , Christensen JAE , Muntean ML , Mollenhauer B , Sixel-Döring F , Sorensen HBD , Trenkwalder C , Jennum P ((2020) ) A data-driven system to identify REM sleep behavior disorder and to predict its progression from the prodromal stage in Parkinson’s disease. Sleep Med, doi: 10.1016/j.sleep.2020.04.010 |
[4] | Lo JC , Loh KK , Zheng H , Sim SKY , Chee MWL ((2014) ) Sleep duration and age-related changes in brain structure and cognitive performance. Sleep 37: , 1171–1178. |
[5] | Meloni M , Bortolato M , Cannas A , Laccu I , Figorilli M , Congiu P , Defazio G , Meloni F , Puligheddu M ((2020) ) Association between dopaminergic medications and REM sleep behavior disorder in Parkinson’s disease: A preliminary cohort study. J Neurol 267: , 2926–2931. |
[6] | Pillai JA , Leverenz JB ((2017) ) Sleep and neurodegeneration: A critical appraisal. Chest 151: , 1375–1386. |
[7] | Santos-García D , Castro ES , de Deus Fonticoba T , Panceiras MJF , Enriquez JGM , González JMP , Bartolomé CC , Planellas LL , Caldentey JG , Caballol N , Legarda I , López IC , Manzanares LL , Rivera MAÁ , Catalán MJ , Nogueira V , Borrué C , Sauco MÁ , Vela L , Cubo E , Castrillo JCM , Alonso PS , Losada MGA , Ariztegui NL , Gastón MI , Kulisevsky J , Pagonabarraga J , Seijo M , Martínez JR , Valero C , Kurtis M , Ardura JG , Prieto C , Mir P , Martinez-Martin P ((2020) ) Sleep problems are related to a worse quality of life and a greater non-motor symptoms burden in Parkinson’s Disease. J Geriatr Psychiatry Neurol doi: 10.1177/0891988720964250 |
[8] | Park KW , Jo S , Lee SH , Hwang YS , Lee D , Ryu HS , Chung SJ ((2020) ) Therapeutic effect of levodopa/carbidopa/ entacapone on sleep disturbance in patients with Parkinson’s disease. J Mov Disord 13: , 205–215. |
[9] | Gan-Or Z , Alcalay RN , Rouleau GA , Postuma RB ((2018) ) Sleep disorders and Parkinson disease; lessons from genetics. Sleep Med Rev 41: , 101–112. |
[10] | Happe S , Klösch G , Lorenzo J , Kunz D , Penzel T , Röschke J , Himanen SL , Gruber G , Zeitlhofer J ((2005) ) Perception of sleep: Subjective versus objective sleep parameters in patients with Parkinson’s disease in comparison with healthy elderly controls - Sleep perception in Parkinson’s disease and controls. J Neurol 252: , 936–943. |
[11] | Griessenberger H , Heib DPJ , Kunz AB , Hoedlmoser K , Schabus M ((2013) ) Assessment of a wireless headband for automatic sleep scoring. Sleep Breath 17: , 747–752. |
[12] | Kelly JM , Strecker RE , Bianchi MT ((2012) ) Recent developments in home sleep-monitoring devices. ISRN Neurol 2012: , 1–10. |
[13] | Sadeh A , Hauri PJ , Kripke DF , Lavie P ((1995) ) The role of actigraphy in the evaluation of sleep disorders. Sleep 18: , 288–302. |
[14] | Van Hilten B , Hoff JI , Middelkoop HAM , Van Der Velde EA , Kerkhof GA , Wauquier A , Kamphuisen HAC , Roos RAC ((1994) ) Sleep disruption in parkinson’s disease: Assessment by continuous activity monitoring. Arch Neurol 51: , 922–928. |
[15] | Whitehead DL , Davies ADM , Playfer JR , Turnbull CJ ((2008) ) Circadian rest-activity rhythm is altered in Parkinson’s disease patients with hallucinations. Mov Disord 23: , 1137–1145. |
[16] | Louter M , Maetzler W , Prinzen J , Van Lummel RC , Hobert M , Arends JBAM , Bloem BR , Streffer J , Berg D , Overeem S , Liepelt-Scarfone I ((2015) ) Accelerometer-based quantitative analysis of axial nocturnal movements differentiates patients with Parkinson’s disease, but not high-risk individuals, from controls. J Neurol Neurosurg Psychiatry 86: , 32–37. |
[17] | Sringean J , Taechalertpaisarn P , Thanawattano C , Bhidayasiri R ((2016) ) How well do Parkinson’s disease patients turn in bed? Quantitative analysis of nocturnal hypokinesia using multisite wearable inertial sensors. Parkinsonism Relat Disord 23: , 10–16. |
[18] | Stefani A , Heidbreder A , Brandauer E , Guaita M , Neier LM , Mitterling T , Santamaria J , Iranzo A , Videnovic A , Trenkwalder C , Sixel-Döring F , Wenning GK , Chade A , Poewe W , Gershanik OS , Högl B ((2018) ) Screening for idiopathic REM sleep behavior disorder: Usefulness of actigraphy. Sleep 41: , zsy053. |
[19] | Mantua J , Gravel N , Spencer RMC ((2016) ) Reliability of sleep measures from four personal health monitoring devices compared to research-based actigraphy and polysomnography. Sensors (Basel) 16: , 646. |
[20] | Razjouyan J , Naik AD , Horstman MJ , Kunik ME , Amirmazaheri M , Zhou H , Sharafkhaneh A , Najafi B ((2018) ) Wearable sensors and the assessment of frailty among vulnerable older adults: An observational cohort study. Sensors (Basel) 18: , 1336. |
[21] | Smith MT , McCrae CS , Cheung J , Martin JL , Harrod CG , Heald JL , Carden KA ((2018) ) Use of actigraphy for the evaluation of sleep disorders and circadian rhythm sleep-wake disorders: An American Academy of Sleep Medicine Clinical Practice Guideline. J Clin Sleep Med 14: , 1231–1237. |
[22] | Maglione JE , Liu L , Neikrug AB , Poon T , Natarajan L , Calderon J , Avanzino JA , Corey-Bloom J , Palmer BW , Loredo JS , Ancoli-Israel S ((2013) ) Actigraphy for the assessment of sleep measures in Parkinson’s disease. Sleep 36: , 1209–1217. |
[23] | Klingelhoefer L , Rizos A , Sauerbier A , McGregor S , Martinez-Martin P , Reichmann H , Horne M , Chaudhuri KR ((2016) ) Night-time sleep in Parkinson’s disease - the potential use of Parkinson’s KinetiGraph: A prospective comparative study. Eur J Neurol 23: , 1275–1288. |
[24] | Bhidayasiri R , Phokaewvarangkul O , Sringean J , Martinez-Martin P , Anan C , Kantachadvanich N , Chaudhuri KR , Hattori N ((2019) ) Evaluation of nocturnal hypokinesia in Parkinson’s disease using a novel patient/proxy questionnaire and correlations with objective monitoring. Parkinsonism Relat Disord 61: , 219–223. |
[25] | Mirelman A , Hillel I , Rochester L , Del Din S , Bloem BR , Avanzino L , Nieuwboer A , Maidan I , Herman T , Thaler A , Gurevich T , Kestenbaum M , Orr-Urtreger A , Brys M , Cedarbaum JM , Giladi N , Hausdorff JM ((2020) ) Tossing and turning in bed: Nocturnal movements in Parkinson’s disease. Mov Disord 35: , 959–968. |
[26] | Meltzer LJ , Hiruma LS , Avis K , Montgomery-Downs H , Valentin J ((2015) ) Comparison of a commercial accelerometer with polysomnography and actigraphy in children and adolescents. Sleep 38: , 1323–1330. |
[27] | de Zambotti M , Goldstone A , Claudatos S , Colrain IM , Baker FC ((2018) ) A validation study of Fitbit Charge 2TM compared with polysomnography in adults. Chronobiol Int 35: , 465–476. |
[28] | Martinez-Ramirez D , De Jesus S , Walz R , Cervantes-Arriaga A , Peng-Chen Z , Okun MS , Alatriste-Booth V , Rodríguez-Violante M ((2015) ) A polysomnographic study of Parkinson’s disease sleep architecture. Parkinsons Dis 2015: , 570375. |
[29] | de Zambotti M , Cellini N , Goldstone A , Colrain IM , Baker FC ((2019) ) Wearable sleep technology in clinical and research settings. Med Sci Sports Exerc 51: , 1538–1557. |
[30] | Fonseca P , Long X , Radha M , Haakma R , Aarts RM , Rolink J ((2015) ) Sleep stage classification with ECG and respiratory effort. Physiol Meas 36: , 2027–2040. |
[31] | Gros P , Mery VP , Lafontaine AL , Robinson A , Benedetti A , Kimoff RJ , Kaminska M ((2015) ) Diagnosis of obstructive sleep apnea in Parkinson’s disease patients: Is unattended portable monitoring a suitable tool? Parkinsons Dis 2015: , 258418. |
[32] | Willemen T , Van Deun D , Verhaert V , Vandekerckhove M , Exadaktylos V , Verbraecken J , Van Huffel S , Haex B , Vander Sloten J ((2014) ) An evaluation of cardiorespiratory and movement features with respect to sleep-stage classification. IEEE J Biomed Health Informatics 18: , 661–669. |
[33] | Fontana P , Martins NRA , Camenzind M , Boesch M , Baty F , Schoch OD , Brutsche MH , Rossi RM , Annaheim S ((2019) ) Applicability of a textile ECG-belt for unattended sleep apnoea monitoring in a home setting. Sensors (Basel) 19: , 3367. |
[34] | Mendez MO , Migliorini M , Kortelainen JM , Nistico D , Arce-Santana E , Cerutti S , Bianchi AM ((2010) ) Evaluation of the sleep quality based on bed sensor signals: Time ariant analysis. In 2010 Annual International Conference of the IEEE Engineering in Medicine and Biology Society, EMBC’10, pp. 3994-3997. |
[35] | Tuominen J , Peltola K , Saaresranta T , Valli K ((2019) ) Sleep parameter assessment accuracy of a consumer home sleep monitoring ballistocardiograph beddit sleep tracker: A validation study. J Clin Sleep Med 15: , 483–487. |
[36] | Waser M , Stefani A , Holzknecht E , Kohn B , Hackner H , Brandauer E , Bergmann M , Taupe P , Gall M , Garn H , Högl B ((2020) ) Automated 3D video analysis of lower limb movements during REM sleep: A new diagnostic tool for isolated REM sleep behavior disorder. Sleep 43: , zsaa100. |
[37] | Crinion SJ , Tiron R , Lyon G , Zaffaroni A , Kilroy H , Doheny E , O’Hare E , Boyle P , Russell A , Traynor M , Kent BD , Ryan S , McNicholas WT ((2020) ) Ambulatory detection of sleep apnea using a non-contact biomotion sensor. J Sleep Res 29: , e12889. |
[38] | Kabelac Z , Tarolli CG , Snyder C , Feldman B , Glidden A , Hsu C-Y , Hristov R , Dorsey ER , Katabi D ((2019) ) Passive monitoring at home: A pilot study in Parkinson disease. Digit Biomarkers 3: , 22–30. |
[39] | Tal A , Shinar Z , Shaki D , Codish S , Goldbart A ((2017) ) Validation of contact-free sleep monitoring device with comparison to polysomnography. J Clin Sleep Med 13: , 517–522. |
[40] | Shambroom JR , Fábregas SE , Johnstone J ((2012) ) Validation of an automated wireless system to monitor sleep in healthy adults. J Sleep Res 21: , 221–230. |
[41] | Mikkelsen KB , Villadsen DB , Otto M , Kidmose P ((2017) ) Automatic sleep staging using ear-EEG. Biomed Eng Online 16: , 111. |
[42] | Arnal PJ , Thorey V , Ballard ME , Hernandez AB , Guillot A , Jourde H , Harris M , Guillard M , van Beers P , Chennaoui M , Sauvet F ((2019) ) The dreem headband as an alternative to polysomnography for EEG signal acquisition and sleep staging. bioRxiv, https://doi.org/10.1101/662734. |
[43] | Shustak S , Inzelberg L , Steinberg S , Rand D , David Pur M , Hillel I , Katzav S , Fahoum F , De Vos M , Mirelman A , Hanein Y ((2019) ) Home monitoring of sleep with a temporary-tattoo EEG, EOG and EMG electrode array: A feasibility study. J Neural Eng 16: , 026024. |