Quadruple Decision Making for Parkinson’s Disease Patients: Combining Expert Opinion, Patient Preferences, Scientific Evidence, and Big Data Approaches to Reach Precision Medicine
Abstract
Clinical decision making for Parkinson’s disease patients is supported by a combination of three distinct information resources: best available scientific evidence, professional expertise, and the personal needs and preferences of patients. All three sources have clear value but also share several important limitations, mainly regarding subjectivity, generalizability and variability. For example, current scientific evidence, especially from controlled clinical trials, is often based on selected study populations, making it difficult to translate the outcome to the care for individual patients in everyday clinical practice. Big data, including data from real-life unselected Parkinson populations, can help to bridge this information gap. Fine-grained patient profiles created from big data have the potential to aid in identifying therapeutic approaches that will be most effective given each patient’s individual characteristics, which is particularly important for a disorder characterized by such tremendous interindividual variability as Parkinson’s disease. In this viewpoint, we argue that big data approaches should be acknowledged and harnessed, not to replace existing information resources, but rather as a fourth and complimentary source of information in clinical decision making, helping to represent the full complexity of individual patients. We introduce the ‘quadruple decision making’ model and illustrate its mode of action by showing how this can be used to pursue precision medicine for persons living with Parkinson’s disease.
CLINICAL DECISION MAKING IN TRANSITION
Traditionally, clinicians were trusted authorities who mainly relied on their training and personal experience to make clinical decisions. From the late sixties and early seventies of the previous century onwards, there was a sharp rise in attention for evidence-based medicine (EBM). EBM recognized the importance of integrating the clinician’s professional training and expertise with individual patient characteristics and the best available evidence [1]. The various evidence-based guidelines for the management of patients with Parkinson’s disease (PD) reflect this line of thinking [2–5]. Currently, the best available evidence is often derived from clinical trials, but following the questioning of the external validity of trial data, other forms of evidence have become increasingly accepted. For example, individual case reports also provide specific value in an evidence-based world, and their merits are often underestimated [6]. One particularly important development has been to better and more formally encompass the patient’s personal preferences into the decision-making process [7]. The notion of shared decision making reflects the idea that patients should be actively involved in a joint decision-making process. As such, shared decision making supports the application of EBM into clinical practice as it aims to integrate all information sources into the final decision [8].
The process of shared decision making still encounters difficulties in making decisions tailored to the unique characteristics of each patient, especially in a multidimensional disease such as PD. First, many relevant characteristics remain unknown, and many examples can be seen every day in our clinical practices. Why does one patient respond excellently to dopaminergic medication, and why does another—seemingly identical—patient fail to respond, or develop cumbersome side effects? This variation in response also extends to non-pharmacological interventions, such as exercise which seems to do miracles to some, but is perceived as useless by others. And why is there such a striking variation in rates of disease progression across PD patients with a comparable set of baseline clinical characteristics? Genetic factors may explain part of these discrepancies, but recognition is growing that many other factors may contribute as well, including epigenetics, microbiome changes, psychological factors and environmental factors such as lifestyle, socio-economic background, nutrition or exercise [9, 10]. As long as these factors are not or only poorly understood, they can obviously not be included in interpreting the intervention effects or in making reliable prognostic predictions. Second, current scientific evidence is often based on observations of selected study populations and usually does not account for the typical heterogeneity within a population. For example, very old patients or those with marked comorbidity are typically excluded from trials, but such patients do regularly show up in clinical practice. Both factors hamper the translation of research findings and the recommendations of guidelines to the diagnosis and treatment of individual patients. Even epidemiological prediction models [11, 12] that have become widespread in the past decades cannot overcome this limitation. Finally, concerns have been raised whether PD patients, given their common difficulties in making complex choices, are actually able to participate in a shared decision making process, particularly when cognitive decline is prominent [13].
Analyses of big data can potentially help to overcome this limitation [14]. Many types of data are generally reckoned to fall under the umbrella term of ‘big data’, including observational study data, large datasets, technology-generated outcomes (e.g., from wearable sensors), passively collected data, and machine-learning generated algorithms. Even though the definition of big data is subject of discussion, a consensual definition is proposed as ‘Information assets characterized by such a high volume, velocity and variety to require specific technology and analytical methods for its transformation into value [15]’. The word ‘big’ in big data can therefore refer to the size of the target population, but also to the richness in numbers of different variables that are open for analysis. When big data comprises multidimensional datasets that include data from large and more unselected ‘real-life’ populations, and when adequate tools, skills and resources for interpretation are available, it can provide a valuable additional source of information in the decision-making process. More specifically, big data approaches have the potential to bridge the gap between EBM and the multidimensional nature of the patient’s personal context, allowing clinicians to incorporate the complexity of an individual into personalized clinical decisions. The combination of all four information resources (current scientific evidence; professional expertise; personal needs and preferences of patients; and big data approaches) should ideally be bundled into what we would call a ‘quadruple decision making’ model (Fig. 1). In PD, there are still major challenges before this stage of quadruple decision making can be integrated in clinical practice. In this viewpoint, we illustrate the potential but also the challenges of this new model to arrive at precision medicine for persons living with PD.
Fig.1
Clinical decision making models.
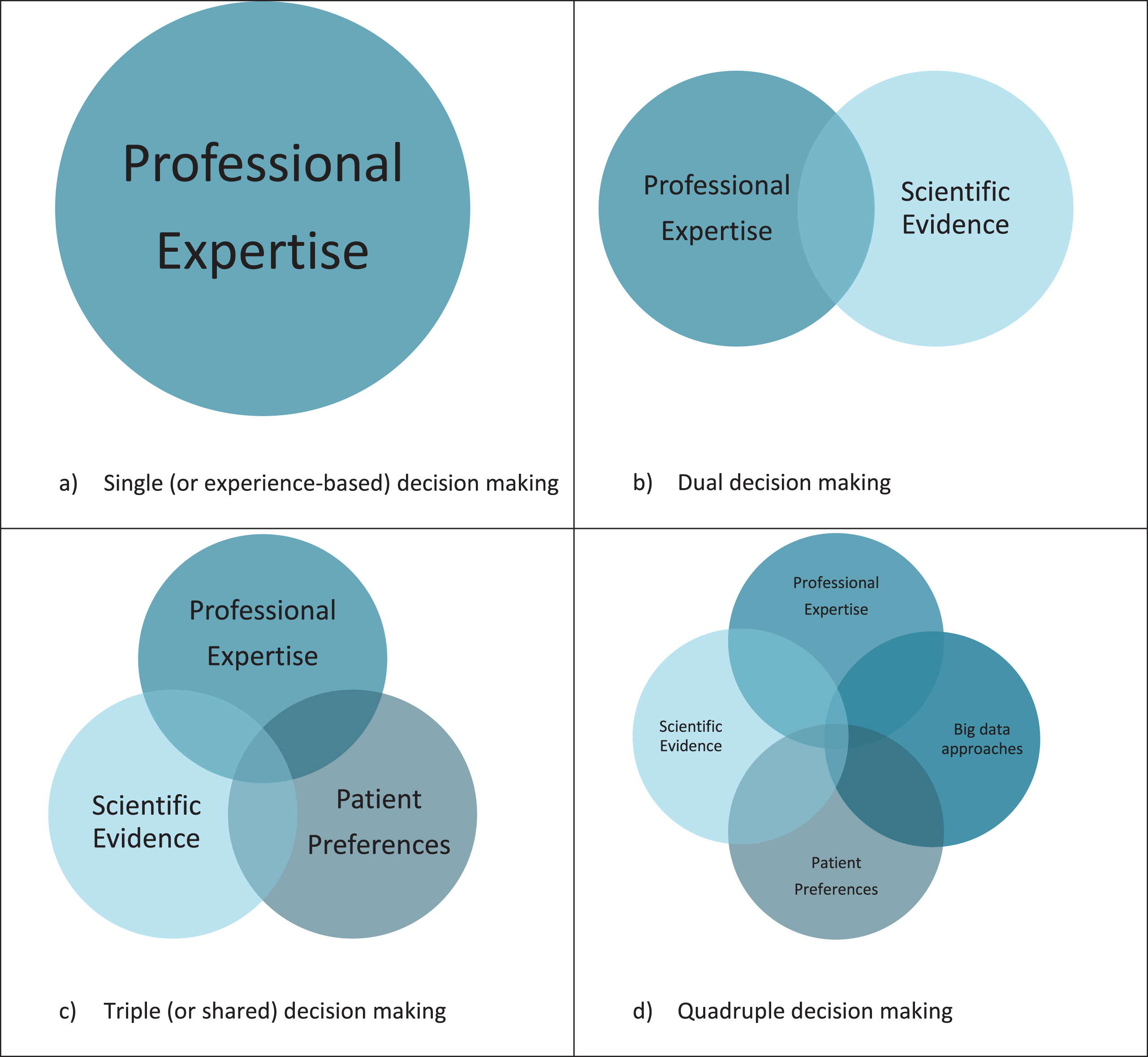
FROM EVIDENCE-BASED MEDICINE TO PRECISION MEDICINE
PD is a representative example of a complex, highly variable and multifaceted chronic condition, with a rather complex treatment repertoire. The response to therapy and also the risk of experiencing adverse effects can differ markedly between different patients, but individual predictions are presently difficult to make. To illustrate this, clinical trials have shown that 3% to 35% of PD patients who are on dopamine agonist drug therapy will develop impulse control disorders [16]. The use of dopamine agonists is effective in most patients, but there is no way of knowing which patients will develop impulse control disorders. Young men clearly have an increased risk, but impulse control disorders can certainly also develop in women and in elderly patients. As a result, clinicians typically resort to a ‘trial and error’ approach to identify patients who are likely to benefit, with an acceptable level of adverse effects. This approach carries a serious risk of depriving patients who would benefit from this particular treatment of its positive effects, and also of harming those who later turn out to develop unacceptable adverse effects. Both groups would, in effect, end up receiving low-value care.
Recognizing such low-value care in our healthcare system, and acknowledging the importance of person-centred care, helps to understand the current call for precision medicine. Precision medicine is an approach that seeks to target prevention, diagnosis, and treatment more closely to the individual characteristics and needs of patients [17]. In order to steer away from the current ‘one size fits all’ concept and head more towards precision medicine, a fourth perspective should be included in decision making, namely the specific context of the individual PD patient.
This context of an individual patient is highly relevant for PD, given the complexity of the disorder and the tremendous variety in both motor and non-motor symptoms, both within and across different patients. For example, clinical subtypes, or clusters, have been recognized, such as ‘mild motor-predominant’, ‘intermediate’ and ‘diffuse malignant’ [18], or ‘old age-at-onset and rapid disease progression’ and ‘young age-at-onset and slow disease progression’ [19]. A different therapeutic responsiveness has been observed for patients with differing subtypes, for example, there is a different levodopa-induced neural response between a ‘tremor-dominant’ subtype compared to the ‘postural instability/gait difficulty’ subtype, as measured on fMRI [20]. Also, the effect of deep brain stimulation as treatment for PD patients seems to be variable depending on the mutated gene. Outcomes appear to be good in patients with the p.G2019S LRRK2-mutation or PRKN-mutations, but poor in patients with the rarer LRRK2 p.R1441G mutation [21]. And having a GBA-mutation appeared to be an important predictor for non-motor symptom disease progression after deep brain stimulation surgery [22]. However, current scientific evidence still consists mainly of studies that are performed in rather selective study populations (often the ‘ideal’ patients, i.e., with little or no co-morbidity) which consider only a limited set of patient characteristics for subtype-classification. A good example was provided in this journal, in a report highlighting the fact that PD patients with cognitive decline are commonly excluded from studies on falls in PD, even though these very falls are particularly common in patients experiencing cognitive decline [23]. As such, many study results do not include the relevant context of the individual patient.
BIG DATA APPROACHES FOR A NOVEL PERSONALIZED INGREDIENT IN DECISION MAKING
The era of digital healthcare opens up new opportunities to bring individual characteristics into the clinical decision-making process in a more comprehensive and systematic way [24]. The amount and diversity of data that can be collected digitally is expanding rapidly. Big data, consisting of structured and unstructured data from real life patients, can be used to develop predictive algorithms considering the natural context of patients, by identifying fine-grained clusters of patients with a comparable profile [25].
A wide range of data types can serve as input for big data analyses, jointly covering all relevant aspects needed to understand the course and clinical management of a disease in real life. Others have already recognized genetics (including pharmacogenetics), comorbidities and phenotypic data to be relevant for precision medicine in PD [9, 10]. In addition, so-called ‘social biomarkers’ can contribute, as they contain information on a patient’s behavior, social and economic environment, psychological effects of treatment, and spiritual or religious commitments [26, 27]. Digital measurement tools, such as body-worn sensors or smart watches, are increasingly being used in experimental settings to generate large amounts of data about individuals as they move about for long periods of time in their real-world environments. These tools have the potential of offering unique insights into the profound variability in symptoms from day to day that is generally not measured in clinical care or studies [28–31]. This is particularly relevant for a condition such as PD that is defined by fluctuating symptoms (such as the variable response to dopaminergic medication, or the changes in clinical presentation elicited by stress and anxiety), relatively rare events (such as falls) or manifestations that develop very gradually over time (such as the tendency to become more sedentary).
Numerous datasets comprising routinely collected data from PD patients are currently available. A well-known illustration is the use of data derived from electronic medical records or from medical claims, which can provide very useful insights into everyday healthcare processes. In this journal, we recently highlighted the great potential offered by medical claims databases, which contain a rich set of longitudinal data collected for large-scale and real-life PD populations [32]. Such medical claims data have, for example, proven to be effective in demonstrating the value of specialized physiotherapy for PD patients [33]. Alternative large datasets, using patient-reported outcomes derived from online communities such as PatientsLikeMe, have been used to assess treatment effects in diseases other than PD [34]. There are multiple other initiatives that systematically collect rich data from large cohorts of PD patients, such as the Parkinson’s Progression Marker Initiative (PPMI), the Oxford Parkinson’s Disease Centre Cohort and the Parkinson’s Foundation cohorts [35–37]. Data from such cohorts have been used, for instance, to design classification models for diagnosing PD [38]. The Parkinson Disease Digital Biomarker DREAM challenge and the mPower mobile Parkinson Disease study are examples of open source datasets compromising traditional behavioral symptom measures with novel metrics from mobile devices [30, 39]. Importantly, various organizations such as the Michael J Fox Foundation and the Critical Path Institute have developed initiatives to couple and sanitize multiple datasets from different sources, with the ultimate aim of data sharing.
HOW BIG DATA GENERATES A FOURTH INFORMATION SOURCE IN CLINICAL DECISION MAKING
Utilizing big data approaches on a large scale to provide personalized, profile-based predictions, closer to the individual context, would offer a big step towards precision medicine. Machine learning techniques, i.e., analytics that learn automatically from data, can be used to find specific patterns and profiles in big data and in this way integrate different types of data to make predictions for clusters of comparable patients. As such, big data approaches holds great promise by expanding the capacity to uncover relevant information and to generate new knowledge [25].
The first steps to make personalized predictions in individual PD patients have been made. For example, in PD patients with deep brain stimulation, personalized predictions that used patient-specific details have been used in experimental settings to predict the motor outcome [40]. The study showed that such techniques can be used to predict the motor outcome of a combined stimulation and medication treatment plan in specific patients, albeit with variable accuracy. Another study used PPMI data to demonstrate that model-free big data machine learning methods can outperform model-based techniques in terms of predictive precision and reliability (e.g., forecasting patient diagnosis) [41]. A recent paper mined the PPMI dataset to explore the predictive power of the MDS-UPDRS and showed that while the MDS-UPDRS is a useful tool to monitor the progression of PD at the group level, it is much less useful for making individual predictions due to large variations in scores across consecutive assessments [42]. This again highlights the importance of developing new tools (informed by big data approaches) that have much greater predictive accuracy at the individual level. However, the actual evidence supporting the potential of big data approaches to make personalized predictions in PD is still scarce.
Personalized decision support systems, that provide an overview of the predicted treatment effect for a specific patient based on a cluster of patients with similar characteristics, can help to bring information from big data approaches into the decision-making process. Within the field of neurology, personalized decision support systems are largely unknown, in contrast to for example oncology where they are used more often. An example of a tool used in oncology is PREDICT, which was designed to help both patients and doctors to decide on the ideal course of an individual treatment following breast cancer surgery [43]. In neurology, an illustrative example is a personalized decision support system that can be used to support specialists in deciding which patients with chronic lower back pain should be considered for a surgical intervention and which patients for a non-surgical intervention, based on their individual profile [44]. Big data approaches are increasingly recognized as a valuable source for developing such personalized decision support systems [45], for example in choosing an antiepileptic drug regimen tailored to an individual patient profile [46]. As such, when coupled with human expertise and careful interpretation, big data approaches can become a fourth and complimentary source of information, alongside professional expertise, current scientific evidence including evidence from clinical trials and patient preferences, with each having their own benefits and challenges (Table 1).
Table 1
The four elements of clinical decision making with their benefits and their challenges [47, 50, 52, 55]
Information source | Benefits | Challenges |
Professionel expertise | •Human judgement •Experience with specific populations •Includes local context | •Depends on numbers and types of patients seen •Blind trust in the leading authority of clinicians •Variations in practice |
Scientific evidence | •Valuable new insights into the (cost-) effectiveness of medical interventions under well-controlled conditions | •Often derived from clinical trials with typically selected groups of patients |
•Difficult to capture personal preferences | ||
•Limited generalizability | ||
•Brief follow up and offering only a fragmented ‘’snapshot” view | ||
•Usually episodically assessment within a hospital setting where patients may behave differently from their usual performance | ||
Patient preferences | •Decision more tailed to individual preferences | •Challenging task on its own |
•Better therapeutic compliance | •Difficult to capture in exact measures | |
Big data approaches | •Unique perspective of an individual | •Challenging to find suitable datasets |
•Personalized prognosis, treatment predictions and adverse effects | •Leans on clinical expertise •Challenging to use outcomes in daily practice | |
•Support for clinicians and patients |
CHALLENGES TO OVERCOME
The challenge remains to integrate and turn the vast amounts of data from diverse sources into meaningful information that can contribute to the decision-making process to improve the value of healthcare for patients. There are several specific issues that need to be addressed before quadruple decision making can meet its expectations.
First, the types of decisions in PD that could benefit from a personalized prediction need to be identified. Decisions should be clinically relevant. Moreover, not every decision is sensitive to the type of predictive information that is yielded by a predictive model [47]. Thereby, it is easier to make predictions for simple, binary decisions than to predict outcomes for complex, multifactorial decisions. In any case, results from predictive models should be evaluated in the clinical context to judge whether outcomes are relevant and accurate. More research is needed to identify the information gap that professionals and patients experience to make personalized decisions in daily life.
Second, finding suitable datasets is a major challenge. Big data is not necessarily equal to good data and potential datasets need to be evaluated critically for clinical relevance. Existing datasets often still include selected or homogeneous populations with only short follow-up periods. Moreover, different datasets usually contain different variables and different data types, which makes it difficult to combine the various sources. The data will typically be generic in nature, but there will be very little specific information on, e.g., the severity of PD. Proxies can be used as an alternative (for example, higher doses of levodopa might suggest greater disease severity), but this remains less than ideal. Also, the accuracy of the diagnosis is often not clear in existing datasets, but it is conceivable that the proportion of misclassifications is higher compared to trials conducted in specialised movement disorders centers. Another challenge is that numerical data can easily be included in different analyses, but data that are stored as text (and that often contain more detailed information on the personal context) are more difficult to include in models, and might therefore be left out. Digital tools have a large potential to unobtrusively generate big data from subjects over long periods of time. However, these tools tend to focus mainly on motor symptoms, and vulnerable populations are less likely to use modern technologies such as smartwatches or smartphones. The limited compatibility between most devices is also problematic [48]. Another issue is the quality of the data, which applies not only to patient-collected data, but also to clinical and medical research data. Many datasets, including electronic medical records, are susceptible to missing or inaccurate data. These limitations are disconcerting, because the quality of personalized predictions depends on the quality of the data from which they are derived. Future research should focus on how to make personalized predictions based on big data approaches and how to handle the limitations of observational data. When we get a more detailed insight into the limitations that existing datasets carry, it might be possible to structure future collected data in such a way that these limitations can be addressed. We might even need an entirely new profession to adequately address the challenges of integrating data-science in medicine and turning large amounts of data into meaningful information for clinical practice [49].
Third, proper interpretation of the machine learning analyses of big data leans on clinical expertise. Studying correlations between multiple variables carries a risk of creating spurious associations and knowledge of disease mechanisms should be included in order to critically evaluate the rationale of the output [50]. A well-known example of this caveat is Google Flu Trends, where Google search results were used to estimate the flu prevalence [51]. It turned out that the algorithm used correlations with seasonal terms such as ‘high school basketball’. These seasonal terms might have coincidently been hot search terms when the algorithm was trained, but they are not specifically related to the flu. Although limitations on the interpretation of machine learning analyses still have to be investigated in the field of PD, much is already known about the risks of using algorithms in healthcare fields other than PD. These insights should be used to further guide research on machine learning derived correlations between variables in PD.
Finally, using findings from big data approaches in the clinical encounter is challenging. The impact of information on clinical decision-making is, for example, influenced by the presentation format [52]. To effectively incorporate this new source of information into clinical practice, it is important that we gain a clear insight in how to best visualize the presentation of this type of information. Furthermore, to use this new source of information, doctors need to change their decision-making behaviour. They not only need to engage their patients actively in a shared decision-making process, but they also need to take the personalized predictive treatment effects into consideration in a shared decision-making process. Several studies outside the field of PD have examined the quality and the uptake of decision support systems, and these identified important barriers and facilitators for implementation [53]. A framework for implementation may include: 1) demystification of the technology, in which the sources of evidence are clarified and the impact on medical decision making is discussed; 2) familiarization of clinicians with the decision support system, including showing professionals benefits in daily activities and encourage first-hand experience; and 3) enhancement of local uptake including improving usability and integration with electronic health care records [54].
In the field of PD, there are still major challenges before this stage of quadruple decision making can be adopted in clinical practice, but an example from the field of oncology may help to illustrate this last step. Women with breast cancer receive a prognosis based on knowledge derived from clinical trials, such as tumour size or presence of any metastases. But the individual’s specific genetic make-up of the tumour material – as tested in the postoperative tissue – can help in deciding whether or not chemotherapy is going to help in improving the chance of being alive after 10 years. Would it not be great if neurologists could add an individual’s intestinal microbiome, the panel of neuron-specific proteins in the cerebrospinal fluid and the density of this patient’s social network into the overall equation that would lead to the decision whether or not to start with dopaminergic treatment in a given individual with PD?
CONCLUSION
Current decision-making is based on best available scientific evidence, professional expertise, and patient preferences. To get a step closer to medicine that is truly precise and personalized, a fourth perspective should be integrated into this decision-making process, namely the context of the individual patient. Analyses of individual-level patient data, that includes not only biophysical but also personal and social information, can provide this complimentary perspective. Personalized predictions derived from big data, using machine learning techniques and being integrated into a decision support system, can be used to help bridging the gap between evidence-based medicine and the multidimensional nature of the patient’s personal context, allowing clinicians to capture the complexity of an individual into making individually tailored clinical decisions. This is what we call quadruple decision making.
CONFLICT OF INTEREST
The authors have no conflict of interest to report.
ACKNOWLEDGMENTS
Lieneke van den Heuvel was involved in the concept design and created the initial and final draft of the manuscript. Ray Dorsey, Barbara Prainsack, Bart Post and Anne Stiggelbout provided critical edits to the manuscript. Marjan Meinders and Bas Bloem were involved in the concept design and they provided critical edits to the manuscript. All authors have approved the final article.
The researchers of the Radboudumc were supported by a center of excellence grant of the Parkinson’s Foundation. Lieneke van den Heuvel was supported by ZonMW (grant number 91215076).
REFERENCES
[1] | Sackett DL , Rosenberg WMC , Gray JAM , Haynes RB , Richardson WS ((1996) ) Evidence based medicine: What it is and what it isn’t. BMJ 312: , 71–72. |
[2] | Fox SH , Katzenschlager R , Lim SY , Barton B , de Bie RMA , Seppi K , Coelho M , Sampaio C ((2018) ) International Parkinson and movement disorder society evidence-based medicine review: Update on treatments for the motor symptoms of Parkinson’s disease. Mov Disord 33: , 1248–1266. |
[3] | Seppi K , Ray Chaudhuri K , Coelho M , Fox SH , Katzenschlager R , Perez Lloret S , Weintraub D , Sampaio C ((2019) ) Update on treatments for nonmotor symptoms of Parkinson’s disease-an evidence-based medicine review. Mov Disord 34: , 180–198. |
[4] | Ferreira JJ , Katzenschlager R , Bloem BR , Bonuccelli U , Burn D , Deuschl G , Dietrichs E , Fabbrini G , Friedman A , Kanovsky P , Kostic V , Nieuwboer A , Odin P , Poewe W , Rascol O , Sampaio C , Schupbach M , Tolosa E , Trenkwalder C , Schapira A , Berardelli A , Oertel WH ((2013) ) Summary of the recommendations of the EFNS/MDS-ES review on therapeutic management of Parkinson’s disease. Eur J Neurol 20: , 5–15. |
[5] | Wilby KJ , Johnson EG , Johnson HE , Ensom MHH ((2017) ) Evidence-based review of pharmacotherapy used for Parkinson’s disease psychosis. Ann Pharmacother 51: , 682–695. |
[6] | Vandenbroucke JP ((1999) ) Case reports in an evidence-based world. J R S Med 92: , 159–163. |
[7] | Stiggelbout AM , Van der Weijden T , De Wit MP , Frosch D , Legare F , Montori VM , Trevena L , Elwyn G ((2012) ) Shared decision making: Really putting patients at the centre of healthcare. BMJ 344: , e256. |
[8] | van der Eijk M , Nijhuis FA , Faber MJ , Bloem BR ((2013) ) Moving from physician-centered care towards patient-centered care for Parkinson’s disease patients. Parkinsonism Relat Disord 19: , 923–927. |
[9] | Titova N , Chaudhuri KR ((2017) ) Personalized medicine in Parkinson’s disease: Time to be precise. Mov Disord 32: , 1147–1154. |
[10] | Bu LL , Yang K , Xiong WX , Liu FT , Anderson B , Wang Y , Wang J ((2016) ) Toward precision medicine in Parkinson’s disease. Ann Transl Med 4: , 26. |
[11] | Liu G , Locascio JJ , Corvol JC , Boot B , Liao Z , Page K , Franco D , Burke K , Jansen IE , Trisini-Lipsanopoulos A , Winder-Rhodes S , Tanner CM , Lang AE , Eberly S , Elbaz A , Brice A , Mangone G , Ravina B , Shoulson I , Cormier-Dequaire F , Heutink P , van Hilten JJ , Barker RA , Williams-Gray CH , Marinus J , Scherzer CR ((2017) ) Prediction of cognition in Parkinson’s disease with a clinical-genetic score: A longitudinal analysis of nine cohorts. Lancet Neurol 16: , 620–629. |
[12] | Engelhardt EG , Garvelink MM , de Haes JH , van der Hoeven JJ , Smets EM , Pieterse AH , Stiggelbout AM ((2014) ) Predicting and communicating the risk of recurrence and death in women with early-stage breast cancer: A systematic review of risk prediction models. J Clin Oncol 32: , 238–250. |
[13] | Martin RC , Okonkwo OC , Hill J , Griffith HR , Triebel K , Bartolucci A , Nicholas AP , Watts RL , Stover N , Harrell LE , Clark D , Marson DC ((2008) ) Medical decision-making capacity in cognitively impaired Parkinson’s disease patients without dementia. Mov Disord 23: , 1867–1874. |
[14] | Mandl KD , Bourgeois FT ((2017) ) The evolution of patient diagnosis: From art to digital data-driven science. JAMA 318: , 1859–1860. |
[15] | De Mauro A , Greco M , Grimaldi M ((2015) ), What is Big Data? A Consensual Definition and a Review of Key Research Topics. International Conference on Integrated Information. AIP Conf. Proc. 1644: , 97–104. Doi: 10.1063/1.4907823 |
[16] | Grall-Bronnec M , Victorri-Vigneau C , Donnio Y , Leboucher J , Rousselet M , Thiabaud E , Zreika N , Derkinderen P , Challet-Bouju G ((2018) ) Dopamine agonists and impulse control disorders: A complex association. Drug Saf 41: , 19–75. |
[17] | National Research Council (US) Committee on A Framework for Developing a New Taxonomy of Disease ((2011) ) The National Academies Collection: Reports funded by National Institutes of Health In Toward Precision Medicine: Building a Knowledge Network for Biomedical Research and a New Taxonomy of Disease National Academies Press (US). National Academy of Sciences, Washington, DC. https://www.ncbi.nlm.nih.gov/pubmed/22536618 |
[18] | Fereshtehnejad SM , Zeighami Y , Dagher A , Postuma RB ((2017) ) Clinical criteria for subtyping Parkinson’s disease: Biomarkers and longitudinal progression. Brain 140: , 1959–1976. |
[19] | van Rooden SM , Heiser WJ , Kok JN , Verbaan D , van Hilten JJ , Marinus J ((2010) ) The identification of Parkinson’s disease subtypes using cluster analysis: A systematic review. Mov Disord 25: , 969–978. |
[20] | Mohl B , Berman BD , Shelton E , Tanabe J ((2017) ) Levodopa response differs in Parkinson’s motor subtypes: A task-based effective connectivity study. J Comp Neurol 525: , 2192–2201. |
[21] | Kuusimaki T , Korpela J , Pekkonen E , Martikainen MH , Antonini A , Kaasinen V ((2019) ) Deep brain stimulation for monogenic Parkinson’s disease: A systematic review. J Neurol. doi: 10.1007/s00415-019-09181-8 |
[22] | Lythe V , Athauda D , Foley J , Mencacci NE , Jahanshahi M , Cipolotti L , Hyam J , Zrinzo L , Hariz M , Hardy J , Limousin P , Foltynie T ((2017) ) GBA-associated Parkinson’s disease: Progression in a deep brain stimulation cohort. J Parkinsons Dis 7: , 635–644. |
[23] | Domingos JM , Godinho C , Dean J , Coelho M , Pinto A , Bloem BR , Ferreira JJ ((2015) ) Cognitive impairment in fall-related studies in Parkinson’s disease. J Parkinsons Dis 5: , 453–469. |
[24] | Klucken J , Kruger R , Schmidt P , Bloem BR ((2018) ) Management of Parkinson’s disease 20 years from now: Towards digital health pathways. J Parkinsons Dis 8: , S85–S94. |
[25] | Murdoch TB , Detsky AS ((2013) ) The inevitable application of big data to health care. JAMA 309: , 1351–1352. |
[26] | Prainsack B ((2014) ) Personhood and solidarity: What kind of personalized medicine do we want? Per Med 11: , 651–657. |
[27] | Institute of Medicine ((2014) ) Capturing Social and Behavioral Domains and Measures in Electronic Health Records: Phase 2. National Academies Press (US), Washington, DC, doi: 10.17226/18951 |
[28] | Silva de Lima AL , Hahn T , Evers LJW , de Vries NM , Cohen E , Afek M , Bataille L , Daeschler M , Claes K , Boroojerdi B , Terricabras D , Little MA , Baldus H , Bloem BR , Faber MJ ((2017) ) Feasibility of large-scale deployment of multiple wearable sensors in Parkinson’s disease. PLoS One 12: , e0189161. |
[29] | Zhan A , Mohan S , Tarolli C , Schneider RB , Adams JL , Sharma S , Elson MJ , Spear KL , Glidden AM , Little MA , Terzis A , Dorsey ER , Saria S ((2018) ) Using smartphones and machine learning to quantify parkinson disease severity: The mobile parkinson disease score. JAMA Neurol 75: , 876–880. |
[30] | Bot BM , Suver C , Neto EC , Kellen M , Klein A , Bare C , Doerr M , Pratap A , Wilbanks J , Dorsey ER , Friend SH , Trister AD ((2016) ) The mPower study, Parkinson disease mobile data collected using ResearchKit. Sci Data 3: , 160011. |
[31] | Espay AJ , Hausdorff JM , Sanchez-Ferro A , Klucken J , Merola A , Bonato P , Paul SS , Horak FB , Vizcarra JA , Mestre TA , Reilmann R , Nieuwboer A , Dorsey ER , Rochester L , Bloem BR , Maetzler W ((2019) ) A roadmap for implementation of patient-centered digital outcome measures in Parkinson’s disease obtained using mobile health technologies. Mov Disord 34: , 657–663. |
[32] | Bloem BR , Ypinga JHL , Willis A , Canning CG , Barker RA , Munneke M , De Vries NM ((2018) ) Using medical claims analyses to understand interventions for Parkinson patients. J Parkinsons Dis 8: , 45–58. |
[33] | Ypinga JHL , de Vries NM , Boonen L , Koolman X , Munneke M , Zwinderman AH , Bloem BR ((2018) ) Effectiveness and costs of specialised physiotherapy given via ParkinsonNet: A retrospective analysis of medical claims data. Lancet Neurol 17: , 153–161. |
[34] | Wicks P , Vaughan TE , Massagli MP , Heywood J ((2011) ) Accelerated clinical discovery using self-reported patient data collected online and a patient-matching algorithm. Nat Biotechnol 29: , 411–414. |
[35] | ((2011) ) The Parkinson Progression Marker Initiative (PPMI). Prog Neurobiol 95: , 629–635. |
[36] | Oxford Parkinson’s Disease Centre. https://opdc.medsci.ox.ac.uk/home, Accessed February 02. |
[37] | Parkinson’s Foundation: Better Lives. Together. http://parkinson.org/, Accessed February 02. |
[38] | Nalls MA , McLean CY , Rick J , Eberly S , Hutten SJ , Gwinn K , Sutherland M , Martinez M , Heutink P , Williams NM , Hardy J , Gasser T , Brice A , Price TR , Nicolas A , Keller MF , Molony C , Gibbs JR , Chen-Plotkin A , Suh E , Letson C , Fiandaca MS , Mapstone M , Federoff HJ , Noyce AJ , Morris H , Van Deerlin VM , Weintraub D , Zabetian C , Hernandez DG , Lesage S , Mullins M , Conley ED , Northover CA , Frasier M , Marek K , Day-Williams AG , Stone DJ , Ioannidis JP , Singleton AB ((2015) ) Diagnosis of Parkinson’s disease on the basis of clinical and genetic classification: A population-based modelling study. Lancet Neurol 14: , 1002–1009. |
[39] | Parkinsons Disease Digital Biomarker DREAM Challenge https://www.synapse.org/#!Synapse:syn8717496, Accessed September 19. |
[40] | Shamir RR , Dolber T , Noecker AM , Walter BL , McIntyre CC ((2015) ) Machine learning approach to optimizing combined stimulation and medication therapies for Parkinson’s disease. Brain Stimul 8: , 1025–1032. |
[41] | Dinov ID , Heavner B , Tang M , Glusman G , Chard K , Darcy M , Madduri R , Pa J , Spino C , Kesselman C , Foster I , Deutsch EW , Price ND , Van Horn JD , Ames J , Clark K , Hood L , Hampstead BM , Dauer W , Toga AW ((2016) ) Predictive big data analytics: A study of Parkinson’s disease using large, complex, heterogeneous, incongruent, multi-source and incomplete observations. PLoS One 11: , e0157077. |
[42] | Evers LJW , Krijthe JW , Meinders MJ , Bloem BR , Heskes TM ((2019) ) Measuring Parkinson’s disease over time: The real-world within-subject reliability of the MDS-UPDRS. Mov Disord. doi: 10.1002/mds.27790 |
[43] | Candido dos Reis FJ , Wishart GC , Dicks EM , Greenberg D , Rashbass J , Schmidt MK , van den Broek AJ , Ellis IO , Green A , Rakha E , Maishman T , Eccles DM , Pharoah PDP ((2017) ) An updated PREDICT breast cancer prognostication and treatment benefit prediction model with independent validation. Breast Cancer Res 19: , 58. |
[44] | van Hooff ML , van Loon J , van Limbeek J , de Kleuver M ((2014) ) The Nijmegen Decision Tool for Chronic Low Back Pain. Development of a clinical decision tool for secondary or tertiary spine care specialists. PLoS One 9: , e104226. |
[45] | Koutkias V , Bouaud J ((2018) ) Contributions from the 2017 literature on clinical decision support. Yearb Med Inform 27: , 122–128. |
[46] | Devinsky O , Dilley C , Ozery-Flato M , Aharonov R , Goldschmidt Y , Rosen-Zvi M , Clark C , Fritz P ((2016) ) Changing the approach to treatment choice in epilepsy using big data. Epilepsy Behav 56: , 32–37. |
[47] | Shah ND , Steyerberg EW , Kent DM ((2018) ) Big data and predictive analytics: Recalibrating expectations. JAMA 320: , 27–28. |
[48] | Espay AJ , Bonato P , Nahab FB , Maetzler W , Dean JM , Klucken J , Eskofier BM , Merola A , Horak F , Lang AE , Reilmann R , Giuffrida J , Nieuwboer A , Horne M , Little MA , Litvan I , Simuni T , Dorsey ER , Burack MA , Kubota K , Kamondi A , Godinho C , Daneault JF , Mitsi G , Krinke L , Hausdorff JM , Bloem BR , Papapetropoulos S ((2016) ) Technology in Parkinson’s disease: Challenges and opportunities. Mov Disord 31: , 1272–1282. |
[49] | Fiske A , Buyx A , Prainsack B ((2019) ) Health information counselors: A new profession for the age of big data. Acad Med 94: , 37–41. |
[50] | Cabitza F , Rasoini R , Gensini GF ((2017) ) Unintended consequences of machine learning in medicine. JAMA 318: , 517–518. |
[51] | Lazer D , Kennedy R , King G , Vespignani A ((2014) ) The parable of Google flu: Traps in big data analysis. Science 343: , 1203–1205. |
[52] | Ben-Shlomo Y , Collin SM , Quekett J , Sterne JA , Whiting P ((2015) ) Presentation of diagnostic information to doctors may change their interpretation and clinical management: A web-based randomised controlled trial. PLoS One 10: , e0128637. |
[53] | Elwyn G , O’Connor A , Stacey D , Volk R , Edwards A , Coulter A , Thomson R , Barratt A , Barry M , Bernstein S , Butow P , Clarke A , Entwistle V , Feldman-Stewart D , Holmes-Rovner M , Llewellyn-Thomas H , Moumjid N , Mulley A , Ruland C , Sepucha K , Sykes A , Whelan T ((2006) ) Developing a quality criteria framework for patient decision aids: Online international Delphi consensus process. BMJ 333: , 417. |
[54] | Liberati EG , Ruggiero F , Galuppo L , Gorli M , Gonzalez-Lorenzo M , Maraldi M , Ruggieri P , Polo Friz H , Scaratti G , Kwag KH , Vespignani R , Moja L ((2017) ) What hinders the uptake of computerized decision support systems in hospitals? A qualitative study and framework for implementation. Implement Sci 12: , 113. |
[55] | Greenhalgh T , Howick J , Maskrey N ((2014) ) Evidence based medicine: A movement in crisis? BMJ 348: , g3725. |