How is Physical Activity Measured in Spinal Muscular Atrophy and Duchenne Muscular Dystrophy?
Abstract
Background:
Physical activity (PA) provides many substantial benefits to help reduce risk for cardiometabolic disease, improve cognitive function, and improve quality of life. Individuals with neuromuscular disorders (NMDs), such as spinal muscular atrophy (SMA) and Duchenne muscular dystrophy (DMD) are characterized by muscular weakness and fatigue, which limits the capacity to reach the recommended guidelines of PA. Measuring PA in these populations can provide insight to participation in daily activities, track disease progression, and monitor efficacy of drug treatments.
Objective:
The objective of this study was to identify how PA is measured in SMA and DMD using instrumented and self-report methods, and how these methods are employed in ambulatory and non-ambulatory groups.
Methods:
A scoping review was performed to identify studies that reported PA in these neuromuscular disorders. Inclusion was determined after a multi-stage review process by several reviewers, followed by an in-depth analysis of metrics reported by each tool that was used.
Results:
A total of nineteen studies were identified and included in this review. Sixteen studies included instrumented measures and four studies utilized self-reported measures, with eleven studies also reporting PA information from a non-ambulatory group. A variety of metrics have been reported using both classes of measurement tools.
Conclusion:
Although a wide variety of research exists that details both instrumented and self-reported measurement tools, feasibility, cost, and study aims are important factors to consider in addition to testing methodology when selecting which type of tool to use. We recommend using a combination of instrumented and self-report measures to provide context to the PA measured in these populations. Improvements in both instrumented and self-report methodologies will add valuable knowledge about the disease burden and efficacy of treatment and disease management methods in SMA and DMD.
INTRODUCTION
Neuromuscular disorders (NMD) are a group of diseases that impact muscle and motor function and can be characterized by muscular weakness and fatigue [1]. Many NMDs limit ambulatory capacity, which may worsen as these individuals age [2]. Spinal muscular atrophy (SMA) and Duchenne muscular dystrophy (DMD) are two NMD’s that present with lower body muscle weakness and impaired motor function [3]. SMA is an autosomal recessively inherited neuromuscular disorder caused by reduced SMN protein resulting from a deletion of the SMN1 gene on chromosome 5q13, and decreased production of full length SMN protein from an imperfect homologue SMN2 gene [4]. DMD is the most common inherited dystrophinopathy, caused by lack in production of the structural protein dystrophin. The estimated incidence of SMA is approximately 1 in 5000 and approximately 1-2 per 10,000 people for DMD [5, 6]. As these diseases progress, muscle weakness increases which can reduce participation in physical activities, leading to an increase in sedentary behavior and adversely affect health [7, 8].
The myriad of health benefits from physical activity (PA) is indisputable and include reduction of risks of developing chronic diseases such as cardiometabolic diseases and cancer, and improves mental health, cognition, sleep, and quality of life. According to the World Health Organization (WHO) physical inactivity is the fourth leading contributory cause of deaths associated with chronic diseases worldwide. For these reasons, participation in PA is encouraged by global public health agencies for all people. However, approximately 31% of the population globally do not meet the recommended amounts of PA, and this number is considerably lower in individuals with disability. Among adults with disability or functional limitations ∼22–26% meet the recommendations for aerobic and muscle strengthening PA [9]. One study of boys living with DMD showed that they were less physically active than healthy boys of the same age, and that they completed less time in moderate and high intensity activity and recorded fewer total steps [10].
Current PA guidelines recommend that children and adolescents 5–17 years of age living with disability should participate in 60 minutes per day of moderate to vigorous PA, and on 3 days per week engage in vigorous PA as well as muscle and bone strengthening exercises [11]. Adults ages 18 years and older should engage in 150-300 minutes of moderate to vigorous PA per week, or 75-150 minutes of vigorous intensity PA per week, as well as incorporating muscle and bone strengthening exercises on at least 2 days per week [12]. Additional recommendations include limiting sedentary time and engaging in at least light intensity PA as much as possible. When mobility is an obstacle to PA, participation in various types of upper extremity exercises and other adaptations to exercise according to disease and ability status is also recommended.
Many individuals with NMD cannot reach these thresholds, but, as the benefits of PA accrue in a dose-response manner, any amount of PA is more beneficial than time spent sedentary, and even minimal amounts of PA can reduce the detrimental effects of increased sedentary time [13]. In SMA and DMD, proximal muscle weakness may limit the ability for those affected to reach the recommended PA levels, but the diminished participation in PA also can exacerbate muscular weakness, muscle wasting, and reduced muscle quality, potentially creating a spiral toward disability. A previous study determined that between 1999 and 2019, less than 5% of all articles published in the five highest-impact medical journals focused on people living with disabilities, and less than 7% of those articles specifically addressed PA [14]. The ability to measure PA in people living with disability can provide further insight into exercise participation, free-living daily physical activities, track disease progression, and evaluate the efficacy of therapies and treatments. Measuring PA is complex in people with disability, and existing work is inconsistent in DMD and limited in SMA.
PA measurement tools can be categorized as either instrumented measures or self-report measures. Instrumented measures include devices such as accelerometers that measure movement in single or multiple planes, or heart rate monitors (e.g., Polar devices) that detect changes in movement intensity. Gyroscopes or inclinometers measure body position, global positioning systems (GPS) track distance, and simple pedometers track step counts. Newer technologies combine multiple sensors, such as accelerometers and heart rate or GPS, which can improve PA measurement and allow for measurement over longer durations, ideally capturing data that better reflects true out-of-lab activity. Each of these technologies have limitations in the type of activities that can be detected and in the various methods of interpretation of the data obtained. Instrumented measures do not involve participant recall or responses, and thus, are often termed “objective” measures. Self-report measures include questionnaires and activity logs. Questionnaires ask about previous PA over a set time frame (e.g., day, week, month, etc.) and activity logs are recorded daily; these methods are reliant on respondent’s perceptions and recall. There is a wide array of self-report instruments available in many languages and of varying lengths. These include questionnaires designed for use in general populations or specific groups such as children, older adults, and people with disability. Both instrumented and self-report measures can be applied to ambulatory or non-ambulatory individuals. While both methods have advantages and drawbacks, and improved instruments continue to be developed, no true “gold standard” measurement of PA exists [15].,
REVIEW AIMS
The aims of this review were [1] to examine the various instrumented and self-report tools used to report PA in people living with SMA and DMD, and [2] to describe tools used to evaluate PA in ambulatory and non-ambulatory people with SMA and DMD.
The protocol for this review was registered with PROSPERO International Prospective Register of Systematic Reviews database [16].
METHODOLOGY
The Cochrane Handbook for Systematic Reviews and Meta-Analyses was used as a guideline to perform a scoping review of three databases: PubMed, EMBASE, and Web of Science [17]. A scoping methodology was used as the research aims were broad and the goal was to include as many articles as possible in the review. The search strategy identified keywords associated with PA, including “physical activity”, “movement”, and “exercise”. These terms were used along with keywords associated with both instrumented and self-reported measures, including “surveys”, “questionnaires”, “actigraphy”, “actimetry”, and “pedometry”. These terms were combined to search for studies that included participants with “neuromuscular disease”, “spinal muscular atrophy” and “Duchenne muscular dystrophy”.
Studies were evaluated and determined suitable for possible inclusion if they met the following criteria [1]: Participants were diagnosed with either SMA or DMD, and [2] included either instrumented or self-report tools designed to measure PA. Studies were excluded if they [1]: were not published in English [2]; measured physical function rather than PA [3]; reported results with mixed neuromuscular disorder groups [4]; were conference abstracts, case studies, reviews, or non-peer reviewed research studies.
An initial search was completed to evaluate articles published up to October 2020. All titles and abstracts were screened by two independent reviewers (DU and LY) to determine if they met inclusion criteria. A full text screening was completed by the same two independent reviewers, and any conflicts were discussed with an independent third reviewer (JM). Following a full text review of included articles, another round of backwards and forwards snowball searching was completed to find additional articles through April 2021. The search was repeated in January 2022 to capture the most recent publications. The remaining studies were analyzed, and the necessary information was extracted from each study by two independent evaluators (DU and LY). The PRISMA-P checklist was utilized to help synthesize general results.
Instrumented PA Assessment Tools: All studies that utilized an instrumented PA assessment tool were assessed for the following variables: make and model of device, placement of device, data collection time frame, amount of time worn per day, number of days worn, criteria for non-wear time, definition of valid and non-valid days, sampling frequency, epoch length, variables created or manipulated from collected data, reported total activity count, definition of sedentary time and intensity levels, cut point method used, axes included, use of supplementary PA tool, and time of the year the study was conducted.
Self-report Outcome Tools: All studies that utilized a self-report PA assessment tool were assessed for the following variables: name and version of the tool, administration method, length of time to recall or report, time frame, and the time of the year that the study was conducted.
RESULTS
The results of the literature search are presented in Fig. 1. The initial search yielded 4264 articles, which were evaluated using criteria mentioned above. After conducting a forward and backwards reference search on selected articles and removing duplicates, 3855 abstracts were screened. There were 68 full text articles screened, with 49 being excluded based on set criteria. Of the 49 studies excluded after full-text review, 7 studies were excluded because the data were reported in a group that did not report SMA and/or DMD separately and were mixed with unrelated conditions, 3 presented data on non-specific muscular dystrophies, and 3 studies reported on the same data set as a separate article. Ultimately, 19 articles met the inclusion criteria and were included in this review.
Fig. 1
PRISMA flow diagram.
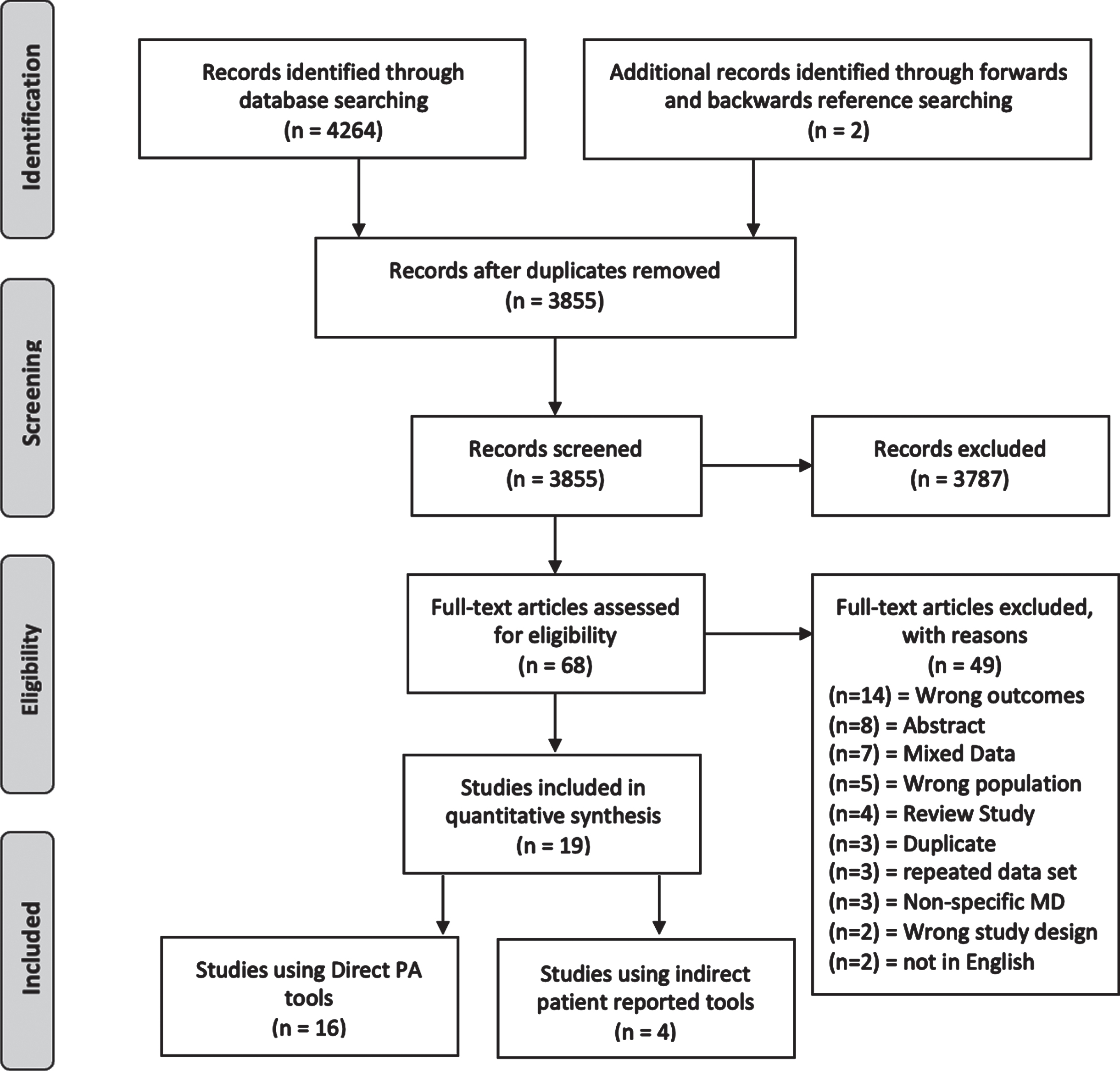
Figure 1. Literature selection flow diagram.
Table 1. Use of Instrumented and self-reported measures in SMA and DMD.
Table 1
Summary of all papers identified using direct and/or patient reported measures in SMA and DMD
Study | Population | Mean (SD) or Age range | Ambulatory Status | Number of Participants (M/F) | Primary Aims | PA Related Results |
McDonald et al. 2005 [10] | DMD | 9.1 (2.1) | Ambulatory | 16 Male | To evaluate the StepWatch activity monitor (SAM) for use in community ambulation in DMD and correlate Sam with body composition and muscle strength | DMD participants averaged 1260 + 103 steps/min in low step rate for 235 + 19 minutes, 1500 + 195 steps/min in moderate step rate for 66 + 8 min, and 1696 + 287 steps in high step rate for 43 + 7 minutes. Total sedentary time was determined to be 1095 + 23 minutes per day. |
Jeannet et al. 2011 [18] | DMD | 4-6 | Ambulatory | 5 Male | To assess feasibility and accuracy of daily PA monitoring in the home environment using this device, and to assess the use of therapeutic steroid treatment after one month of prednisone treatment | The five DMD participants reported sitting 37.4 + 3.9% of the time and walking or standing 51.9 + 8.4% of the time during 2 consecutive weekend days. The average number of steps taken for this period was 18,942 + 3137 steps. Participants took a higher number of steps at a low step rate 392 + 62 steps/min, compared to a higher step rate 81 + 13 steps min. |
Kimura et al. 2014 [19] | DMD | ambulatory=6.9 (1.3), Non-ambulatory=13.8 (2.6) | Both | 22 Male | To evaluate the utility of wrist actigraphy for estimating muscle strength in DMD | Ambulatory ZCM score was 140.3 + 30.1 counts/mi, while non-ambulatory was 96.3 + 34.3 counts/min. Ambulatory PIM was 58,750 + 15,537, and non-ambulatory PIM was 22,344 + 11,624 bits. |
Montes et al. 2014 [20] | SMA | Exercise group = 10-44, non-exercise group = 10-48 | Ambulatory | 11 | To assess the effects of exercise on measures of function, strength, and exercise capacity in ambulatory SMA patients | The exercise group spent 76.9 (71-85%) of time in sedentary activity levels. The No-exercise group spent 90 (80-100%) of time in sedentary activity levels. |
Davidson et al. 2015 [21] | DMD | 9.0 (2.1) | Ambulatory | 15 Male | To conduct a preliminary exploration into accelerometry in DMD, describe relationship between activity monitor data and standard functional assessments | The DMD group reported Inactive minutes = 1103 + 134, low minutes = 205 + 74, medium = 107 + 51, and high min = 25 + 17. The number of steps was 5138 + 2500. |
Elliot et al. 2015 [22] | DMD | 8.44 (1.90) | Ambulatory | 14 Male | To determine if parents of boys with DMD can accurately report their child’s PA compared with the reference measure of Total Energy Expenditure | Mean PAL was 1.37 + 0.18, compared to an estimated PAL of 1.45 + 0.23. Mean TEE was 1778 + 260 kcals |
de Valle et al. 2016 [23] | DMD/BMD | 11.4 (3.9) | Both | 51 Male | To document mainstream and alternative therapies provided to DMD patients and record PA level by males with DMD and BMD | Ambulatory DMD and BMB reported participating in structured PA such as swimming (51% or respondents), or “other organized sports” (38%), and unstructured PA including walking (51%), bike riding (31%, visiting a playground, (57%), horse riding or other. About 70% of parents state that PA benefited their child. While 7 of the 23 boys in the late ambulatory phase and non-ambulatory phase report no PA. |
Heutinck et al. 2017 [24] | DMD | 15.5 (6.4) | Both | 84 Male | To describe PA and perception of PA in different stages of boys with DMD | 87% of EAS reported > 6 days of light intensity, and 13% reported 1-5 days. 56% of LAS reported > 6 days, 44% 1-5 days. 43% of ENAS report > 6 days light and 43% 1-5 days. 20% of LNAS reported > 6 days, and 33% report 1-5 days. 47% of LNAS report no light intensity at all. 39% of EAS report > 6 days strenuous, 52% 1-5 days. 11% of LAS report > 6 days strenuous, 56% 1-5 days. 9% of ENAS report > 6 days strenuous, 81% 1-5days. 3% of LNAS report > 6 days, 30% 1-5 days. 33% of LAS report no strenuous, and 67% of LNAS report no strenuous. |
Chabanon et al. 2018 [25] | SMA | Non-sitter median age 14.9 IQR (6.3-17.2), Sitter median age 4.6 IQR (2.5-7.1) | 8 Non-sitters, 5 Sitters | 13 | To define the natural history of SMA | Non-sitters had a median activity percent of 46.9 IQR (40.3-50.9), and sitters had a median activity percent of 59.4 IQR (48.4-75.2) |
Fowler et al. 2018 [26] | DMD | 7.9 (2.9) | Ambulatory | 32 Male | To examine longitudinal SA in boys with DMD as part of a natural history study | Average strides per day for whole group was 4881, and the older group was 4453. Mean low frequency was 1345 (423), mean moderate was 2665 (1331), mean high was 871 (620), mean pediatric high was 144 (204) |
Jacques et al. 2018 [27] | DMD/BMD | DMD=24.2 (6.1), BMD = 42.4 (13.5) | Both | 15 DMD/18 BMD Male | To investigate the relationship between muscle strength and size in DMD, and these relationships with PA in DMD | DMD participants reported total physical activity as 13.5 + 16.1 mins, and a sedentary behavior percentage of 96.4 + 4.5%. |
Fujii et al. 2019 [28] | DMD | 12-24 | Non ambulatory | 7 Male | To assess utility and reliability of accelerometry in non-ambulatory DMD | Mean Cumulative jerk was 352.072 (range 151,906-698,372) |
Arteaga et al. 2020 [29] | DMD | 13.6 (4.0) | Both | 49 Male | Determine amount of time DMD patients spend in sedentary activity and if ambulatory DMD patients participate in greater amount of PA compared to non-ambulatory | Ambulatory DMD spent less time in sedentary activity (70.7 + 8.8) compared to non-ambulatory (91 + 7.7), and more time in Low intensity (26 + 8 to 8.7 + 7.3,) and more time in Moderate-to-vigorous (3.3 + 1.4 to 0.3 + 0.6) |
Killian et al. 2020 [30] | DMD | 13.0 (4.3) | Ambulatory | 43 Male | To examine the association of accelerometry as a measure of functional status with QMT as a measure of muscle strength in DMD | Total Awake Wrist VM was 6180 + 2690 counts in 40 participants, and Total Awake Ankle VM was 448 + 597 counts in 34 participants. |
Siegel et al. 2020 [31] | DMD | ambulatory 8.6 (2.2), non-ambulatory 14 (3.1) | Both | 23 Male | To characterize sleep disturbances and relationship to quality of life, and then use actigraphy to evaluate associations between rest-activity, sleep quality, and 6MWT performance | Represented as median(SD) the ambulatory showed IS = 0.65 (0.14), IV = 0.62 (0.13), L5 = 860 (421), M10 = 36583 (16,622). The Non-ambulatory group showed IS = 0.61 (0.14), IV = 0.71 (0.16), L5 = 507 (774), M10 = 11846 (7907) |
Van der Geest et al. 2020 [32] | DMD | 124 (3.2) | Both | 16 Male | To evaluate the validity of home measurements of upper extremity accelerometry | The group with the lower Brooke score recorded a lower arm activity count of 378 + 119 counts/min, and an upper arm count of 279 + 84 counts/min. The group with the high Brook’s score recorded lower arm activity counts of 166 + 35 counts/min and upper arm count of 138 counts/min. |
Jacque et al. 2021 [33] | BMD | 33.7 (12.2) | Ambulatory | 7 Male | To assess sedentary behavior and physical activity in ambulatory adults with BMD as well as sitting and standing time in ambulatory adults compared to a healthy control group | The BMD group recorded Mean(SD) 14.8 (1.7) hours of Waking Time, 10.7 (2.7) hours of sedentary behavior, 4.1 (1.2) hours of Physical Activity. From posture classification, the BMD group recorded 9.9 (2.8) hours of sitting time and 5.0 (1.6) hours of standing time. |
Lott et al. 2021 [34] | DMD | 8.7 (2.0) | Ambulatory | 50 males | To determine the amount of time needed to assess step activity in DMD boys were remained ambulatory 2 years later vs those who lost ambulation | The mean steps reported for the total DMD group was 5147 (2145) steps. The 5-7 age groups recorded 7033 (1957) steps, the 7-9 age group 5055(1889) steps, the 9-11 age group recorded 4443(1587) steps, and the 11-13 age group recorded 3385 (1522) steps. |
McErlane et al. 2021 [35] | DMD | 9-15 | NA | 8 Male | To determine the utility of wearable technologies in PA assessment in DMD | The DMD cohort recorded 903.4 average daily maximum steps, 4919 average daily maximum steps, and 246.3 average steps per 30 min epoch. |
Selected study characteristics
As shown in Table 1, of the selected studies included in this review, seventeen reported on DMD, and two reported on SMA. Across both conditions, the study sample sizes ranged from 5-84 participants, with ages ranging from 4-44 years. The qualification of genetic diagnosis or muscle biopsy was required in sixteen of the nineteen studies. Sixteen studies utilized instrumented measures and three utilized self-reported measures. Eleven studies included a non-ambulatory subgroup. Eighteen studies had an observational study design, one study was a randomized control trial (RCT) to evaluate a specific intervention. In the RCT, PA was not the primary aim of the study, and only baseline PA measures were extracted in this review.
Table 2: Instrumented PA measurement tools in SMA and DMD.
Table 2
Summary of direct measurement tools used to quantify PA in NMD
Study | Make and model | Monitor placement | Minimum number of valid days/data points | Weekdays or weekends or both | Sampling frequency | Epoch |
McDonald et al. 2005 [10] | StepWatch | Right Ankle | 3 days | both | 1 min | |
Jeannet et al. 2011 [18] | ASUR autonomous sensing unit recorder, 55x40x18 mm, 50 g) | Chest | 2 days | Weekend | 25 Hz | |
Kimura et al. 2014 [19] | Motionlogger Watch; ambulatory monitoring | Non-dominant wrist | 7 days | both | 10 Hz | 1 min |
Montes et al. 2014 [20] | ActiLife 5 | Waist and each ankle | 7 days | both | 10 hz | |
Davidson et al. 2015 [21] | StepWatch | ankle | 4 full days | 1 min | ||
Chabanon et al. 2018 [25] | ActiMyo | Wrist and wheelchair | 180 hours | both | ||
Fowler et al. 2018 [26] | StepWatch | ankle | 5 days | both | 1 min | |
Jacques et al. 2018 [27] | GENEActive | Preferred wrist | 24 bours | both | 100 Hz | 1 min |
Fuji et al. 2019 [28] | Silmee Bar-type Light (Toshiba) | Dorsal face of dominant forearm near wrist | 8 hours | Weekday | 15.625 Hz | |
Arteaga et al. 2020 [29] | Actigraph GTX3 | Dominant ankle and wrist | ≥3 days with≥2 weekdays and≥1 weekend day, each with≥10 hours of wear from 6: 00 am to 9: 00 pm. | Both | 30 Hz | Wrist accelerometers 1 min epoch |
Killian et al. 2020 [30] | Actigraph GTX3 | Dominant wrist and ankle | ≥3 days with≥2 weekdays and≥1 weekend day, each with≥6 hours of wear from 7am-10pm | both | 30 Hz | 15 seconds |
Siegel et al. 2020 [31] | Actiwatch 2 | Non-dominant wrist | 5 hours per day for 10 days | both | 40 counts/epoch | 30 seconds |
Van der Geest et al. 2020 [32] | MOX Accelerometry | Upper arm, lower arm, wheelchair/trousers | at least 1 day | 25 Hz | 1 min | |
Jacques et al. 2021 [33] | GENEActive | Dominant wrist | 7 days | both | 100 Hz | 15 |
Lott et al. 2021 [34] | Actigraph GTX3 | Right waist | 2 weekend, 1 weekday | both | 30 Hz | 15 seconds |
McErlane et al. 2021 [35] | Novel wearable device (unnamed) | wrist | 12 weeks | both | 30 min |
Study | Variables created/manipulated from Data | Reported total counts (Steps or activity) | Definition of sedentary time low intensity, MVPA | Sedentary Time | Cut point method used | Axes used |
McDonald et al. 2005 [10] | inactive=0 steps/min, low activity = 1-15, medium = 16-30, high = more than 30 | Yes | inactive=0 steps/min, low activity = 1-15, medium = 16-30, high = more than 30 | Yes | inactive=0 steps/min, low activity = 1-15, medium = 16-30, high = more than 30 | |
Jeannet et al. 2011 [18] | Posture parameters,Walking parameters: | Yes | inactive=0 steps/min, low activity = 1-15, medium = 16-30, high = 31-60, very high=>60 steps/min | Yes | inactive=0 steps/min, low activity = 1-15, medium = 16-30, high = 31-60, very high=>60 steps/min | 3 |
Kimura et al. 2014 [19] | ZCM (Zero crosing method), PIM (Proportional Integration Measure) | Yes (ZCM is a method of frequency of a movement) | ||||
Montes et al. 2014 [20] | % of time spent sedentary | Yes | 3 | |||
Davidson et al. 2015 [21] | inactive=0 steps/min, low activity = 1-15, medium = 16-30, high = more than 30 | Yes | inactive=0 steps/min, low activity = 1-15, medium = 16-30, high = more than 30 | inactive=0 steps/min, low activity = 1-15, medium = 16-30, high = more than 30 | ||
Chabanon et al. 2018 [25] | % of time spent sedentary | Yes | 3 | |||
Fowler et al. 2018 [26] | low activity = 1-15, medium = 16-40, high = more than 40, Mean strides/day, number of strides at each level,>60 = high for pediatric, % of low, moderate and high in pediatric | Yes | Step count levels | Yes | Step count levels | |
Jacques et al. 2018 [27] | Total time spent physically active (TPA mins), Sedentary behavior % (SB) | Yes | Due to data processing restrictions, individual intensity levels not processed, PA only represented with TPA | Yes, SB represented as a percentage of waking time | only TPA recorded | 3 |
Fuji et al. 2019 [28] | Jerk=rate of change of acceleration, J = change in G x frequency. Composite vector Jerk is the sum of 3 axis (Cumulative Jerk Sum; Cj) | Yes, Cj | The unit of acceleration in this device is G (9.81 m/s2), and the measurable range is -2 G -+2 G. The lowest detectable range is 3.91 mG | 3 | ||
Arteaga et al. 2020 [29] | minutes per day of wearing an accelerometer (min/day wear), minutes per day of wearing and awake (min/day awake), Total vector magnitude, vector magnitudes per min, vector magnitude during wake time | Sedentary 1 = 0-119 VM/min, Sedentary 2 = 120-1000, Sedentary 3 = 1001-3660, Low Intensity 1 = 3663-4912, Low Intensity 2 = 4913-9804, MVPA=>9804 | 0-3660 VM/min, 1-2 METS | Cut-points averaged to calculate the subcategory | 3 | |
Killian et al. 2020 [30] | Vector magnitude sums of each axis (VM), Total ankle VM (counts per minute), total wrist VM, Awake ankle VM, awake wrist VM | Cut-points in VM/15 s. Calculated subcategory | ||||
Siegel et al. 2020 [31] | Inter-daily stability (IS), intra-daily variability (IV), most active 10 hours (M10), least active 5 hours (L5) | Definition of 5-hour sedentary time, but not throughout the day | ||||
Van der Geest et al. 2020 [32] | intensity, level of arm elevation (orientation), and elevation rate (frequency of arm elevation) | Transfer of arm elevation, which was the frequency of elevation of the arm from low to middle elevation and from middle to high elevation. | ||||
Jacques et al. 2021 [33] | posture classification, Waking Hours Physical Activity (sedentary, Light, Moderate, Vigorous) | Sedentary<1.5 METS, light = 1.5-3.99 METS, moderate = 4-6.99 METS, vigorous=+7 METS | Yes | Left side: sedentary < 217, light = 217-644, moderate = 645-1810, vigorous=>1810 … ...Right: sedentary=<386, light = 386-439, moderate = 4402098, Vigorous=+2098 | 3 | |
Lott et al. 2021 [34] | Step count | Yes | 3 | |||
McErlane et al. 2021 [35] | Average daily maximum steps, average daily steps, average steps per epoch | Yes | 3 |
Instrumented measures
There were sixteen studies selected that used instrumented measures. Characteristics of these studies and the eleven different devices used are represented in Table 2. The ActiGraph ActiLife 5 (ActiGraph, Pensacola, FL, USA), a triaxial accelerometer was used to report sedentary time [20]. Studies using the ActiGraph GTX3 (ActiGraph, Pensacola, FL, USA), a triaxial accelerometer, reported total wrist and ankle vector magnitude counts (omnidirectional acceleration), total steps, and time spent in sedentary, low/moderate/high level PA [29, 30, 34]. A device known as the ActiMyo (Sysnav, Vernon, France), contains a triaxial accelerometer and gyroscope, was used to report the median values of wrist acceleration [25]. The ActiWatch 2 (AW2, Philips Respironics, Bend, OR USA), an actigraphy device, recorded activity in the least active 5 hours, as well as the most active 10 hours [31]. The ASUR measures changes in body position through accelerometry was used to report percentage of time spent sitting, average steps per day, and the number of steps per minute [18]. The GENEActiv device (Cambridge, United Kingdom), another triaxial accelerometer, was able to report total time of PA, percent of time spent sedentary, average sitting time, and average PA time [27, 33]. The Motionlogger watch (Ambulatory Monitoring, Ardsley, NY, USA), another triaxial accelerometer, was utilized to report absolute ZCM (zero crossing mode) as a measure of frequency, and PIM (proportional integration mode) as a measure of intensities [19]. ZCM is a count of the number of times that a movement crosses a threshold, zero in this case, and is a measure of movement frequency. PIM is the calculated area under the curve for a time period and is a measure of intensity during a given time period. The MOX accelerometer (Maastricht Instruments BV, Maastricht, the Netherlands), a three-dimensional accelerometer, used in one study reported upper arm acceleration in the form of total activity counts [32]. The study using the Silmee Bar-type light biologic sensor (TDK, Tokyo, Japan), a triaxial accelerometer, reported mean cumulative jerk, or rate of change in acceleration, specifically the upper extremity in this study [28]. The devices included the StepWatch activity monitor (Orthocare Innovations, Washington, USA), a device designed specifically to measure cadence, was used to report a combination of sedentary time, cadence, total sedentary time in low/moderate/high intensity PA, total steps per day, and average steps/day [10, 21, 26]. And lastly, an unnamed non-commercially available three-dimensional accelerometer was used in one study to measure maximum daily steps, average daily steps, and steps in 30 min periods [35]. No study reported in this review used a consumer-grade device, while the ASUR was noted as a non-commercialized device. All devices, except for the unnamed 3-D accelerometer, could be classified as a research grade device at the time of the study.
Table 3. Self-reported PA Tools in SMA and DMD.
Table 3
Summary of indirect patient report measurement tools used to quantify PA in NMD
Study | Tool used | Administration Method | Population | Time Frame | variables created/manipulated from data | Definition of sedentary time, low intensity, MVPA | Sedentary Time |
Montes et al 2014 [20] | Activity log | self report | 11 SMA | 7 days | Yes | ||
Elliot et al. 2015 [22] | Modified activity diary | Self report | 14 DMD | 2 weekday+2 weekend | Categorization of activities into 9 levels based on MET values, Total daily METs, estimated PAL (total energy expenditure divided by resting energy expenditure) | PA of less than 1.37 | Yes |
de Valle et al. 2016 [23] | Self made survey | Report by parents | 51 DMD/BMD | As of that day | Categorization by ability to walk 500, 50, or 5 meters, or are unable to walk | ||
Heutinck et al. 2017 [24] | Custom PA Questionnaire | Report by parents | 84 DMD | Previous 14 days | physical activity minutes per class during school, swimming minutes per class at school | Light intensity = do not increase heart rate or strenuous breathing = increased breathing and heart rate | Measured by screen time |
Self-reported measures
There were four studies using self-reported outcome measures as shown in Table 3. These studies included an activity log which measured sedentary time [20]. A second study utilized a PA diary [22]. which calculated total daily METs based on values from a compendium of PA [36]. and yielded an estimated PA level (PAL). A third study used a self-designed survey [23]. which asked questions regarding structured and unstructured PA. The fourth study included a customized PA questionnaire [24]. which reported the number of days per week specific physical activities were completed. The PAs included a range of light, moderate and high intensity activities such as tai chi, soccer, and snow skiing. A composite score based on time completing the activity along with its corresponding MET value was calculated. The activity log and modified diary were completed by the participant, while the self-made survey and customized PA survey were completed by the parent/guardian.
Table 4: Use of PA tools in Ambulatory and Non-ambulatory SMA and DMD.
Table 4
Summary of all tools used with ambulatory and non-ambulatory participants
Ambulatory | |
Direct Tools | Indirect Patient Reported Tools |
Actigraph GT3X | self made questionnaire |
Motionlogger Watch | modified activity diary |
Actiwatch 2 | PA questionnaire |
StepWatch | activity log |
GENEActive | |
ASUR | |
MOX Accelerometry | |
ActiLife 5 | |
Non-Ambulatory | |
Direct Tools | Indirect Patient Reported Tools |
Actigraph GT3X | self made questionnaire |
Silmee Bar-type Light | modified activity diary |
Motionlogger Watch | PA questionnaire |
Actiwatch 2 | |
GENEActive | |
MOX Accelerometry | |
Actimyo |
Ambulatory and non-ambulatory measures
Of the selected studies, a total of ten tools consisting of both instrumented and self-report tools were used in a least a subset of participants to assess non-ambulatory individuals, as shown in Table 4. In order to be classified in the non-ambulatory subgroup, participants must report at least partial wheelchair use [19, 23, 24, 27–29, 31–33]. The selected studies included participants using manual or motorized wheelchair use, and one study reported the use of unspecified “mobility aids [27]”.
There were three devices that were exclusive to the ambulatory group: StepWatch [10, 21, 26], the ActiGraph [20, 29, 30, 34], and the ASUR [18]. The StepWatch, ActiGraph, and ASUR were placed on the ankle, waist and ankle, and chest, respectively. The tools used with a both ambulatory and non-ambulatory groups included the Silmee Bar type Light [28], MOX accelerometry [32], and the ActiMyo (Sysnav, Vernon, France) [25]. The Silmee Bar type Light was placed on the forearm, the MOX accelerometry device was placed on the upper and lower arm as well as the wheelchair, and the ActiMyo was used by placing one device on the wrist of the participant and one device fixed to their wheelchair. These devices measured activity of the arm in relation to a resting position, rate of change in arm acceleration (cumulative jerk), or movement counts [37]. Each type of self-report tool was used in both ambulatory and non-ambulatory participants.
DISCUSSION
This scoping review highlights the variety of tools that are currently being used to report PA in SMA and DMD, using both instrumented and self-report outcome measures. Instrumented measurement tools can incorporate a wide variety of variables and settings when processing data, including device placement, length of data collection, sampling frequency, epoch length, total activity count, and a clear definition for non-valid data. The variation in these metrics between studies can make it challenging to compare data across studies.
As shown in Table 4, the studies that used a form of accelerometer, placement varied from wrist [10, 19, 25, 27–31, 35], waist [20] chest [18] or ankle [10, 20, 21, 26, 29, 30]. Placement is an important variable to consider, especially when evaluating populations where ambulatory status may differ. For example, upper extremity activities would need to be captured if lower body ambulation is limited, but only if the accelerometer is placed to be able to capture the signals that can be interpreted as movement. There were also variations in dominant side or preferred side, or fixed to arm of the wheelchair, which may be considered when interpreting data [25, 32]. In general, the algorithms used by the devices to process the data were developed for specific anatomical placement of the accelerometer. Using the device on the body aside from its intended location could negatively impact the accuracy of the results. Error of measurement can result in of over/underestimating activity, not capturing activity entirely, and misclassifying intensity of different activities. For use in non-ambulatory DMD and SMA, using multiple accelerometers with one placed on the wheelchair and one on the wrist might improve the accuracy of the results, as was shown in a previous study [38].
In addition to device placement, there are variations in cut points based on the vector magnitudes which represent a summation of accelerations in all planes of measurement, in a given time frame, additional variables synthesized, epoch length, and data collection time frame/days when using different devices. Cut point methods to estimate PA intensity and sedentary time have been developed according to age group. (e.g., preschool, children, adults, older adults) and for use in general populations and in specific populations such as in people living with cerebral palsy, Parkinson’s disease, and others [39]. Commonly employed accelerometers have the capability to measure step count and vector magnitude, and PA intensity, and proprietary data analysis software comes preprogrammed with evidence-based data collection and processing strategies such as selection of epoch, cut points, wear time, and other methodologies. In addition, there are open-source data analysis code available in software such as RStudio (RStudio, Inc., Boston, MA) and MATLAB (MathWorks Inc. Natick, Massachusetts).
Activity monitors have been shown in some cases to overestimate distance traveled when GPS was also available, as well display a higher degree of variability in vigorous intensity exercise when compared to self-report measures [40]. Although the results of the studies which use instrumented measures were heterogeneous, the overall results of the studies reviewed generally reported typical measures of PA, including total steps and sedentary time, which can make it easier to compare results between these studies. Fewer studies evaluated ZCM, cumulative jerk, and changes in posture. Postural changes are more easily measured in devices which incorporate an inclinometer, which have become standard in accelerometry devices at this point. This is important for estimation of sedentary time.
In the four studies that evaluated self-reported measures, two studies asked participants about activities from the previous seven days [20, 22], one study asked about activities they usually complete [24] and one study asked about activities and therapies that they received in the previous year [23]. Variability in recall, different definitions of PA, and the manner in which questions are asked can influence the results. The lack of consistency affects the ability to compare data collected between different tools.
A previous review, which compared instrumented and self-report measures, determined that self-report measures may underestimate PA by an average of 1.74 hours/day compared to instrumented measures [41]. This same review also found a significant degree of variability in results reported by self-report measures, which can be interpreted as a lack of reproducibility in the results. They specified that using a single-item to evaluate sedentary behavior, such as the sitting question on the IPAQ, may not be sufficient where using a multi-item questionnaire to assess PA may provide more detailed estimates of PA. This is in contrast to what was reported by Milton et al (2011) in that a single-item questionnaire based on one-week recall showed strong reproducibility and a modest concurrent validity with the Global Physical Activity Questionnaire [42]. In addition, one significant aspect where self-reported measures are strong is the ability to provide context to PA, something that instrumented measures are not capable of doing. Instrumented tools explicitly capture body movement while self-report measures can elaborate on the exact type of movement being done.
Each self-reported tool included in this review reported results from both weekdays and weekends, but no studies specifically noted the time of the year in which the study was conducted, which has been shown to have an estimated 15-20% impact on leisure time PA in healthy adults depending on geographic location [43]. In a study involving healthy children from the United Kingdom around the age of 7 years who wore an ActiGraph for continuous monitoring for at least 3 days, 15-30% less moderate to vigorous PA was observed in autumn and winter compared to spring, with an elevated total sedentary time and a greater variation in weekend PA compared to weekday PA as well [44]. Differences between weekday and weekend values are likely attributed to the structure of the school day, while seasonal differences in rainfall have shown to be a factor in seasonal PA differences [44].
One study identified in this review incorporated both an instrumented and self-reported assessment tool, specifically an ActiGraph accelerometer paired with an activity log [20]. Incorporating multiple instrumented or self-reported measures can increase the quality and accuracy of data by providing a method of confirming the results. Including a second tool may prevent significant changes from being overlooked or provide insight into the quality of PA, and the context of PA as mentioned above [45]. For example, a chest-worn device with an accelerometer and inclinometer may not detect a moderate-to-high stationary cycling activity due to a lack of upper body movement, whereas a self-report measure can. Combining the two measures, in this case, may help better characterize PA.
Many of the studies reported in this review have only been completed recently. It has never been easier to measure PA, thanks to a recent boom in public interest in wearable technology. This area of interest is becoming more popular and PA measurement is being included in more studies, but nonetheless does not seem to be a priority in NMD. This is extremely evident in SMA, as only two studies were captured in this review, compared to the larger number of studies investigating PA in DMD. While not identified in studies included in this review, the authors acknowledge the prevalence of consumer-grade wearable devices and their growing inclusion in PA studies. Due to limited validation of these devices, continuing to use research grade activity trackers may be more appropriate given that the devices have not included individuals with altered movement patterns which can impact how a device measures activity counts. To date, only the ActiMyo has been validated to measure digital PA endpoints in SMA or DMD. Validation of instruments in these specific populations using standardized anatomical placement or epoch length has the potential to increase accuracy of the measurements but may not be entirely necessary. Given the rare nature of NMDs like SMA, it may not be feasible to truly validate devices due to small sample sizes, but instead may be worthwhile to evaluate if similar methods can be used for interpreting data across NMDs.
Confirming these methods may make it more acceptable to report values of PA as part of a larger group of NMDs. Including PA measurement in standard SMA and DMD assessments can help determine if someone is achieving the recommended levels of PA, while also providing insight to daily life, function, and quality of life. These results can also be used to help develop an effective exercise and treatment plan going forward, and can be a key variable to measure as drug treatments become widely available.
CONCLUSION
This review identifies PA measurement tools that have been used in ambulatory and non-ambulatory people with SMA and DMD and highlights the variability in data reporting by both instrumented and self-reported tools. Since no gold standard exists for measuring PA in these groups, consideration should be given to the positive and negative aspects of both instrumented and self-reported outcome measures. Instrumented measures offer a consistent and unobtrusive way to measure all ranges of activity or inactivity, as well as bouts of intensity, in both ambulatory and non-ambulatory individuals. After data collection, additional variables can be extrapolated using well known techniques, as well as proprietary novel methods. Self-report tools can yield reasonable, low-cost estimations and provide important information about the individuals’ perception of PA. These tools can be suitable for use regardless of ambulatory status. Self-report tools allow for data collection over longer periods of time without having to remember to wear or charge the device, and can be conducted remotely if needed. Taking all of this information into consideration, we recommend using a combination of instrumented and self-report measures to provide context to the PA measured in these populations. Further research is needed to understand their measurement properties and validate PA tools in SMA and DMD. Studying PA tools across different NMDs will permit comparisons across groups.
FUNDING
This work was supported by the Eunice Kennedy Shriver National Institute of Child Health and Human Development (K01HD084690), and the PNCR infrastructure grant from Cure SMA.
REFERENCES
[1] | McDonald CM Physical activity, health impairments, and disability in neuromuscular disease. Am J Phys Med Rehabil. (2002) ;81: (11 Suppl):S108–20. https://doi.org/10.1097/00002060-200211001-00012 |
[2] | Ryder S , Leadley RM , Armstrong N , Westwood M , de Kock S , Butt T , et al. The burden, epidemiology, costs and treatment for Duchenne muscular dystrophy: an evidence review. Orphanet J Rare Dis. (2017) ;12: (1):79. https://doi.org/10.1186/s13023-017-0631-3 |
[3] | McDonald CM Clinical approach to the diagnostic evaluation of hereditary and acquired neuromuscular diseases. Phys Med Rehabil Clin N Am. (2012) ;23: (3):495–563. https://doi.org/10.1016/j.pmr.2012.06.011 |
[4] | Feldkotter M , Schwarzer V , Wirth R , Wienker TF , Wirth B Quantitative analyses of SMN1 and SMN2 based on real-time lightCycler PCR: fast and highly reliable carrier testing and prediction of severity of spinal muscular atrophy. Am J Hum Genet. (2002) ;70: (2):358–68. https://doi.org/10.1086/338627 |
[5] | Mendell JR , Shilling C , Leslie ND , Flanigan KM , al-Dahhak R , Gastier-Foster J , et al. Evidence-based path to newborn screening for Duchenne muscular dystrophy. Ann Neurol. (2012) ;71: (3):304–13. https://doi.org/10.1002/ana.23528 |
[6] | Verhaart IEC , Robertson A , Wilson IJ , Aartsma-Rus A , Cameron S , Jones CC , et al. Prevalence, incidence and carrier frequency of 5q-linked spinal muscular atrophy – a literature review. Orphanet J Rare Dis. (2017) ;12: (1):124. https://doi.org/10.1186/s13023-017-0671-8 |
[7] | Darras BT Spinal muscular atrophies. Pediatr Clin North Am. (2015) ;62: (3):743–66. https://doi.org/10.1016/j.pcl.2015.03.010 |
[8] | Boland BJ , Silbert PL , Groover RV , Wollan PC , Silverstein MD Skeletal, cardiac, and smooth muscle failure in Duchenne muscular dystrophy. Pediatr Neurol. (1996) ;14: (1):7–12. https://doi.org/10.1016/0887-8994(95)00251-0 |
[9] | Kamil-Rosenberg S , Greaney ML , Hochman T , Garber CE How Do Physical Activity and Health Vary Among Younger, Middle-Aged, and Older Adults With and Without Disability? J Aging Phys Act. (2019) ;27: (2):234–41. https://doi.org/10.1123/japa.2017-0215 |
[10] | McDonald CM , Widman LM , Walsh DD , Walsh SA , Abresch RT Use of step activity monitoring for continuous physical activity assessment in boys with Duchenne muscular dystrophy. Archives of Physical Medicine and Rehabilitation. (2005) ;86: (4):802–8. https://doi.org/10.1016/j.apmr.2004.10.012 |
[11] | Carty C , van der Ploeg HP , Biddle SJH , Bull F , Willumsen J , Lee L , et al. The First Global Physical Activity and Sedentary Behavior Guidelines for People Living With Disability. J Phys Act Health. (2021) ;18: (1):86–93. https://doi.org/10.1123/jpah.2020-0629 |
[12] | Kohl HW , 3rd , Craig CL , Lambert EV , Inoue S , Alkandari JR , Leetongin G , et al. The pandemic of physical inactivity: global action for public health. Lancet. (2012) ;380: (9838):294–305. https://doi.org/10.1016/S0140-6736(12)60898-8 |
[13] | Bull FC , Al-Ansari SS , Biddle S , Borodulin K , Buman MP , Cardon G , et al. World Health Organization guidelines on physical activity and sedentary behaviour. Br J Sports Med. (2020) ;54: (24):1451–62. https://doi.org/10.1136/bjsports-2020-102955 |
[14] | Martin Ginis KA , van der Ploeg HP , Foster C , Lai B , McBride CB , Ng K , et al. Participation of people living with disabilities in physical activity: a global perspective. The Lancet. (2021) ;398: (10298):443–55. https://doi.org/10.1016/S0140-6736(21)01164-8 |
[15] | Hills AP , Mokhtar N , Byrne NM Assessment of physical activity and energy expenditure: an overview of objective measures. Front Nutr. (2014) ;1: :5. https://doi.org/10.3389/fnut.2014.00005 |
[16] | Uher D , Yoon L , Montes, J , Physical activity levels achieved by children and adults with SMA and DMD. PROSPERO. 2020 CRD4202021807. (2020) . |
[17] | Higgins JPT TJ , Chandler J , Cumpston M , Li T , Page MJ , Welch VA (editors). Cochrane Handbook for Systematic Reviews of Interventions version 6.3. Cochrane, 2021. (2021) . |
[18] | Jeannet PY , Aminian K , Bloetzer C , Najafi B , Paraschiv-Ionescu A Continuous monitoring and quantification of multiple parameters of daily physical activity in ambulatory Duchenne muscular dystrophy patients. Eur J Paediatr Neurol. (2011) ;15: (1):40–7. https://doi.org/10.1016/j.ejpn.2010.07.002 |
[19] | Kimura S , Ozasa S , Nomura K , Yoshioka K , Endo F Estimation of muscle strength from actigraph data in Duchenne muscular dystrophy. Pediatr Int. (2014) ;56: (5):748–52. https://doi.org/10.1111/ped.12348 |
[20] | Montes J , Garber CE , Kramer SS , Montgomery MJ , Dunaway S , Kamil-Rosenberg S , et al. A Randomized, Controlled Clinical Trial of Exercise in Patients with Spinal Muscular Atrophy: Methods and Baseline Characteristics. J Neuromuscul Dis. (2014) ;1: (2):151–61. |
[21] | Davidson ZE , Ryan MM , Kornberg AJ , Walker KZ , Truby H Strong Correlation Between the 6-Minute Walk Test and Accelerometry Functional Outcomes in Boys With Duchenne Muscular Dystrophy. Journal of Child Neurology. (2015) ;30: (3):357–63. https://doi.org/10.1177/0883073814530502 |
[22] | Elliott SA , Davidson ZE , Davies PS , Truby H Accuracy of Parent-Reported Energy Intake and Physical Activity Levels in Boys With Duchenne Muscular Dystrophy. Nutr Clin Pract. (2015) ;30: (2):297–304. https://doi.org/10.1177/0884533614546696 |
[23] | de Valle KL , Davidson ZE , Kennedy RA , Ryan MM , Carroll KM Physical activity and the use of standard and complementary therapies in Duchenne and Becker muscular dystrophies. J Pediatr Rehabil Med. (2016) ;9: (1):55–63. https://doi.org/10.3233/PRM-160364 |
[24] | Heutinck L , van Kampen N , Jansen M , de Groot IJM Physical Activity in Boys With Duchenne Muscular Dystrophy Is Lower and Less Demanding Compared to Healthy Boys. Journal of Child Neurology. (2017) ;32: (5):450–7. https://doi.org/10.1177/0883073816685506 |
[25] | Chabanon A , Seferian AM , Daron A , Pereon Y , Cances C , Vuillerot C , et al. Prospective and longitudinal natural history study of patients with Type 2 and 3 spinal muscular atrophy: Baseline data NatHis-SMA study. PLoS One. (2018) ;13: (7):e0201004. https://doi.org/10.1371/journal.pone.0201004 |
[26] | Fowler EG , Staudt LA , Heberer KR , Sienko SE , Buckon CE , Bagley AM , et al. Longitudinal community walking activity in Duchenne musculardystrophy. Muscle Nerve. (2018) ;57: (3):401–6. https://doi.org/10.1002/mus.25743 |
[27] | Jacques MF , Onambele-Pearson GL , Reeves ND , Stebbings GK , Smith J , Morse CI Relationships between muscle size, strength, and physical activity in adults with muscular dystrophy. Journal of Cachexia Sarcopenia and Muscle. (2018) ;9: (6):1042–52. https://doi.org/10.1002/jcsm.12347 |
[28] | Fujii T , Takeshita E , Iwata Y , Yajima H , Nozaki F , Mori M , et al. Cumulative jerk as an outcome measure in nonambulatory Duchenne muscular dystrophy. Brain & Development. (2019) ;41: (9):796–802. https://doi.org/10.1016/j.braindev.2019.06.002 |
[29] | Arteaga D , Donnelly T , Crum K , Markham L , Killian M , Burnette WB , et al. Assessing Physical Activity Using Accelerometers in Youth with Duchenne Muscular Dystrophy. Journal of Neuromuscular Diseases. (2020) ;7: (3):331–42. https://doi.org/10.3233/Jnd-200478 |
[30] | Killian M , Buchowski MS , Donnelly T , Burnette WB , Markham LW , Slaughter JC , et al. Beyond ambulation: Measuring physical activity in youth with Duchenne muscular dystrophy. Neuromuscul Disord. (2020) ;30: (4):277–82. https://doi.org/10.1016/j.nmd.2020.02.007 |
[31] | Siegel BI , Cakmak A , Reinertsen E , Benoit M , Figueroa J , Clifford GD , et al. Use of a wearable device to assess sleep and motor function in Duchenne muscular dystrophy. Muscle & Nerve. (2020) ;61: (2):198–204. https://doi.org/10.1002/mus.26759 |
[32] | van der Geest A , Essers JMN , Bergsma A , Jansen M , de Groot IJM Monitoring daily physical activity of upper extremity in young and adolescent boys with Duchenne muscular dystrophy: A pilot study. Muscle Nerve. (2020) ;61: (3):293–300. https://doi.org/10.1002/mus.26763 |
[33] | Jacques MF , Onambele-Pearson GL , Edwards B , De Goede CG , Morse CI Quantitative assessment of sitting time in ambulant adults with Muscular Dystrophy. PLoS One. (2021) ;16: (11):e491. https://doi.org/10.1371/journal.pone.0260491 |
[34] | Lott DJ , Taivassalo T , Senesac CR , Willcocks RJ , Harrington AM , Zilke K , et al. Walking activity in a large cohort of boys with Duchenne muscular dystrophy. Muscle Nerve. (2021) ;63: (2):192–8. https://doi.org/10.1002/mus.27119 |
[35] | McErlane F , Davies EH , Ollivier C , Mayhew A , Anyanwu O , Harbottle V , et al. Wearable Technologies for Children with Chronic Illnesses: An Exploratory Approach. Ther Innov Regul Sci. (2021) ;55: (4):799–806. https://doi.org/10.1007/s43441-021-00278-9 |
[36] | Ainsworth BE , Haskell WL , Herrmann SD , Meckes N , Bassett DR, Jr , Tudor-Locke C , et al, Compendium of Physical Activities: a second update of codes and MET values. Med Sci Sports Exerc. (2011) ;43: (8):1575–81. https://doi.org/10.1249/MSS.0b013e31821ece12 |
[37] | Osu R , Ota K , Fujiwara T , Otaka Y , Kawato M , Liu MG Quantifying the quality of hand movement in stroke patients through three-dimensional curvature. Journal of Neuroengineering and Rehabilitation. (2011) ;8. https://doi.org/10.1186/1743-0003-8-62 |
[38] | Cleland I , Kikhia B , Nugent C , Boytsov A , Hallberg J , Synnes K , et al. Optimal placement of accelerometers for the detection of everyday activities. Sensors (Basel). (2013) ;13: (7):9183–200. https://doi.org/10.3390/s130709183 |
[39] | Leeger-Aschmann CS , Schmutz EA , Zysset AE , Kakebeeke TH , Messerli-Burgy N , Stulb K , et al. Accelerometer-derived physical activity estimation in preschoolers – comparison of cut-point sets incorporating the vector magnitude vs the vertical axis. BMC Public Health. (2019) ;19: (1):513. https://doi.org/10.1186/s12889-019-6837-7 |
[40] | Dowd KP , Szeklicki R , Minetto MA , Murphy MH , Polito A , Ghigo E , et al. A systematic literature review of reviews on techniques for physical activity measurement in adults: a DEDIPAC study. Int J Behav Nutr Phys Act. (2018) ;15: (1):15. https://doi.org/10.1186/s12966-017-0636-2 |
[41] | Prince SA , Cardilli L , Reed JL , Saunders TJ , Kite C , Douillette K , et al. A comparison of self-reported and device measured sedentary behaviour in adults: a systematic review and meta-analysis. Int J Behav Nutr Phys Act. (2020) ;17: (1):31. https://doi.org/10.1186/s12966-020-00938-3 |
[42] | Milton, K , Bull, FC , Bauman, A , Reliability and validity testing of a single-item physical activity measure. Br J Sports Med. (2011) ;45: (3):203–208. https://doi.org/10.1136/bjsm.2009.068395 |
[43] | Pivarnik JM , Reeves MJ , Rafferty AP Seasonal variation in adult leisure-time physical activity. Med Sci Sports Exerc. (2003) ;35: (6):1004–8. https://doi.org/10.1249/01.MSS.0000069747.55950.B1 |
[44] | Atkin AJ , Sharp SJ , Harrison F , Brage S , Van Sluijs EM Seasonal Variation in Children’s Physical Activity and Sedentary Time. Med Sci Sports Exerc. (2016) ;48: (3):449–56. https://doi.org/10.1249/MSS.0000000000000786 |
[45] | Jimenez-Moreno AC , Newman J , Charman SJ , Catt M , Trenell MI , Gorman GS , et al. Measuring Habitual Physical Activity in Neuromuscular Disorders: A Systematic Review. J Neuromuscul Dis. (2017) ;4: (1):25–52. https://doi.org/10.3233/JND-160195 |