A trust evaluation model for secure data aggregation in smart grids infrastructures for smart cities
Abstract
New technologies and automation systems have changed the traditional smart grid systems into new and integrated intelligent systems. These new smart systems are adopted for energy efficiency, demand and response, management and control, fault recovery, reliability and quality of services. With various benefits, smart grids have vulnerabilities due to open communication systems, and open infrastructures. Smart grids systems are based on real-time services, where privacy and security id one of the major challenge. In order to address these challenges and deal with security and privacy issues, we proposed a Trust Evaluation Model for Smart Grids (TEMSG) for secure data aggregation in smart grids and smart cities. This model tackles privacy and security issues such as data theft, denial of services, data privacy and inside and outside attacks and malware attacks. Machine learning methods are used to gather trust values and then estimate the imprecise information to secure the data aggregation in smart grids. Experiments are conducted to evaluate and analyze the proposed model in terms of detection rate, trustworthiness, and accuracy.
1.Introduction
With the rising population and the largest wave of urbanization leads to massive and complex cities all over the world. It is estimated that by the end of 2030, the urban area population all over the world reaches to around 5 billion [25]. This number alarms the shortage of economic and development resources. Furthermore, the massive urbanization also leads to an excessive burden on energy resources, infrastructure, and other living resources. Traditional power grids are undoubtedly outdated and do not meet the current and future electric demand for the stable electric supply [23,29]. Traditional grids have been suffered from large data latency, one-way supply management, weak manual controlling and tracking systems. In some places, the traditional grids have adopted few intelligent systems to handle and tackle the current user’s demands.
Smart and integrated technologies have changed the traditional power systems and offer new and advanced smart meters, smart distribution, log servers, power generation plants, and other networks. New technologies have improved the traditional power systems and changed into more cost-effective systems by using new techniques, hardware plus software solutions [16,32]. Smart grids are based on intelligent electrical grids and other components to control and manage the systems. Grid systems are complex due to their huge infrastructures, networks, controlling plants, monitoring and tracking systems. Smart grid systems provide new solutions for energy management, consumer management, load sharing techniques and data handling to fulfill the user’s demands. In these systems, the user data and information disseminated through a number of devices and components including electric generation systems, distribution, transmission, and electric consumption. Smart grids also offer uninterrupted power supply, integrated energy resources, bidirectional processes, fault management, tracking capabilities and self-based decision management.
There are three main challenges faced by smart grids including reliability, delivery, privacy and security. Real-time monitoring and controlling systems should be reliable and able to deliver the services by using sustainable management and control systems. The privacy in smart grid refers to consumer rights to control their personal information. On the other hand, security refers to all user’s data security which should be secure [19,31]. Security also is one of the significant system requirements during an exchange of services among main and subsystems [1,18]. Smart grid systems have five main components including hardware, software, servers and data where time by time these components have been updated or replaced. Smart grids systems should be able to defend their information from unauthorized access, disruption, inspection, modification, and disclosure. All data and communication processes should be integrated, confidential, non-repudiation from attackers and outside world [4,33]. Although, various models and frameworks have been proposed to secure the smart grid communication process still these networks are suffered from security and privacy issues. The data has confidential information related to user’s real-time data, operation status, utilities information and electricity usage private data. In order to address these security and privacy concerns, this paper presents a trust evaluation model for smart grids. Other objectives of this paper are as follows:
Discuss smart grid systems, architecture and applications in detail.
Review all possible attacks in smart grids and review the existing solution.
Proposed a trust evaluation model for smart grid systems.
Evaluate the proposed model with existing models.
The rest of the paper is organized as follows: Section 2 presents the complete detail about smart grid operation and services, its architecture and applications. Section 3 presents the security and privacy fundamentals and possible attacks. Section 4 presents the related work for smart grids. Section 5 presents the proposed trust in evaluating a model for smart grid systems. Section 6 presents the experimental results to evaluate the proposed model with existing models. The last section concludes the paper with a future direction.
2.Smart grid systems
Smart grids have changed the traditional electric management services and offered innovative systems. These new integrated systems provide more convenience to fulfill the energy demand by controlling the utility expenses. Smart systems are based on new communication technologies where user share their data related to energy usage, utilities, and energy supplies by using cellular and other data communication networks. In smart cities, the energy distribution is always on top priority and need more advance metering infrastructure from power generation to consumers [9,40]. Smart meters provide real-time monitoring systems, price management, power distribution and controlling power and supply management. Smart cities projects have started in various countries for remote management and improve the energy efficiency and generation. The interconnection of different systems makes these networks more vulnerable to different internal and external attacks [27]. There are various new and advanced features of smart grids when compared with traditional grids. The main enormous differences between traditional grids and smart grids are generation methods, monitoring systems, metering, controlling, power flow management, restoration and grid architecture. The conventional grid has central generation methods whereas the smart grid is based on decentralized generation methods. Furthermore, conventional grids have manual monitoring where the control method is limited and passive [36]. On the other hand, smart grids have active control methods and based on the self-monitoring mechanism. The power flow is one way in which conventional grids and restoration systems are manual and local based. Whereas the smart grid has a self-restoration mechanism and two-way power flow management.
2.1.Smart grid architecture
Smart grids refer to infrastructure for delivering electricity by using three phases including electricity generation, transmission, and distribution. In the generation phase, electricity is generated by using huge centralized power stations operated with different natural resources such as coal, gas and power, nuclear and hydro systems. Generation systems are based on modern hydrogenated and combine power and heat systems [7]. After electricity generation, the next step is electricity transmission where electric supply transported from stations to substations and the end-users. In the distribution phase, the electricity is distributed among industrial and residential zones.
The traditional grid system has suffered from voltage instability, curtailments, heavy load, and intermittency issues. The new smart grids systems control the demand and supply management, monitoring and generation management. Smart grid infrastructure and architecture provide secure, reliable data communication [15]. The Energy Independent Act of the USA in 2007, defined the smart grids policies including modernization of electric grid, smart grid systems, research and development of smart grids, advisory committee, interoperability framework, and security elements.
The smart grid architecture has various features including digital systems to improve the grids security, supply management, dynamic optimization, renewable resources, demand-side resources, real-time communication, smart metering, distribution automation, and peak shaving technologies. Figure 1 shows the smart grid architecture and its different modules.
Fig. 1.
Smart grid architecture and its modules.
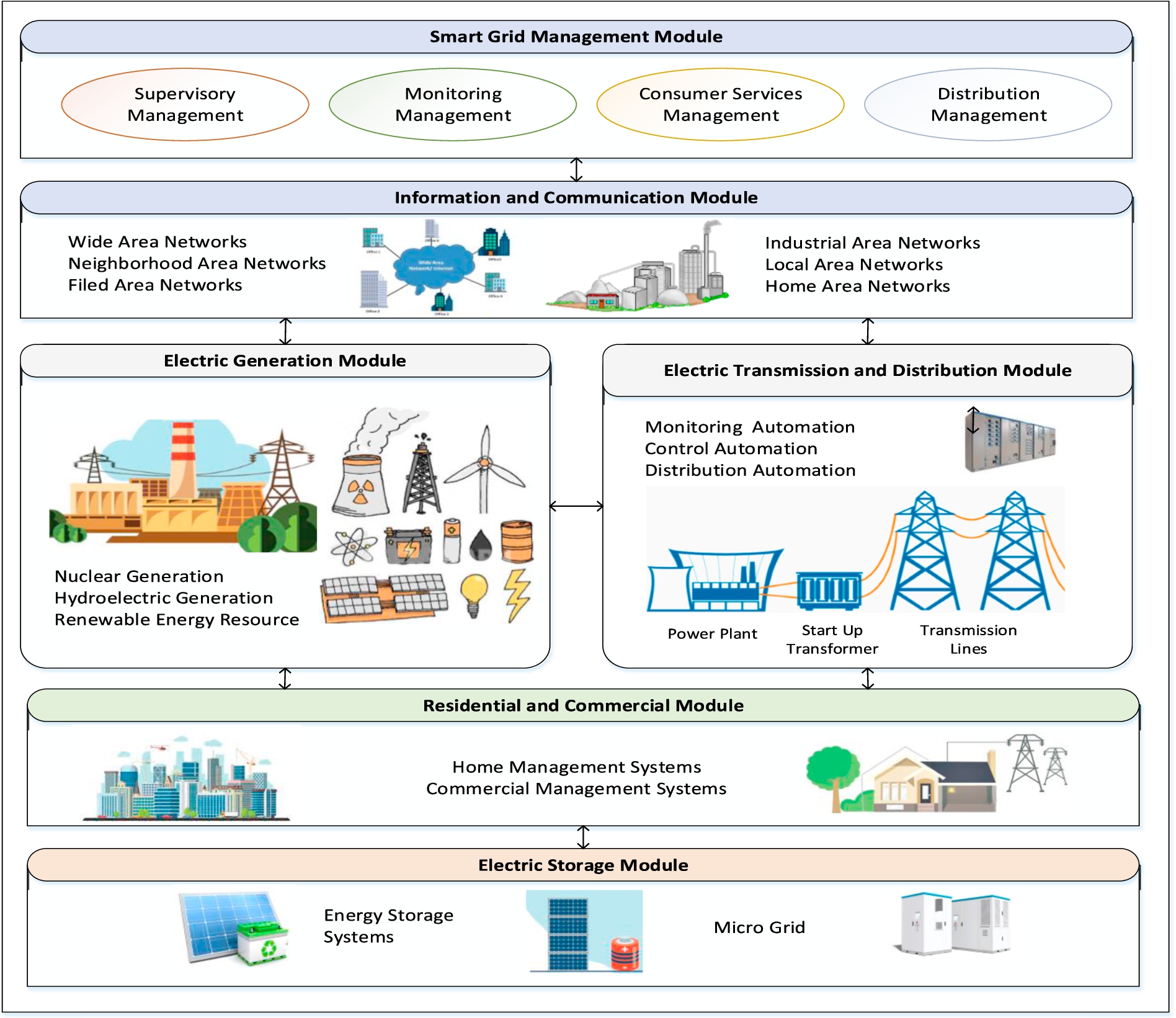
2.2.Smart grid applications
Smart grid is an attractive domain based on the different processes including electric generation, transmission and distribution [22]. These all processes are working with more advance and integrated communication standards, and wireless technologies.
3.Security and privacy in smart grids
Smart grids are using advance sensing and control methods for data communication related to power generation, usage, delivery in a real-time environment. All the data in smart grids is related to corresponding, information provision and recommendation with stakeholders. Smart grids are based on systems of systems where electricity and information technologies and all operational and governess is involved [43]. These complex systems lead to various security challenges in terms of privacy and cybersecurity aspects. Smart grids are a potential target of malicious adversaries [10]. The smart grids are subject to attackers where they inject any malicious activity to gain their resources or control. Due to heavy electric based systems, any sort of malicious activity is harmful and has a serious impact on urban life. Threats are also in the form of any manipulation in national defense electric resources and initiate the attacks on defense systems. Radar system jamming is another serious threat for defense where attackers stop the system for any airstrike. In addition, the personal information of users invade is another type of privacy attacks [6,21]. Different types of strategies have been adopted to handle these security attacks such as Virtual Private Networks (VPN) and Public Key Infrastructure (PKI), Intrusion Detection Systems (IDS), Anti-Virus tools, firewalls and secure IT infrastructure [2]. However, these existing systems are not effective because of their differences and complex strategies.
The applications are divided based on above discussed modules and shows in Table 1.
Table 1
Details of smart grids applications module wise
S# | Applications | Module | Used communication technologies | Hardware | Data rate |
1 | Smart metering | Residential and commercial | DSL, ethernet, wired or wireless | Smart meters, mobile devices, display unit | Up to 100 Bytes |
2 | Prepayment app | Residential and commercial | DSL, ethernet, wired or wireless | Mobile devices, computer system | 50 to 150 Bytes |
3 | Customer messaging and information | Residential and commercial | Wireless | Smart phones | 50 to 200 Bytes |
4 | Service switch operations | Residential and commercial | Wired | Switching device, system | Up to 25 Bytes |
5 | Distribution automation | Electric transmission and distribution | Wireless, cellular networks | Field devices | 150 to 250 Bytes |
6 | Wide area transmission control app | Electric generation module | Wireless, WiMAX, cellular | Access points, base stations, infrastructure | 4 to 157 Bytes |
7 | Wide area power monitoring | Electric generation module | Wireless, WiMAX, cellular | Access points, base stations, infrastructure | 4 to 55 Bytes |
9 | Home automation | Residential | Wired and wireless | Fixed and mobile devices | 10 to 100 Bytes |
10 | Service switch operation | Electric generation module | Wired | Access points, base stations, infrastructure | 1 to 25 Bytes |
11 | Demand response | Residential and commercial | Wired | Switching device, system | 100 Bytes |
3.1.Possible attacks in smart grids
There are various attacks have been noticed in smart grid systems, where the attackers disturbed the normal electric flow [14]. Table 2 shows the possible attacks in smart grids.
Table 2
Security and privacy attacks in smart grids
S# | Attack type | Description |
1 | Denial of service attack | In these attacks, the attackers seek to make the network resources unavailable the services and disturbing the normal flow of electric supply or information. |
2 | On–off attack | In these attacks, the devices behavior are compromised and they provide incorrect information to misguide users with malicious intent to disperse or create the blockade over the smart grid networks. |
3 | Bad mouthing attack | In these attackers, the attackers collude negative feedback and cause of deteriorate the smart grid system performance. |
4 | Data attack | In these attackers, the attackers manipulate, alter or delete the data and mislead the smart grids for wrong decisions. |
5 | Physical attack | In these attackers, the attackers compromise the smart grid devices and also the first step of any sophisticated attack. |
6 | Network attack | In this attacks, the attackers learn or infer the users private information like electricity usage. |
4.Related work
Since maximum cyber-security vulnerabilities in clever grids are associated with the protection of records, it’s miles essential to cozy data, now not only in transit (when it’s miles injected in a community) but also at relaxation, the statistics stored and processed. The survey using [34] makes a specialty of a taxonomy of the life cycle of smart grid records, which may be decomposed into four sequential levels: the era of statistics, acquisition, storage, and processing. Specific safety traits are summarized for each stage. In particular, generation and acquisition encompass one of a kind sources of facts along with PMUs and clever meters, and the acquisition manner performed by means of the communique infrastructure. Vulnerabilities in these levels overlap with other surveys that focus on the distinctive conversation protocols, and the troubles on sharing sensitive information. However, one specific characteristic of this survey is the analysis of garage facts and processing statistics. Data storage within the clever grid is an essential aspect used for a huge quantity of functionalities, which include failure detection, demand reaction, forecasting, and billing. The authors also address distinctive defense mechanisms that they know as data analytics. They argue that a large number of statistics may be processed to identify patterns, predict, and pre-scribe answers, the use of statistical analysis, information mining, and information visualization.
Fadul, et al. [8] proposed a robust and configurable trust-management system to protect and secure smart grids from cyber-attacks. Trust-management toolkit assigns low trust value to indicate a higher level of risk and vice versa. It is a baseline to protect the smart grid system. This toolkit integrates reputation-based trust with network-flow algorithms to discover and patch the vulnerable devices. All devices are assigned with different values by the trust management toolkit. However, the faulty devices are assigned lower values which indicate a prior warning that this device has a high probability of failure. The researchers have proven after running simulations of the proposed toolkit that this approach is useful to protect smart-grid systems.
In [13], the authors proposed Trust System Placement (TSP) scheme for smart grid Supervisory Control and Data Acquisition (SCADA) networks. This trust system comes with a NIDS along with the firewalling. These features enable this system to monitor external and internal traffic. This research also aims to reduce the Capital Expenditure (CAPEX) & Operational Expenditure (OPEX) but this system is only integrated with selective devices and as per the name, these devices are known as Trust Devices. This paper taken an analytical approach and analyzed the problems in trust systems and stated that these systems may create problems in the network hierarchy of the smart grid. Hence, an approach with the least possible trust devices has been preferred by segmenting the network to scatter the trust devices. To observe the scattering of the devices, the Minimum spanning tree method has been used. Size balancing and Geographic dispersion of the trust devices are identified as the major problems in the segment-based network approach. The size balancing is responsible for the required trust devices and the geographic dispersion problem deals with the delay (response time). The authors have developed a scheme that combines Linear Programming Problem (LPP) and local search. The experiments and simulations are tested using numerical analysis.
Authors in [24], presented a Real Alert technique which is a secure and trustworthy sensing scheme based on the policy. This technique assesses the data trustworthiness and IoT node attributes using the inconsistent IoT and related networks data packets and contextual information which depicts the network environment whose anomalous devices acquires the data packets. The trustworthiness of different devices in alternate scenarios are evaluated on the basis of the policy based rules. Arrival of any new devices with distinct attributes or bearing different characters or any different joining is normally considered as an attack by any malicious or selfish user who is following an outdated policy. This novel technique [30] is based on the entropy based method used for dynamic optimization which effectively employs global trust to encounter collusion based attacks and in it network is divided into logic based groups. Entropy function based on weights and reputation’s standard deviation calculates the node’s local trust and updates instantly. Despite efficient in detecting malicious node still unveils limitations like greater energy consumption and restrained to counter several internal attacks.
Aref and Tran [5] described the relationship between multi-agents and the Internet of Things (IoT) in the field of Internet of Agents (IoA) that shows the autonomous and intelligent system. These multi-system agents are used to model distributed systems for different sectors of smart grids. An important factor is the “trust relationship” between two parties that are required for successful interaction. These interactions required a trust-based mechanism between multi-agents for better results in the field of Trust relationship. The author proposed the Internet of Agent (IoA) trust model based upon multi-criteria to improve the trustworthiness and interaction between trustors and trustees. Criteria are improved as per single interaction which leading to form multi-criteria and model predicted both appropriate value and its importance. The proposed model uses the provided feedback from trustors regarding how satisfied they were with recent transactions to predict the importance of different service dimensions for trustors and adjust the behavior of trustee(s) accordingly. Trustees aim to enhance their trustworthiness scores with the hope to be selected for future interactions. Simulation results indicate that, in a competitive environment, trustees can improve their portion of transactions if they use MCTE to adjust their proposed UG.
In [3], the authors proposed a model to secure routing in smart grid networks called A Fuzzy Logic Trust Model for Secure Routing in Smart Grids. This research aims to provide a solution that can protect smart grids from cybersecurity attacks. The well-known trust method is being used to protect the whole system against cyber-attacks. The fuzzy logic trust model detects untrusted devices and compares with the existing model to improve efficiency and detection rate. It is observed in the simulation results that this approach increases the routing efficiency and threat finding capabilities. In the results, this proposed method is compared with the existing and Dependable Trust System (LDTS) model. However, it is proven by the results that a new and improved fuzzy logic model has shown a 90% improvement in terms of efficiency when the infected devices are less than 25%.
Authors in [11] offered a remote attestation technique basing upon trust mechanism based cloud to certify the credibility of the network and enables credible access control among the devices. This technique enables trusted communication among terminal and controlling server node, ensures the node credibility during data transmission over the network in a secure network environment. It passes evaluator node integrity status to the verifying node. After receiving status, the authenticating node verifies and judges it to testify about trust worthiness of the sender and whether the corresponding nodes fulfils the communication requirements
Liu, et al. [26] described real-time electricity consumption and there is a need to protect single-user data. Data aggregation is a solution for privacy-preservation but most of the aggregation schemes are depending upon Trusted Third Party (TTP). This approach is having a negative impact on reliability because the system can easily hack by using Denial of Service (DoS) attacks. This research proposed a data aggregation scheme, named 3PDA, that uses a virtual aggregation area to mask the metering data of individual users. Although 3PDA does not rely on a trusted party to aggregate the data, its system model is simplified (it has only a service provider, aggregator, and SMs), thus not practical to deploy in an existing grid architecture. Victual aggregation area is used to make 3PDA more practicable. Communication overhead and computational cost are also reduced, and performance evaluation describes the proposed scheme is robust and efficient.
Authors in [28] calculated the trust among the nodes in distributive manner. It adds recommendations to subsequent trust model in which the investigating nodes send their trust table to all nodes in the network periodically. Trust values received from neighbours having less trust score and holding contradictory values to trust value of investigating node are discarded. An investigator node updates the trust score of the nodes in IoT or alike environment by aggregating previous trust score and adding it with the filtered trust weights by comparing to direct trust value of evaluator node. This technique is useful for selecting a confident guarantor node which leads to moderate trust performance if a malicious node is mistakenly for data transmission. It uses the weight recommendations for trust evaluation to cater for the malicious and honest nodes determination basing upon the neighbouring trust score at different trust levels. It employs mechanism to seek dissimilarity between recommendations while matching the investigating node recommendation which may be vulnerable to colluded bad mouthing attacks.
Authors in [42], presented a hierarchical network phenomenon AF-TNS which utilized various link communication technologies like the dual & single based link technology with diverse characteristics to assume reliability of a node in identification of a malevolent nodes. It is based on assumption that Dual Link nodes are secure, more reliable and trustworthy. It also emphasizes to calculate the trust for identifying the malicious nodes basing upon the weighted trust in which each sensor keeps the record of readings from all the nodes in the network in neighborhood and then each sensor node sends this record to the cluster for data aggregation. Then trustworthiness of the equivalent readings calculated as weights are selected and prioritized on the basis of majority voting method to vastly detect the malevolent or selfish nodes.
Authors in [35] proposed a block chain solution by using trust method to detect the malicious nodes in sensor node networks. It is a trusted framework conceptualized over block chain data structure to detect malicious nodes. This technique recognizes the malicious node using sensor quadrilateral and block chain contracts. This technique effectively warrants the tractability of malicious node detection process. This technique is suitable for oil and gas industry whose strategy is to achieve efficiency at strategic and management levels while shifting to digitalization and intelligence.
In [39], the authors presented a multi-agent-based System Integrity Protection (SIP) solution to provide resilience against cyber-attacks. A decentralized SIP set-up is installed for anomaly detection and adaptive load rejection. Support Vector Machine embedded Layered Decision Tree (SVMLDT) provided a centralized solution after an individual’s agent carried out anomaly detection. The researchers have implemented this method by using situational awareness and a self-adaptive approach. This method generally focused on data-driven anomaly detection and adaptive load rejection. However, the whole idea is implemented after deeply studying the working of cybersecurity on hardware in power grid systems. The proposed method protects the smart grid systems against the Denial of Service (DoS) attacks. The simulation results prove that this proposed solution has great potential to identify odd grid operations states and then patch them as well.
4.1.Discussion and findings
All networks in smart grids and smart cities are responsible to deliver the information, data and provide data communication among nodes. To establish the secure data communication and isolate the disturbance and data drop, identification of honest and malicious/selfish nodes is still a big challenge in these networks. Most of nodes in these systems are un-attended without human interaction which are prone to suffered due to malicious attacks [12]. Security is one of the main concerns and still a problem and difficult to predict the integrity of data. The existing solution including cryptography and authentication mechanism are able to provide certain level of security. These solutions are not able to find the malicious nodes within the network due to computation complexities. The malicious nodes in smart cities data communication degrade the data delivery performance and consider a real threat. These existing security solutions are not able to tackle internal attacks. The security-based evaluation approach needs to provide reliability to handle malicious nodes and prevent smart cities data communication from malicious nodes. If any malicious node exists in network, it may offer the other internal attacks to make network vulnerability.
The security based approach is needed to enhance the degree of confidence of deployed nodes in smart cities based on their past interactions, directly or indirectly to record the all information for decision making.
Most of the existing research is based on modelling the security frameworks without takes periodic re-evaluation of nodes. Malicious nodes are periodically changing its behavior where they drop fewer number of data packets and sometime behave more data delivery ratio in the network. In order to address these issues in smart cities networks, there is a need to design more secure approach to handle malicious nodes in the network.
Smart city and related IoT based devices are open to malicious attacks, as they are deployed in the open environment thus a malicious node can be inserted in the network or any node can be compromised from the internal attacker. Table 3 shows the comparison of existing schemes.
Table 3
Technical analysis of exiting schemes
S# | Trust models | Trust technique | Trust evidence collected | Attacks defended | Malicious node detected | Validation technique | Domain | Trust estimation method | Trust calculation metrics | |||||
Distributed | Centralized | Cross layer | Direct trust | Indirect trust | Reputation | Watchdog | ||||||||
1 | CTMS [8]/2013 | Network-flow algorithms | Segments | Search patch in the vulnerable devices | No | Simulation | Smart grids | Yes | No | Yes | Yes | No | No | No |
2 | TSP [13]/2016 | Spanning tree method | Segments | Different security attacks | No | Simulation | Smart grids | Yes | No | Yes | Yes | Yes | No | No |
3 | Real Alert [24]/ 2017 | Policy based | Policy management node | Bad-mouthing, on–off | Yes | Experimental | IoT | Yes | Yes | No | Yes | No | No | No |
4 | Trust-Doe Nie [30]/ 2017 | Entropy based | Packets | Collusion | Yes | Experiment | WSN | Yes | No | No | Yes | Yes | No | No |
5 | IoA [5]/2017 | Multi-system agents | Packets | Different security attacks | No | Simulation | IoT | Yes | No | Yes | Yes | Yes | No | No |
6 | FLTM [3]/2017 | Fuzzy logic | Packets | Different security attacks | No | Simulation | Smart grids | Yes | No | Yes | Yes | No | No | No |
7 | Remote Attestation [11] 2018 | Identity and authentication | Packets | Replay, on key | No | Case study | Cloud | Yes | Yes | No | No | No | No | No |
8 | TTP [26]/2018 | Data aggregation | Packets | Denial of Service (DoS) attacks | No | Simulation | Yes | No | Yes | Yes | No | No | No | |
9 | TMSC [28]2018 | Theory based on cloud and weighing scores | Adjacent nodes service requests | Bad and good mouth, DoS, selected forwarding, on–off | Yes | Simulation | IoT and cloud | Yes | No | Yes | Yes | Yes | No | No |
10 | AF-TNS [42]/2019 | Activation function | Packets | None | Yes | Case study | WSN | Yes | No | No | Yes | No | No | No |
11 | Block chain [35] 2019 | Block chain | Segments | None | No | Case study | IoT | Yes | No | Yes | Yes | No | Yes | Yes |
12 | SIP [39]/2020 | Machine learning | Packets | Anomaly detection, Denial of Service (DoS) attacks | No | Simulation | Smart grids | Yes | No | Yes | Yes | No | No | No |
Now question arises how to identify these nodes. As IoT devices have very low energy and computational power so complex algorithms will just create an overhead and will drain the energy. Added with, as the smart city is composed of huge volume of smart devices and various types of networks, the energy of sensors along with battery utilization of different devices is more and also is eligible for drainage of network resources in terms of storage and processing. The most efficient way of finding the malicious node is to find the trustworthiness of the node. There are two types of trust direct and indirect trust. These two types of trust are further extended to achieve the cumulative trust to testify the sincerity, credibility and efficacy of the node during calculating the best suitable path for the communication. Both type of trust helps to diagnose the selfish and malicious nodes and helps the network administrators to technically handle, deprive and eliminate the malevolent nodes from the network and invariably inform all the nodes in the network to remove such nodes from their path list.
5.Proposed trust evaluation model for smart grids (TEMSG)
The proposed TEMSG model is designed to evaluate the trust in smart grid systems. This model is elucidating its supportive method to handle the detection, separation of malicious devices and monitor the networks from external attacks such as on-off, bad mouthing and DoD attacks. The proposed model calculates the cumulative trust by using direct and indirect interaction to search the trustworthy path of devices to transmit the data. The proposed model is divided into two main modules including a trust evaluation module and initializing module. In the first evaluation module, the identifies the internal attacks and malicious devices in the networks. The second module evaluates the direct and indirect trust of devices based on data drop rate and time analysis. We handle three types of attacks including On and Off Attack, bad-mouthing attack and DoS attacks. In DoS Attack, the bulk data is forwarded by the malicious or the selfish device and creates a burden on the network resources. This attack is managed by keeping the track of the data rate of the packets sent and received [38]. In bad-mouthing attack, the device recommends incorrect values about the neighboring devices involved in the network. This attack is handled by keeping the track of packet dropping and delaying rate, end-to-end delay, trustworthiness level by acquiring indirect trust via the direct trust of the neighboring devices and calculated over the communication parameters. Direct trust values of different devices are considered with the targeted devices to evaluate the true network picture and transactional history/record of device behavior instead of recommendation based trust [20]. In On–Off Attack, the Device’s behaviors are compromised and by using direct trust values abnormally incorrectly to misguide the communicating devices with malicious intent to disperse or create the blockade over the network. The selfish or malicious devices behave unpredictably like some time behave good and sometimes bad, over the communication network. This type of attack is handled by using network lifetime span, trustworthiness level, packet delay parameters based on the time, drop rate and the data rate parameters [37].
To evaluate the proposed model, we design an attack scenario where we transmit and forward the data to the concerning devices and also at times generate the data flood instructions with garbage messages or retransmission of the same messages to targeted devices for creating the network link failure. In an attack scenario, we flood false traffic over the network and create incorrect trust values for the adjacent devices with malafide intentions. Trust models are conceived and developed to enhance and upgrade the security of the application [17]. The device’s trust values and data items along the evaluation model are under a constant threat from attackers by adopting the three attack types [41]. Figure 2 shows the flow diagram of each module in the TEMSG model.
In flow chart, the TEMSG first check the available paths and evaluate the all paths. In evaluation process, the direct and indirect trust is evaluated of devices and compared the IPT of direct trust and indirect trust. After this all calculation, the cumulative trust is evaluated. If the threshold is less than then the device is trust worthy and selected as trustworthy path and finish the process.
5.1.Trust evaluation module
This module is very comprehensive and deals with the monitoring of the packet transmission and reception at different devices which records the device’s behavior concerning data rate or throughput along with packet drop rate. Packet profiler is maintained at each device to keep the record of each device behavior and the essential information of the packet traffic.
Fig. 2.
Flow diagram of TEMSG model.
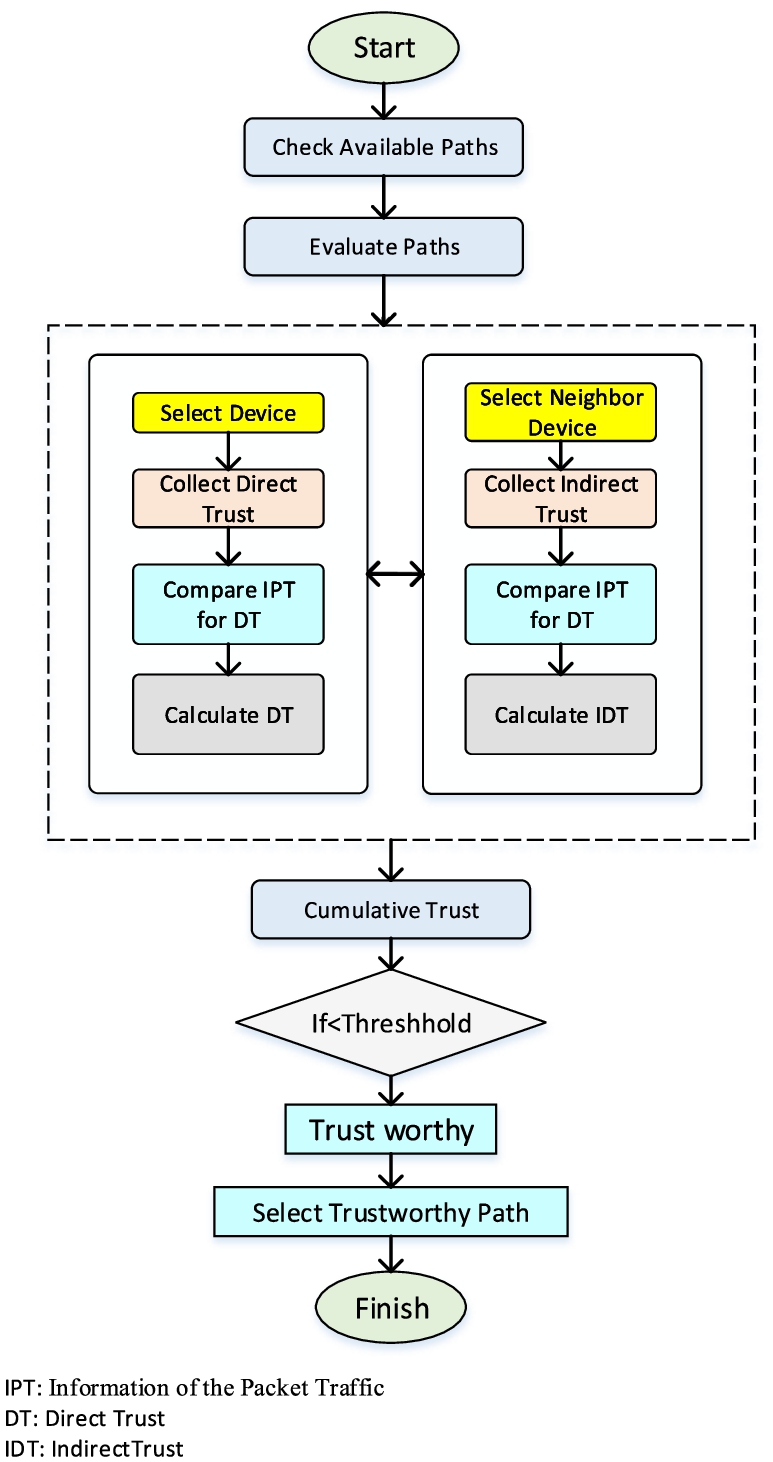
The secondary part deals with the analytical evaluation of the packet traffic concerning the data rate and the data drop rate with respect of time of packet transmitted from source device and packet received at destination or the intermediate devices, and using these trafficking parameters the Direct Trust (DT) and Indirect-Trust (IDT) among the devices involved in analysis and further the output of this modules facilitates in identification of malicious or selfish devices and selection of the trust worthy devices and further the trust worthy path for the data transmission.
Here the direct and indirect trust is calculated and further it jointly creates the cumulative trust i.e. CT(Indirect trust, Direct trust). The trust of the adjacent devices basing up on its packet data rate, and packet drop rate in the time domain. It computes the overall cumulative trust of devices, as the device indirect-direct trust along with direct trust values both are calculated, for which the module considered the record and profile of IPT created and stored at each device composed of different types of packet information like Packet Sent (PS) from transmitting device, Time taken in Packet Sent (TPS) from transmitting device, Packet received (PR) at recipient device, Time taken by recipient device in Receiving the Packet (TPR) and the Total Number of Packets (TNP) transferred from one device to the another device.
For trust evaluation among smart grid devices, the following parameters are taking into account:
The number of packets sent from source device or the intermediate device acting as a transmitter.
The number of packets received by device which can be destination or the intermediate device acting as a receiver.
The time taken by transmitter device to send or forward the packets to the receiver.
The time taken by receiving device to receive the packets from the transmitter device.
The packet drops ratio of the number of packets dropped while transmission from one device to another device.
These parameters are used to establish the trust and analytically evaluate the trustworthiness of the devices and their relationship. Initially the path detector detects the possible paths among the devices. Path detector is a structure which maintains the devices links entries and calculates the trust values of each device for calculating direct trust among them. The packet information profiler analyses and store the packet data rate and the drop rate existed between devices. The relationship among the devices are testified and prone to be effected due to insecure and unstable transmission channel, due to electromagnetic noise and congestion, which indirectly effects the trust score values. The level of trust worthiness is evaluated based on Theory of Probabilistic Bayesian Estimation which invariable used for substantiation and certification of the device’s trust worthiness. Equation (1) calculates the Direct Trust (DT) among two devices in two executive time intervals including the Packet Sent (PS) and Packet Received (PR) time.
After positive verification, both the DTS-D, D-3 and ITS-D, D-3 are combined and the cumulative trust is calculated between the two devices i.e device S and device 3 by the formula given in the Eq. (3).
5.2.Initializing module
The packet profiling records and the verified and testified results of additive metrics are forwarded to this module for concluding the probability of the trust worthier devices, malicious devices, and selfish devices while comparing the trust values of direct and indirect-direct based trust, and then the cumulative trust of the devices on different possible paths with the thresholds. Normally the trust value ranges from 0 to 1 where if the DT or the IT based trust estimates more than 0.5 then it is considered the trust worthy. If the trust values are equal to 1 then that device is considered to be the most trust worthier. On contrary, if the estimated trust values are less in 0.5 then such device is considered to depict the selfish behavior or the malicious device where if the trust scores are less than 0.3 then the devices are marked as malicious. If the trust score is equal to 0 then that device is the most malicious device having a worst bad behavior or the generator of highest number of packets to create the infinite flood situation over the network and such devices has to be earmarked for elimination from the network, the nomination of malicious device is shown in Fig. 3.
Fig. 3.
Malicious device on the available paths.
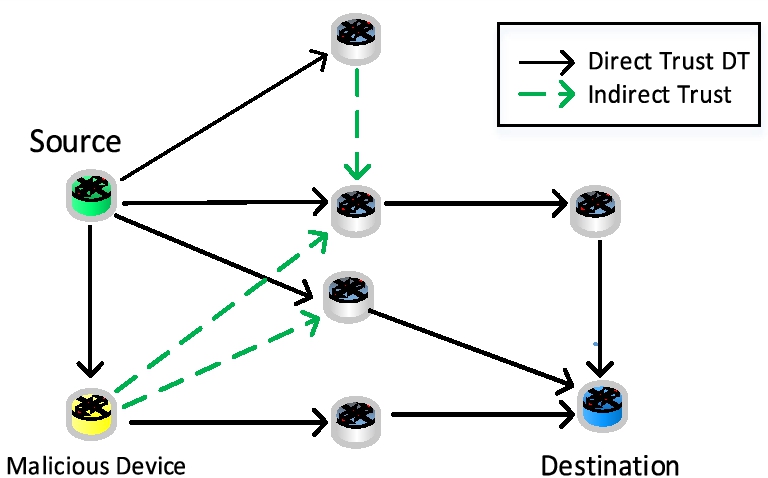
After testing each device values on the possible paths between the source and the destination device, then identifies the highest score cumulative trust and evaluates it against the path threshold cumulative trust value ranging from 0 to 1. If the cumulative trust is above and equal to 0.5 then the path is considered to be trust worthy where as if the path approaches to 0 then the path is considered to be the worst for transmission. The path trust values are updated in the IPT and prepared for selection for the next module. The malicious devices and the bad devices are also earmarked for stoppage, termination or elimination from the network and IPT is updated for such bad devices. The trusted path scenario is illustrated in Fig. 4.
Fig. 4.
Trusted path among the available paths.
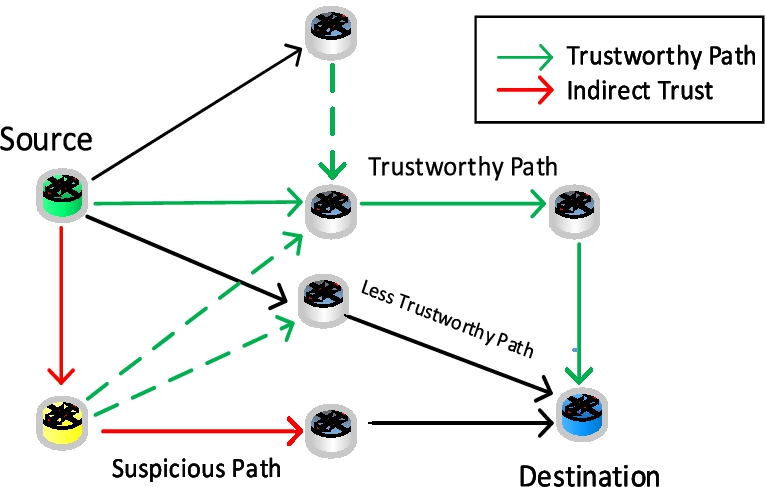
The proposed model evaluates the level of trustworthiness and the integrity of the devices involved in the network and manages the trust based environment by identifying the malicious and selfish devices responsible for causing different internal attacks and causing hindrance in efficient and effective delivery of packets. The proposed model efficiently caters for the false positive ratio and incorrect recommendations and evaluates the trust basing upon the direct trust and indirect based direct trust values of the interconnected or the semi connected values. The trust evaluation involves different communication parameters like packet data rate and packet drop rate basing on packets sent, receive, the time required for both and related attributes.
6.Experimental results
The proposed model is tested in simulation tool OMNET++ which is a discrete event simulator. The deployed devices are randomly distributed in the network. The network area is 100 × 100 meter. The devices communication range set at 20 meter. All devices are static in nature where some devices are malicious devices and simulated by internal attacks. OMNET++ is a graphical user interface based discrete event simulator provides the graphical presentation of the network devices with visual depiction of devices behavior. This tool is suitable for evaluation of trust in smart grid networks due to its diverse descriptive ability to cover the interdevice communication and identification of different types of internal attacks. Table 4 shows the simulation metrics.
Table 4
Simulation parameters
S# | Parameters | Values |
1 | Field size | 100 m × 100 m |
2 | Device deployment | Random |
3 | Simulator time | 100–1000 s |
4 | Traffic type | CBR |
5 | Agent type | UDP |
5 | Packet size | 50 Bytes |
6 | Physical standard | IEEE 802.15.4 |
7 | Traffic load | CBR |
8 | No. of devices | 10, 20, 30, 40, 50, 60, 70, 80 |
9 | Initial power | 100 J |
10 | Tx power | 1 J |
11 | Rx power | 1 J |
12 | Queue type | Drop tail |
13 | Routing protocol | AODV |
The level of trustworthiness, trust detection rate, false-positive rate detection, and end-to-end delay are used for the performance evaluation of the TEMSG mechanism. Check how to evaluate these parameters. The following performance parameters are taking into account to evaluate the proposed approach in the simulator.
Impact of Level of Trustworthiness: This parameter presents the accurate identification percentage of malicious devices and false reporting or data generation of selfish devices after adopting the proposed mechanism.
Impact of Detection Rate: This parameter presents the ratio of the malicious devices detected in lieu of percentage after implementing the proposed approach.
Detection of False Positive Rate: This parameter is used to check the false positive rate of different internal attacks. It is a metric calculated as the number of incorrect positive results divided by a total number of negatives.
End-to-end Delay analysis: This parameter checks the end-to-end delay of the proposed approach in the presence of malicious devices or selfish devices. It is also called one-way delay (OWD) which calculates the time taken for a packet during transmission from host to destination across an interconnected network
The experimental results of TEMSG in terms of trustworthiness in respect of time, malicious nodes, proportional malicious nodes against internal attacks, rate of detecting malicious nodes against internal attacks, rate of accurate detection of malicious nodes, rate of detecting false positive against malicious nodes, average packet delay, average data rate and network lifetime with number of sensor nodes and their trust values with metrics are discussed. The elaborate description of these metrics is as follows:
6.1.Trustworthiness analysis
This parameter presents the accurate identification percentage of malicious devices and false reporting or data generation of selfish devices after adopting the proposed mechanism. This parameter is calculated on the basis of trust threshold values 0.5–1 gathered at a distinctive observation period of simulator execution changing from 100 s to the 1000 s. To ascertain, the ratio of malevolent or false reporting devices is analyzed as the percentage increase while comparing the threshold values with respect to time. The first experiment is to analyze the trust level of the trusted devices among malicious devices in the existing number of devices in a smart grid network. The results of TEMSG is compared with TSP [13], and IoA trust model [5] as illustrated in Fig. 5 and 6. TEMSG shows the increasing trend as compared to the other two trust models due to its predictive behavior and avoiding malicious nodes after time identification. TEMSG achieved a higher trust level. The proposed model also analyzes the false reporting and accurate detection of the malicious and selfish devices with more level of trustworthiness as compared to existing models.
Fig. 5.
Trustworthiness analysis with time.
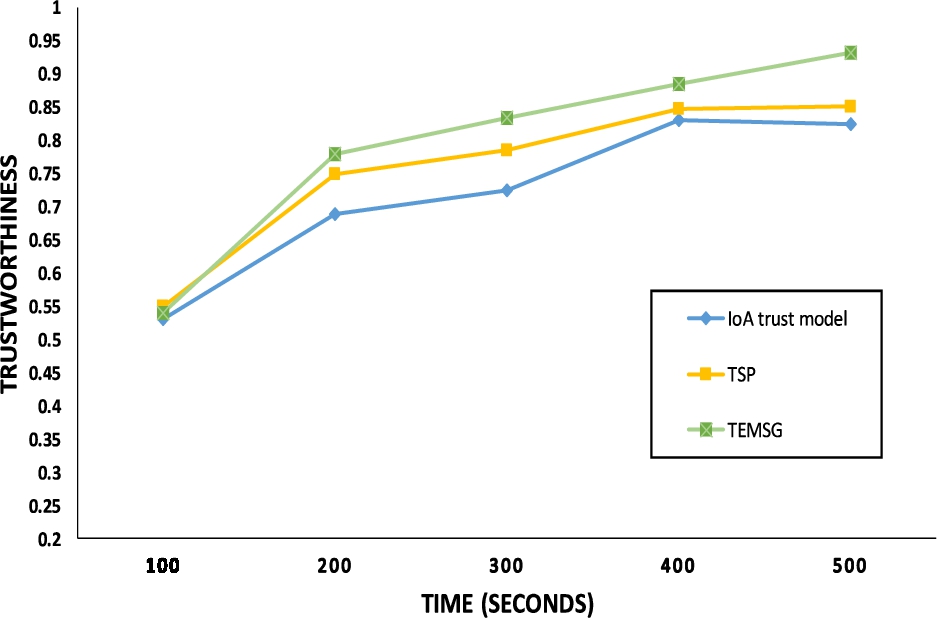
Fig. 6.
Trustworthiness analysis with time.
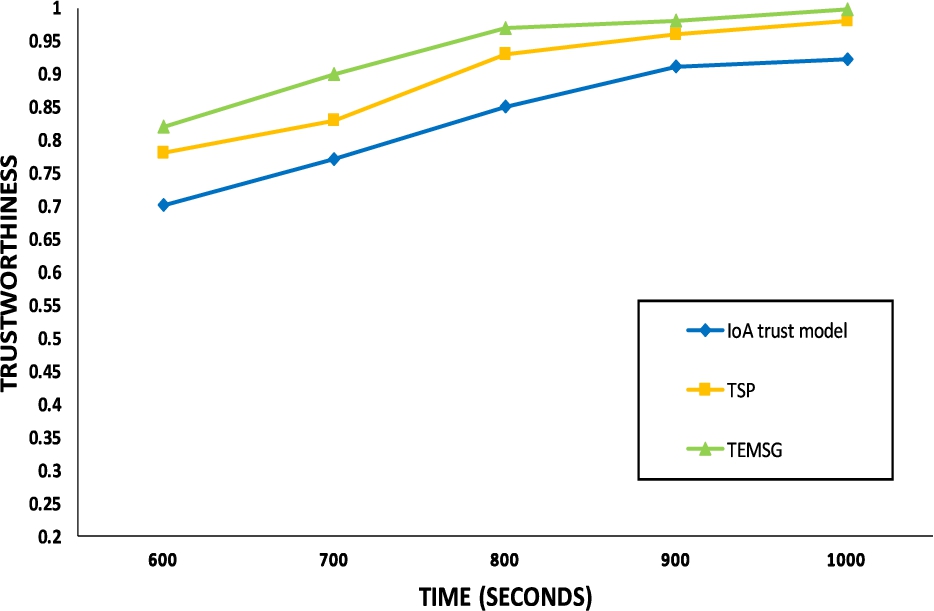
6.2.Detection rate analysis
This analysis shows the ratio of the malicious devices detected after implementing the proposed model. The varying number of malicious devices over the whole topology ranging from 10 to 50 % with 10% increment is simulated against the Bad-mouth, On–Off and DoS attack proving positive trend over the trust detection ratio. The rate of trust detection of trustworthy devices has simulated among the malicious nodes in the smart grid networks. Figure 7 shows the rate of trust detection in the presence of the malicious devices where the TEMSG mechanism shows the high success rate in detecting the malicious devices and identifying the trustworthy nodes as compares to other techniques. The number of malicious nodes is considered in the proportionality of 5 to 50 percentage with 5% increase rate in numbers. TEMSG is compared with TSP [13], and IoA trust model [5]. Figure 7 shows that the trust detection rate of TEMSG is more than due to the capability of detecting the information about the data sent, received and transmitted while performing the trust evaluation of each data packet. It is also observed that a number of malicious devices are inversely proportional to a number of false positives which means an increase in the volume of nodes increases the ratio of more false reporting nodes in the network, hence the probability of malicious node detection is decreased due to increase in sensor nodes.
Fig. 7.
Malicious nodes detection against internal attacks.
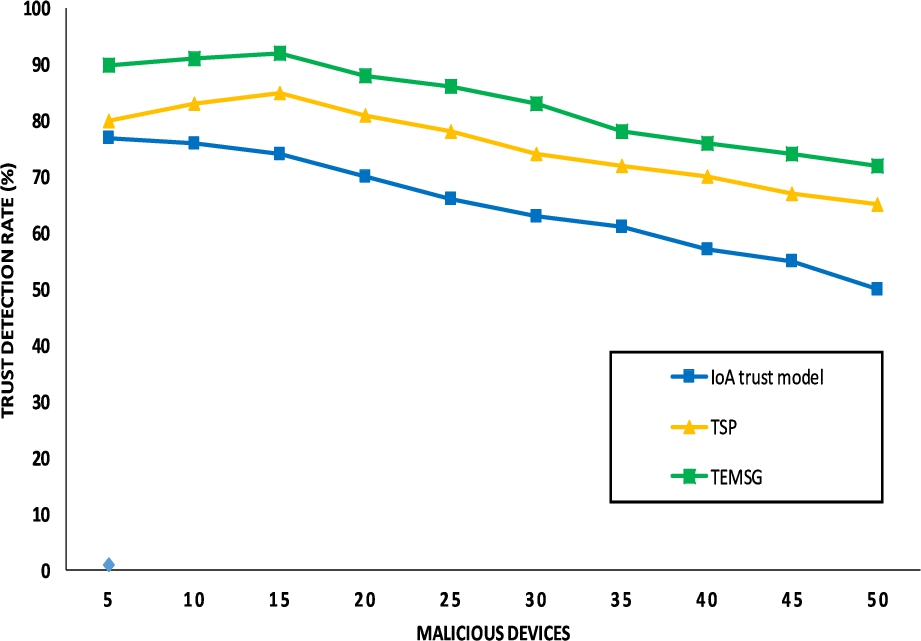
6.3.Detection of false positive rate
This analysis is used to check the false positive rate of different internal attacks. It is a metric calculated as the number of incorrect positive results divided by the total number of negatives results. The best false positive rate is 0.0 whereas the worst is 1. The rate of false positives which analyzed against internal attacks like DoS, Bad Mouthing and On–Off is the influence of the trust values of trusted and malicious devices. False Positive detection rate bears lesser value for the trustworthy devices whereas for the malicious devices it is higher in comparison with the counterpart trust mechanism. TEMSG is compared with TSP [13], and IoA trust model [5] as shows in Fig. 8 and 9.
Fig. 8.
Rate of false positive between 5 to 50 nodes.
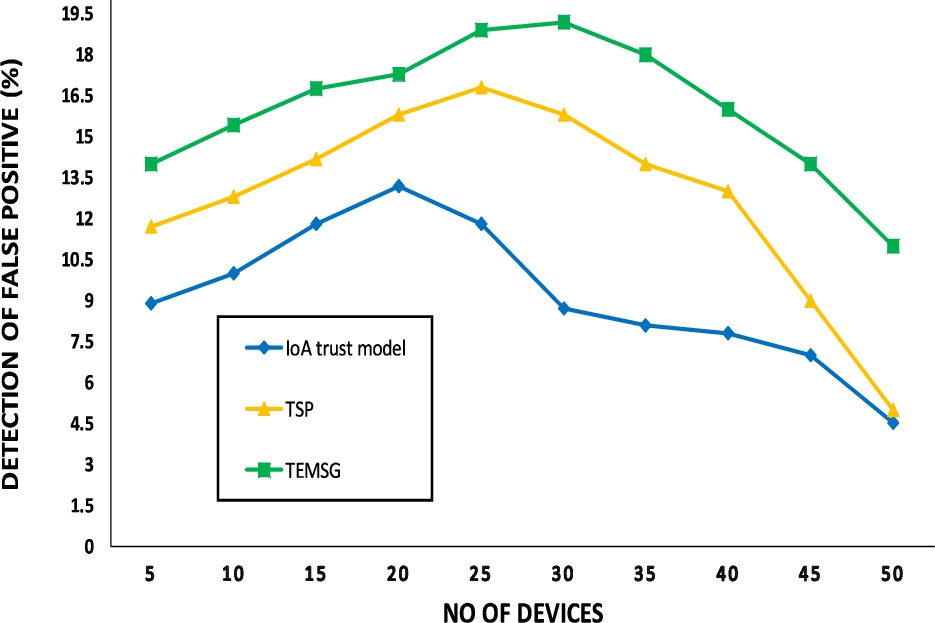
Fig. 9.
Rate of false positive between 55 to 100 nodes.
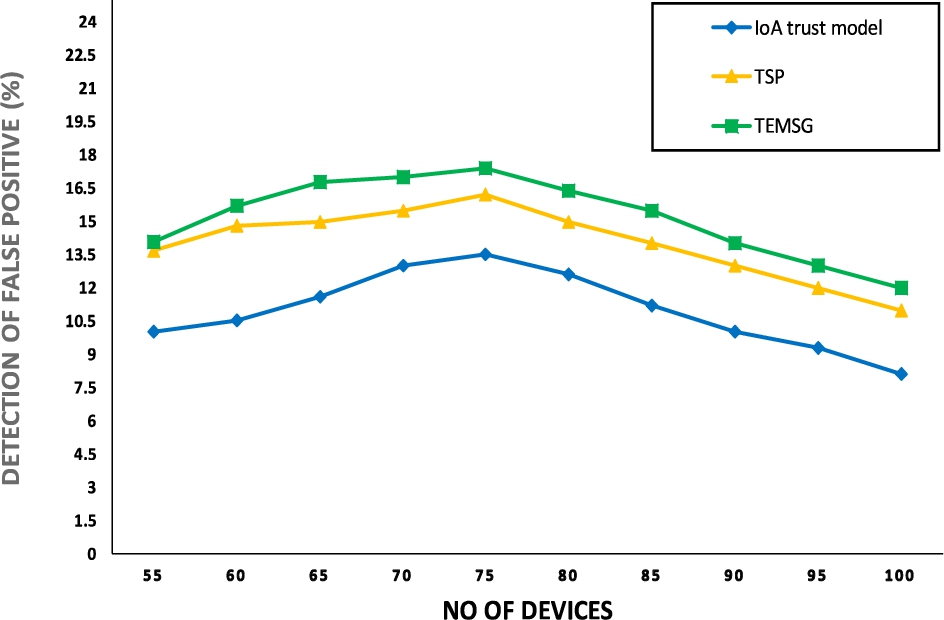
Fig. 10.
Average packet delay ratio with number of devices.
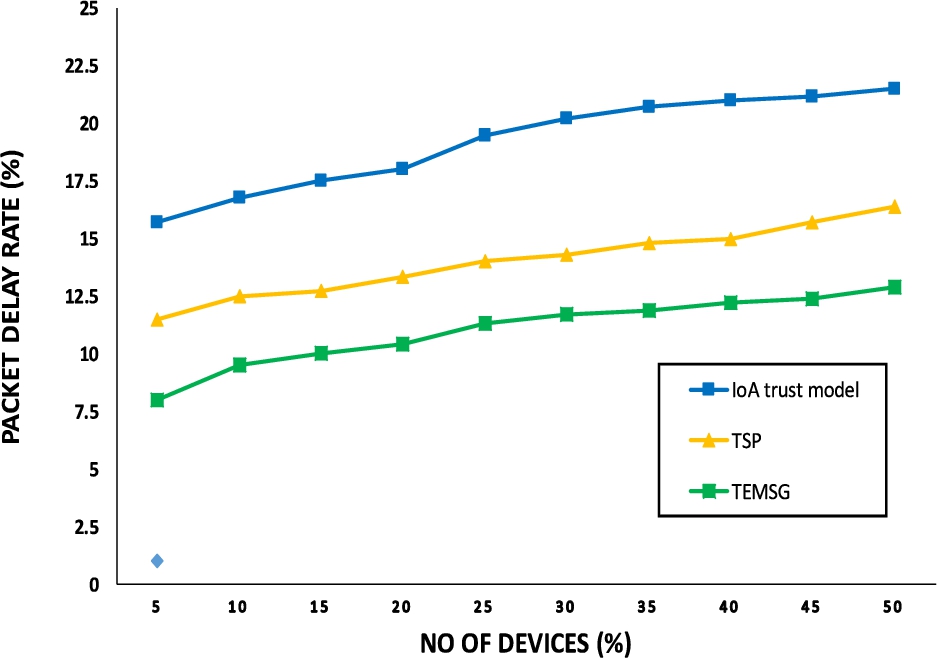
6.4.Average packet delay analysis
This analysis is used to analyze the packet delay persist among the communicating devices in the presence of the malicious devices exhibited during network transmission. It measures the performance of the proposed model considering the lesser packet delay in the presence of malicious devices in the smart grid network. In another scenario, the average packet delay is examined over a varying number of malicious devices. This parameter measures the performance of the proposed model considering the lesser packet delay in the presence of the malicious devices in the network. The average packet delay is still higher than its counterpart trust models i.e. like TEMSG is compared with TSP [13], and IoA trust model [5] respectively as shown in Fig. 10.
7.Conclusion
In this paper, Trust Evaluation Model for Smart Grids (TEMSG) model is presented which applies to the smart grid devices based interconnected networks with a minimum overhead with greater packet data rate and trustworthiness level. TEMSG enhances services in the existing smart grid networks in terms of their operation, tangibility processing, architectures and securing the smart grid devices with the establishment of trustworthy mechanisms. TEMSG tackles the different internal attacks and identifying the malicious and selfish nodes in the smart grid networks. The simulation results depicted that TEMSG has high data delivery ratio, minimum delay, and better performance smart grid network by using the Bayesian estimation and the interaction quality metric to gather the direct and indirect trust values of the devices based to correlate the data over the time-lapses and select the trustworthy devices and trust worthier path for data transmission. In future, the proposed trust evaluation model will be tested to detect other internal and external attacks which makes the devices malicious, selfish and bad/contradictory like Sybil, Wormhole, Conflicting Behavior, Routing Attacks, Camouflages Adversaries and Ballot Stuffing.
References
[1] | A. Ahmad, A. Paul, M.M. Rathore and H. Chang, Smart cyber society: Integration of capillary devices with high usability based on cyber–physical system, Future Generation Computer Systems 56: ((2016) ), 493–503. doi:10.1016/j.future.2015.08.004. |
[2] | M.S. Aliero, K.N. Qureshi, M.F. Pasha, I. Ghani and R.A. Yauri, Systematic review analysis on SQLIA detection and prevention approaches, Wireless Personal Communications ((2020) ), 1–37. |
[3] | A. Alnasser and H. Sun, A fuzzy logic trust model for secure routing in smart grid networks, IEEE Access 5: ((2017) ), 17896–17903. doi:10.1109/ACCESS.2017.2740219. |
[4] | S. Anwar, F. Al-Obeidat, A. Tubaishat, S. Din, A. Ahmad, F.A. Khan, G. Jeon and J. Loo, Countering malicious URLs in Internet-of-Thing (IoT) using a knowledge-based approach and simulated expert, IEEE Internet of Things Journal ((2019) ). |
[5] | A. Aref and T. Tran, Multi-criteria trust establishment for internet of agents in smart grids, Multiagent Grid Systems 13: (3) ((2017) ), 287–309. doi:10.3233/MGS-170272. |
[6] | N. Chaudhry, M.M. Yousaf and M.T. Khan, Indexing of real time geospatial data by IoT enabled devices: Opportunities, challenges and design considerations, Journal of Ambient Intelligence and Smart Environments ((2020) ), 1–32, Preprint. |
[7] | D.A. Chekired, L. Khoukhi and H.T. Mouftah, Decentralized cloud-SDN architecture in smart grid: A dynamic pricing model, IEEE Transactions on Industrial Informatics 14: (3) ((2017) ), 1220–1231. doi:10.1109/TII.2017.2742147. |
[8] | J.E. Fadul, K.M. Hopkinson, T.R. Andel and C.A. Sheffield, A trust-management toolkit for smart-grid protection systems, IEEE Transactions on Power Delivery 29: (4) ((2013) ), 1768–1779. doi:10.1109/TPWRD.2013.2289747. |
[9] | M. Farmanbar, K. Parham, Ø. Arild and C. Rong, A widespread review of smart grids towards smart cities, Energies 12: (23) ((2019) ), 4484. doi:10.3390/en12234484. |
[10] | A. Ghosal and S. Halder, A survey on energy efficient intrusion detection in wireless sensor networks, Journal of Ambient Intelligence and Smart Environments 9: (2) ((2017) ), 239–261. doi:10.3233/AIS-170426. |
[11] | B. Gong, Y. Zhang and Y. Wang, A remote attestation mechanism for the sensing layer nodes of the Internet of Things, Future Generation Computer Systems 78: ((2018) ), 867–886. |
[12] | K. Govindan and P. Mohapatra, Trust computations and trust dynamics in mobile adhoc networks: A survey, IEEE Communications Surveys & Tutorials 14: ((2011) ), 279–298. |
[13] | M.M. Hasan and H. Mouftah, Optimal trust system placement in smart grid SCADA networks, IEEE Access 4: ((2016) ), 2907–2919. doi:10.1109/ACCESS.2016.2564418. |
[14] | H. He and J. Yan, Cyber-physical attacks and defences in the smart grid: A survey, IET Cyber-Physical Systems: Theory Applications 1: (1) ((2016) ), 13–27. doi:10.1049/iet-cps.2016.0019. |
[15] | S. Iqbal, A.H. Abdullah, M.M. Mohamad, K.N. Qureshi and K. Hussain, Adaptive interface reconfiguration in low-rate mesh WPANs, Journal of Computational Theoretical Nanoscience 13: (7) ((2016) ), 4703–4710. doi:10.1166/jctn.2016.5340. |
[16] | S. Iqbal, A.H. Abdullah and K.N. Qureshi, An adaptive interference-aware and traffic-aware channel assignment strategy for backhaul networks, Concurrency Computation: Practice Experience ((2019) ), e5650. |
[17] | J. Jiang, G. Han, F. Wang, L. Shu and M. Guizani, An efficient distributed trust model for wireless sensor networks, IEEE Transactions on Parallel and Distributed Systems 26: ((2014) ), 1228–1237. |
[18] | J. Jow, Y. Xiao and W. Han, A survey of intrusion detection systems in smart grid, International Journal of Sensor Networks 23: (3) ((2017) ), 170–186. doi:10.1504/IJSNET.2017.083410. |
[19] | J.M. Junior, J.P.C. da Costa, C.C. Garcez, R. de Oliveira Albuquerque, A. Arancibia, L. Weichenberger, F.L.L. de Mendonça and G.d. Galdo, Data security and trading framework for smart grids in neighborhood area networks, Sensors 20: (5) ((2020) ), 1337. doi:10.3390/s20051337. |
[20] | N. Karthik and V.S. Ananthanarayana, A hybrid trust management scheme for wireless sensor networks, Wireless Personal Communications 97: ((2017) ), 5137–5170. |
[21] | S. Kumar, U. Dohare, K. Kumar, D.P. Dora, K.N. Qureshi and R. Kharel, Cybersecurity measures for geocasting in vehicular cyber physical system environments, IEEE Internet of Things Journal 6: (4) ((2018) ), 5916–5926. doi:10.1109/JIOT.2018.2872474. |
[22] | L. Lampe, A.M. Tonello and T.G. Swart, Power Line Communications: Principles, Standards and Applications from Multimedia to Smart Grid, John Wiley & Sons, (2016) . |
[23] | F. Li and L. Yang, Securing smart grid in-network aggregation through false data detection, 2017. |
[24] | W. Li, H. Song and F. Zeng, Policy-based secure and trustworthy sensing for Internet of Things in smart cities, IEEE Internet of Things Journal 5: (2) ((2018) ), 716–723. |
[25] | X. Li, X. Liang, R. Lu, X. Shen, X. Lin and H. Zhu, Securing smart grid: Cyber attacks, countermeasures, and challenges, IEEE Communications Magazine 50: (8) ((2012) ), 38–45. doi:10.1109/MCOM.2012.6257525. |
[26] | Y. Liu, W. Guo, C.-I. Fan, L. Chang and C. Cheng, A practical privacy-preserving data aggregation (3PDA) scheme for smart grid, IEEE Transactions on Industrial Informatics 15: (3) ((2018) ), 1767–1774. doi:10.1109/TII.2018.2809672. |
[27] | F. Mannhardt, S.A. Petersen and M.F. Oliveira, A trust and privacy framework for smart manufacturing environments, Journal of Ambient Intelligence and Smart Environments 11: (3) ((2019) ), 201–219. doi:10.3233/AIS-190521. |
[28] | C.V.L. Mendoza and J.H. Kleinschmidt, A distributed trust management mechanism for the Internet of things using a multi-service approach, Wireless Personal Communications 103: ((2018) ), 2501–2513. |
[29] | W. Mesbah, Securing smart electricity meters against customer attacks, IEEE Transactions on Smart Grid 9: (1) ((2016) ), 101–110. doi:10.1109/TSG.2016.2545524. |
[30] | S. Nie, A novel trust model of dynamic optimization based on entropy method in wireless sensor networks, Cluster Computing 22: ((2019) ), 11153–11162. |
[31] | P. Parvin, S. Chessa, M. Kaptein and F. Paternò, Personalized real-time anomaly detection and health feedback for older adults, Journal of Ambient Intelligence and Smart Environments 11: (5) ((2019) ), 453–469. doi:10.3233/AIS-190536. |
[32] | K.N. Qureshi, M.U. Bashir, J. Lloret and A. Leon, Optimized cluster-based dynamic energy-aware routing protocol for wireless sensor networks in agriculture precision, Journal of Sensors 2020: ((2020) ). |
[33] | M. Talaat, A.S. Alsayyari, A. Alblawi and A. Hatata, Hybrid-cloud-based data processing for power system monitoring in smart grids, Sustainable Cities and Society 55: ((2020) ), 102049. doi:10.1016/j.scs.2020.102049. |
[34] | S. Tan, D. De, W.-Z. Song, J. Yang and S.K. Das, Survey of security advances in smart grid: A data driven approach, IEEE Communications Surveys & Tutorials 19: (1) ((2017) ), 397–422. doi:10.1109/COMST.2016.2616442. |
[35] | S. Theodorou and N. Sklavos, Blockchain-based security and privacy in smart cities, in: Smart Cities Cybersecurity and Privacy, Elsevier, (2019) , pp. 21–37. doi:10.1016/B978-0-12-815032-0.00003-2. |
[36] | Y. Wadhawan, A. AlMajali and C. Neuman, A comprehensive analysis of smart grid systems against cyber-physical attacks, Electronics 7: (10) ((2018) ), 249. doi:10.3390/electronics7100249. |
[37] | E.K. Wang, Y. Li, Y. Ye, S.-M. Yiu and L.C.K. Hui, A dynamic trust framework for opportunistic mobile social networks, IEEE Transactions on Network and Service Management 15: ((2017) ), 319–329. |
[38] | H. Wang, L. Xu and G. Gu, Floodguard: A dos attack prevention extension in software-defined networks, in: 2015 45th Annual IEEE/IFIP International Conference on Dependable Systems and Networks, IEEE, (2015) , pp. 239–250. doi:10.1109/DSN.2015.27. |
[39] | P. Wang and M. Govindarasu, Multi-agent based attack-resilient system integrity protection for smart grid, IEEE Transactions on Smart Grid ((2020) ). |
[40] | C.W.R. Webster and C. Leleux, Searching for the real sustainable smart city?, Information Polity 24: (3) ((2019) ), 229–244. doi:10.3233/IP-190132. |
[41] | Z. Yan, P. Zhang and A.V. Vasilakos A survey on trust management for Internet of Things, Journal of Network and Computer Applications 42: ((2014) ), 120–134. |
[42] | F. Zawaideh and M. Salamah, An efficient weighted trust-based malicious node detection scheme for wireless sensor networks, International Journal of Communication Systems 32: ((2019) ), e3878. |
[43] | K. Zhang, J. Ni, K. Yang, X. Liang, J. Ren and X.S. Shen, Security and privacy in smart city applications: Challenges and solutions, IEEE Communications Magazine 55: (1) ((2017) ), 122–129. doi:10.1109/MCOM.2017.1600267CM. |