Socio-Cognitive Determinants of Lifestyle Behavior in the Context of Dementia Risk Reduction: A Population-Based Study in the Netherlands
Abstract
Background:
Unhealthy behavior increases the risk of dementia. Various socio-cognitive determinants influence whether individuals persist in or alter these unhealthy behaviors.
Objective:
This study identifies relevant determinants of behavior associated to dementia risk.
Methods:
4,104 Dutch individuals (40–79 years) completed a screening questionnaire exploring lifestyle behaviors associated with dementia risk. Subsequently, 3,065 respondents who engaged in one or more unhealthy behaviors completed a follow-up questionnaire investigating socio-cognitive determinants of these behaviors. Cross-tables were used to assess the accuracy of participants’ perceptions regarding their behavior compared to recommendations. Confidence Interval-Based Estimation of Relevance (CIBER) was used to identify the most relevant determinants of behavior based on visual inspection and interpretation.
Results:
Among the respondents, 91.3% reported at least one, while 65% reported two or more unhealthy lifestyle behaviors associated to dementia risk. Many of them were not aware they did not adhere to lifestyle recommendations. The most relevant determinants identified include attitudes (i.e., lacking a passion for cooking and finding pleasure in drinking alcohol or smoking), misperceptions on social comparisons (i.e., overestimating healthy diet intake and underestimating alcohol intake), and low perceived behavioral control (i.e., regarding changing physical inactivity, altering diet patterns, and smoking cessation).
Conclusions:
Individual-level interventions that encourage lifestyle change should focus on enhancing accurate perceptions of behaviors compared to recommendations, while strengthening perceived control towards behavior change. Given the high prevalence of dementia risk factors, combining interventions at both individual and environmental levels are likely to be the most effective strategy to reduce dementia on a population scale.
INTRODUCTION
A growing body of evidence has identified modifiable risk and protective factors for dementia [1, 2]. These insights pave the way for the development of individual and environmental level preventive interventions to reduce the rising total prevalence of dementia. The current strategy strongly revolves around multidomain approaches to encourage healthy lifestyle changes among individuals at-risk [3], particularly in middle and older age. Across the lifespan, a wide variety of modifiable lifestyle factors have been associated with dementia risk [1], such as physical inactivity, unhealthy diet, psychological stress, smoking, low sleep quality, and limited social and cognitive stimulating activities [4–6]. All these lifestyle factors are partly influenced by behavior specific socio-cognitive determinants that determine if individuals persist in or alter their behavior [7, 8]. Examples of socio-cognitive determinants are attitudes (i.e., a latent disposition or tendency to respond with favorableness or unfavorableness to a behavior), social norms (i.e., the perceived social pressure to perform or not perform a behavior), and perceived behavioral control (i.e., the perceived degree of being capable of, or have control over, performing a behavior) [9].
These socio-cognitive determinants influence behavioral choices, yet environmental factors also play a pivotal role [10, 11]. For instance, such environmental factors include the availability of green spaces for exercise, the affordability of fruits and vegetables, as well as policies that prohibit the sale of alcohol or impose taxes on tobacco products. These environmental factors can impact behavior directly or indirectly by influencing socio-cognitive determinants such as attitudes, norms, and perceived behavioral control [8]. This underscores the complexity of behavior change. While there is extensive literature on determinants of behavior [8], insights into the specific determinants relevant to dementia risk reduction are limited. For example, the Motivation to Change Lifestyle and Health Behaviors for Dementia Risk Reduction Scale (MCLHB-DRR) offers understanding of determinants of generic lifestyle change [12], without delving into determinants of specific behaviors, such as attitudes about diet or self-efficacy towards smoking cessation. This represents a limitation, as individuals are often more positive towards broader lifestyle change than to altering particular unhealthy behaviors [13]. Furthermore, it is well-known that determinants are highly behavior specific, which makes it impossible to intuitively select relevant determinants, even for experts in behavior change [14].
With this study, we aim to obtain a better understanding of relevant socio-cognitive determinants in the context of dementia risk reduction, to inform the selection of appropriate behavior change methods to change these determinants through interventions [7, 8]. The current focus is primarily at the individual level, as this research is part of a larger project that includes a clinical trial on a digitally supported lifestyle program to promote brain health among older adults (LETHE, clinicaltrial.gov identifier NCT05565170) [15, 16]. Nevertheless, the findings may offer valuable insights for new research to explore how environmental factors influence behavior directly and through socio-cognitive determinants.
METHODS
In this cross-sectional study, we used a screening questionnaire to identify individuals with room to improve their lifestyle behavior and we invited them for a follow-up questionnaire to explore socio-cognitive determinants of these behaviors. The study protocol was pre-registered [17] and materials and data to replicate the findings are open access available [18]. The findings are reported using the Strengthening the Reporting of Observational studies in Epidemiology (STROBE) checklist [19].
Recruitment
In September 2022, respondents were recruited via the International Organization Standardization (ISO)-certified Internet research agency Flycatcher that has a large panel in the Netherlands. Dutch speaking respondents aged 40 to 79 years were eligible for participation because lifestyle behavior is associated with dementia in mid-life and late-life [20, 21].
A sample size estimation indicated that at least 4,000 respondents were required for the screening questionnaire to ensure sufficient statistical power for analyses using data from the follow-up. This estimation accounted for population prevalence of lifestyle behaviors (i.e., smoking had the lowest population prevalence, ≈20% [22]), the response rate expectancy of Flycatcher (≈75%), and the ability to detect correlations coefficients of 0.2 with a half-width of 0.1 using a confidence interval of 95% [23]).
Questionnaire development
Questionnaires were developed in triangulation with literature, an interview study (n = 23) [13] and think-aloud sessions with experts in dementia risk reduction (n = 4). The questionnaires were pre-tested with three middle-aged and older individuals of low socio-economic status (SES) who participate in an advisory board consulting on research. This iterative and collaborative development process refined our approach. For instance, the screening questionnaire included twelve modifiable risk and protective factors from the LIfestyle for BRAin health (LIBRA) index [6, 24] to identify respondents with room for lifestyle improvement and experts assisted with setting inclusion cut-offs based on their experience and research.
The follow-up questionnaire assessed socio-cognitive determinants derived from various theories to achieve a comprehensive understanding of their impact [8, 25]. Definitions and measurement instructions for behavioral determinant research informed the item development [26]. Insights from a prior interview study [13] led to the development of items targeting behavior-specific determinants. For example, interviewees frequently mentioned they did not enjoy cooking, which resulted in an item to assess attitudes towards cooking. Expert think-aloud sessions and a pre-test with low SES individuals supported further refinement of the items in terms of face and content validity.
The screening questionnaire
The screening questionnaire, based on the LIBRA index, aimed to identify respondents who actively engaged in one or more unhealthy lifestyle behaviors. This included physical inactivity, low adherence to a Mediterranean diet, overconsumption of alcohol, smoking, and low engagement in social and cognitive activities. The 9-item Rapid Assessment of Physical Activity (RAPA) [27] was used to identify respondents who were physically inactive, according to Dutch recommendations of doing at least 150 minutes of moderate-to-vigorous activity per week, spread over several days [28]. The 14-item Mediterranean Diet Adherence Screener (MEDAS14; scores ranging from 0–14) [29] was utilized to select respondents with low adherence to the Mediterranean Diet (i.e., score≤5). According to Dutch diet guidelines, the Mediterranean diet aligns with most recommendations by emphasizing high consumption of vegetables, fruits, whole grain products, nuts, legumes, oils, unsaturated fats, poultry, and fish as well as low intake of red or processed meat, high-fat dairy, hard fats, salt, and sugar-sweetened beverages [30]. Two items from the Alcohol Use Disorders Identification Test (AUDIT) [31, 32] were utilized to identify respondents who consumed more than 1 unit of alcohol per day on average. Although research on dementia risk suggests higher alcohol intake as harmful (i.e., 14 to 21 units per week), the approach used in this study aligns with existing recommendations in the Netherlands to prevent disease in general [30]. Self-constructed items were employed to identify respondents who smoked and to assess the number of cigarettes (or other tobacco products) they consumed daily [33]. Engagement in social and cognitive activities was assessed using a self-constructed list of 12 activities, based on previous questionnaires used in reseaerch on dementia risk reduction [34]. Respondents were classified as socially and cognitively inactive based on a < median-split of weekly activities within our sample [33].
Other health-related modifiable risk factors included in LIBRA were assessed by asking respondents if a doctor had ever informed them about having coronary heart disease, renal dysfunction, diabetes, high cholesterol, hypertension, or depression [35]. Obesity (body mass index, BMI≥30) was determined using self-reported weight and height (i.e., BMI = kg/m2).
The follow-up questionnaire
Respondents who actively engaged in one or more unhealthy lifestyle behaviors were invited to participate in the follow-up questionnaire exploring socio-cognitive determinants of these behaviors. The follow-up questionnaire employed self-constructed items assessing determinants separately for physical activity, Mediterranean diet, alcohol intake, smoking, and engagement in social and cognitive activities. All respondents completed items on physical activity, Mediterranean diet, and social and cognitive activities because, to an extent, everyone engages in these behaviors. Items on alcohol and smoking were only completed by participants who indicated to drink alcohol or smoke.
The follow-up questionnaire utilized tailored items for each lifestyle behavior to investigate potentially relevant determinants. Overall, the following categories of socio-cognitive determinants were assessed for each lifestyle behavior separately: (a) beliefs about engaging in sufficient levels of a particular behavior, (b) intentions to change behavior, (c) attitudes, (d) risk perceptions, (e) social and environmental influences, and (f) perceived behavioral control.
Data analyses
Distribution and spread were calculated for sample characteristics in SPSS. A summative LIBRA-score was calculated, ranging from –5.9 to +12.7, with higher scores indicating a higher relative risk for dementia [6]. Cross-tables were calculated to assess the extent to which respondents perceived they engaged in sufficient levels of a specific lifestyle behavior, in comparison to Dutch lifestyle recommendations, which served as cut-offs for inviting respondents for the follow-up questionnaire.
Per lifestyle behavior the most relevant determinants were identified using Confidence Interval-Based Estimation of Relevance (CIBER) [14, 36]. CIBER was favored over regression analysis due to potential distortion caused by theoretical overlap among determinants [37]. Utilizing diamond plots, CIBER visualizes univariate distributions of items that measure socio-cognitive determinants and their bivariate association with behavior [14]. These plots allow to identify the relevance of determinants based on visual inspection and interpretation. Specifically, scoring distribution and means provide an indication of the room for improvement at the determinant level [36]. In an intervention context, altering a behavioral determinant is relevant only when there is room for improvement. For instance, enhancing knowledge is meaningful if respondents have limited knowledge. Additionally, plotted zero-order associations between determinants and behavior indicate if it is relevant to alter a determinant [36]. For instance, increasing limited knowledge is only relevant if it is associated with healthier behavior.
The CIBER function is part of the R package behaviorchange [38]. We used the binaryCIBER function to identify relevant socio-cognitive determinants for physical inactivity, given the outcome of RAPA is binary (i.e., physically inactive versus physically active). For assessing determinants of the other lifestyle behaviors, we employed the regular CIBER function, which uses continuous outcome scores. Specifically, separate CIBER plots were calculated using summative outcome scores for Mediterranean diet, weekly units of alcohol intake, daily number of tobacco products, and weekly social and cognitive activities.
Ethics
The study was approved by the Research Ethics Committee of the Faculty of Health, Medicine and Life Sciences of Maastricht University, the Netherlands (FHML-REC/2022/064). Participants gave digital consent and received a standard compensation through Flycatcher of € 0.60 (≈$0.65) for the screening questionnaire and € 2.00 (≈$2.18) for the follow-up questionnaire.
RESULTS
The screening questionnaire was sent to 6,228 panel members and was completed by 4,104 respondents (65.9%). Of these respondents, 91.3% (n = 3,746) reported at least one unhealthy lifestyle behavior (Table 1), while 65% (n = 2,666) reported two or more. The follow-up questionnaire was sent to 3,746 respondents and was completed by 81.6% (n = 3,056).
Table 1
Respondent characteristics and risk factors (N = 4,104)
Age in years, Mean (SD); min to max | 59.1 (10.57); 40 to 79 |
Gender, male (%)/female (%) | 1,710 (41.7)/2,394 (58.3) |
Migration background, N (%) | |
First generation | 158 (3.8) |
Second generation | 347 (8.5) |
Highest completed educational level1, N (%) | |
Low | 823 (20.1) |
Medium | 1,545 (37.6) |
High | 1,736 (42.3) |
LIBRA-factors, N (%) | |
Physical inactivity* | 2,172 (52.9%) |
Low adherence to Mediterranean diet* | 2,237 (54.5%) |
Overconsumption of alcohol* | 1,005 (24.5%) |
Smoking* | 456 (11.1%) |
Low social and cognitive activity* | 2,057 (50.1%) |
Coronary heart disease | 652 (15.9%) |
Renal dysfunction | 84 (2.0%) |
Diabetes | 434 (10.6%) |
High blood cholesterol | 1,257 (30.6%) |
Obesity** | 918 (22.4%) |
Hypertension | 1,400 (34.1%) |
Depression | 734 (17.9%) |
LIBRA-score, Mean (SD); min to max | 0.35 (2.90); –5.9 to+10.6 |
Percentiles, 25;50;75 | –2.6; –0.5; 1.6 |
Protective factors are inverted and represented as risk factors to enhance interpretability. 1Based on educational classification (SOI 21) of Statistics Netherlands *Behaviors used to invite respondents for the follow-up questionnaire. **Calculated with data of 4,099 respondents.
Perceptions about behavior versus lifestyle recommendations
Cross-tables (Table 2) demonstrate that 39.4% of the respondents, whom we considered to be physically inactive, perceived that they engaged in sufficient levels of physical activity. For Mediterranean diet, this figure was 54.8%; for alcohol consumption, it was 43.2%; and for cognitive and social engagement, it was 51.5%.
Table 2
Perceptions about behavior versus lifestyle recommendations
Do you think you do enough weekly physical activity? (n = 3,065) | ||
Physically inactive , N (%) | Physically active, N (%) | |
Yes | 701 (39.4%) | 985 (76.5%) |
Somewhat | 680 (38.2%) | 256 (19.9%) |
No | 397 (22.3%) | 46 (3.6%) |
Do you think you eat healthy? (n = 3,065) | ||
Low Mediterranean diet, N (%) | Medium-high Mediterranean diet, N (%) | |
Yes | 1007 (54.8%) | 943 (76.8%) |
Somewhat | 753 (41.0%) | 273 (22.2%) |
No | 77 (4.2%) | 12 (1.0%) |
Do you think you overconsume alcohol? (n = 2,410)* | ||
Overconsumption of alcohol , N (%) | Regular consumption of alcohol, N (%) | |
Yes | 93 (11.1%) | 4 (.2%) |
Somewhat | 384 (45.7%) | 122 (5.5%) |
No | 363 (43.2%) | 1444 (64.9%) |
Do you think that you are socially and actively engaged in life? (n = 3,065) | ||
Low activities , N (%) | Medium-high activities, N (%) | |
Yes | 423 (51.5%) | 1506 (67.1%) |
Somewhat | 321 (39.1%) | 601 (26.8%) |
No | 77 (9.4%) | 137 (6.1%) |
Physically inactive is less than 150 min of moderate or 60 min of vigorous activity spread over several days of the week. Low adherence to Mediterranean diet is a summative score≤5 on the MEDAS14. Overconsumption of alcohol is drinking over 1 unit per day on average (these items were only completed by respondents who indicated to consume alcohol). Low activity is based on the lowest 25% of weekly social and cognitive activities.
Interpretating CIBER-plots to indicate relevant behavioral determinants
Figures 1and 2 present the CIBER-plots, visualizing scoring distributions with scatterplots and means, and illustrating associations between determinants and outcome behavior. Tables with the underlying data are available in the Supplementary Material.
Fig. 1
Socio-cognitive determinants of physical activity.
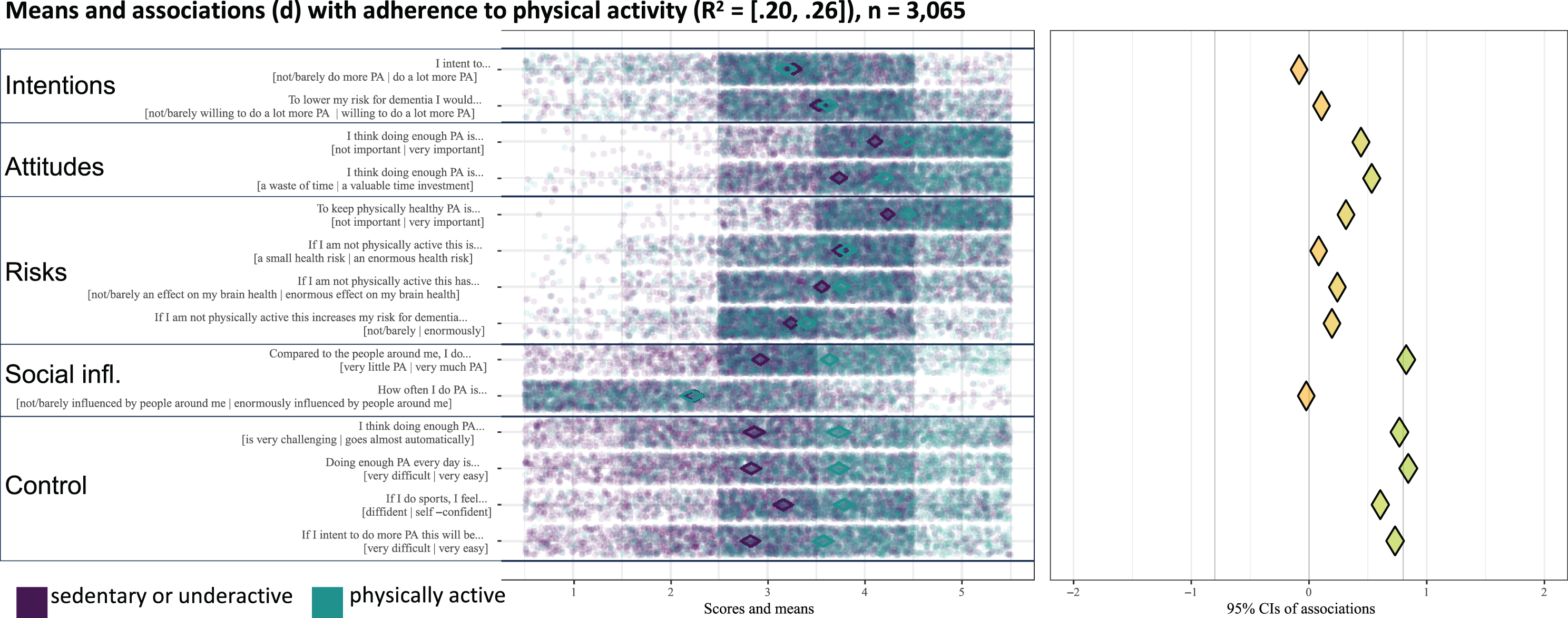
Fig. 2
Socio-cognitive determinants of diet, alcohol intake, smoking, and social and cognitive activity.
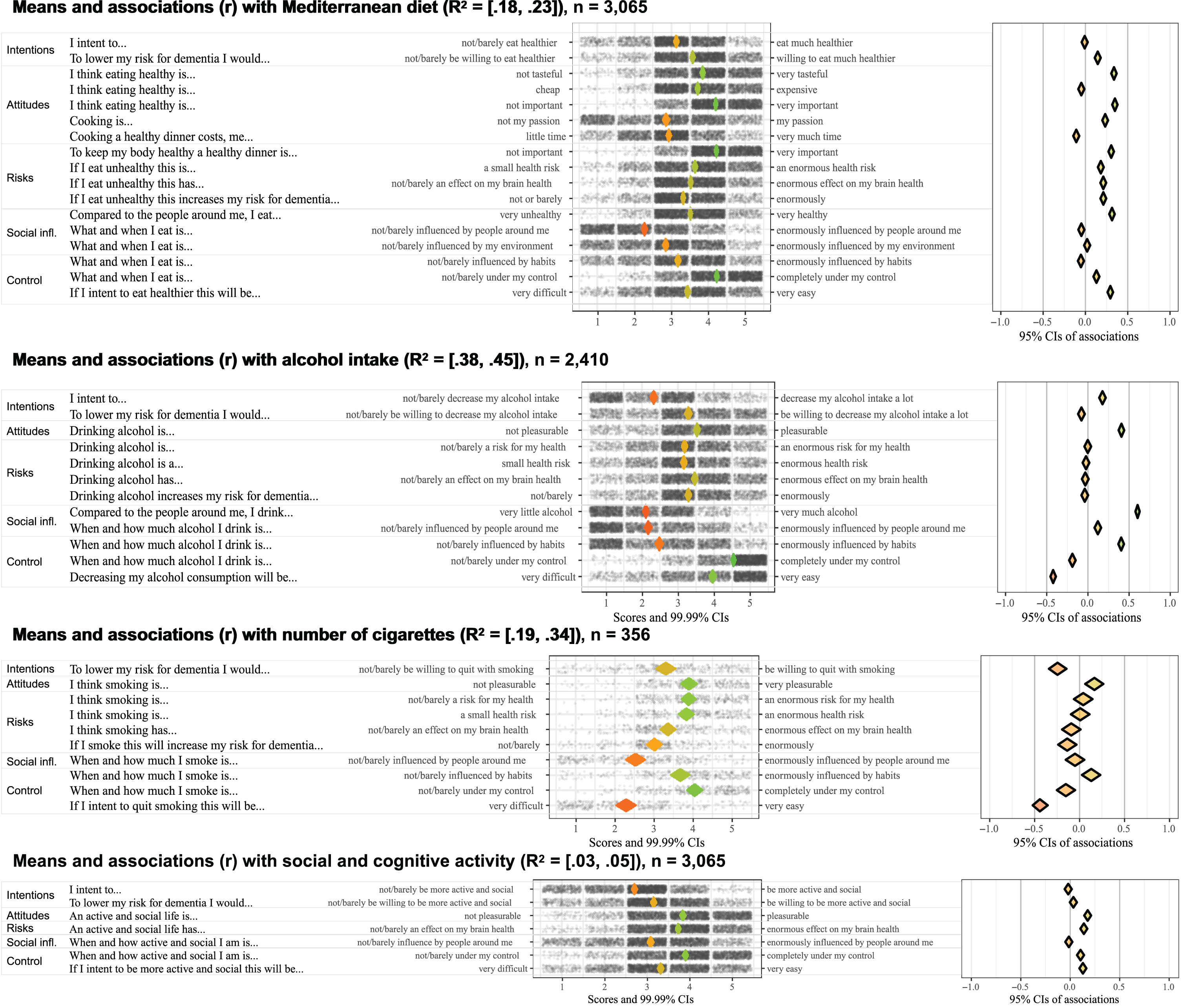
Intentions to change lifestyle behavior
Scoring distributions indicate respondents had moderate intentions to change lifestyle behaviors, with scores averaging around 3 on a 5-point scale (Figs. 1 and 2). Intentions to reduce future dementia risk were slightly higher than general behavior change intentions. Despite this, the weak associations between intentions and outcome behavior suggest restricted relevance.
Attitudes
Distributions and associations highlight the relevance of passion for cooking and pleasure from alcohol or smoking (Figs. 1 and 2). Scores for cooking passion average below 3 on a 5-point scale, indicating room for improvement, which has a moderate positive association with a healthier diet. Scoring distributions for pleasure derived from alcohol or smoking are above 3.5 on a 5-point scale, suggesting an opportunity to reduce these positive attitudes, especially in light of their moderate to strong associations with higher consumption levels.
Risk perceptions
Distributions show respondents had realistic risk perceptions about the negative effects of unhealthy behavior on physical health, brain health, and dementia risk (Figs. 1 and 2). Scores concentrate between 3 and 4 on a 5-point scale, indicating limited room for enhancement of these risk perceptions. Restricted relevance is further supported by weak associations with outcome behaviors.
Social influences
Respondents viewed their diet as relatively healthy compared to others, with scores between 3 and 4 on a 5-point scale (Figs. 1 and 2). This indicates that there is room for improvement, considering that 54.5% of the respondents had a low adherence to the Mediterranean diet. Similarly, there is room to improve accurate perceptions regarding alcohol intake compared to others. Scores ranged mainly between 1 and 3 on a 5-point scale, while 24.5% of the respondents indicated that their alcohol consumption exceeds the recommended level.
Behavioral control
Across behaviors, perceived control was identified as the most relevant determinant (Figs. 1 and 2). Mean scores among physically inactive respondents fall below 3 on a 5-point scale, suggesting room for improvement, while strong associations indicate that more control is associated with higher activity levels. Although respondents felt in control of their eating behavior, they perceived less control over initiating dietary changes, with scores averaging 3.5 on a 5-point scale. Higher perceived control was moderately correlated with a healthier diet, which indicates relevance. Additionally, perceived control over smoking cessation was low, as scores average around 2 on a 5-point scale. This underscores its relevance, especially since higher perceived control towards cessation strongly correlates with less smoking. In contrast to smoking, respondents perceived high control over alcohol intake, suggesting limited room for enhancement.
Explained variance
The CIBER-plots had varying explanatory power (95% CI of R2). The plot for alcohol intake had the highest explanatory power (R2 = 38–45%), followed by smoking (R2 = 19–34%), physical activity (R2 = 20–26%), and diet (R2 = 18–23%). The plot for social and cognitive activities had low explanatory power (R2 = 3% to 5%), with no determinants identified as relevant based on scoring distributions and associations.
DISCUSSION
Key findings
Most of our respondents had room for improvement in lifestyle behavior but were unaware about this room for improvement. Other relevant socio-cognitive determinants identified are attitudes (i.e., limited passion for cooking and deriving pleasure from alcohol or smoking), misperceptions on social comparisons (i.e., overestimating healthy diet intake and underestimating alcohol intake), and low perceived control over behavior change (i.e., being more physically active, diet improvements, and smoking cessation).
Interpretation
Misconceptions about adhering to lifestyle recommendations can stem from insufficient awareness about guidelines, combined with overestimation of healthy behaviors and an underestimation of unhealthy ones. The Protection Motivation Theory provides a framework for understanding how these misconceptions about behavior can reduce the motivation to change [39, 40] by influencing threat appraisal (i.e., perceived susceptibility and severity) [41]. For instance, misconceptions about adhering to recommendations may lead to lower perceived susceptibility, which decreases the inclination to act [40]. This may explain why our respondents had limited intentions towards initiating change, which aligns with other research showing only 32% of the physically inactive individuals, 18% of those not adhering to diet recommendations, and 29% of high alcohol consumers have intentions to change [42].
Our findings further highlight the relevance of perceived behavioral control, a concept akin to self-efficacy [41, 43]. Individuals who feel more in control over their behavior, particularly in overcoming barriers [43], are more inclined to initiate behavior change [44]. However, many of our respondents perceived to have limited control over initiating behavior change, potentially diminishing their intentions. Notably, respondents who drank alcohol perceived high levels of control while underestimating their consumption compared to others. These findings are consistent with earlier indicators that drinkers are often overconfident about their ability to control alcohol intake [45], while they overestimate others’ and underestimate their own consumption levels [46].
Based on the findings, motivating individuals to reduce dementia risk through lifestyle changes should prioritize enhancing accurate perceptions about behavior while strengthening behavioral control. Individual-level interventions could use self-monitoring methods like measuring step count or completing a food diary to decrease misconceptions about the adherence to recommendations. Self-monitoring may already be a steppingstone towards positive lifestyle change, through the mere measurement effect [47, 48]. Providing timely, individualized, non-punitive, and actionable feedback may further encourage healthy lifestyle improvements by making individuals aware of their own behavior and by providing specific cues to action [49, 50]. Goal setting and action planning methods are also well-known methods to enhance perceived behavioral control, preferably by gradually increasing the achievability of certain behavioral changes [30]. Formulating coping responses to overcome specific barriers and cope with difficult situations may further facilitate goal achievement [51]. In earlier qualitative research [13], we observed that low perceived control frequently resulted from earlier failed attempts to change lifestyle behavior. This underscores the importance of providing support to individuals who experience relapse, for example by encouraging them to view it as a part of the behavior change process and use it as an opportunity to learn from the experience [52]. Based on these findings, we strongly advocate for the adoption of specific, evidence-based behavior change methods to support individuals with changing their lifestyles to reduce dementia risk. This requires careful reconsideration of intervention ellements to prioritize methods that are able to target relevant socio-cognitive determinants to increase the likelihood of behavior change. For instance, by emphasizing enjoyable cooking activities, drawing inspiration from successful programs [53] to make participants enhousiastic about creating new eating habits.
While individual-level interventions are important, they must be supplemented by environmental-level strategies to reduce dementia prevalence [54]. Although our sample is only partially representative of the Dutch public, many of our participants had room to improve one or multiple lifestyle behaviors to reduce their future risk of dementia. We observed slightly lower prevalence rates for unhealthy lifestyle behaviors compared to the broader Dutch population [55], indicating that our results might understate the potential for dementia risk reduction in the Netherlands. Given the high prevalence of both unhealthy behavior and dementia, as well as the substantial impact of the environment on behavior, the effectiveness of individual-level interventions alone is questionable [54]. Therefore, we advocate for a multi-faceted approach that combines individual and environmental interventions to facilitate healthy lifestyle behaviors and reduce the risk of dementia at the population level.
Practical applications
The research findings are currently used to shape the development of individual-level behavior change applications that are integrated in the digital LETHE intervention to prevent cognitive decline and dementia [15, 16]. LETHE utilizes a smartphone app and Fitbit smartwatch to monitor lifestyle behavior. One of the features under development is a system to classify participants into weekly adherence pathways (i.e., green, yellow, red). This system would enable lifestyle monitoring to offer personalized digital and in person feedback and support. Furthermore, smartphone functionalities are under development to empower participants to actively monitor a range of lifestyle behaviors. For instance, through a weekly performance score with motivational feedback messages. These examples show how the research findings can guide the development of individual-level applications to support behavior change.
Strengths and limitations
This cross-sectional study employed several measures to enhance credibility, including pre-registration [17] of the protocol to ensure transparency and minimize reporting bias. All materials and data are openly accessible, supporting reproducibility. A key strength of this study is the recruitment through the research agency Flycatcher. Unlike health-related panels, Flycatcher surveys a wide range of topics, including marketing and policy research. This may have helped us to reach a broad audience that is not only interested in participating in health-related research. However, this approach might have introduced selection bias because individuals who volunteer for panels may not accurately reflect the entire population. For instance, individuals with limited digital skills might find it more challanging to participate in an Internet-based panel. Although 80% of the Dutch public has basic digital skills [56] this indicates a substantial part might be excluded from our research.
The study utilized a robust screening process with the validated LIBRA-index, but we relied on unvalidated items to assess social and cognitive activities, which may have affected measurement accuracy that limited the exploratory power. Additionally, future research is needed to explore the determinants of social and cognitive activities more thoroughly and to investigate the impact of environmental factors and demographic differences, particularly socio-economic status, on lifestyle behavior in the context of dementia risk.
Conclusions
Promoting lifestyle changes to reduce dementia risk is complex. Individual-level interventions that encourage lifestyle change should prioritize enhancing accurate perceptions of lifestyle behaviors in comparison to recommendations, while strengthening perceived control over initiating behavioral changes.
AUTHOR CONTRIBUTIONS
Jeroen Bruinsma (Conceptualization; Data curation; Formal analysis; Investigation; Methodology; Visualization; Writing – original draft; Writing – review & editing); Vasileios S. Loukas (Conceptualization; Investigation; Writing – original draft; Writing – review & editing); Thomas Kassiotis (Conceptualization; Methodology); Irene Heger (Conceptualization; Methodology; Writing – review & editing); Anna Rosenberg (Writing – review & editing); Leonie N.C. Visser (Writing – review & editing); Francesca Mangialasche (Writing – review & editing); Dimitrios I. Fotiadis (Funding acquisition; Writing – review & editing); Sten Hanke (Conceptualization; Funding acquisition; Writing – review & editing); Rik Crutzen (Conceptualization; Formal analysis; Funding acquisition; Investigation; Methodology; Supervision; Writing – original draft; Writing – review & editing).
ACKNOWLEDGMENTS
We thank the experts as well as individuals from the Dutch public who consulted us during the development of the questionnaires.
FUNDING
This research is part of the LETHE-project and funded by the European Union’s Horizon 2020 research and innovation program, under the grant agreement with number 101017405.
CONFLICT OF INTEREST
The authors have no conflict of interest to report.
DATA AVAILABILITY
The materials and data supporting the conclusions are available on the Open Science Framework through https://osf.io/eqbgr/.
SUPPLEMENTARY MATERIAL
[1] The supplementary material is available in the electronic version of this article: https://dx.doi.org/10.3233/JAD-231369.
REFERENCES
[1] | Livingston G , Huntley J , Sommerlad A , Ames D , Ballard C , Banerjee S , Brayne C , Burns A , Cohen-Mansfield J , Cooper C , Costafreda SG , Dias A , Fox N , Gitlin LN , Howard R , Kales HC , Kivimäki M , Larson EB , Ogunniyi A , Ritchie K , Rockwood K , Sampson EL , Samus Q , Schneider LS , Selbæk G , Teri L , Mukadam N ((2020) ) Dementia prevention, intervention, and care: 2020 report of the Lancet Commission. Lancet 396: , 413–446. |
[2] | World Health Organization (2019) Risk reduction of cognitive decline and dementia: WHO guidelines. https://apps.who.int/iris/bitstream/handle/10665/312180/9789241550543-eng.pdf, Last updated 2019, Accessed on November 17, 2023. |
[3] | Rohr S , Kivipelto M , Mangialasche F , Ngandu T , Riedel-Heller SG ((2022) ) Multidomain interventions for riskreduction and prevention of cognitive decline and dementia: Current developments. Curr OpinPsychiatry 35: , 285–292. |
[4] | Jia J , Zhao T , Liu Z , Liang Y , Li F , Li Y , Liu W , Li F , Shi S , Zhou C , Yang L , Liao H , Li Y , Zhao H , Zhang J , Zhang K , Kan M , Yang S , Li H , Liu Z , Ma R , Lv J , Wang Y , Yan X , Liang F , Yuan X , Zhang J , Gauthier S , Cummings J ((2023) ) Association between healthy lifestyleand memory decline in older adults: 10 year, population based,prospective cohort study. BMJ 380: , e072691. |
[5] | Bubu OM , Brannick M , Mortimer J , Umasabor-Bubu O , Sebastiao YV , Wen Y , Schwartz S , Borenstein AR , Wu Y , Morgan D , Anderson WM ((2017) ) Sleep, cognitive impairment, and Alzheimer’s disease: A systematicreview and meta-analysis. Sleep 40: , zsw032. |
[6] | Schiepers OJG , Köhler S , Deckers K , Irving K , O’Donnell CA , vanden Akker M , Verhey FRJ , Vos SJB , de Vugt ME , van Boxtel MPJ ((2018) ) Lifestyle for Brain Health (LIBRA): A new model for dementiaprevention. Int J Geriatr Psychiatry 33: , 167–175. |
[7] | Kok G , Gottlieb NH , Peters GJ , Mullen PD , Parcel GS , Ruiter RA , Fernández ME , Markham C , Bartholomew LK ((2016) ) A taxonomy of behaviour change methods: An Intervention Mapping approach. Health Psychol Rev 10: , 297–312. |
[8] | Eldredge LKB , Markham CM , Ruiter RA , Fernandez ME , Kok G , Parcel GS (2019) Planning health promotion programs: An intervention mapping approach, JohnWiley, San Francisco. |
[9] | Fishbein M , Ajzen I (2011) Predicting and changing behavior: The reasoned action approach, Taylor & Francis, New York. |
[10] | Kremers SP , de Bruijn GJ , Visscher TL , van Mechelen W , de Vries NK , Brug J ((2006) ) Environmental influenceson energy balance-related behaviors: A dual-process view. Int J Behav Nutr Phys Act 3: , 9. |
[11] | Hagger MS ((2016) ) Non-conscious processes and dual-process theories in health psychology. HealthPsychol Rev 10: , 375–380. |
[12] | Kim S , Sargent-Cox K , Cherbuin N , Anstey KJ ((2014) ) Development of the motivation to change lifestyle andhealth behaviours for dementia risk reduction scale. Dement Geriatr Cogn Disord Extra 4: , 172–183. |
[13] | Bruinsma J , Heger I , Loukas VS , Kassiotis T , Karanasiou G , Fotiadis DI , Hanke S , Crutzen R ((2023) ) Publicperspectives on lifestyle-related behavior change for dementia risk reduction: An exploratory qualitativestudy in The Netherlands. J Alzheimers Dis 95: , 1635–1642. |
[14] | Crutzen R , Peters GY ((2023) ) A lean method for selecting determinants when developing behavior changeinterventions. Health Psychol Behav Med 11: , 2167719. |
[15] | National Library of Medicine, Digitally Supported Lifestyle Programme to Promote Brain Health Among OlderAdults (LETHE): NCT05565170, https://www.clinicaltrials.gov/study/NCT05565170#contacts, Last updated June 18, 2023, Accessed on November 17, 2023. |
[16] | Hanke S , Mangialasche F , Bödenler M , Neumayer B , Ngandu T , Mecocci P , Untersteiner H , Stögmann E ((2022) ) AI-based predictive modelling of the onset and progression of dementia. Smart Cities 5: , 700–714. |
[17] | Bruinsma J , Loukas V , Karanasiou G , Heger I , Topolski TD , Hanke S , Crutzen R (2023) Lifestyle and dementia risk reduction: Identifying determinants of behavior change in the general public of the Netherlands [pre-registration]. https://doi.org/10.17605/OSF.IO/GB4M5, Last updated September 12, 2022, Last accessed November 17, 2023. |
[18] | Bruinsma J , Loukas V , Karanasiou G , Heger I , Topolski TD , Hanke S , Crutzen R Materials and data belonging to research to identify socio-cognitive determinants of lifestyle behavior in the context of dementia risk reduction [open materials and data]. https://doi.org/10.17605/OSF.IO/EQBGR, Last updated November 23, 2023, Last accessed November 23, 2023. |
[19] | Von Elm E , Altman DG , Egger M , Pocock SJ , Gøtzsche PC , Vandenbroucke JP ((2007) ) The Strengthening theReporting of Observational Studies in Epidemiology (STROBE) statement: Guidelines for reportingobservational studies. Bull World Health Organ 85: , 867–872. |
[20] | Exalto LG , Quesenberry CP , Barnes D , Kivipelto M , Biessels GJ , Whitmer RA ((2014) ) Midlife risk score for theprediction of dementia four decades later. Alzheimers Dement 10: , 562–570. |
[21] | Deckers K , Barbera M , Köhler S , Ngandu T , van Boxtel M , Rusanen M , Laatikainen T , Verhey F , Soininen H , Kivipelto M , Solomon A ((2020) ) Long-term dementia risk prediction by the LIBRA score: A 30-year follow-up ofthe CAIDE study. Int J Geriatr Psychiatry 35: , 195–203. |
[22] | National Institute for Public Health and the Environment (2023). Roken onder volwassenen. https://www.rivm.nl/leefstijlmonitor/roken-onder-volwassenen ,Last updated March 17, 2023, Accessed on November 17, 2023. |
[23] | Moinester M , Gottfried R ((2014) ) Sample size estimation for correlations with pre-specified confidenceinterval. Quant Meth Psych 10: , 124–130. |
[24] | Deckers K , van Boxtel MP , Schiepers OJ , de Vugt M , Munoz Sanchez JL , Anstey KJ , Brayne C , Dartigues JF , Engedal K , Kivipelto M , Ritchie K , Starr JM , Yaffe K , Irving K , Verhey FRJ , Köhler S ((2015) ) Target riskfactors for dementia prevention: A systematic review and Delphi consensus study on the evidence fromobservational studies. Int J Geriatr Psychiatry 30: , 234–246. |
[25] | Peters GY , Crutzen R ((2017) ) Pragmatic nihilism: How a Theory of Nothing can help health psychologyprogress. Health Psychol Rev 11: , 103–121. |
[26] | Peters GJ, Crutzen R ((2024) ) Knowing What We’re Talking About: Facilitating Decentralized, Unequivocal Publication of and Reference to Psychological Construct Definitions and Instructions. Meta-Psychology, 8: . |
[27] | Topolski TD , LoGerfo J , Patrick DL , Williams B , Walwick J , Patrick MMB ((2006) ) The Rapid Assessment ofPhysical Activity (RAPA) among older adults. Prev Chronic Dis 3: , A118. |
[28] | Weggemans RM , Backx FJG , Borghouts L , Chinapaw M , Hopman MTE , Koster A , Kremers S , van Loon LJC , May A , Mosterd A , van der Ploeg HP , Takken T , Visser M , Wendel-Vos GCW , de Geus EJC , Committee Dutch PhysicalActivity Guidelines 2017 ((2018) ) The 2017 Dutch Physical Activity Guidelines. Int J Behav Nutr PhysAct 15: , 58. |
[29] | Garcia-Conesa MT , Philippou E , Pafilas C , Massaro M , Quarta S , Andrade V , Jorge R , Chervenkov M , Ivanova T , Dimitrova D , Maksimova V , Smilkov K , Ackova DG , Miloseva L , Ruskovska T , Deligiannidou GE , Kontogiorgis CA , Pinto P ((2020) ) Exploring the validity of the 14-item Mediterranean Diet Adherence Screener (MEDAS): Across-national study in seven European countries around the Mediterranean region. Nutrients 12: , 2960. |
[30] | Kromhout D , Spaaij CJ , de Goede J , Weggemans RM ((2016) ) The 2015 Dutch food-based dietary guidelines. Eur J Clin Nutr 70: , 869–878. |
[31] | Babor TF, Higgins-Biddle J, Saunders JB, Monteiro MG ((2001) ) AUDIT—the alcohol use disorders identification test: Guidelines for use in primary care. World Health Organzation, Geneva. |
[32] | Berner MM , Kriston L , Bentele M , Härter M ((2007) ) The alcohol use disorders identification test fordetecting at-risk drinking: A systematic review and meta-analysis. J Stud Alcohol Drugs 68: , 461–473. |
[33] | Deckers K , Kohler S , Ngandu T , Antikainen R , Laatikainen T , Soininen H , Strandberg T , Verhey T , Kivipelto M , Solomon A ((2021) ) Quantifyingdementia prevention potential in the FINGER randomized controlledtrial using the LIBRA prevention index. Alzheimers Dement 17: , 1205–12. |
[34] | Ngandu T , Lehtisalo J , Korkki S , Solomon A , Coley N , Antikainen R , Bäckman L , Hänninen T , Lindström J , Laatikainen T , Paajanen T , Havulinna S , Peltonen M , Neely AS , Strandberg T , Tuomilehto J , Soininen H , Kivipelto M , ((2022) ) The effect of adherence on cognition in a multidomain lifestyle intervention(FINGER). Alzheimers Dement 18: , 1325–1334. |
[35] | Heger I , Deckers K , de Vugt M , Verhey F , Oenema A , van Boxtel Köhler M.S ((2023) ) Using mHealth forprimary prevention of dementia: A proof-of-concept study on usage patterns, appreciation, and beliefs andattitudes regarding prevention. J Alzheimers Dis 94: , 938–948. |
[36] | Crutzen R , Peters GY , Noijen J ((2017) ) Using confidence interval-based estimation of relevance to selectsocial-cognitive determinants for behavior change interventions. Front Public Health 5: , 165. |
[37] | Crutzen R , Peters GY ((2023) ) The regression trap: Why regression analyses are not suitable for selectingdeterminants to target in behavior change interventions. Health Psychol Behav Med 11: , 2268684. |
[38] | Crutzen R , Peters GY ((2019) ) (2019) The Book of Behavior Change. 1st Ed. 2019.https://zenodo.org/records/3570967, Last updated May 9, 2019, Accessed on November 17, 2023. |
[39] | Jansink R , Braspenning J , Keizer E , van der Weijden T , Elwyn G , Grol R ((2012) ) Misperception of patientswith type 2 diabetes about diet and physical activity, and its effects on readiness to change. JDiabetes 4: , 417–423. |
[40] | Norcross JC , Krebs PM , Prochaska JO ((2011) ) Stages of change. J Clin Psychol 67: , 143–154. |
[41] | Norman P , Boer H , Seydel ER , Mullan B (2015) Protection motivation theory. Predicting and changing health behaviour: Research and practice with social cognition models, Open University Press, Berkshire. |
[42] | Vrijsen J , Matulessij TF , Joxhorst T , de Rooij SE , Smidt N ((2021) ) Knowledge, health beliefs and attitudestowards dementia and dementia risk reduction among the Dutch general population: A cross-sectional study. BMC Public Health 21: , 857. |
[43] | Ajzen I ((2020) ) The theory of planned behavior: Frequently asked questions. Hum Behav Emerg Technol 2: , 314–324. |
[44] | Hagger MS , Cheung MW , Ajzen I , Hamilton K ((2022) ) Perceived behavioral control moderating effects in thetheory of planned behavior: A meta-analysis. Health Psychol 41: , 155–67. |
[45] | Caliendo M , Hennecke J ((2022) ) Drinking is different! Examining the role of locus of control for alcoholconsumption. Empir Econ 63: , 2785–2815. |
[46] | Gual A , Angel Arbesu J , Zarco J , Balcells-Olivero MLM , Lopez-Pelayo H , Miquel L , Bobes J ((2017) ) Riskydrinkers underestimate their own alcohol consumption. Alcohol 52: , 516–517. |
[47] | Broekaerts M , Pintrich PR , Zeidner M (2000) Handbook of self-regulation, Academic Press, San Diego. |
[48] | Morwitz VG , Johnson E , Schmittlein D ((1993) ) Does measuring intent change behavior? J Consum Res 20: , 46–61. |
[49] | Hysong SJ , Best RG , Pugh JA ((2006) ) Audit and feedback and clinical practice guideline adherence: Makingfeedback actionable. Implement Sci 1: , 9. |
[50] | Larson EL , Patel SJ , Evans D , Saiman L ((2013) ) Feedback as a strategy to change behaviour: The devil is inthe details. J Eval Clin Pract 19: , 230–234. |
[51] | Kwasnicka D , Dombrowski SU , White M , Sniehotta F ((2016) ) Theoretical explanations for maintenance ofbehaviour change: A systematic review of behaviour theories. Health Psychol Rev 10: , 277–926. |
[52] | Graham S ((2020) ) An attributional theory of motivation. Contemp Educ Psychol 61: , 101861. |
[53] | Farmer N , Touchton-Leonard K , Ross A ((2019) ) Psychosocial benefits of cooking interventions: A systematicreview. Health Edu Behav 45: , 167–80. |
[54] | Walsh S , Govia I , Wallace L , Richard E , Peters R , Anstey KJ , Brayne C ((2022) ) A whole-population approach is required for dementia riskreduction. Lancet Healthy Longev 3: , e6–e8. |
[55] | Kloosterman R , Akkermans R , Reep C , Tummers –van der Aa M (2022) (On)gezonde leefstijl 2022: Opvattingen, motieven en gedragingen. https://www.cbs.nl/nl-nl/longread/rapportages/2023/on–gezonde-leefstijl-2022-opvattingen-motieven-en-gedragingen, Last updated April 20 9, 2023, Accessed on November 17, 2023. |
[56] | Statistics Netherlands (2023) Digital proficiency continues to rise.https://www.cbs.nl/en-gb/news/2023/45/digital-proficiency-continues-to-rise ,Last updated November 13, 2023, Accessed on November 17, 2023. |