Reduced Prefrontal-Thalamic Theta Flow During Working Memory Retrieval in APP/PS1 Mice
Abstract
Background:
Working memory deficits in Alzheimer’s disease (AD) are linked to impairments in the retrieval of stored memory information. However, research on the mechanism of impaired working memory retrieval in Alzheimer’s disease is still lacking.
Objective:
The medial prefrontal cortex (mPFC) and mediodorsal thalamus (MD) are involved in memory retrieval. The purpose of this study is to investigate the functional interactions and information transmission between mPFC and MD in the AD model.
Methods:
We recorded local field potentials from mPFC and MD while the mice (APP/PS1 transgenic model and control) performed a T-maze spatial working memory task. The temporal dynamics of oscillatory activity and bidirectional information flow between mPFC and MD were assessed during the task phases.
Results:
We mainly found a significant decrease in theta flow from mPFC to MD in APP/PS1 mice during retrieval.
Conclusions:
Our results indicate an important role of the mPFC-MD input for retrieval and the disrupted information transfer from mPFC to MD may be the underlying mechanism of working memory deficits in APP/PS1 mice.
INTRODUCTION
Alzheimer’s disease (AD) is a common neurodegenerative disease characterized by progressive memory loss and subsequent loss of broader cognitive functions [1, 2]. Working memory refers to a system for the temporary holding and manipulation of information that is vital for a range of cognitive tasks, such as learning, comprehension, and reasoning [3–5]. Deficits in working memory have been frequently discussed in AD-related studies [6, 7].
In particular, studies in rodents have focused on spatial working memory, typically tested using maze-based tasks [4, 6, 7]. The spatial working memory tasks generally involve three distinct phases: encoding, maintenance, and retrieval [3–5]. For instance, in the ‘delayed non-match-to-sample’ task, animals are first required to visit a specific spatial location in a T-maze during the sample phase, which involves information encoding. Subsequently, the animals must maintain a memory trace of the previously visited position during the delay phase. Finally, the animals have to visit the locations that were not visited to get a reward during the retrieval phase. Substantial evidence suggests impairments in the retrieval of stored memory information in patients with AD or animal models [1, 8–10].
Accumulating evidence has indicated that the prefrontal cortex (PFC) plays a major role in working memory [4, 11, 12]. Dysfunction of the PFC is associated with working memory deficits [13, 14]. Optogenetic inhibition of prefrontal pyramidal neurons impaired the mice’s performance during a spatial working memory task [4]. In the mouse models of AD, medial PFC (mPFC) is one of the earliest brain areas to generate pathognomonic features such as amyloid-β (Aβ) plaques [15]. In addition, the neuronal activity in mPFC exhibited deficits in a mice model of AD [16]. Therefore, PFC is regarded as a key substrate for cognitive decline in AD [15, 17, 18].
Besides, the PFC function cannot be separated from its closely linked thalamic partners. As a higher-order thalamic relay of the PFC, the mediodorsal thalamus (MD) is considered to support the transfer of information across the PFC [4, 19, 20]. Furthermore, MD lesions affect PFC-dependent aspects of spatial working memory [21]. In AD patients, functional magnetic resonance imaging findings revealed significant atrophy of thalamic structures [22].
Moreover, the functional connections between mPFC and MD were found to be related to working memory [23]. A study on healthy mice revealed the directional interaction from mPFC to MD in retrieval using both pathway-specific optogenetic inhibition experiments and directionality analyses of multisite recordings [5]. Our previous study also reported an increased mPFC-MD flow accompanying successful memory retrieval [24]. So far, it is still unclear whether the impaired working memory in AD is related to the disrupted functional connectivity and information transmission in the mPFC-MD circuit.
Recently, brain networks have gained popularity due to their ability to reflect cognitive processes in the brain [25–28]. The network analysis approaches (such as Granger causality and directed transfer function) can be used to estimate directed connectivity across different brain regions and to investigate fundamental mechanisms of neurological and mental conditions, and may even be predictive of further explanations of the pathogenesis of neurodegenerative diseases [29–31]. Therefore, in this study, we recorded local field potentials (LFPs) from mPFC and MD while the mice (APP/PS1 transgenic model and control) performed a T-maze spatial working memory task. The bidirectional information flow between mPFC and MD was assessed by using the directed transfer function (DTF) method during the distinct phases. It is expected to yield new insights into the abnormal information transmission in the prefrontal-thalamic circuit which may induce the working memory deficits in the APP/PS1 model of AD.
MATERIAL AND METHODS
Subjects
Male APP/PS1 mice (aged 6–9 months) and adult C57BL/6 wild-type mice (Experimental Animal Center of Tianjin Medical University, Tianjin, China) were used in this study. The APP/PS1 mice overexpress the Swedish mutation of amyloid precursor protein (APP), in combination with the delta exon 9 variant of presenilin 1 (PS1; also known as PSEN1). The mutations in both genes are associated with familial AD [32]. Therefore, the APP/PS1 mouse model is commonly used to study the mechanisms of neuropathology of AD and evaluate potential therapeutic interventions. All mice were group housed (4–5/cage) in 12 : 12 h light-dark cycles and allowed access to food and water ad libitum until the experiment began. The experimental procedures were approved by the Animal Care and Use Committee of Tianjin Medical University (License number: TMUaMEC 2021060).
Delayed non-match-to-place task
Animals were trained on a T-maze delayed non-match-to-place (DNMTP) task, as described previously [5, 33]. During the task period, all mice were kept on food restriction to limit their weights to 85% of free-feeding weight [33, 34]. Mice were then given a 2-day habituation to the T-maze (including free exploration and foraging for food rewards). In the following two days, mice experienced behavioral shaping that involved running in alternate directions to baited goal arms for 10 minutes. Afterward, mice were subjected to the T-maze training until they met the performance criteria (70% of their trials correctly on two out of three consecutive days) [4, 31]. Figure 1A shows the schematic diagram of a single trial of the DNMTP task. The maze consisted of three arms (one center arm and two goal arms), each 30 cm long, 8 cm broad, and 20 cm high. Each trial of the task consisted of a sample, delay, and choice phase. In the sample phase, one side of the arms was blocked so that mice could only enter the open arm to get a food reward. Then the mice would be delayed in the start box for 10 s after turning back. In the choice phase, the mouse was required to enter the arm opposite to that visited during the sample phase to receive a reward. The inter-trial latency period in this experiment was 20 s [33]. Response accuracy served as a gauge of the mice’s performance during training sessions (Fig. 1B).
Fig. 1
Experiment setup. A) Experimental paradigm for conducting a single trial of the DNMTP task in T-maze. Each trial comprised a sample phase and a choice phase separated by a 10-s delay. B) Accuracy was indicated by the percentage of correct trials (n = 8 mice in each group). C) Trial durations varied with training days (n = 8 mice in each group). D) Schematic of simultaneous multi-channel microelectrodes in vivo recordings. E) Typical LFP tracings from mPFC (orange) and MD (green). F) Histological verification of the recording sites in mPFC (PrL region, left) and MD (right). Locations of recording sites are indicated by rectangles. Scale bar, 500μm. Data are represented as mean±SEM.
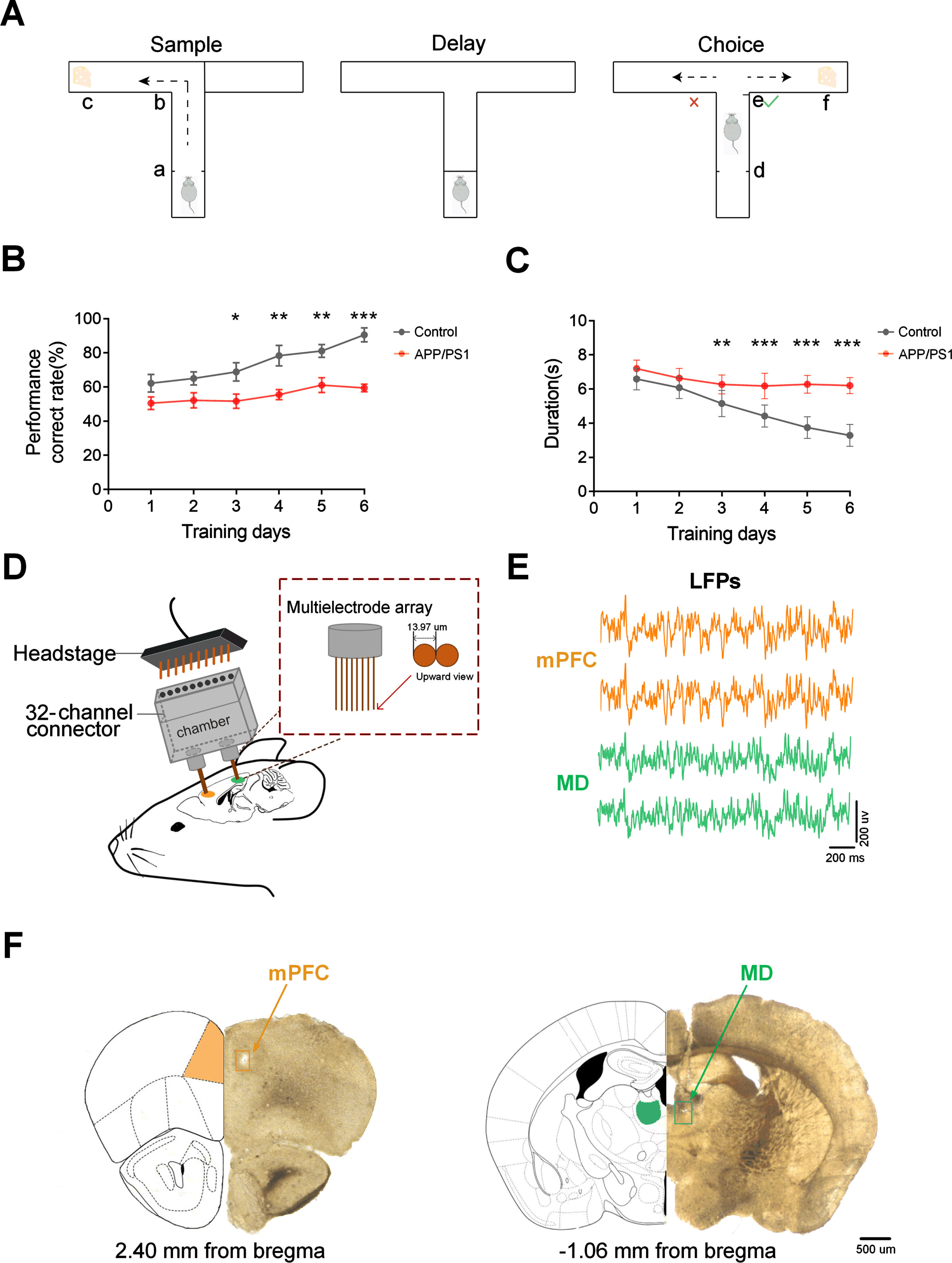
Electrodes implantation and neurophysiological recording
We used 32-channel electrodes made from 13-μm tungsten fine wire and less than 0.5 MΩ impedance for extracellular recording. The skull screws served as ground and reference [8]. After inducing profound anesthesia, the dura over the implanted brain regions of mice were removed gingerly. Electrodes were implanted independently in mPFC (AP: 2.4 mm; ML: 0.4 mm; DV: -1.5 mm) and MD (AP: -1.06 mm; ML: 0.35 mm; DV: -3.2 mm) and were secured to the skull with two jewelry screws, medical glue and dental cement [8]. After implantation, all mice were caged individually to prevent electrodes from being destroyed by cagemates.
After a week of recovery, neurophysiological recordings were made from mPFC and MD while the mice underwent the spatial working memory task (Fig. 1D). Extracellular signals were recorded with the Cerebus Acquisition System (Blackrock Microsystems Inc., UT, USA). For LFPs, the signals were amplified, bandpass-filtered (0.5-120 Hz), and digitized at 1 kHz. The behavioral data were simultaneously recorded by using a settled camera. Figure 1E shows the example of LFP tracings from mPFC and MD.
Histology
At the end of the experimentation, PBS and 4% PFA were transcardially infused into mice. Next, fixed tissues were sliced into 30μm sections with microtome cryostat and then placed on slides for viewing and photographing electrode insertion sites [35]. The brain images were then superimposed on a typical drawing extracted from the Rat Brain in Stereotaxic Coordinates. The locations of the mPFC and MD recordings were verified (Fig. 1F). We chose the data from the mice with successful electrode sites for subsequent analysis.
LFP power spectrogram
Time-frequency spectral analysis was applied to determine the dominant frequencies during the task. Spectral analysis of oscillatory activity was calculated using the short-Fourier transform (500-ms Hamming window, 125-ms steps). Then the different frequency bands of LFPs were obtained by the Chebyshev band-pass filters. In this study, we converted the band power to z-scores by subtracting the mean and dividing by the standard deviation for each animal.
Quantification of information transfer between mPFC and MD
We measured the bidirectional information flow between mPFC and MD based on the DTF, as described previously [24]. The DTF method was proposed based on the Granger frequency domain causal analysis and was known as a statistical method for inferring causality in time series [26, 36]. We transformed LFPs into multivariate autoregressive (MVAR) as follows [37]:
(1)
X (t) stands for N channels of LFPs, p is the model order that the Bayesian information criterion counts. Cn is the N * N coefficient matrix. The vector of multivariate zero mean uncorrelated white noise at time t is denoted as W (t).
(2)
(3)
H (f) is the matrix of the transfer function following the Fourier transform, and C (0) = - I, I is an identity matrix. H (f), as an asymmetric matrix, can distinguish the direction of information transfer between the two channels.
(4)
(5)
(6)
Statistical tests
All data were analyzed using built-in functions or custom code in Matlab. The statistical analyses were performed using GraphPad Prism 9. Two-way repeated measures ANOVA followed by Bonferroni test for multiple comparisons was used to compare the behavioral results in the inter-group. One-way ANOVA followed by Bonferroni test for multiple comparisons was applied to compare the power and information flow among the different frequencies in the intra-group. Mann-Whitney test followed by a post hoc two-tailed t-test was used to test the statistical differences between APP/PS1 and control mice. All data are expressed as means±SEM. Differences were considered statistically significant when p < 0.05.
RESULTS
Working memory deficits in APP/PS1 mice
In this study, we used a T-maze DNMTP task to test spatial working memory. The behavioral results showed that the correct rate of control mice gradually rose with the training days while the APP/PS1 mice maintained a relatively stable accuracy rate (Fig. 1B; control: from 62.22% ±5.21% to 90.56% ±4.12%, APP/PS1: from 50.56% ±3.68% to 59.44% ±2.27%; two-way repeated measures ANOVA followed by Bonferroni’s test, F (1,96) = 60.21, p < 0.0001: p < 0.05 for APP/PS1 versus control group).
From the third day of training, the APP/PS1 mice showed obviously lower correct rates than the control mice. Besides, we also found that both groups of mice took less time to obtain rewards. Compared to the APP/PS1 mice, the control mice shortened the time more rapidly during the choice phase as the training days increased (Fig. 1C; control: from 6.59±0.22 s to 3.30±0.23 s, APP/PS1: from 7.20±0.18 s to 6.20±0.17 s; two-way repeated measures ANOVA followed by Bonferroni’s test, F (1,84) = 158.6, p < 0.0001: p < 0.05 for control group versus APP/PS1 group). These results indicate the impaired spatial working memory in APP/PS1 mice.
Decreased theta oscillatory activity in mPFC and MD during retrieval in APP/PS1 mice
We observed the LFP activities from mPFC and MD for the APP/PS1 and control mice (n = 8 mice in each group) as the mice performed the DNMTP task. In total, we analyzed 415 trials (207 trials from APP/PS1 mice and 208 trials from control mice) in the present paper.
To examine the dominant frequencies during retrieval, we first calculated a time-frequency map of the LFP power spectra for each site (Fig. 2A, B, and Supplementary Figure 1). We further compared the power spectral density in different frequency bands (Fig. 2C, D, and Supplementary Figure 2). The statistical results revealed that the theta range is the dominant frequency among all the frequency bands in both APP/PS1 and control mice.
Fig. 2
Oscillatory dynamics in mPFC and MD during the choice phase. A) Time-frequency power spectrum of mPFC and MD during the choice phase for a control mouse. B) Same as A, but for an APP/PS1 mouse. C) Comparison of power among different frequencies in mPFC and MD for the control mice (n = 8 mice; mPFC: one-way ANOVA followed by Bonferroni’s test, F (3,56) = 7.345, p = 0.0003; MD: one-way ANOVA followed by Bonferroni’s test, F (3,52) = 7.07, p = 0.0004). D) same as (C), but for the APP/PS1 mice (n = 8 mice; mPFC: one-way ANOVA followed by Bonferroni’s test, F (3,64) = 5.731, p = 0.0015; MD: one-way ANOVA followed by Bonferroni’s test, F (3,60) = 15.71, p < 0.0001). E) Left: Average power spectral density (z-score) in mPFC during retrieval; Right: Comparison of peak theta power in mPFC (n = 8 mice per group, left: control: one-way ANOVA followed by Bonferroni’s test, F (59,7867) = 170.1, p < 0.0001, APP/PS1: one-way ANOVA followed by Bonferroni’s test, F (59,8680) = 117.9, p < 0.0001. Right: **p < 0.01 by Mann-Whitney test). F) Same as E, but for MD (n = 8 mice per group; Left: Control: one-way ANOVA followed by Bonferroni’s test, F (59,7333) = 57.86, p < 0.0001, APP/PS1: one-way ANOVA followed by Bonferroni’s test, F (59,8598) = 37.42, p < 0.0001. Right: Mann-Whitney test, ns, not significant). G) Schematics illustrate the time when the theta power peaked and ‘Δt’ is defined as the interval between the peak time in mPFC and MD. Left: control, right: APP/PS1. H) Comparison of normalized Δt from (G) (n = 8 mice per group; Mann-Whitney test, ***p < 0.001). Data are represented as mean±SEM.
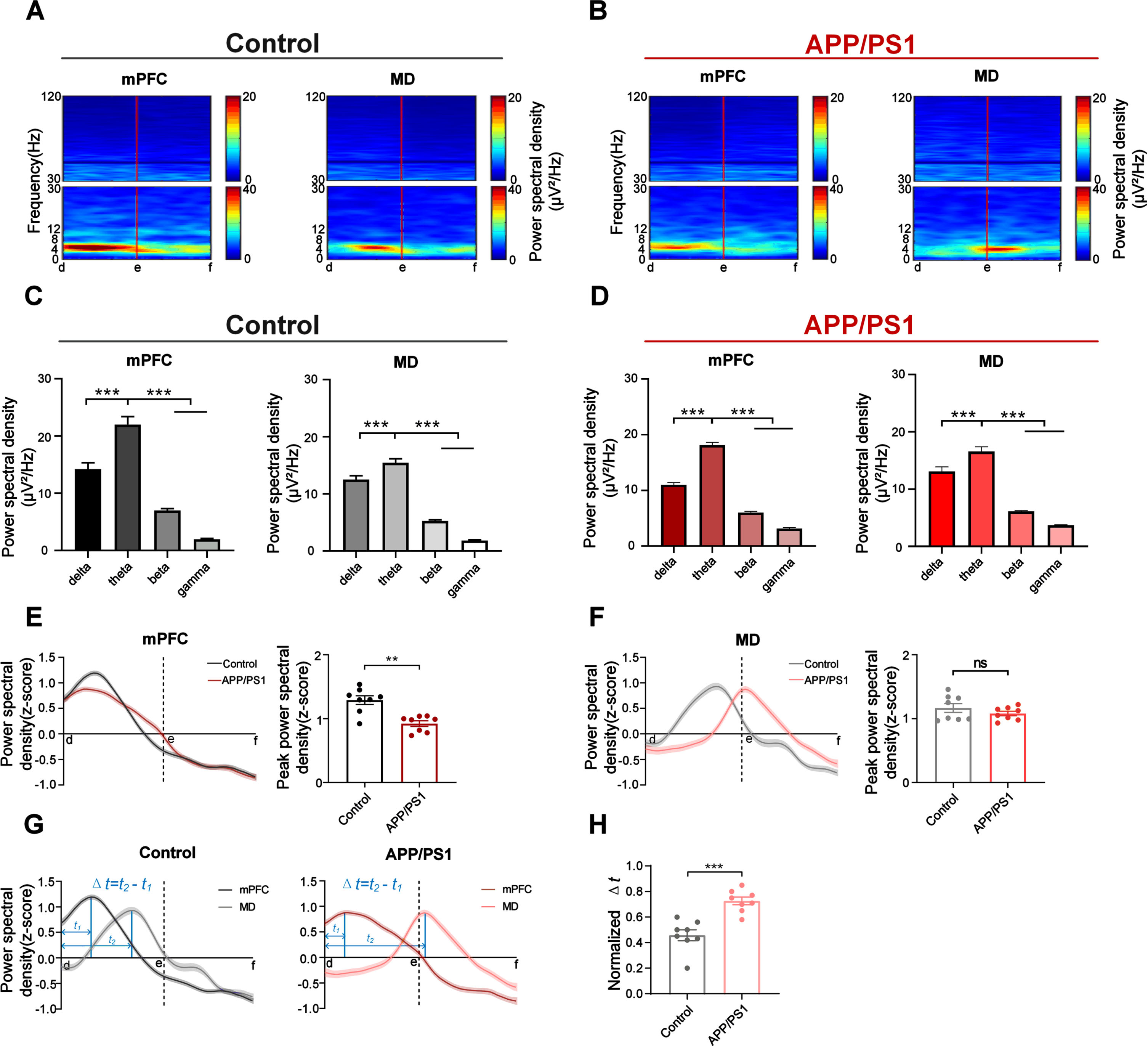
We further normalized the power (z-score) to exclude the effects of electrode impedance and mouse individual differences. For mPFC, the theta power in control mice showed a noticeable increase prior to the choice point (point ‘e’), which was significantly higher than that in APP/PS1 mice (Fig. 2E; right: control: 0.92±0.044, APP/PS1 : 1.29±0.068; Mann-Whitney test, p = 0.0030). For MD, the theta power showed no statistical difference between the two groups (Fig. 2F; right: control: 1.08±0.036, APP/PS1 : 1.17±0.070; Mann-Whitney test, p = 0.5054).
Notably, the theta power peaked in mPFC before that in MD in both groups (Fig. 2G and Supplementary Figure 3). The intervals in APP/PS1 mice were statistically longer than those in control mice (Fig. 2H; control: 0.46±0.043, APP/PS1 : 0.73±0.030; Mann-Whitney test, p = 0.0003). These results may suggest the prefrontal priming signature during retrieval and potential information transmission from mPFC to MD.
Fig. 3
Reduced theta flow from mPFC to MD during retrieval in APP/PS1 mice. A) Average information flow from mPFC to MD as a function of frequency during the choice phase (n = 8 mice per group; control: one-way ANOVA followed by Bonferroni’s test, F (119,13756) = 6.735, p < 0.0001; APP/PS1: one-way ANOVA followed by Bonferroni’s test, F (119,12822) = 6.927, p < 0.0001). B) Information flow from mPFC to MD across the different frequency bands in control mice (n = 8 mice; one-way ANOVA followed by Bonferroni’s test, F (4,504) = 82.71, p < 0.0001). C) Same as B, but for APP/PS1 mice (n = 8 mice; one-way ANOVA followed by Bonferroni’s test, F (4,472) = 82.94, p < 0.0001). D) Theta flow from mPFC to MD as a function of spatial positions in the T-maze (n = 8 mice per group, gray: one-way ANOVA followed by Bonferroni’s test, F (21,4554) = 3.189, p < 0.0001; red: one-way ANOVA followed by Bonferroni’s test, F (21,4576) = 5.551, p < 0.0001). E) Comparison of the average theta flow between APP/PS1 and control mice during the choice phase (n = 8 mice per group; ***p < 0.001 by Mann-Whitney test). F) Theta flow showed a relatively smaller increase in APP/PS1 mice (ΔIFmPFC ⟶MD is defined as the peak value of theta flow (the maximum of theta flow during the choice period: d ⟶e) minus the initial value of theta flow (at location ‘d’)) (n = 8 mice; *p < 0.05 by Mann-Whitney test). G) Shuffled theta flow from mPFC to MD during the choice phase (n = 8 mice per group; control: one-way ANOVA followed by Bonferroni’s test, F (21,3183) = 0.4554, p = 0.9839; APP/PS1: one-way ANOVA followed by Bonferroni’s test, F (21,1915) = 0.7947, p = 0.7292). H) The shuffled data showed no statistically significant difference between the two groups (n = 8 mice per group; ns, not significant by Mann-Whitney test). I) The changes in the theta flow are independent of animals’ running speed (n = 8 mice; ns, not significant by Mann-Whitney test). Data are represented as mean±SEM.
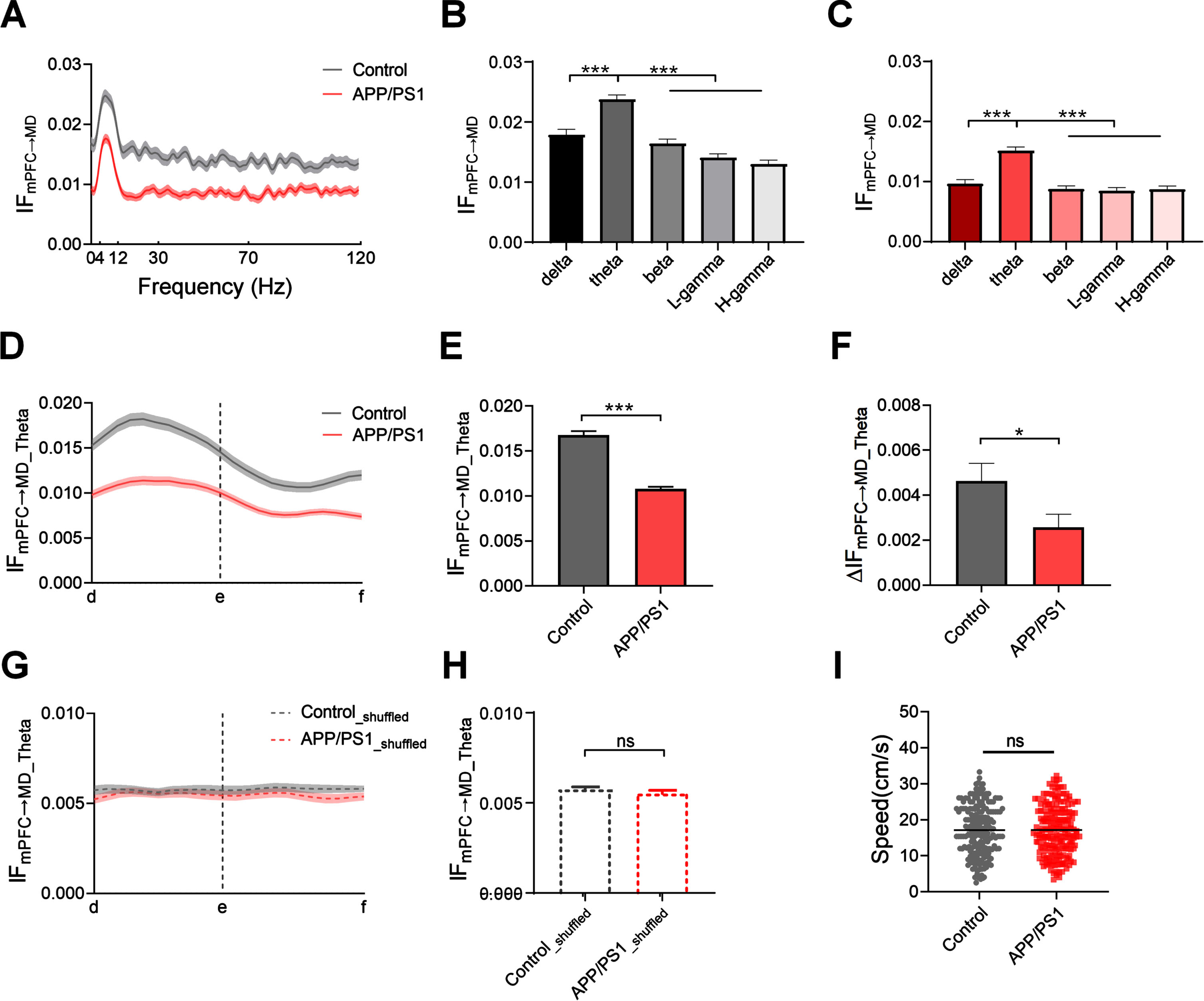
Reduced theta flow from mPFC to MD during retrieval in APP/PS1 mice
To gain more insight into directional functional interaction during retrieval, we quantified the information flow from mPFC to MD using the DTF method. We plotted the curve of information flow as a function of frequency to determine the dominant frequency during the choice phase. The results showed that the theta flow from mPFC to MD in APP/PS1 mice was significantly lower than that in control mice (Fig. 3 and Supplementary Figure 4A). Moreover, the theta flow from mPFC to MD was significantly higher than those in other frequency bands in both control (Fig. 3B and Supplementary Figure 4) and APP/PS1 mice (Fig. 3C and Supplementary Figure 4).
Fig. 4
Directionality of the theta flow in APP/PS1 and control mice during retrieval. A) Bidirectional theta flow between mPFC and MD across the control mice during the choice phase on the correctly performed trials. Left: the gray and green curves represent the theta flow from mPFC to MD and from MD to mPFC, respectively (n = 8 mice; left: gray: one-way ANOVA followed by Bonferroni’s test, F (21,4554) = 3.189, p < 0.0001, green: one-way ANOVA followed by Bonferroni’s test, F (21,3014) = 1.035, p = 0.4154). Right: the theta flow from mPFC to MD was significantly higher than the reverse (n = 8 mice; ***p < 0.001 by Mann-Whitney test). B) Same as (A), but for the APP/PS1 mice (n = 8 mice; left: red: one-way ANOVA followed by Bonferroni’s test, F (21,4576) = 5.551, p < 0.0001; blue: one-way ANOVA followed by Bonferroni’s test, F (21,4400) = 1.137, p = 0.2993, right: ***p < 0.001 by Mann-Whitney test).
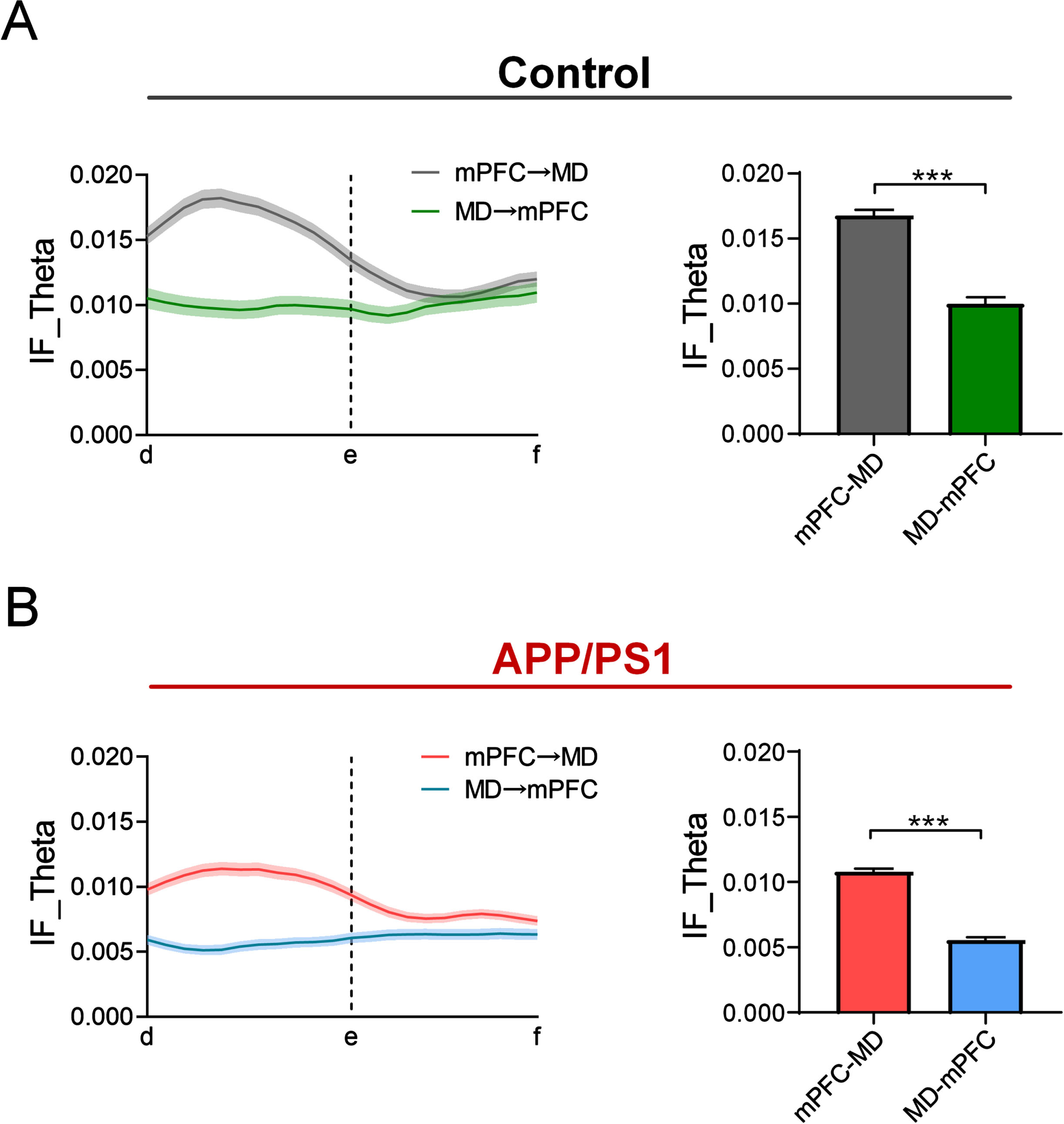
Given the prominent theta flow, we quantified the theta flow as a function of position in the T-maze to examine the patterns of theta flow during the choice phase. The results showed that the theta flow from mPFC to MD rose and peaked before the turning in both groups (Fig. 3D). We further compared the theta flow from mPFC to MD between the two groups. Prior to point ‘e’, which showed that the flow in APP/PS1 mice was significantly lower than that in control mice (Fig. 3E; control: 0.017±0.0004, APP/PS1 : 0.011±0.0002; Mann-Whitney test, p < 0.0001). Moreover, the theta flow in APP/PS1 mice showed a relatively lower increment across the choice phase (Fig. 3F; control: 0.0046±0.0008, APP/PS1 : 0.0025±0.0006; Mann-Whitney test, p = 0.0407). We also examined the temporal patterns of the theta flow with those obtained from shuffled data, demonstrating that theta flow during the choice phase does not simply reflect random fluctuations in activity (Fig. 3G and 3 H; control: 0.0057±0.0002, APP/PS1 : 0.0055±0.0002; Mann-Whitney test, p = 0.7245). To exclude the differences in locomotor ability, we tested the running speed of the APP/PS1 and control mice. The results showed that the elevated theta flow was not caused by the motor function, as no difference in the animals’ running speed was found between the two groups (Fig. 3I; control: 17.16±0.50; APP/PS1 : 17.18±0.49, Mann-Whitney test, p = 0.9060).
To further determine the directionality of theta flow during retrieval, we also calculated the information flow from MD to mPFC during the choice phase. For control mice, the theta flow from mPFC to MD displayed a noticeable increase before the choice points. By contrast, no changes in theta flow were observed during the choice phase (Fig. 4A left and Supplementary Figure 5).
The statistical results revealed that the theta flow from mPFC to MD was noticeably higher than that from MD to mPFC (Fig. 4A right: mPFC to MD: 0.017±0.0004, MD to mPFC: 0.0099±0.0005; Mann-Whitney test, p < 0.0001). Compared to the controls, the APP/PS1 mice showed a relatively smaller increase in the theta flow from mPFC to MD (Fig. 4B left and Supplementary Figure 5) and it is still stronger than that from MD to mPFC (Fig. 4B right: mPFC to MD: 0.011±0.0002, MD to mPFC: 0.0055±0.0002; Mann-Whitney test, p < 0.0001). These results suggest an important role of the elevated theta flow from mPFC to MD for retrieval and the working memory deficits in APP/PS1 mice are linked with the disrupted information flow in the mPFC-MD circuit.
DISCUSSION
We mainly report a decreased theta flow from mPFC to MD during working memory retrieval in the APP/PS1 mouse model of AD. Our results provide evidence that directional frequency-specific communication in the prefrontal-thalamic pathway plays an important role in spatial working memory. The disrupted information transfer from mPFC to MD may be the underlying mechanism of working memory deficits in the APP/PS1 model of AD.
The pathogenesis of AD is highly complex and has attracted the interest of many scholars. Previous studies have suggested the pathology of AD includes primarily amyloid plaques, tau tangles, neuronal and synaptic loss, and so on [39]. In particular, Aβ peptide precipitation is an early event in the onset of AD [40] and has been regarded as the disease’s leading contributing factor according to the amyloid cascade hypothesis [41]. Nevertheless, amyloid deposition is associated with functional disruption of the default network after excluding late-stage effects such as cognitive impairments but ignoring early effects [42, 43].
Furthermore, the functional deficits in various APP overexpressing mouse lines were detected before the early plaque formation stage, although there is only a slight decrease [40, 44]. Studies using functional magnetic resonance imaging and positron emission tomography suggested that the functional connectivity in the resting state declined in AD patients, which had no direct relationship to amyloid plaques [45, 46]. This fact raised a possibility that fibrillar Aβ in plaques does not directly contribute to the functional deficits in AD. Instead, soluble Aβ species (i.e., monomeric, oligomeric, and protofibrillary Aβ species that linger in aqueous solution after high-speed centrifugation), which appear before plaque and ultimately gather to form plaques, seem to be the primary culprit of the functional connectivity impairment in AD [47]. In particular, comparisons of transgenic lines of mice with various APP expressions showed that the reduction in presynaptic terminals was strongly reliant on soluble Aβ levels in the cortex and occurred earlier than plaque deposition [48, 49]. Coinciding with this fact, there is a point that soluble forms of starch can exert toxic effects at the synaptic level, altering synaptic transmission and ultimately leading to impaired function [44, 47].
Several electrophysiological studies have also released smaller excitatory postsynaptic potentials and concurrent decay of long-term potentiation (an electrophysiological correlate of synaptic plasticity) for the AD transgenic model than nontransgenic mice and the functional synaptic rather than structural changes are responsible for the impaired performance on spatial working memory tasks in aged transgenic mice [45, 50–52]. Severe disruption in long-range axonal connectivity was found using intravital calcium and voltage imaging in the AD model mice. The damage was related to the action potential transmission block. The block in neurons may lead to behavioral state-associated LFP oscillations delivery block among the brain regions [53]. Therefore, the abnormal synaptic transmission with the action potential transmission block may be the possible reason for the disrupted functional connectivity from mPFC to MD in the APP/PS1 model.
The brain generates rhythmic oscillations to support information processing in cognitive functions. The fluctuations in theta frequency have long been linked to memory. Increased theta oscillations are associated with successful memory [54]. Aberrant theta rhythms underlie working memory deficits in AD patients [55]. Our results are consistent with those reported in the literature. In addition, recent studies have proposed that gamma oscillations play an irreplaceable role in information processing [56, 57]. Dampened gamma activities were also found in AD patients [58]. Electrophysiological studies of AD-related alteration in mouse models also yielded encouraging findings of improved AD pathogenesis through invasive and non-invasive brain stimulation in the gamma range [59–61]. Moreover, theta-gamma cross-frequency coupling may serve as a key participant in memory processing [62, 63]. Reduced theta-gamma coupling may serve as a biomarker for early AD-related changes in brain dynamics [62].
Clinically, AD is classified into two subtypes: early onset (EOAD) and late onset (LOAD). EOAD is predominantly familial, with mutations in genes encoding amyloid protein precursor (APP), presenilin-1 (PSEN1), and presenilin-2 (PSEN2) [64]. However, the intricate interaction between genetic and environmental variables that affect the development, course, and severity of the illness makes the genetic factors driving LOAD difficult to understand. To date, the apolipoprotein E (APOE) gene is the strongest genetic risk factor for developing LOAD [65, 66].
In this context, as essential tools for AD research, many lines of genetically altered mice were used to study the pathogenesis of AD and evaluate potential therapeutic interventions [67, 68]. In general, most transgenic animal models are based on rare, early-onset AD genes. For instance, the amyloid precursor protein/presenilin 1 (APP/PS1) model was commonly used and mainly characterized as the accumulation of Aβ peptide. Genetic mutations in this model of mice are associated with familial AD. Therefore, the APP/PS1 model has exhibited strong neuropathological markers of familial AD-like patterns at early ages [32, 69, 70].
It should be clarified that the APP/PS1 mice are limited to the expression of AD-related pathology linked to particular mutations present in EOAD. This model provides critical insights into amyloid accumulation, pathology, and clearance, but it is unable to capture all transcriptome characteristics and the entire neuropathology of LOAD [71]. Indeed, Aβ and tau pathology in sporadic and familial cases are morphologically similar, rationalizing the utilization of animal models with genetically engineered familial AD mutations for studying LOAD [71]. It is well known that Aβ deposition is generated by an imbalance between Aβ production and clearance. Evidence has suggested that the defective clearance of Aβ could be the driving force behind LOAD using the method of metabolic labeling in AD patients. According to the findings, reduced Aβ catabolism is a possible mechanism in LOAD, whereas enhanced anabolism of pathogenic Aβ results in pathological accumulation in familial AD [72]. Notably, reduced Aβ phagocytosis was found in the APP/PS1 mouse models. To be more specific, prolonged exposure to Aβ leads to persistent activation of microglial cells which attempt to clear the pathological deposits of Aβ through phagocytosis and degradation [73]. However, it is still a big challenge to understand the extent to which transgenic model mice recapitulate LOAD. More accurate animal models are expected to bring preclinical research closer to clinical trials in humans, especially for LOAD.
The interpretation of the conclusive description of the comparison between our mouse model and human AD remains challenging. A key point is the lack of concordance between animal models and human studies. Most of the models are missing the entire pathology, but rather specific features. Additionally, the host’s genetic background might alter gene expression, and pharmacokinetics may vary throughout species [74–76].
In summary, our results revealed the disrupted prefrontal-thalamic information transmission during retrieval in APP/PS1 mice with working memory deficits. Further research is needed to clarify the relationship between abnormal information transmission and declined cognitive function in mice and humans, as well as the potential value as a biomarker of pre-symptomatic AD.
CREDIT AUTHOR STATEMENT
Shengnan Zhang (Investigation; Formal Analysis; Software; Writing – Original Draft; Writing – Review & Editing); Hongrui Ai (Formal Analysis; Software); Jia Wang (Investigation); Tiaotiao Liu (Investigation; Writing – Review & Editing); Xuyuan Zheng (Conceptualization; Methodology; Supervision; Funding acquisition); Xin Tian (Conceptualization; Methodology; Supervision; Funding acquisition); Wenwen Bai (Conceptualization; Methodology; Writing – Original Draft; Writing – Review & Editing; Supervision; Funding acquisition).
ACKNOWLEDGMENTS
We thank Huiyun Yang for the comments and Qingying Cao for technical assistance.
FUNDING
This work was supported by the National Natural Science Foundation of China (61971311,61976157), Scientific Research Project of Tianjin Municipal Education Commission (2021KJ260) and Tianjin Research Innovation Project for Postgraduate Students (2022SKY220).
CONFLICT OF INTEREST
The authors have no conflict of interest to report.
DATA AVAILABILITY
The data that support the findings of this study are available from the corresponding author upon reasonable request.
SUPPLEMENTARY MATERIAL
[1] The supplementary material is available in the electronic version of this article: https://dx.doi.org/10.3233/JAD-231078.
REFERENCES
[1] | Fernandez G , Manes F , Rotstein NP , Colombo O , Mandolesi P , Politi LE , Agamennoni O ((2014) ) Lack of contextual-word predictability during reading in patients with mild Alzheimer disease. Neuropsychologia 62: , 143–151. |
[2] | Canter RG , Penney J , Tsai LH ((2016) ) The road to restoring neural circuits for the treatment of Alzheimer’s disease. Nature 539: , 187–196. |
[3] | Baddeley A ((2003) ) Working memory: Looking back and looking forward. Nat Rev Neurosci 4: , 829–839. |
[4] | Vogel P , Hahn J , Duvarci S , Sigurdsson T ((2022) ) Prefrontal pyramidal neurons are critical for all phases of working memory. Cell Rep 39: , 110659. |
[5] | Bolkan SS , Stujenske JM , Parnaudeau S , Spellman TJ , Rauffenbart C , Abbas AI , Harris AZ , Gordon JA , Kellendonk C ((2017) ) Thalamic projections sustain prefrontal activity during working memory maintenance. Nat Neurosci 20: , 987–996. |
[6] | Webster SJ , Bachstetter AD , Van Eldik LJ ((2013) ) Comprehensive behavioral characterization of an APP/PS-1 double knock-in mouse model of Alzheimer’s disease. Alzheimers Res Ther 5: , 28. |
[7] | Dudchenko PA ((2004) ) An overview of the tasks used to test working memory in rodents. Neurosci Biobehav Rev 28: , 699–709. |
[8] | Roy DS , Arons A , Mitchell TI , Pignatelli M , Ryan TJ , Tonegawa S ((2016) ) Memory retrieval by activating engram cells in mouse models of early Alzheimer’s disease. Nature 531: , 508–512. |
[9] | Li J , Broster LS , Jicha GA , Munro NB , Schmitt FA , Abner E , Kryscio R , Smith CD , Jiang Y ((2017) ) A cognitive electrophysiological signature differentiates amnestic mild cognitive impairment from normal aging. Alzheimers Res Ther 9: , 3. |
[10] | Bueno-Junior LS , Lopes-Aguiar C , Ruggiero RN , Romcy-Pereira RN , Leite JP ((2012) ) Muscarinic and nicotinic modulation of thalamo-prefrontal cortex synaptic plasticity [corrected]. PLoS One 7: , e47484. |
[11] | Lara AH , Wallis JD ((2015) ) The role of prefrontal cortex in working memory: A mini review. Front Syst Neurosci 9: , 173. |
[12] | Wood JN , Grafman J ((2003) ) Human prefrontal cortex: Processing and representational perspectives. Nat Rev Neurosci 4: , 139–147. |
[13] | Minzenberg MJ , Laird AR , Thelen S , Carter CS , Glahn DC ((2009) ) Meta-analysis of 41 functional neuroimaging studies of executive function in schizophrenia. Arch Gen Psychiatry 66: , 811–822. |
[14] | Perlstein WM , Carter CS , Noll DC , Cohen JD ((2001) ) Relation of prefrontal cortex dysfunction to working memory and symptoms in schizophrenia. Am J Psychiatry 158: , 1105–1113. |
[15] | Mirzayi P , Shobeiri P , Kalantari A , Perry G , Rezaei N ((2022) ) Optogenetics: Implications for Alzheimer’s disease research and therapy. Mol Brain 15: , 20. |
[16] | Wei J , Yi H , Zhang D , Bai W , Tian X ((2015) ) Aberrant neuronal activity and dysfunctional connectivity in Abeta1-42-mediated memory deficits in rats. Curr Alzheimer Res 12: , 964–973. |
[17] | Liu C , Zhang X , Chai H , Xu S , Liu Q , Luo Y , Li S ((2022) ) Identification of immune cells and key genes associated with Alzheimer’s disease. Int J Med Sci 19: , 112–125. |
[18] | Jobson DD , Hase Y , Clarkson AN , Kalaria RN ((2021) ) The role of the medial prefrontal cortex in cognition, ageing and dementia. Brain Commun 3: , fcab125. |
[19] | Mitchell AS ((2015) ) The mediodorsal thalamus as a higher order thalamic relay nucleus important for learning and decision-making. Neurosci Biobehav Rev 54: , 76–88. |
[20] | Saalmann YB ((2014) ) Intralaminar and medial thalamic influence on cortical synchrony, information transmission and cognition. Front Syst Neurosci 8: , 83. |
[21] | Bailey KR , Mair RG ((2005) ) Lesions of specific and nonspecific thalamic nuclei affect prefrontal cortex-dependent aspects of spatial working memory. Behav Neurosci 119: , 410–419. |
[22] | Stepan-Buksakowska I , Szabo N , Horinek D , Toth E , Hort J , Warner J , Charvat F , Vecsei L , Rocek M , Kincses ZT ((2014) ) Cortical and subcortical atrophy in Alzheimer disease: Parallel atrophy of thalamus and hippocampus. Alzheimer Dis Assoc Disord 28: , 65–72. |
[23] | de Kloet SF , Bruinsma B , Terra H , Heistek TS , Passchier EMJ , van den Berg AR , Luchicchi A , Min R , Pattij T , Mansvelder HD ((2021) ) Bi-directional regulation of cognitive control by distinct prefrontal cortical output neurons to thalamus and striatum. Nat Commun 12: , 1994. |
[24] | Wang J , Zhang S , Liu T , Zheng X , Tian X , Bai W ((2022) ) Directional prefrontal-thalamic information flow is selectively required during spatial working memory retrieval. Front Neurosci 16: , 1055986. |
[25] | Gilson M , Zamora-Lopez G , Pallares V , Adhikari MH , Senden M , Campo AT , Mantini D , Corbetta M , Deco G , Insabato A ((2020) ) Model-based whole-brain effective connectivity to study distributed cognition in health and disease. Netw Neurosci 4: , 338–373. |
[26] | Park HJ , Friston K ((2013) ) Structural and functional brain networks: From connections to cognition. Science 342: , 1238411. |
[27] | Wang X , Wang R , Li F , Lin Q , Zhao X , Hu Z ((2020) ) Large-scale Granger causal brain network based on resting-state fMRI data. Neuroscience 425: , 169–180. |
[28] | Fogel H , Levy-Lamdan O , Zifman N , Hiller T , Efrati S , Suzin G , Hack DC , Dolev I , Tanne D ((2021) ) Brain network integrity changes in subjective cognitive decline: A possible physiological biomarker of dementia. Front Neurol 12: , 699014. |
[29] | Zhang L , Glascher J ((2020) ) A brain network supporting social influences in human decision-making. Sci Adv 6: , eabb4159. |
[30] | Chen J , Tam A , Kebets V , Orban C , Ooi LQR , Asplund CL , Marek S , Dosenbach NUF , Eickhoff SB , Bzdok D , Holmes AJ , Yeo BTT ((2022) ) Shared and unique brain network features predict cognitive, personality, and mental health scores in the ABCD study. Nat Commun 13: , 2217. |
[31] | Alipour A , Mozhdehfarahbakhsh A , Nouri S , Petramfar P , Tahamtan M , Kamali AM , Rao KS , Nami M ((2020) ) Studies on the bottom-up and top-down neural information flow alterations in neurodegeneration. J Alzheimers Dis 78: , 169–183. |
[32] | Hickman SE , Allison EK , El Khoury J ((2008) ) Microglial dysfunction and defective beta-amyloid clearance pathways in aging Alzheimer’s disease mice. J Neurosci 28: , 8354–8360. |
[33] | Spellman T , Rigotti M , Ahmari SE , Fusi S , Gogos JA , Gordon JA ((2015) ) Hippocampal-prefrontal input supports spatial encoding in working memory. Nature 522: , 309–314. |
[34] | Liu L , Ikonen S , Heikkinen T , Heikkila M , Puolivali J , van Groen T , Tanila H ((2002) ) Effects of fimbria-fornixlesion and amyloid pathology on spatial learning and memory in transgenic APP+PS1 mice. Behav Brain Res 134: , 433–445. |
[35] | Benthem SD , Skelin I , Moseley SC , Stimmell AC , Dixon JR , Melilli AS , Molina L , McNaughton BL , Wilber AA ((2020) ) Impaired hippocampal-cortical interactions during sleep in a mouse model of Alzheimer’s disease. Curr Biol 30: , 2588–2601 e2585. |
[36] | Seth AK ((2010) ) A MATLAB toolbox for Granger causal connectivity analysis. J Neurosci Methods 186: , 262–273. |
[37] | Bressler SL , Kumar A , Singer I ((2021) ) Brain synchronization and multivariate autoregressive (MVAR) modeling in cognitive neurodynamics. Front Syst Neurosci 15: , 638269. |
[38] | He B , Dai Y , Astolfi L , Babiloni F , Yuan H , Yang L ((2011) ) eConnectome: A MATLAB toolbox for mapping and imaging of brain functional connectivity. J Neurosci Methods 195: , 261–269. |
[39] | Serrano-Pozo A , Frosch MP , Masliah E , Hyman BT ((2011) ) Neuropathological alterations in Alzheimer disease. Cold Spring Harb Perspect Med 1: , a006189. |
[40] | Grandjean J , Schroeter A , He P , Tanadini M , Keist R , Krstic D , Konietzko U , Klohs J , Nitsch RM , Rudin M ((2014) ) Early alterations in functional connectivity and white matter structure in a transgenic mouse model of cerebral amyloidosis. J Neurosci 34: , 13780–13789. |
[41] | Reitz C ((2012) ) Alzheimer’s disease and the amyloid cascade hypothesis: A critical review. Int J Alzheimers Dis 2012: , 369808. |
[42] | Hedden T , Van Dijk KR , Becker JA , Mehta A , Sperling RA , Johnson KA , Buckner RL ((2009) ) Disruption of functional connectivity in clinically normal older adults harboring amyloid burden. J Neurosci 29: , 12686–12694. |
[43] | Sperling RA , Laviolette PS , O’Keefe K , O’Brien J , Rentz DM , Pihlajamaki M , Marshall G , Hyman BT , Selkoe DJ , Hedden T , Buckner RL , Becker JA , Johnson KA ((2009) ) Amyloid deposition is associated with impaired default network function in older persons without dementia. Neuron 63: , 178–188. |
[44] | Shah D , Jonckers E , Praet J , Vanhoutte G , Delgado YPR , Bigot C , D’Souza DV , Verhoye M , Van der Linden A ((2013) ) Resting state FMRI reveals diminished functional connectivity in a mouse model of amyloidosis. PLoS One 8: , e84241. |
[45] | Adriaanse SM , Sanz-Arigita EJ , Binnewijzend MA , Ossenkoppele R , Tolboom N , van Assema DM , Wink AM , Boellaard R , Yaqub M , Windhorst AD , van der Flier WM , Scheltens P , Lammertsma AA , Rombouts SA , Barkhof F , van Berckel BN ((2014) ) Amyloid and its association with default network integrity in Alzheimer’s disease. Hum Brain Mapp 35: , 779–791. |
[46] | Filippini N , MacIntosh BJ , Hough MG , Goodwin GM , Frisoni GB , Smith SM , Matthews PM , Beckmann CF , Mackay CE ((2009) ) Distinct patterns of brain activity in young carriers of the APOE-epsilon4 allele. Proc Natl Acad Sci U S A 106: , 7209–7214. |
[47] | Spires-Jones T , Knafo S ((2012) ) Spines, plasticity, and cognition in Alzheimer’s model mice. Neural Plast 2012: , 319836. |
[48] | Mucke L , Masliah E , Yu GQ , Mallory M , Rockenstein EM , Tatsuno G , Hu K , Kholodenko D , Johnson-Wood K , McConlogue L ((2000) ) High-level neuronal expression of abeta 1-42 in wild-type human amyloid protein precursor transgenic mice: Synaptotoxicity without plaque formation. J Neurosci 20: , 4050–4058. |
[49] | Lue LF , Kuo YM , Roher AE , Brachova L , Shen Y , Sue L , Beach T , Kurth JH , Rydel RE , Rogers J ((1999) ) Soluble amyloid beta peptide concentration as a predictor of synaptic change in Alzheimer’s disease. Am J Pathol 155: , 853–862. |
[50] | Larson J , Lynch G , Games D , Seubert P ((1999) ) Alterations in synaptic transmission and long-term potentiation in hippocampal slices from young and aged PDAPP mice. Brain Res 840: , 23–35. |
[51] | Chapman PF , White GL , Jones MW , Cooper-Blacketer D , Marshall VJ , Irizarry M , Younkin L , Good MA , Bliss TV , Hyman BT , Younkin SG , Hsiao KK ((1999) ) Impaired synaptic plasticity and learning in aged amyloid precursor protein transgenic mice. Nat Neurosci 2: , 271–276. |
[52] | Selkoe DJ ((2002) ) Alzheimer’s disease is a synaptic failure. Science 298: , 789–791. |
[53] | Yuan P , Zhang M , Tong L , Morse TM , McDougal RA , Ding H , Chan D , Cai Y , Grutzendler J ((2022) ) PLD3 affects axonal spheroids and network defects in Alzheimer’s disease. Nature 612: , 328–337. |
[54] | Herweg NA , Solomon EA , Kahana MJ ((2020) ) Theta oscillations in human memory. Trends Cogn Sci 24: , 208–227. |
[55] | Wirt RA , Crew LA , Ortiz AA , McNeela AM , Flores E , Kinney JW , Hyman JM ((2021) ) Altered theta rhythm and hippocampal-cortical interactions underlie working memory deficits in a hyperglycemia risk factor model of Alzheimer’s disease. Commun Biol 4: , 1036. |
[56] | Mehak SF , Shivakumar AB , Kumari S , Muralidharan B , Gangadharan G ((2022) ) Theta and gamma oscillatory dynamics in mouse models of Alzheimer’s disease: A path to prospective therapeutic intervention. Neurosci Biobehav Rev 136: , 104628. |
[57] | Fernández-Ruiz A , Oliva A , Soula M , Rocha-Almeida F , Nagy GA , Martin-Vazquez G , Buzsáki G ((2021) ) Gamma rhythm communicationbetween entorhinal cortex and dentate gyrus neuronal assemblies. Science 372: , eabf3119. |
[58] | Casula EP , Pellicciari MC , Bonni S , Borghi I , Maiella M , Assogna M , Minei M , Motta C , D’Acunto A , Porrazzini F , Pezzopane V , Mencarelli L , Roncaioli A , Rocchi L , Spampinato DA , Caltagirone C , Santarnecchi E , Martorana A , Koch G ((2022) ) Decreased frontal gamma activity in Alzheimer disease patients. Ann Neurol 92: , 464–475. |
[59] | Bobola MS , Chen L , Ezeokeke CK , Olmstead TA , Nguyen C , Sahota A , Williams RG , Mourad PD ((2020) ) Transcranial focused ultrasound, pulsed at 40Hz, activates microglia acutely and reduces Aβ load chronically, as demonstrated. Brain Stimul 13: , 1014–1023. |
[60] | Iaccarino HF , Singer AC , Martorell AJ , Rudenko A , Gao F , Gillingham TZ , Mathys H , Seo J , Kritskiy O , Abdurrob F , Adaikkan C , Canter RG , Rueda R , Brown EN , Boyden ES , Tsai LH ((2016) ) Gamma frequency entrainment attenuates amyloid load and modifies microglia. Nature 540: , 230–235. |
[61] | Martorell AJ , Paulson AL , Suk HJ , Abdurrob F , Drummond GT , Guan W , Young JZ , Kim DN , Kritskiy O , Barker SJ , Mangena V , Prince SM , Brown EN , Chung K , Boyden ES , Singer AC , Tsai LH ((2019) ) Multi-sensory gamma stimulation ameliorates Alzheimer’s-associated pathology and improves cognition. Cell 177: , 256–271.e222. |
[62] | Goutagny R , Gu N , Cavanagh C , Jackson J , Chabot JG , Quirion R , Krantic S , Williams S ((2013) ) Alterations in hippocampal network oscillations and theta-gamma coupling arise before Aβ overproduction in a mouse model of Alzheimer’s disease. Eur J Neurosci 37: , 1896–1902. |
[63] | Rajji TK , Zomorrodi R , Barr MS , Blumberger DM , Mulsant BH , Daskalakis ZJ ((2017) ) Ordering information in working memory and modulation of gamma by theta oscillations in humans. Cereb Cortex 27: , 1482–1490. |
[64] | Pandey RS , Graham L , Uyar A , Preuss C , Howell GR , Carter GW ((2019) ) Genetic perturbations of disease risk genes in mice capture transcriptomic signatures of late-onset Alzheimer’s disease. Mol Neurodegener 14: , 50. |
[65] | Bu G ((2009) ) Apolipoprotein E and its receptors in Alzheimer’s disease: Pathways, pathogenesis and therapy. Nat Rev Neurosci 10: , 333–344. |
[66] | Mahan TE , Wang C , Bao X , Choudhury A , Ulrich JD , Holtzman DM ((2022) ) Selective reduction of astrocyte apoE3 and apoE4 strongly reduces Aβ accumulation and plaque-related pathology in a mouse model of amyloidosis. Mol Neurodegener 17: , 13. |
[67] | Hall AM , Roberson ED ((2012) ) Mouse models of Alzheimer’s disease. Brain Res Bull 88: , 3–12. |
[68] | Esquerda-Canals G , Montoliu-Gaya L , Güell-Bosch J , Villegas S ((2017) ) Mouse models of Alzheimer’s disease. J Alzheimers Dis 57: , 1171–1183. |
[69] | Lok K , Zhao H , Shen H , Wang Z , Gao X , Zhao W , Yin M ((2013) ) Characterization of the APP/PS1 mouse model of Alzheimer’s disease in senescence accelerated background. Neurosci Lett 557 Pt B: , 84–89. |
[70] | Porquet D , Andrés-Benito P , Griñán-Ferré C , Camins A , Ferrer I , Canudas AM , Del Valle J , Pallàs M ((2015) ) Amyloidand tau pathology of familial Alzheimer’s disease APP/PS1 mousemodel in a senescence phenotype background (SAMP8). Age(Dordr) 37: , 9747. |
[71] | Sasaguri H , Nilsson P , Hashimoto S , Nagata K , Saito T , De Strooper B , Hardy J , Vassar R , Winblad B , Saido TC ((2017) ) APP mouse models for Alzheimer’s disease preclinical studies. EMBO J 36: , 2473–2487. |
[72] | Mawuenyega KG , Sigurdson W , Ovod V , Munsell L , Kasten T , Morris JC , Yarasheski KE , Bateman RJ ((2010) ) Decreased clearance of CNS beta-amyloid in Alzheimer’s disease. Science 330: , 1774. |
[73] | Heneka MT , Kummer MP , Stutz A , Delekate A , Schwartz S , Vieira-Saecker A , Griep A , Axt D , Remus A , Tzeng TC , Gelpi E , Halle A , Korte M , Latz E , Golenbock DT ((2013) ) NLRP3 is activated in Alzheimer’s disease and contributes to pathology in APP/PS1 mice. Nature 493: , 674–678. |
[74] | Huang Y , Mucke L ((2012) ) Alzheimer mechanisms and therapeutic strategies. Cell 148: , 1204–1222. |
[75] | Miller JA , Horvath S , Geschwind DH ((2010) ) Divergence of human and mouse brain transcriptome highlights Alzheimer disease pathways. Proc Natl Acad Sci U S A 107: , 12698–12703. |
[76] | Bilkei-Gorzo A ((2014) ) Genetic mouse models of brain ageing and Alzheimer’s disease. Pharmacol Ther 142: , 244–257. |