FACEmemory®, an Innovative Online Platform for Episodic Memory Pre-Screening: Findings from the First 3,000 Participants
Abstract
Background:
The FACEmemory® online platform comprises a complex memory test and sociodemographic, medical, and family questions. This is the first study of a completely self-administered memory test with voice recognition, pre-tested in a memory clinic, sensitive to Alzheimer’s disease, using information and communication technologies, and offered freely worldwide.
Objective:
To investigate the demographic and clinical variables associated with the total FACEmemory score, and to identify distinct patterns of memory performance on FACEmemory.
Methods:
Data from the first 3,000 subjects who completed the FACEmemory test were analyzed. Descriptive analyses were applied to demographic, FACEmemory, and medical and family variables; t-test and chi-square analyses were used to compare participants with preserved versus impaired performance on FACEmemory (cut-off = 32); multiple linear regression was used to identify variables that modulate FACEmemory performance; and machine learning techniques were applied to identify different memory patterns.
Results:
Participants had a mean age of 50.57 years and 13.65 years of schooling; 64.07% were women, and 82.10% reported memory complaints with worries. The group with impaired FACEmemory performance (20.40%) was older, had less schooling, and had a higher prevalence of hypertension, diabetes, dyslipidemia, and family history of neurodegenerative disease than the group with preserved performance. Age, schooling, sex, country, and completion of the medical and family history questionnaire were associated with the FACEmemory score. Finally, machine learning techniques identified four patterns of FACEmemory performance: normal, dysexecutive, storage, and completely impaired.
Conclusions:
FACEmemory is a promising tool for assessing memory in people with subjective memory complaints and for raising awareness about cognitive decline in the community.
INTRODUCTION
Alzheimer’s disease (AD) represents one of the major global health problems of the 21st century [1]. Its social and health impact has been highlighted by the World Health Organization (WHO), encouraging institutions to improve early detection of AD worldwide. While there is currently no cure for AD, cognitive training [2] and implementing preventive healthcare and lifestyle measures [3] may reduce dementia and promote overall brain health. With the approval of the first disease-modifying therapies for AD [4], the current challenge consists of detecting people at risk of developing AD to apply precision medicine before irreparable brain damage occurs. This challenge requires public engagement, that is, doing research that is relevant to society [5].
Because standard neuropsychological tests have not been designed to screen large numbers of people with hidden cognitive deficits in the community, computerized cognitive batteries, such as the Cambridge Neuropsychological Test Automated Battery (CANTAB) [6] and CogState [7], are receiving increasing interest [8]. However, to date, most computerized cognitive tests need the supervision of an examiner or utilize only visual or non-verbal tasks [8], such as a computerized version of the Face-Name Associative Memory Exam (FNAME). In this test, people are asked to remember 12 face-name pairs presented serially, and 12 to 15 min later, each face is presented with three names, and participants have to select the correct name on a touch screen (recognition memory) [9, 10]. For this reason, FACEmemory® [11], the first self-administered face-name associative memory test with voice recognition and automated scoring, was developed at the Ace Alzheimer Center Barcelona. This test is recognized to have a high quality based on the Newcastle-Ottawa Quality Assessment Scale Criteria [8].
FACEmemory is a computerized version of the complex episodic memory test FNAME abbreviated to 12 items (FNAME-12) [11], which has been demonstrated to be sensitive to early- and late-onset amnestic mild cognitive impairment (MCI), as well as the AD endophenotype and biomarkers. That is, performance on FACEmemory, self-administered under minimal supervision of a neuropsychologist at the Ace Alzheimer Center Barcelona, was found suitable for discriminating between cognitively healthy and amnestic MCI individuals. The outcomes of the test correlated with performance on the Free and Cued Selective Reminding Test and were associated with AD-related cerebrospinal fluid and magnetic resonance imaging biomarkers of AD, but also with other etiologies [11, 12].
The detection of individuals with amnestic MCI is relevant because of their increased risk of conversion to dementia, especially AD, which occurs in around 18% of cases annually. Specifically, the amnestic MCI phenotype, with an impairment of memory storage, has an 8.5 times higher risk of converting to dementia than the non-amnestic MCI phenotype [13]; therefore, assessing memory is key to detecting AD. It is well known that using tests of greater complexity than those typically administered in clinical neuropsychological practice allows the detection of more subtle cognitive impairments. Concerning memory, associating unfamiliar faces with proper names is a more complex task than many other memory tasks because it is a completely arbitrary association [14]. For this reason, the FNAME is more sensitive to AD and has ecological validity as it tests a universal skill [15, 16].
To promote brain health and facilitate early detection of early MCI and AD, in 2008 the Ace Alzheimer Center Barcelona initiated an Open House Initiative (OHI), offering a free memory pre-screening test to Barcelona citizens older than 50 years through local marketing campaigns [17]; to date, 63% have been diagnosed as cognitively healthy, 34% as having MCI, and only 3% as suffering from dementia. This strategy allows people with subtle cognitive deficits to access appropriate health services directly without the need for prior consultation with primary care. Notably, individuals with memory complaints identified through the OHI had milder cognitive impairment than those referred by primary care to the Ace Memory Unit [16, 17]. Next, the innovative European MOPEAD (Models of Patient Engagement for Alzheimer’s Disease) project, led by the Ace Alzheimer Center Barcelona, demonstrated the validity of an online pre-screening cognitive assessment with two computerized non-verbal CANTAB subtests to detect undiagnosed MCI and dementia in individuals who would not have consulted health care without online advertising campaigns [18].
The present study aims to move FACEmemory further toward the goal of community-based cognitive screening. Having demonstrated its efficacy as a self-administered computerized screening test for use in the clinic with minimal supervision, we developed FACEmemory into an online tool with voice recognition to facilitate the kind of societal reach that is needed for the early and large-scale detection of cognitive impairment and AD. The online FACEmemory platform is currently offered in Spanish and Catalan, making it accessible to a very large number of people worldwide.
Initially, the target population of the FACEmemory platform’s campaign was people over 50 years old, who spoke Spanish or Catalan, and who were able to use a tablet or a computer. However, we finally decided to make memory testing accessible to anyone over 18 years old without restrictions and present all the data. The main aims of the present study are to investigate the demographic and clinical variables associated with the total FACEmemory score, and to identify distinct patterns of memory performance among the first 3,000 individuals who completed the FACEmemory test.
METHODS
Participants
The first 3,000 individuals over 18 years old who completed the online FACEmemory test after its launch on May 29, 2021, were included in the present study. The platform was advertised following a proactive and informative communication strategy, designed to reach an audience that was not affected by dementia.
The campaign resulted in the generation of content for the media, the web, social networks (Facebook, Twitter, LinkedIn, Instagram), and Ace’s contact database. There was also printed material that clinicians and medical staff could give away in Ace’s facilities. This content was focused on the importance of memory, with claims such as “If you check your cholesterol, why not your memory?"
The FACEmemory® platform
As detailed elsewhere [11], the FACEmemory test is an innovative adaptation of the abbreviated version [19] of the Spanish FNAME [20]. The FACEmemory platform has now become a free-to-use online resource (https://www.fundacioace.com/en/check-your-memory-online-with-facememory.html). that provides a self-administered face-name association memory task with voice recognition to make it easy to use and to enable automated scoring. To our knowledge, it is the first completely self-administered memory test with voice recognition, pre-tested in a memory clinic with a high level of satisfaction independently of the results obtained, offered freely worldwide on a website platform assessing memory, registered in a database, and able to send feedback by e-mail to each user. It is accessible to the entire Spanish- and Catalan-speaking population with an internet connection.
The application has three major components: 1) The web client is the page published to the web and allows the end users to access the test. It interacts with the user, displays the test, and collects the user’s answers. It is built with Ionic-Vue and used as a single-page application (SPA). 2) The backend server, where all the data processing happens, validates all the data sent by the client and handles all the test processes. It is built with Laravel, a popular PHP framework. 3) The admin panel is where the FACEmemory team can check the user’s answers and scores, see how the test was performed, extract detailed data and information, and draw conclusions about the results of the test. A personalized analysis of each participant is performed. The analysis is built with the Inertia stack in Vue and is served directly by the backend server. Finally, the application uses the Google speech-to-text API to understand the users’ answers to the memory test questions (12 names and 12 occupations). The hosting is a cloud VPS that handles all requests given that the user flow is constant. Importantly, the FACEmemory platform ensures that voice data cannot be linked to any user or score.
Regarding the FACEmemory procedure, after selecting the preferred language of administration (Spanish or Catalan), people who enter the FACEmemory platform are first asked to accept informed consent for completing the test. Then, they provide basic demographical data, including age, sex, schooling level, the country from which they are completing the test, and an e-mail address to receive their results. Moreover, participants are asked three questions about subjective memory complaints: 1) “Do you feel that your memory has worsened?” (yes/no), 2) “Are you worried about it?” (yes/no), and 3) “Since when have noticed it? (number in years). Then, a brief video introduces the FACEmemory test procedure, followed by an audio test to ensure the correct functioning of the voice recognition for optimal administration by the selected device (tablet or computer).
In a study published previously [11], the FACEmemory test was self-administered on a tablet under minimal supervision of a psychologist, who detected some difficulties in handling the voice recognition button. In the next step, before launching the completely self-administered FACEmemory online platform, an audio test was added to verify microphone functionality and the proper use of the voice button by the participant.
The test includes two trials to learn the 12 face-name-occupation associations, a short-term memory task, and a long-term memory assessment that includes face, name, and occupation memory recognition tasks. After the participant has completed the test, performance is scored automatically, registered in a database, and communicated to the participant by e-mail, mentioning that “After evaluating your results, your cognitive profile is established as cognitively healthy” or “After evaluating your results, we inform you that you have obtained a score slightly lower than expected. Bear in mind that this may be due to various factors that could have influenced the performance of the FACEmemory® test. We recommend that you undergo a complete cognitive examination.”
The first learning trial consists of showing pictures of a total of 12 faces, each one associated with a name and an occupation that appear beneath the face for 8 seconds. The participant is instructed to read aloud the name and occupation appearing beneath each face and to try to remember them. Immediately after the first learning trial, the same faces are presented, one by one, only now with a red microphone button (that is, without the name and occupation below), and the participant is asked to press the red microphone button and to say the name (LN1) and occupation (LO1) he/she remembers as being associated with that face. When the 12 faces have consecutively appeared, the second learning trial starts (LN2 and LO2). It follows the same procedure as the first learning trial, except that the faces appear in another order. Once the 12 stimuli have been presented and the participant has been asked to finish the learning task, there is a 2-min delay before the short-term memory assessment starts. Each face is presented again, only now with a red microphone button, and the participant is asked to say the name (RSN) and occupation (RSO) he/she remembers as being associated with each face. Finally, 15 min after the second learning trial has ended, the long-term memory assessment starts. First, each participant is instructed to recognize from a set of three faces the one that appeared in the learning trials and to select it (FR). Then, the correct face appears, and the participant is asked to say the name (RLN) and occupation (RLO) he/she remembers for each face. After each answer, a screen shows the correct face with below it three name and three occupation options. Each participant is instructed to select the name (REN) and occupation (REO) recognized as being associated with that face.
During the 15-min delay between the end of the learning stage and the start of the long-term memory probe, a questionnaire about medical (that is, psychiatric disease, hypertension, diabetes) and family history (that is, AD, Parkinson’s disease) is self-administered. However, since the main goal is the standardized administration of the FACEmemory test, the medical and family history questionnaire is not mandatory. That is, in those cases in which participants have not completed or finished, for any reason, the medical and family history questionnaire during the 13 min, the system moves on to the FACEmemory long-term recall test, and then it is no longer possible to go back to complete the questionnaire.
The oral and tactile responses of the participants were automatically registered and entered into a database anonymously. All FACEmemory subscores (LN1, LO1, LN2, LO2, RSN, RSO, RLN, RLO, FR, REN, and REO) ranged from 0 to 12, and the total FACEmemory score ranged from 0 to 96 (without recognition). Time of execution was also registered.
The FACEmemory test has been proven to be highly effective in discriminating between cognitively healthy individuals and patients with MCI, mainly of the amnestic type. The cut-offs of the test, when administered on a tablet under minimal supervision of a psychologist at a memory unit, have previously been reported [9]. An adequate area under the curve (AUC) of 0.85 was ensured before determining the total FACEmemory cut-off of 32. This cut-off has been found to be highly effective, with 80.5% sensitivity and 80.0% specificity, when cognitively healthy and amnestic MCI groups were contrasted. Moreover, the high correlation values obtained between automatic and manual FACEmemory scores (r = 0.98) demonstrated the reliability of FACEmemory’s automatic scoring [9].
Statistical analysis
Statistical analyses were performed using the Statistical Package for the Social Sciences (SPSS) for Windows (version 26.0; SPSS Inc., Chicago, IL, USA) and Python version 3.9.13. Variables were examined for normality, skewness, and restriction of range. Descriptive analyses of demographic data, FACEmemory scores, and medical and family history questionnaire variables were carried out.
The percentage of subjects with impaired performance on the total FACEmemory score was calculated using the cut-off of 32 points obtained at the Ace Alzheimer Center Barcelona’s Memory Unit [11]. Further, t-test and chi-square analyses were carried out to compare demographic data and clinical variables from the medical and family history questionnaire between participants 1) with a preserved and impaired performance on the FACEmemory test (>31 and <32 points, respectively), 2) with and without memory complaints, and 3) having completed the test inside and outside Spain. Moreover, a multiple linear regression was carried out to identify the impact of demographic and clinical variables on the total FACEmemory score (0 to 96 points).
Finally, unsupervised machine learning techniques were applied to search for different patterns of memory performance in the whole sample using the FACEmemory subscores. All the FACEmemory subscores are depicted in Table 1 (LN1, LO1, LN2, LO2, RSN, RSO, RLN, RLO, FR, REN, and REO) and were standardized to z-scores and modeled. To reduce the dimensionality of the data, a principal component analysis (PCA) was applied with all the FACEmemory subscores. The input data for the models consisted of the first five components, accounting for 85.04% of the variance.
Table 1
Performance on the FACEmemory variables
Variable (min-max) | Whole sample (mean, SD) | Preserved (mean, SD) | Impaired (mean, SD) |
LN1 (0–12) | 2.50 (2.54) | 2.99 (2.60) | 0.58 (0.87) |
LO1 (0–12) | 5.29 (2.84) | 6.05 (2.56) | 2.32 (1.71) |
LN2 (0–12) | 6.27 (3.55) | 7.37 (3.04) | 1.99 (1.65) |
LO2 (0–12) | 8.16 (2.89) | 9.11 (2.15) | 4.44 (2.38) |
RSN (0–12) | 6.05 (3.66) | 7.17 (3.17) | 1.68 (1.58) |
RSO (0–12) | 8.13 (3.04) | 9.14 (2.26) | 4.19 (2.44) |
REN (0–12) | 10.20 (2.29) | 10.86 (1.59) | 7.62 (2.75) |
REO (1–12) | 11.64 (0.96) | 11.86 (0.44) | 10.79 (1.69) |
RLN (0–12) | 5.92 (3.72) | 7.04 (3.26) | 1.52 (1.49) |
RLO (0–12) | 7.98 (3.08) | 9.02 (2.26) | 3.91 (2.40) |
FR (2–12) | 11.88 (0.58) | 11.94 (0.32) | 11.67 (1.08) |
Total score (0–96) | 50.33 (20.90) | 57.91 (15.76) | 20.75 (8.47) |
Execution time (min) (20–60) | 27.06 (5.20) | 26.56 (4.57) | 28.89 (6.76) |
SD, standard deviation; min-max, minimum-maximum registered; LN1, names recalled in learning 1; LN2, names recalled in learning 2; LO1, occupations recalled in learning 1; LO2, occupations recalled in learning 2; RSN, names in short-term recall; RSO, occupations in short-term recall; RLN, names in long-term recall; RLO, occupations in long-term recall; FR, face recognition; REN, names correctly recognized; REO, occupations correctly recognized.
Unsupervised analyses were performed using Gaussian mixture models (GMMs). The assignment of a given individual to a cluster is not absolute (hard) but probabilistic (soft), and the final cluster membership is defined as the cluster to which an individual belongs with the highest probability. Briefly, these models assume that the data are generated by a series of C unobserved groups (aka component distributions), each with a different probability distribution describing the observed variables
The Scikit-learn implementation of GMMs was used [21]. In the models, each component was allowed to have its own covariance matrix. The initial groups were determined by the k-means algorithm, and a maximum number of iterations of the expectation-maximization algorithm of 1,000 was selected by performing 150 random initializations. The rest of the hyperparameters of the model were left as default. The number of groups was selected based on the Bayesian information criterion (BIC) [22]. Finally, a descriptive analysis of the resulting groups was performed considering the values of the FACEmemory subscores and the demographic and clinical variables. Analysis of variance (ANOVA) tests were applied for quantitative variables, and χ2 tests for binary variables. The groups obtained from the FACEmemory subscales were interpreted from a neuropsychological point of view [23] to search for clinical memory patterns, mainly optimal, dysexecutive and storage impairment (to detect AD type) [13, 24].
For all analyses, an effect was considered significant when the p-value was less than 0.05 after applying the Bonferroni correction for multiple comparisons. All hypotheses were tested directionally at a 95% confidence level.
Ethics approval and consent to participate
Prior to completion of the FACEmemory test, each participant completed an informed consent form on FACEmemory’s website. The research was conducted in accordance with the Declaration of Helsinki and with Spanish biomedical laws (Law 14/2007, July 3, about biomedical research; Royal Decree 1716/2011, November 18). The study was approved by the Research Ethics Committee of the Hospital de Bellvitge (Barcelona).
RESULTS
Demographic variables
The study sample consisted of the first 3,000 participants over 18 years old who completed the FACEmemory platform since its opening on May 29, 2021. This number was reached on January 18, 2023 (that is, after 1.65 years). As detailed in Table 2, the study sample had a mean age of 50.57 years (standard deviation, SD: 13.98, ranging from 18 to 89), 56.20% were older than 50 years, and 64.07% were women. Most participants had a high level of schooling: 61.57% had a university degree, 37.17% had elementary or high school, and only 1.27% were literate with less than elementary school (that is, from 1 to 5 years of formal education). In terms of the language of administration, most participants (84.53%) chose Spanish, and 15.47% Catalan. The FACEmemory test was carried out from 37 different countries, mainly from Spain (71.97%), followed by Argentina and Mexico (5.17% and 4.90%, respectively).
Table 2
Demographic (n = 3,000), memory complaints (n = 3,000), and medical and family history (n = 2,103) variables of the whole study sample and comparison between participants with a preserved and an impaired FACEmemory total score
Whole sample | Preserved | Impaired | Statistics | |
FACEmemory | FACEmemory | |||
N | 3,000 | 2,388 | 612 | |
Age (mean, SD) | 50.57 (13.98) | 49.22 (13.06) | 55.84 (16.06) | 9.431* |
Sex (N, % woman) | 1,922 (64.07) | 1570 (65.75) | 352 (57.52) | 13.972* |
Years of schooling (mean, SD) | 13.65 (6.13) | 14.04 (6.12) | 12.11 (5.93) | 7.151* |
Level of schooling (N, %) | 184.302* | |||
Less than elementary school | 38 (1.27) | 9 (0.38) | 29 (4.74) | |
Elementary or high school | 1,115 (37.17) | 784 (32.83) | 331 (54.08) | |
University degree | 1,847 (61.57) | 1,595 (66.79) | 252 (41.18) | |
Country (N, % Spain) | 2,159 (71.97) | 1,762 (73.79) | 397 (64.87) | 18.762* |
Language (N, % Spanish) | 2,536 (84.53) | 2,029 (84.97) | 507 (82.84) | 1.522 |
Memory complaints (N, %) | 2,463 (82.10) | 1,951 (81.70) | 512 (83.66) | 1.142 |
Questionnaire completed (N, %) | 2,103 (70.10) | 1,763 (73.83) | 340 (55.56) | 76.732* |
N | 2,103 | 1,763 | 340 | |
Smoking (N, %) | 343 (16.31) | 288 (16.34) | 55 (16.18) | 0.022 |
Alcohol consumption (N, %) | 813 (38.66) | 685 (38.85) | 128 (37.65) | 0.982 |
Auditory abnormalities (N, %) | 402 (19.12) | 338 (19.17) | 64 (18.82) | 0.182 |
Visual abnormalities (N, %) | 813 (38.66) | 671 (38.06) | 142 (41.76) | 0.322 |
Hypertension (N, %) | 365 (17.36) | 280 (15.88) | 85 (25.00) | 24.242* |
Diabetes mellitus (N, %) | 108 (5.14) | 84 (4.76) | 24 (7.06) | 7.392 |
Dyslipidemia (N, %) | 544 (25.87) | 439 (24.90) | 105 (30.88) | 4.032 |
Cardiopathy (N, %) | 130 (6.18) | 96 (5.45) | 34 (10.00) | 3.252 |
Stroke (N, %) | 19 (0.90) | 13 (0.74) | 6 (1.76) | 1.202 |
Neurologic/psychiatric disease (N, %) | 509 (24.20) | 416 (23.60) | 93 (27.35) | 3.402 |
Family history of vascular dementia (N, %) | 246 (11.70) | 209 (11.85) | 37 (10.88) | 0.182 |
Family history of AD/ neurodegenerative | 1,251 (59.49) | 1,053 (59.73) | 198 (58.24) | 27.152* |
disease (N, %) |
SD, standard deviation; 1t-test; 2 χ2-test; *Bonferroni corrected p-value < 0.05.
Concerning the subjective cognitive complaints, most participants (2,463 out of 3,000, 82.10%) reported memory complaints with associated worries (that is, they answered “yes” to the questions “Do you feel that your memory has worsened?” and “Are you worried about it?”).
FACEmemory data
The mean total FACEmemory score obtained was 50.33 (SD: 20.90). The average execution time was 27 min. The total FACEmemory score was weakly correlated with execution time (r = 0.21, p < 0.001). The total score and subscores obtained on the FACEmemory test are detailed in Table 1. Using the FACEmemory total score cut-off of 32 points, performance was found impaired in 20.40% of the whole sample, increasing to 24.25% in those older than 50 years. As detailed in Table 2, the group with impaired performance on FACEmemory was significantly older, had fewer years of formal education, had a higher frequency of men, had a higher proportion of people who answered from outside Spain, had a higher percentage of hypertension, and had more often a family history of neurodegenerative disease than the group with a preserved performance.
People with memory complaints and associated worries performed worse on FACEmemory than those without [49.58 (20.69) and 53.76 (21.49), respectively; t= 4.11, p < 0.001]. Of the 3,000 subjects who completed the FACEmemory test, 29.90% (n = 897) did not fill out the medical and family history questionnaire. Compared with those who completed the medical and family history questionnaire, those who did not were older [49.46 (13.52) and 53.17 (14.67), respectively; t= 6.48, p < 0.001], were more often female (62.25% and 67.76%, respectively; χ2 = 6.99, p = 0.008), had fewer years of schooling [14.63 (4.29) and 13.58 (4.55), respectively; t= 5.60, p < 0.001], had a lower total FACEmemory score [52.76 (20.27) and 44.63 (21.25), respectively; t= 9.72, p < 0.001], and scored more often below the cut-off of 32 (16.17% and 30.32%, respectively; χ2 = 77.60, p < 0.001).
The group that completed the FACEmemory test from Spain performed better than the group from outside Spain [50.33 (20.90) and 46.30 (20.33), respectively; t= 6.71, p < 0.001)], were younger [44.34 (14.23) and 52.99 (13.10), respectively; t= 15.27, p < 0.001)], had a higher frequency of women (69.44% and 61.97%, respectively; χ2 = 14.33, p < 0.001), and had a higher frequency of people with subjective memory complaints (87.99% and 79.81%, respectively; χ2 = 5.78, p < 0.001).
Multiple regression analyses showed that a younger age, more years of schooling, female sex, inside Spain, and completion of the medical and family history questionnaire were statistically associated with a better total FACEmemory score (for details, see Table 3). Moreover, in the subsample who completed the medical and family history questionnaire (n = 2,013), the presence of subjective memory complaints with associated worries was also statistically associated with a worse performance on the FACEmemory test (Table 4).
Table 3
Multiple regression analyses with the total FACEmemory score as the dependent variable and the demographic features as independent variables (n = 3,000)
Variable | Coefficient | z-statistic | p1 | 95% CI |
Years of schooling | 0.46 | 7.94 | <0.001 | [0.37, 0.56] |
Age | –0.39 | –14.64 | <0.001 | [–0.44, –0.35] |
Sex2 | 4.60 | 6.19 | <0.001 | [3.38, 5.82] |
Country3 | 8.82 | 10.32 | <0.001 | [7.41, 10.23] |
Language4 | 2.68 | 2.63 | 0.054 | [1.00, 4.36] |
Questionnaire completed5 | 5.39 | 6.78 | <0.001 | [4.08, 6.69] |
1Bonferroni corrected p-value for multiple comparisons; 2woman = 1 and man = 0; 3Spain = 1 and other country = 0; 4Spanish = 1 and other language = 0; 5Open House Initiative questionnaire completed = 1 and not completed = 0.
Table 4
Multiple regression analyses with the total FACEmemory score as the dependent variable and the demographic and questionnaire items as independent variables. In this analysis, the subsample that filled out the medical and family history questionnaire was included (n = 2,103)
Variable | Coefficient | z-statistic | p1 | 95% CI |
Years of schooling | 0.33 | 4.87 | <0.001 | [0.22, 0.44] |
Age | –0.34 | –9.42 | <0.001 | [–0.40, –0.28] |
Sex2 | 4.45 | 4.91 | <0.001 | [2.96, 5.94] |
Country3 | 10.10 | 9.45 | <0.001 | [8.35, 11.86] |
Language4 | 2.88 | 2.42 | 0.285 | [0.92, 4.84] |
Memory complaints5 | –3.34 | –3.05 | 0.038 | [–5.15, –1.54] |
Smoking5 | –1.51 | –1.30 | >0.999 | [–3.4, 0.40] |
Alcohol consumption5 | 2.26 | 2.53 | >0.999 | [0.79, 3.72] |
Auditory abnormalities5 | 0.44 | 0.39 | >0.999 | [–1.39, 2.26] |
Visual abnormalities5 | 0.25 | 0.28 | >0.999 | [–1.24, 1.74] |
Hypertension5 | –1.35 | –1.10 | >0.999 | [–3.36, 0.67] |
Diabetes mellitus5 | –1.07 | –0.54 | >0.999 | [–4.32, 2.17] |
Dyslipidemia5 | 1.33 | 1.31 | >0.999 | [–0.35, 3.00] |
Cardiopathy5 | –1.43 | –0.79 | >0.999 | [–4.40, 1.54] |
Stroke5 | –7.58 | –1.69 | >0.999 | [–14.99, –0.18] |
Neurological/psychiatric disease5 | –0.20 | –0.20 | >0.999 | [–1.84, 1.44] |
Family history of vascular dementia5 | 1.75 | 1.31 | >0.999 | [–0.44, 3.95] |
Family history of AD/neurodegenerative disease5 | 0.09 | 0.11 | >0.999 | [–1.33, 1.52] |
1Bonferroni corrected p-value for multiple comparisons; 2female = 1 and male = 0; 3Spain = 1 and other than Spain = 0; 4Spanish = 1 and Catalan = 0; 5binary variable from the questionnaire, where 1 indicates an affirmative answer and 0 indicates a negative answer.
Finally, unsupervised machine learning analysis identified four different clusters of FACEmemory performance. In Fig. 1, cluster membership is represented in probabilistic terms (a higher intensity indicates that the subject has a higher probability of belonging to the cluster), indicating the uncertainty of assigning individuals to each cluster. The descriptive analyses of the sociodemographic variables, the medical and family history questionnaire, and the test subscales are shown in Table 5. From a neuropsychological point of view, the first cluster (Cluster 1) was associated with a dysexecutive memory pattern, exhibiting a low recall performance compensated for the recognition task. The second cluster (Cluster 2), representing a storage memory pattern, obtained low scores on all the subscales except for the face recognition task. The third cluster (Cluster 3) contained the best performers, characterized by a preserved memory pattern. The fourth cluster (Cluster 4) grouped subjects with the worst scores on all subscales, including the face recognition subscale, although with the greatest variability in scores (Fig. 2).
Fig. 1
UMAP [39] bidimensional projection of the variables used to perform the clustering. The intensity represents the probability of cluster membership given by the GMM.
![UMAP [39] bidimensional projection of the variables used to perform the clustering. The intensity represents the probability of cluster membership given by the GMM.](https://ip.ios.semcs.net:443/media/jad/2024/97-3/jad-97-3-jad230983/jad-97-jad230983-g001.jpg)
Table 5
Description of the sociodemographic variables, questionnaire, and scores obtained on the online FACEmemory for each of the empirical clusters identified by the unsupervised analysis (n = 3,000)
Variable | Cluster 1 | Cluster 2 | Cluster 3 | Cluster 4 | p1 |
Age (y) (mean, SD) | 50.42 (13.66) | 53.87 (15.13) | 47.40 (12.10) | 55.20 (14.47) | <0.0012 |
Years of schooling (mean, SD) | 13.95 (6.08) | 12.43 (5.10) | 14.74 (6.01) | 12.10 (5.93) | <0.0012 |
Sex4 (%) | 63.2 | 57.7 | 70.4 | 66.7 | 0.4593 |
Questionnaire completed5 (%) | 54.8 | 40.0 | 46.0 | 52.8 | 0.0023 |
Memory complaints6 (%) | 83.9 | 85.5 | 77.7 | 81.7 | >0.9993 |
Smoking6 (%) | 15.8 | 17.2 | 17.6 | 24.5 | >0.9993 |
Alcohol consumptionl6 (%) | 40.5 | 38.5 | 43.6 | 40.0 | >0.9993 |
Auditory abnormalities6 (%) | 20.9 | 19.7 | 17.6 | 26.4 | >0.9993 |
Visual abnormalities6 (%) | 40.5 | 38.3 | 38.2 | 42.3 | >0.9993 |
Hypertension6 (%) | 16.4 | 25.5 | 15.3 | 26.6 | <0.0013 |
Diabetes mellitus6 (%) | 5.6 | 6.6 | 4.1 | 9.2 | 0.6783 |
Dyslipidemia6 (%) | 27.0 | 30.2 | 25.7 | 31.0 | >0.9993 |
Cardiopathy6 (%) | 5.3 | 7.8 | 4.4 | 4.7 | 0.9493 |
Stroke6 (%) | 0.6 | 1.5 | 0.9 | 1.7 | >0.9993 |
Family history of vascular dementia6 (%) | 12.9 | 9.0 | 13.5 | 20.3 | 0.1833 |
Family history of AD/neurodegenerative | 42.7 | 35.0 | 47.6 | 41.1 | 0.0603 |
disease6 (%) | |||||
LN17 (mean, SD) | 1.82 (1.75) | 1.14 (1.34) | 4.51 (2.83) | 2.05 (2.62) | <0.0012 |
LO17 (mean, SD) | 5.09 (2.42) | 3.69 (2.32) | 7.14 (2.56) | 4.01 (2.95) | <0.0012 |
LN27 (mean, SD) | 5.58 (2.63) | 3.59 (2.77) | 9.75 (1.95) | 4.47 (3.72) | <0.0012 |
LO27 (mean, SD) | 8.06 (2.68) | 6.52 (2.71) | 10.14 (1.62) | 6.23 (3.54) | <0.0012 |
RSN7 (mean, SD) | 5.15 (2.70) | 3.26 (2.76) | 9.79 (1.89) | 4.40 (3.69) | <0.0012 |
RSO7 (mean, SD) | 7.97 (2.71) | 6.27 (2.73) | 10.35 (1.56) | 6.14 (3.72) | <0.0012 |
RLN7 (mean, SD) | 4.96 (2.71) | 3.08 (2.68) | 9.79 (1.93) | 4.16 (3.73) | <0.0012 |
RLO7 (mean, SD) | 7.87 (2.74) | 6.00 (2.79) | 10.21 (1.57) | 5.93 (3.69) | <0.0012 |
FR7 (mean, SD) | 12.00 (0.00) | 12.00 (0.00) | 12.00 (0.00) | 10.80 (1.41) | <0.0012 |
REN7 (mean, SD) | 10.24 (1.27) | 8.28 (2.78) | 12.00 (0.00) | 8.75 (3.12) | <0.0012 |
REO7 (mean, SD) | 12.00 (0.00) | 11.00 (0.84) | 12.00 (0.00) | 10.61 (2.17) | <0.0012 |
Duration in min (mean, SD) | 25.77 (2.39) | 28.02 (4.92) | 25.60 (2.11) | 31.68 (9.32) | <0.0012 |
1Bonferroni corrected p-value for multiple comparisons; 2ANOVA; 3 χ2-test; 4female = 1 and male = 0; 5version A = 1 and version B = 0; 6binary variable from the Open House Initiative questionnaire, where 1 indicates an affirmative answer and 0 indicates a negative answer; 7variable used for model clustering; LN1, names recalled in learning 1; LN2, names recalled in learning 2; LO1, occupations recalled in learning 1; LO2, occupations recalled in learning 2; RSN, names in short-term recall; RSO, occupations in short-term recall; RLN, names in long-term recall; RLO, occupations in long-term recall; FR: face recognition; REN, names correctly recognized; REO, occupations correctly recognized.
Fig. 2
Four patterns of memory performance identified by machine learning analysis. LN1, names recalled in learning 1; LN2, names recalled in learning 2; LO1, occupations recalled in learning 1; LO2, occupations recalled in learning 2; RLN, names in long-term recall; RLO, occupations in long-term recall; REN, names correctly recognized; REO, occupations correctly recognized; FR, face recognition.
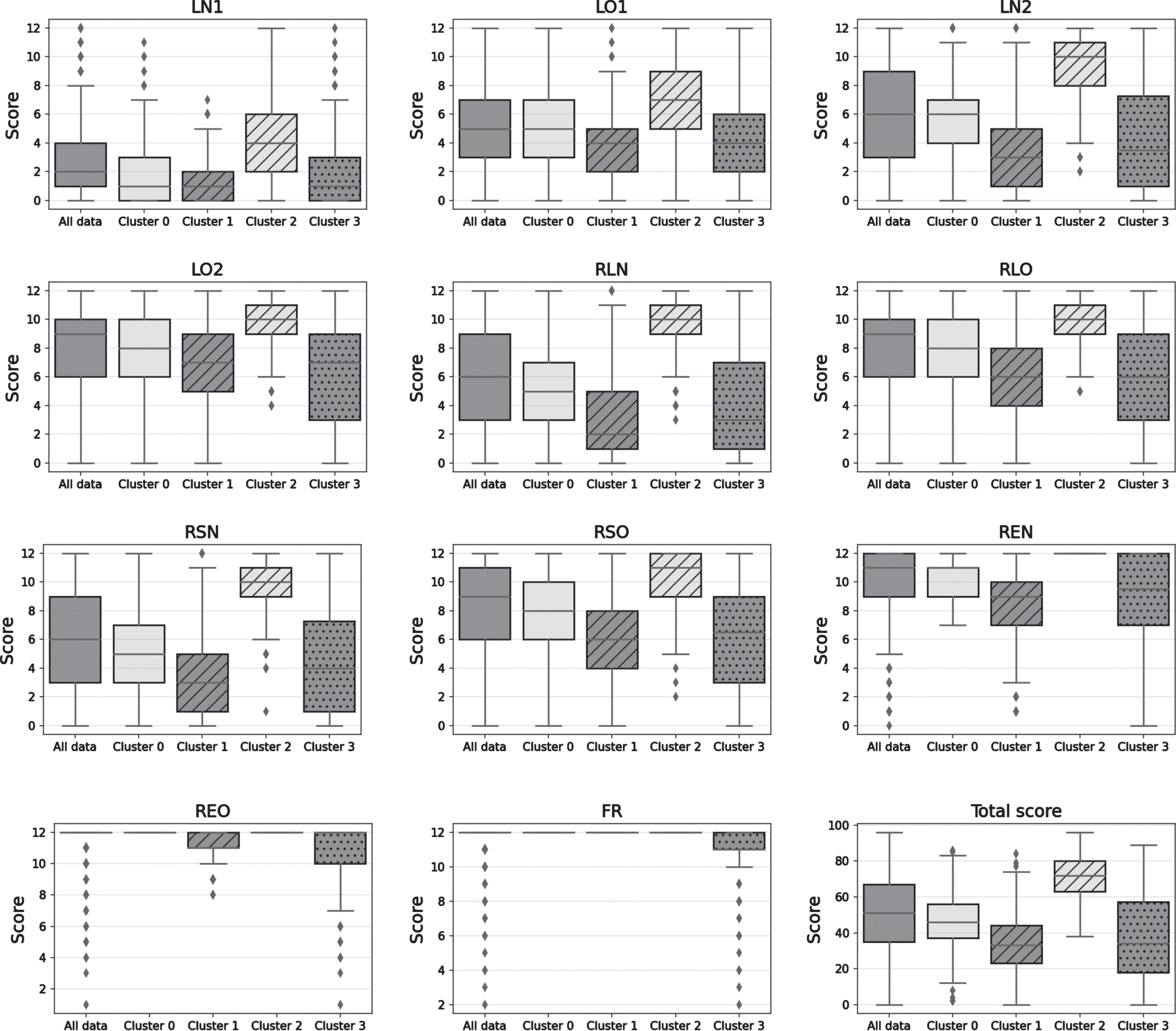
DISCUSSION
The results of the present study indicate that the FACEmemory online platform is a promising tool for Spanish- and Catalan-speaking individuals worldwide interested in assessing their memory, only needing a tablet or computer with internet access at home. To our knowledge, this is the first scientific study of a completely self-administered memory test with voice recognition, pre-tested in a memory clinic [11, 12], using information and communication technologies (ICTs), and offered freely worldwide on a website platform. The fact that 3,000 people older than 18 years from 37 different countries completed the FACEmemory test in just over 1.5 years, most of them with worries about their memory, makes FACEmemory a promising tool for memory pre-screening and early detection of cognitive impairment and AD.
The growing role of ICTs and the increasing availability of technologies worldwide offer a great chance to expand preventive healthcare systems [25]. Novel applications of ICTs to the early detection of cognitive impairment reduce cost, ease logistics, speed up recruitment, and reduce administrator bias compared with traditional paper and pencil tests [26], thus facilitating the identification of people in the community with hidden memory deficits [18]. Recently, Rentz et al. [9] reported a high correlation between performance on a computerized version of FNAME (face-name matching with a touch screen) [10] administered in the clinic and performance on the test when self-administered at home and monitored from the hospital, demonstrating the reliability and feasibility of remote testing [9].
Before the release of the version of the online FACEmemory platform that is completely self-administered at home, an audio test was added to verify microphone functionality and ensure the proper use of the voice button by the user. The previous self-administered version under minimal supervision of a neuropsychologist at the Ace Alzheimer Center Barcelona had been demonstrated to be a promising tool for the detection of MCI, mainly of the amnestic type [11]. Moreover, its automatic scoring was reliable, with a correlation value between automatic and manual total FACEmemory scores of close to 1 [11]. Additionally, FACEmemory proved to be sensitive to the early AD endophenotype and AD-related biomarkers [11]. That is, a worse performance on FACEmemory was found to be related to an impairment of memory storage, suitable for discriminating between cognitively healthy and amnestic MCI individuals, and to be associated with cerebrospinal fluid and magnetic resonance imaging AD biomarkers [11, 12].
Notably, 82.10% of individuals who completed the FACEmemory test reported subjective memory complaints with associated worries, which is relevant for identifying subjects in the community at risk of developing AD [27] and for targeted dementia prevention [28]. It is well known that age [1] and subjective cognitive decline (SCD) are important risk factors for developing objective cognitive impairment and AD dementia [29, 30]. Additionally, the presence of SCD “plus” criteria, which among others include concerns or worries associated with SCD, further increases the likelihood of preclinical AD in individuals with SCD [27]. Although the cross-sectional correlation between subjective and objective cognitive impairment is frequently poor [12, 24, 31], in the present study, individuals with subjective memory complaints who worried about them performed worse on FACEmemory than those without subjective memory complaints or who did not worry about them. This finding may be explained as follows. The subjective memory complaints were enriched with worries (that is, participants were asked whether they felt that their memory had worsened and whether they were worried about it), as recommended by Jessen et al. [32], and memory was assessed with a more complex test than the classical memory tests since associating unfamiliar faces with proper names is an arbitrary association [14], so this type of memory is strongly hippocampus dependent [11, 12].
When we applied the cut-off for impairment of 32 points obtained previously using FACEmemory on a tablet device under minimal supervision at the ACE Memory Unit [11], 20.40% of the study sample (612 out of 3,000) showed impaired performance. This group, in contrast with those with preserved performance on FACEmemory, had an older age, a lower level of schooling, a higher frequency of men and people from Spain, an increased percentage of hypertension, and a family history of neurodegenerative disease. These findings are consistent with recent reports about the global prevalence of MCI in the community being over 15% and associated with age, sex, schooling, and region of study sites [33].
In the whole sample, a worse performance on FACEmemory was associated with an older age, a lower level of schooling, being a man, having completed the test from outside Spain, and having failed to complete the medical and family history questionnaire in the required time of 13 min between short-term and long-term recall tasks; it was also related to the presence of subjective memory complaints with associated worries. Although the effects of age, schooling, and sex vary depending on the study, these results are consistent with those reporting that performance on the original 16-item and adapted versions of FNAME worsens with older age [8, 16, 34–36] and a lower level of schooling [16, 19, 20, 35–37] and that women score better than men [15, 20, 37]. Despite having a higher frequency of subjective memory complaints, people answering from Spain performed better on FACEmemory than those from outside Spain, maybe because this group was younger and had a higher frequency of women and also because FACEmemory was designed with images representative of the Spanish population [11]. Finally, the subset of individuals who completed the medical and family history questionnaire performed better on FACEmemory than those who did not, maybe because they were younger with a higher level of schooling. In addition, they may have been more motivated to assess their memory or had better global cognitive functioning, such as language comprehension and executive abilities, which may be needed to answer all the questions on the platform.
Memory function involves several cognitive processes (encoding after initial exposure, learning with repeated exposure, consolidation, and storage and retrieval, which depend on both recall and recognition) that are governed by different neural networks [24]. We therefore expected to observe different patterns of memory performance across the group of people performing the FACEmemory task, with the inclusion of people older than 18 years enhancing the scores of a cluster of normal or preserved performance. Using machine learning analysis, four patterns of memory performance were identified. The first pattern (Cluster 0, named dysexecutive memory pattern) corresponded to low scores on all tests, except for preserved face recognition, and showed better performance on recognition than recall. The second pattern (Cluster 1, named storage memory pattern) showed low scores on all subtests, except for face recognition—that is, impaired delayed recall without obtaining benefit from the recognition task. The third pattern (Cluster 2, named preserved memory performance) represented the best overall performers, with high scores on all subtests. The last pattern (Cluster 3, named severe memory impairment) showed the worst scores on all subtests, including face recognition, although it also had the greatest variability in scores. This last cluster probably included dementia cases, but it could also contain errors due to other causes (such as prosopagnosia, distractions, disinterest, etc.). These findings underscore the potential of machine learning techniques for the automatic and probabilistic detection of individuals at risk of developing cognitive impairment or AD [38] through the online FACEmemory platform.
The present study has limitations. Firstly, the study includes only data from Spanish- and Catalan-speaking individuals, so translation to other languages is needed. Although a well-functioning English version is now available worldwide, international marketing campaigns will be carried out to collect data from individuals who speak English, and other language versions will be needed to make FACEmemory a global tool. Secondly, limitations related to remote testing are inevitable, and features such as environmental distractions (e.g., noise, interruptions), help from other individuals, quality and size of the electronic device’s screen employed to perform the test, and the level of attention of the participants during the test have not been monitored. Although FACEmemory includes a video at the beginning emphasizing the need to pay attention and to perform the test in a place without any distractions, people who did not pay attention will have been classified as impaired, and future optimized versions might solve this problem by improving the test monitoring. Thirdly, the cut-off for impairment was obtained previously in a supervised self-administration study conducted in a Spanish memory clinic. Thus, it might be an inadequate cut-off for the online version, especially for those subjects who have completed the test outside Spain. Also, the lack of confirmation of the clinical diagnosis (cognitively healthy, MCI, or dementia) with a formal cognitive assessment in a memory clinic limits the interpretation of the current FACEmemory data. Finally, it was unknown whether individuals without any medical appointment or access to online platforms were reached.
The fact that the present study has given the opportunity to all people concerned about their memory from the age of 18 years to freely use FACEmemory opens a window for detecting dysfunctional memory patterns in younger and middle-aged people. Further studies are needed to validate these preliminary FACEmemory results, comparing the pattern of performance on FACEmemory with the diagnosis obtained with a formal cognitive assessment in a memory clinic to detect individuals in the community at risk of developing dementia. Furthermore, studies are needed to determine whether individuals with a storage or hippocampal memory pattern on FACEmemory end up developing AD and whether individuals belonging to the group with the best performance are indeed cognitively healthy. Since people older than 50 years are most at risk of developing AD, in a future study we are going to present standardized data and cut-offs of impaired performance using people older than 50 years who were diagnosed at the Memory Unit of the Ace Alzheimer Center Barcelona. Also, there is a need to establish novel specific (by age, sex, schooling, country, etc.) cut-off scores for the present online version of FACEmemory and to report their sensitivity and specificity values. Besides, the correlation of FACEmemory scores with AD-related biomarkers (CSF, magnetic resonance imaging, and genetics) and with the risk of future conversion to AD dementia needs to be analyzed.
In conclusion, the results of the present study confirm that FACEmemory is a promising tool for assessing memory in people with subjective memory complaints and for raising awareness about cognitive decline in the community. Taking advantage of the growing interest in the use of new technologies, the FACEmemory online platform has been demonstrated to be an opportunity for facilitating memory assessment. We are convinced that giving people the opportunity to complete the test at home at the moment they prefer allows them to perform their best. Moreover, due to the complexity of the FACEmemory test, it will allow the detection of individuals with milder cognitive impairment and AD compared with classic neuropsychological assessments, which is crucial for early prevention strategies focused on applying precision medicine to slow down the progress of cognitive decline and to reduce the risk of dementia.
ACKNOWLEDGMENTS
We acknowledge all individuals who completed FACEmemory® online; without their collaboration, this work would not have been accomplished. The Ace Alzheimer Center Barcelona is a member of the Centro de Investigación Biomédica en Red sobre Enfermedades Neurodegenerativas (CIBERNED), Instituto de Salud Carlos III, Ministerio de Ciencia, Innovación y Universidades. We also want to thank Raul Espinosa for his support and persistence in finding a way to automate and optimize the tool; Xavier Manrubia from Editorial Glosa; Pau Plana and Martin Dans from 3iPunt Solucions Informàtiques; and all the participating investigators and personnel from the Ace Alzheimer Center Barcelona for their close collaboration and continuous intellectual input.
FUNDING
This work was funded by research funds of the Ace Alzheimer Center Barcelona and was partially supported by funding from the Instituto de Salud Carlos III (ISCIII) Acción Estratégica en Salud, integrated into the Spanish National RCDCI Plan and financed by ISCIII-Subdirección General de Evaluación and the Fondo Europeo de Desarrollo Regional (FEDER) grants PI22/01403 and PI19/00335, as well as by some sponsors (Grifols SA, Life Molecular Imaging GmbH, Araclon Biotech, Laboratorios Echevarne S.A., and the Ace Alzheimer Center Barcelona). MB, AR, and MM acknowledge the support of the Spanish ISCIII, Acción Estratégica en Salud, integrated into the Spanish National R + D+I Plan and financed by the ISCIII Subdirección General de Evaluación and the Fondo Europeo de Desarrollo Regional (FEDER “Una manera de hacer Europa”) grants PI13/02434, PI16/01861, PI17/01474, PI19/01240, PI19/01301, PI19/00335, and PI22/00258 and the ISCIII national grant PMP22/00022, funded by the European Union (NextGenerationEU). We also acknowledge the support of CIBERNED (ISCIII) under the grants CB06/05/2004 and CB18/05/00010. Further, we are grateful for the support from the ADAPTED and MOPEAD projects, European Union/EFPIA Innovative Medicines Initiative Joint (grant numbers 115975 and 115985, respectively); the support from the PREADAPT project, Joint Program for Neurodegenerative Diseases (JPND) grant No AC19/00097; and the support from the HARPONE project, Agency for Innovation and Entrepreneurship (VLAIO) grant No PR067/21, and Janssen. The DESCARTES project is funded by the German Research Foundation (DFG).
CONFLICT OF INTEREST
The authors declare that they have no conflict of interest to report. Some of the authors (MA, SV, AR, and MB) are Editorial Board Members of this journal, but they were neither involved in the peer review process nor had access to any information regarding the manuscript’s peer review.
REFERENCES
[1] | ((2023) ) Alzheimer’s disease facts and figures. Alzheimers Dement 19: , 1598–1695. |
[2] | Mowszowski L , Batchelor J , Naismith SL ((2010) ) Early intervention for cognitive decline: Can cognitive training be used as a selective prevention technique? Int Psychogeriatr 22: , 537–548. |
[3] | Livingston G , Huntley J , Sommerlad A , Ames D , Ballard C , Banerjee S , Brayne C , Burns A , Cohen-Mansfield J , Cooper C , Costafreda SG , Dias A , Fox N , Gitlin LN , Howard R , Kales HC , Kivimäki M , Larson EB , Ogunniyi A , Orgeta V , Ritchie K , Rockwood K , Sampson EL , Samus Q , Schneider LS , Selbæk G , Teri L , Mukadam N ((2020) ) Dementia prevention, intervention, and care: 2020 report of the Lancet Commission. Lancet 396: , 413–446. |
[4] | Rahman A , Hossen MA , Chowdhury MFI , Bari S , Tamanna N , Sultana SS , Haque SN , Al Masud A , Saif-Ur-Rahman KM ((2023) ) Aducanumab for the treatment of Alzheimer’s disease: a systematic review. Psychogeriatrics 23: , 512–522. |
[5] | Stahl BC , Bitsch L ((2021) ) Building a responsible innovation toolkit as project legacy. Front Res Metr Anal 88: , 1112106. |
[6] | Robbins TW , James M , Owen AM , Sahakian BJ , McInnes L , Rabbitt P ((1994) ) Cambridge neuropsychological test automated battery (CANTAB): a factor analytic study of a large sample of normal elderly volunteers. Dementia 5: , 266–281. |
[7] | Mielke MM , Machulda MM , Hagen CE , Edwards KK , Roberts RO , Pankratz VS , Knopman DS , Jack CRJ , Petersen RC ((2015) ) Performance of the CogState computerized battery in the Mayo Clinic Study on Aging. Alzheimers Dement 11: , 1367–1376. |
[8] | Ding Z , Lee TL , Chan AS ((2022) ) Digital cognitive biomarker for mild cognitive impairments and dementia: a systematic review. J Clin Med 11: , 4191. |
[9] | Rentz DM , Dekhtyar M , Sherman J , Burnham S , Blacker D , Aghjayan SL , Papp K V , Amariglio RE , Schembri A , Chenhall T , Maruff P , Aisen P , Hyman BT , Sperling RA ((2016) ) The feasibility of at-home iPad cognitive testing for use in clinical trials. J Prev Alzheimers Dis 3: , 8–12. |
[10] | Papp KV , Rentz DM , Maruff P , Sun CK , Raman R , Donohue MC , Schembri A , Stark C , Yassa MA , Wessels AM , Yaari R , Holdridge KC , Aisen PS , Sperling RA ((2021) ) The computerized cognitive composite (C3) in A4, an Alzheimer’s disease secondary prevention trial. J Prev Alzheimers Dis 8: , 59–67. |
[11] | Alegret M , Muñoz N , Roberto N , Rentz DM , Valero S , Gil S , Marquié M , Hernández I , Riveros C , Sanabria A , Perez-Cordon A , Espinosa A , Ortega G , Mauleón A , Abdelnour C , Rosende-Roca M , Papp KV , Orellana A , Benaque A , Tarraga L , Ruiz A , Boada M ((2020) ) A computerized version of the Short Form of the Face-Name Associative Memory Exam (FACEmemory®) for the early detection of Alzheimer’s disease. Alzheimers Res Ther 12: , 25. |
[12] | Alegret M , Sotolongo-Grau O , de Antonio EE , Pérez-Cordón A , Orellana A , Espinosa A , Gil S , Jiménez D , Ortega G , Sanabria A , Roberto N , Hernández I , Rosende-Roca M , Tartari JP , Alarcon-Martin E , de Rojas I , Montrreal L , Morató X , Cano A , Rentz DM , Tárraga L , Ruiz A , Valero S , Marquié M , Boada M ((2022) ) Automatized FACEmemory® scoring is related to Alzheimer’s disease phenotype and biomarkers in early-onset mild cognitive impairment: the BIOFACE cohort. Alzheimers Res Ther 14: , 43. |
[13] | Espinosa A , Alegret M , Valero S , Vinyes-Junqué G , Hernández I , Mauleón A , Rosende-Roca M , Ruiz A , López O , Tárraga L , Boada M ((2013) ) A longitudinal follow-up of 550 mild cognitive impairment patients: evidence for large conversion to dementia rates and detection of major risk factors involved. J Alzheimers Dis 34: , 769–780. |
[14] | Werheid K , Clare L ((2007) ) Are faces special in Alzheimer’s disease? Cognitive conceptualisation, neural correlates, and diagnostic relevance of impaired memory for faces and names. Cortex 43: , 898–906. |
[15] | Rentz DM , Amariglio RE , Becker JA , Frey M , Olson LE , Frishe K , Carmasin J , Maye JE , Johnson KA , Sperling RA ((2011) ) Face-name associative memory performance is related to amyloid burden in normal elderly. Neuropsychologia 49: , 2776–2783. |
[16] | Alegret M , Rodríguez O , Espinosa A , Ortega G , Sanabria A , Valero S , Hernández I , Rosende-Roca M , Vargas L , Abdelnour C , Mauleón A , Gailhajanet A , Martín E , Tárraga L , Rentz DM , Amariglio RE , Ruíz A , Boada M ((2015) ) Concordance between subjective and objective memory impairment in volunteer subjects. J Alzheimers Dis 48: , 1109–1117. |
[17] | Abdelnour C , Rodríguez-Gómez O , Alegret M , Valero S , Moreno-Grau S , Sanabria Á , Hernández I , Rosende-Roca M , Vargas L , Mauleón A , Sánchez D , Espinosa A , Ortega G , Pérez-Cordón A , Diego S , Gailhajanet A , Guitart M , Sotolongo-Grau Ó , Ruiz A , Tárraga L , Boada M ((2017) ) Impact of recruitment methods in subjective cognitive decline. J Alzheimers Dis 57: , 625–632. |
[18] | Rodrigo A , Trigueros P , Jamilis L , Alegret M , Rodríguez O , Tárraga L , González-Pérez A , Kramberger M , Winblad B , Visser PJ , Jessen F , Campo L , Boada M , Valero S , Rodriguez I , Gurruchaga M , Iradier F , Belger M , Dumas A , Shering C , Maguire P , Semancik K , Garro IP , Johansson G , Wimo A , Zlobec S , Krivec D , Sannemann L , Bon J , Simo R , Cuidin A , Zwan M , Vermunt L , Stoekenbroek M , Waterink L , Georges J ((2021) ) Identification of undiagnosed dementia cases using a web-based pre-screening tool: the MOPEAD project. Alzheimers Dement 17: , 1307–1316. |
[19] | Papp KV , Amariglio RE , Dekhtyar M , Roy K , Wigman S , Bamfo R , Sherman J , Sperling RA , Rentz DM ((2014) ) Development of a psychometrically equivalent short form of the face-name associative memory exam for use along the early Alzheimer’s disease trajectory. Clin Neuropsychol 28: , 771–785. |
[20] | Alegret M , Valero S , Ortega G , Espinosa A , Sanabria A , Hernández I , Rodríguez O , Rosende-Roca M , Mauleón A , Vargas L , Martín E , Ruíz A , Tárraga L , Amariglio RE , Rentz DM , Boada M ((2015) ) Validation of the Spanish version of the face name associative memory exam (S-FNAME) in cognitively normal older individuals. Arch Clin Neuropsychol 30: , 712–720. |
[21] | Buitinck L , Louppe G , Blondel M , Pedregosa F , Mueller A , Grisel O , Niculae V , Prettenhofer P , Gramfort A , Grobler J , Layton R , Vanderplas J , Joly A , Holt B , Varoquaux G (2013) API design for machine learning software: experiences from the scikit-learn project. arXiv:1309.0238.https://doi.org/10.48550/arXiv.1309.0238 |
[22] | Schwarz G ((1978) ) Estimating the dimension of a model. Ann Statist 6: , 461–464. |
[23] | Lopez OL , Becker JT , Wahed AS , Saxton J , Sweet RA , Wolk DA , Klunk W , DeKosky ST ((2009) ) Long-term effects of the concomitant use of memantine with cholinesterase inhibition in Alzheimer disease. J Neurol Neurosurg Psychiatry 80: , 600–607. |
[24] | Wolk DA , Dickerson BC ((2011) ) Fractionating verbal episodic memory in Alzheimer’s disease. Neuroimage 54: , 1530–1539. |
[25] | Williams F , Oke A , Zachary I ((2019) ) Public health delivery in the information age: the role of informatics and technology. Perspect Public Health 139: , 236–254. |
[26] | Feenstra HEM , Vermeulen IE , Murre JMJ , Schagen SB ((2017) ) Online cognition: factors facilitating reliable online neuropsychological test results. Clin Neuropsychol 31: , 59–84. |
[27] | Jessen F ((2014) ) Subjective and objective cognitive decline at the pre-dementia stage of Alzheimer’s disease. Eur Arch Psychiatry Clin Neurosci 264: , 3–7. |
[28] | Vellas B , Aisen PS , Sampaio C , Carrillo M , Scheltens P , Scherrer B , Frisoni GB , Weiner M , Schneider L , Gauthier S , Gispen-de Wied CC , Hendrix S , Feldman H , Cedarbaum J , Petersen R , Siemers E , Andrieu S , Prvulovic D , Touchon J , Hampel H ((2011) ) Prevention trials in Alzheimer’s disease: an EU-US task force report. Prog Neurobiol 95: , 594–600. |
[29] | Jessen F , Amariglio RE , van Boxtel M , Breteler M , Ceccaldi M , Chetelat G , Dubois B , Dufouil C , Ellis KA , van der Flier WM , Glodzik L , van Harten AC , de Leon MJ , McHugh P , Mielke MM , Molinuevo JL , Mosconi L , Osorio RS , Perrotin A , Petersen RC , Rabin LA , Rami L , Reisberg B , Rentz DM , Sachdev PS , de la Sayette V , Saykin AJ , Scheltens P , Shulman MB , Slavin MJ , Sperling RA , Stewart R , Uspenskaya O , Vellas B , Visser PJ , Wagner M; Subjective Cognitive Decline Initiative (SCD-I) Working Group((2014) ) A conceptual framework for research on subjective cognitive decline in preclinical Alzheimer’s disease. Alzheimers Dement10: ,844–852. |
[30] | Mitchell AJ , Beaumont H , Ferguson D , Yadegarfar M , Stubbs B ((2014) ) Risk of dementia and mild cognitive impairment in older people with subjective memory complaints: meta-analysis. Acta Psychiatr Scand 130: , 439–451. |
[31] | Jungwirth S , Fischer P , Weissgram S , Kirchmeyr W , Bauer P , Tragl K-H ((2004) ) Subjective memory complaints and objective memory impairment in the Vienna–Transdanube aging community. J Am Geriatr Soc 52: , 263–268. |
[32] | Jessen F , Wiese B , Bachmann C , Eifflaender-Gorfer S , Haller F , Kölsch H , Luck T , Mösch E , van den Bussche H , Wagner M , Wollny A , Zimmermann T , Pentzek M , Riedel-Heller SG , Romberg H-P , Weyerer S , Kaduszkiewicz H , Maier W , Bickel H ((2010) ) Prediction of dementia by subjective memory impairment: effects of severity and temporal association with cognitive impairment. Arch Gen Psychiatry 67: , 414–422. |
[33] | Bai W , Chen P , Cai H , Zhang Q , Su Z , Cheung T , Jackson T , Sha S , Xiang Y-T ((2022) ) Worldwide prevalence of mild cognitive impairment among community dwellers aged 50 years and older: a meta-analysis and systematic review of epidemiology studies.afac. Age Ageing 51: , 173. |
[34] | Kormas C , Megalokonomou A , Zalonis I , Evdokimidis I , Kapaki E , Potagas C ((2018) ) Development of the Greek version of the Face Name Associative Memory Exam (GR-FNAME12) in cognitively normal elderly individuals. Clin Neuropsychol 4046: , 1–12. |
[35] | Vila-Castelar C , Papp K V , Amariglio RE , Torres VL , Baena A , Gomez D , Rendon J , Samaroo A , Lopera F , Rentz DM , Quiroz YT ((2020) ) Validation of the Latin American Spanish version of the face-name associative memory exam in a Colombian sample. Clin Neuropsychol 34: (sup1), 1–12. |
[36] | Amariglio RE , Frishe K , Olson LE , Wadsworth LP , Lorius N , Sperling RA , Rentz DM ((2012) ) Validation of the Face Name Associative Memory Exam in cognitively normal older individuals. J Clin Exp Neuropsychol 34: , 580–587. |
[37] | Alviarez-Schulze V , Cattaneo G , Pachón-García C , Solana-Sánchez J , Tormos-Muñoz JM , Alegret M , Pascual-Leone A , Bartrés-Faz D ;Barcelona Brain Health Initiative group ((2021) ) Validation and normative data of the Spanish version of the Face Name Associative Memory Exam (S-FNAME).J Int Neuropsychol Soc 28: , 74–84. |
[38] | Myszczynska MA , Ojamies PN , Lacoste AMB , Neil D , Saffari A , Mead R , Hautbergue GM , Holbrook JD , Ferraiuolo L ((2020) ) Applications of machine learning to diagnosis and treatment of neurodegenerative diseases. Nat Rev Neurol 16: , 440–456. |
[39] | McInnes L , Healy J , Melville J (2018) UMAP: Uniform manifold approximation and projection for dimension reduction. arXiv:1802.03426.https://doi.org/10.48550/arXiv.1802.03426 |