Bioinformatics-Based Analysis of Circadian Rhythm Regulation Mechanisms in Alzheimer’s Disease
Abstract
Background:
There is a close association between Alzheimer’s disease (AD) and circadian rhythms, and neuroinflammatory-related pathways are associated with both interactions.
Objective:
To reveal the relationship between circadian rhythm (CR) and AD at the level of genes, pathways, and molecular functions through bioinformatics.
Methods:
We analyzed the differential genes between AD and control groups in GSE122063 and found the important gene modules; obtained CR-related genes from GeenCard database; used Venn 2.1 database to obtain the intersection of genes of AD important modules with CR-related genes; and used STRING database and Cytoscape 3.7.1 to construct the gene protein-protein interaction network. The MCODE plugin was used to screen pivotal genes and analyze their differential expression. We trranslated with www.DeepL.com/Translator (free version) to obtain transcriptional regulatory relationships from the TRRUST database and construct a hub gene-transcription factor relationship network.
Results:
A total of 42 common genes were screened from AD and CR genes, mainly involving signaling pathways such as neuroactive ligand-receptor interactions. A total of 10 pivotal genes were screened from the common genes of CR and AD, which were statistically significant in the comparison of AD and control groups (p < 0.001), and ROC analysis showed that all these pivotal genes had good diagnostic significance. A total of 36 TFs of pivotal genes were obtained.
Conclusion:
We identified AD- and CR-related signaling pathways and 10 hub genes and found strong associations between these related genes and biological processes such as inflammation.
INTRODUCTION
Alzheimer’s disease is a chronic and progressive decline in cognitive function, mainly manifested by cognitive decline, decline in daily living ability and mental behavior abnormalities, which seriously endangers the health of the elderly. Its pathogenesis is closely related to genetics, aging, lifestyle, environmental factors, and nervous system diseases [1–4], but so far, the etiology and pathogenesis of AD is not clear. Although researchers have proposed the hypothesis of amyloid-β (Aβ) cascade damage [5], tau hyperphosphorylation [5], cholinergic injury hypothesis [6], the ApoE hypothesis [7], neuroinflammatory hypothesis [8], and mitochondrial dysfunction hypothesis [9] for AD, they have yet to fully explain the complex mechanism of this disease. The prevalence of AD continues to rise, seriously affecting healthcare systems worldwide [10].
The complex connection between the body’s intrinsic biological rhythms and AD has recently attracted a lot of attention. The “sunset phenomenon” is a symptom of a distinct temporal pattern in AD, manifested by symptoms such as hallucinations, hallucinations, emotional instability, anxiety, and cognitive impairment at dusk, lasting several hours in mild cases or affecting sleep in severe cases [11]. Circadian rhythm (CR) is a biological timer [12], a 24-hour physiological and behavioral cycle generated by endogenous biological clock oscillations, which can regulate the biological processes of the body at different levels, such as neural, molecular, and cellular [13]. Sunset syndrome in AD patients is both one of the symptoms of circadian rhythm disorder and aggravated by circadian rhythm disorder [14, 15]. There is an intricate relationship between AD and CR. On the one hand, Aβ42 production and secretion increase when the body is awake, while Aβ clearance increases and production decreases during sleep, and sleep in the elderly mostly shows an increase in daytime sleep fragmentation and a decrease in nighttime sleep [16, 17]. Sleep deprivation also increases tau protein in the interstitial fluid, and its hyperphosphorylation may destabilize the microtubule system, leading to neurogenic fiber tangles and neurodegeneration of neuronal synaptic terminals [18], causing the onset of AD. Interestingly, melatonin correction of the sleep/wake cycle has achieved positive results in improving sunset/agitation behavior in patients with dementia [19]. On the other hand, clinical studies have shown a significant shortening of rapid eye movement (REM) sleep and increased fragmentation of slow-wave sleep in AD patients, which may be caused by a decrease in cholinergic activity in AD [20–22].
The intrinsic mechanism link between AD and CR is not yet clear, and bioinformatics has a bright future in the analysis of disease differential genes and the screening of important pivotal genes and pathways. We use bioinformatics to analyze biomarkers and pathways commonly associated with AD and CR, and to point out new directions for future research in the prevention and treatment of AD from correcting circadian rhythm disorders.
METHODS
Acquisition of genes
Differential genes in AD
Using “GSE122063” as the search term, we obtained the differential genes between the AD group and the control group in this study from the GEO database [23] (https://www.ncbi.nlm.nih.gov/geo/), removed the duplicate genes, and established the AD differential gene database. A volcano diagram was used to visualize the differential genes that were screened based on gene expression.
Construction of co-expression network and identification of key modules
WGCNA was used to explore gene modules highly related to the external traits of the samples. The weighted gene co-expression network of differentially expressed genes (DEGs) in our study was constructed through the R package WGCNA (V1.69). First, we calculated the MAD (Median Absolute Deviation) of each gene in the gene expression profile separately, eliminated the top 50% of genes with the smallest MAD, used the good Samples Genes method of the R package WGCNA to eliminate outlier genes and samples, and further used WGCNA to construct a scale-free co-expression network. To classify genes with similar expression profiles into gene modules, average linkage hierarchical clustering was performed based on the TOM-based dissimilarity measure with a minimum gene dendrogram size (genome) of 30, setting the sensitivity to 3. To further analyze the modules, we calculated the dissimilarity of the module feature genes, selected a cut line for the module dendrogram, and merged some modules. In addition, we merged modules with distance less than 0.25, and finally obtained 3 co-expression modules. It is worth noting that gray modules are considered as the set of genes that cannot be assigned to any module.
After identifying significant interest of modules, gene significance (GS) and module membership (MM) were calculated for each gene. In WGCNA, MM refers to the correlation between genes and gene expression profiles. Hub genes are a subset of highly interconnected genes (nodes) within key modules of co-expression network and are significantly associated with biological functions.
Targets of CR
The CR-related genes were retrieved from the GeneCard database (https://www.genecards.org/) [24] using the search term “circadian rhythm,” and a CR gene database was created.
Targets for CR intervention in AD
Using Venny2.1(http://bioinformatics.psb.ugent.be/webtools/Venn/), the AD differential genes obtained earlier were intersected with the CR-related genes to create a gene database for CR interventions in AD, and the corresponding Venn diagrams were plotted.
Target analysis and enrichment analysis
Protein-protein interaction (PPI) network construction of key genes
Common genes were imported into String database [25] (https://cn.string-db.org/) to obtain protein interactions; the obtained protein information was imported into Cytoscape 3.7.2 software to construct protein-protein interaction networks and calculate the Degree values of nodes, and adjust the nodes in the network according to the Degree values. The PPI network was generated by adjusting the properties of the nodes in the network according to the Degree value.
KEGG signaling pathway enrichment analysis
The genes of CR intervention AD were uploaded to the Metascape platform (https://metascape.org/gp/index.html#/main/step1) [26] for Kyoto Encyclopedia of Genes and Genomes (KEGG) pathway enrichment analysis (p < 0.05), and the top 20 signaling pathways were filtered according to p-value to draw bubble maps for visualization.
Gene ontology analysis
The genes of CR intervention AD were uploaded to the Metascape platform for Gene ontology (GO) analysis enrichment analysis (p < 0.05), and the enrichment results of molecular function, biological process, and cellular component were obtained.
Hub genetic analysis
Screening of HUB targets and construction of PPI networks
Using cytohHubba plug-in and MCODE plug-in in Cytoscape 3.7.2 [27], the top 10 differential genes were screened as hub genes based on EPC from the results obtained above, and the PPI network maps of the hub genes were drawn.
Differential expression of Hub genes in AD
The expression of the hub genes in GSE122063 was downloaded from the GEO database for each sample in the AD and Control groups, respectively. Cluster analysis of the hub genes was performed and box plots of the differences in expression of the different genes within the two groups were plotted separately. The diagnostic and discriminant values of DEGs in the AD group and control group were evaluated by receiver operating characteristic curve analysis. GSE122063 datasets were used as the external validation datasets.
ROC curve analysis of hub genes
ROC analysis was performed using the R package pROC (version 1.17.0.1) to obtain AUC, and the ci function of pROC was used to evaluate AUC and confidence intervals to obtain the final AUC results.
KEGG and GO analysis of HUB gene
KEGG signaling pathway and GO analysis of hub genes using Metascape, and visualization of results.
Analysis of hub gene-associated transcription factor
Each of the 10 HUB genes was imported into Transcriptional Regulatory Relationships Unraveled by Sentence-base Text mining database (TRRUST) [28] (https://www.grnpedia.org/trrust/) to obtain the transcription factors (TFs) of HUB genes. Then Cytoscape 3.7.2 was applied, and the hub gene-IF network was constructed.
Statistical analysis
In the above parts, it is considered that the research results meet p < 0.05 was statistically significant.
RESULTS
Acquisition of gene
In the GEO database GSE122063 study, 56 samples in the AD group and 44 samples in the Control group, and 14,200 differential genes were obtained between the AD and Control groups, volcano plot in Fig. 1A, and the sample clustering plot in Fig. 1B. A total of 2,320 CR-related genes were obtained.
Fig. 1
A) Differentially expressed genes between the blank and Alzheimer’s disease groups in the GSE122063 study in the GEO database; B) sample information.
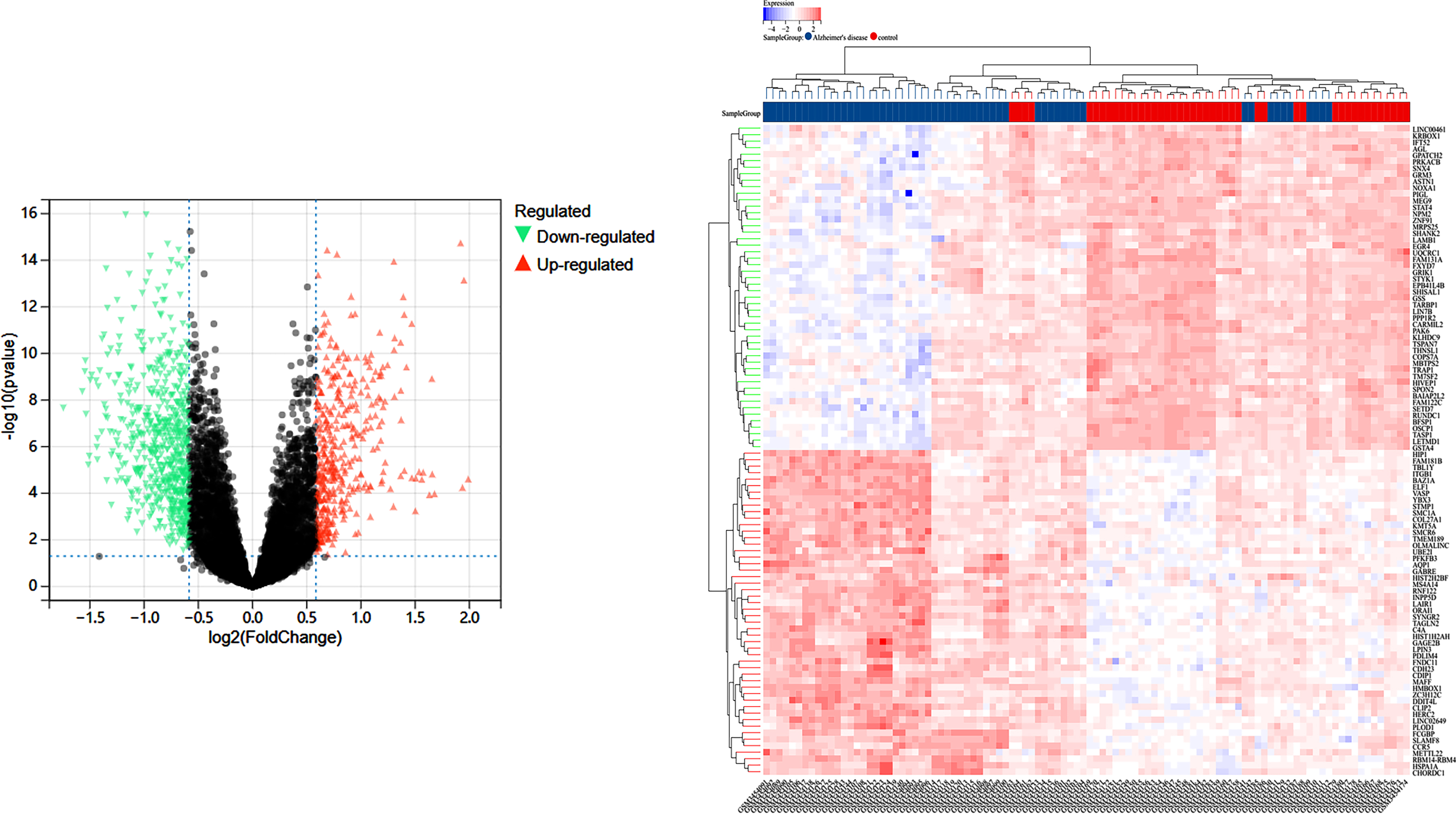
WCGNA analysis and module significance calculation
The gene expression matrix of 14,200 pretreatment genes from AD patients in the GEO dataset was used for the WGCNA of the R package. Sample clustering is shown in Fig. 2A. To ensure high scale independence (0.85), we set β= 10 in this analysis (Fig. 2B). To ensure average connectivity (15.24), we set β= 10 in this analysis (Fig. 2C). Based on the average hierarchical clustering and dynamic tree clipping, we obtained a total of 9 co-expression modules, module feature vector clustering (Fig. 2E), module-phenotype correlation heat map (Fig. 2D), turquoise module’s GS and MM correlation scatter plot (Fig. 2F).
Fig. 2
A) sample clustering; B) Scalar independence; C) average connectivity; D) module-phenotype correlation heat map; E) module feature vector clustering; F) turquoise module’s GS and MM correlation scatter plot.
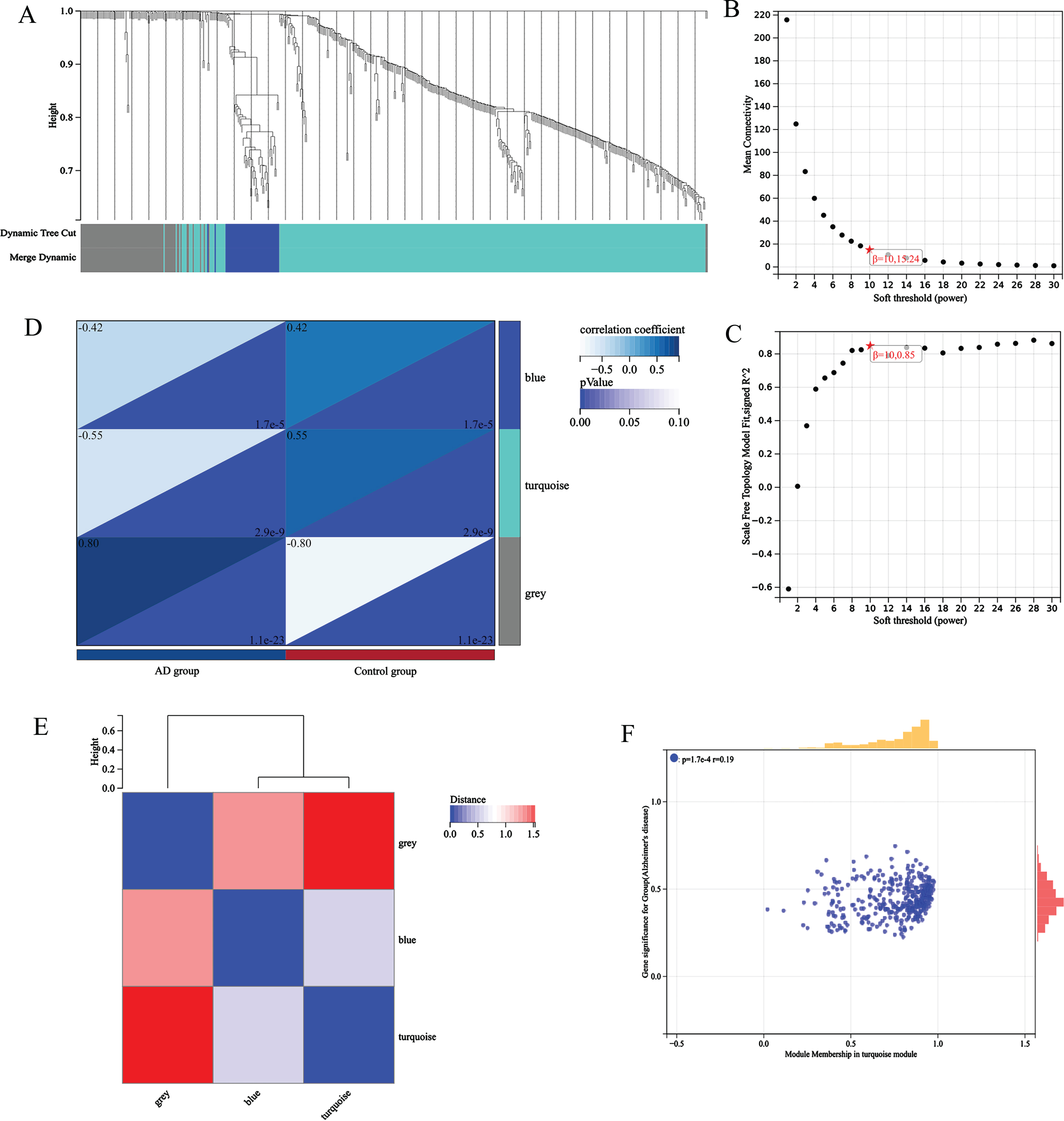
Screening of common targets and construction of PPI networks
A total of 381 AD-related genes were obtained in the turquoise module and intersected with 2,320 CR-related genes to obtain 42 key genes acting on both AD and CR, Venn diagram (Fig. 3A) and PPI network diagram (Fig. 3B).
Fig. 3
A) Venn diagram of the intersection of differential genes and circadian rhythm-associated genes in Alzheimer’s disease; B) PPI network diagram of intersecting genes; C) PPI network diagram of 10 hub genes.
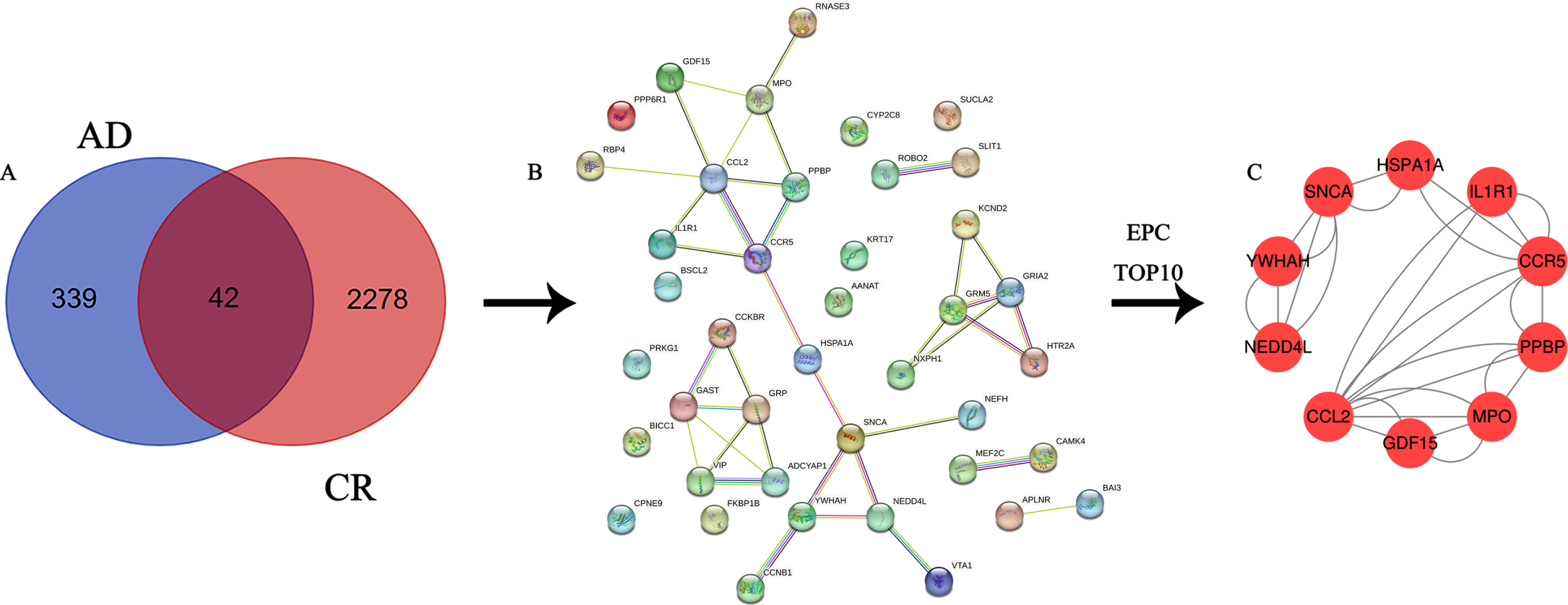
Enrichment of the KEGG signaling pathway
Analysis of DEGs by Metascape revealed a total of 42 signaling pathways enriched by CR and AD common targets p < 0.05, mainly including Neuroactive ligand-receptor interaction, Cytokine-cytokine receptor interaction, Viral protein interaction with cytokine and cytokine receptor, Chemokine signaling pathway, Human cytomegalovirus infection, etc. (Fig. 4A).
Fig. 4
A) Bubble diagram of KEGG signaling pathway; B) bar graph of Gene Ontology analysis diagram.
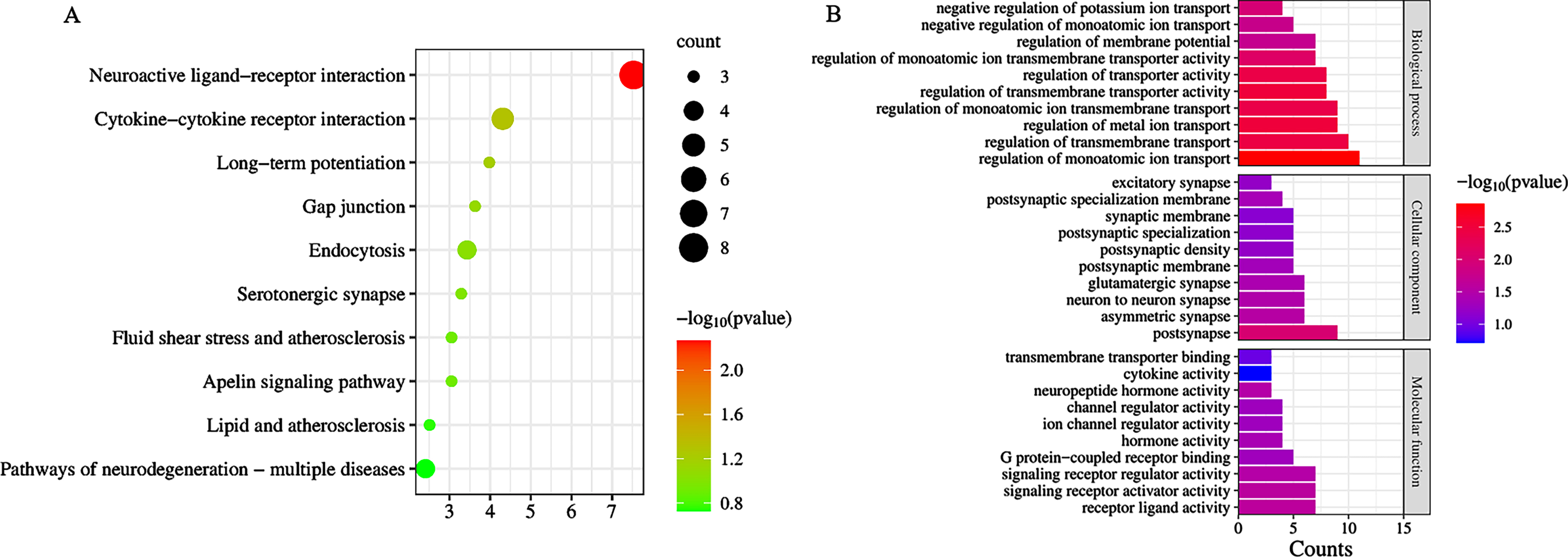
Enrichment of the GO
Analysis of DEGs by Metascape revealed a total of 289 items (p < 0.05). There were 19 molecular functions, including receptor ligand activity, signaling receptor activator activity, signaling receptor regulator activity, neuropeptide hormone activity; 23 cellular components, including postsynapse, asymmetric synapse, neuron to neuron synapse, glutamatergic synapse, postsynaptic specialization membrane; and 247 biological processes, including regulation of monoatomic ion transport, regulation of metal ion transport, regulation of transmembrane transporter activity, regulation of transmembrane transport, regulation of transporter activity; the top 10 p-values in each of the three groups were selected for visualization (Fig. 4B).
Hub genetic analysis
Hub target screening
A total of 10 hub genes were screened in this study, included CCL2, CCR5, GDF15, HSPA1A, IL1R1, MPO, NEDD4L, PPBP, SNCA, and YWHAH. The specific information calculated by Cytoscape 3.7.2 is shown in Table 1.
Table 1
Table1
Name | Degree | Average Shortest PathLength | Betweenness Centrality | Closeness Centrality | Clustering Coefficient | Neighborhood Connectivity | Number Of Directed Edges | Radiality |
CCL2 | 6 | 2.5 | 0.362637 | 0.4 | 0.266667 | 2.666667 | 6 | 0.785714 |
MPO | 4 | 3.142857 | 0.148352 | 0.318182 | 0.333333 | 3 | 4 | 0.693878 |
CCR5 | 4 | 2.285714 | 0.543956 | 0.4375 | 0.333333 | 3.25 | 4 | 0.816327 |
SNCA | 4 | 2.571429 | 0.538462 | 0.388889 | 0.166667 | 2.25 | 4 | 0.77551 |
PPBP | 3 | 2.714286 | 0.087912 | 0.368421 | 0.666667 | 4.666667 | 3 | 0.755102 |
YWHAH | 3 | 3.214286 | 0.142857 | 0.311111 | 0.333333 | 2.666667 | 3 | 0.683673 |
NEDD4L | 3 | 3.214286 | 0.142857 | 0.311111 | 0.333333 | 2.666667 | 3 | 0.683673 |
GDF15 | 2 | 3.285714 | 0 | 0.304348 | 1 | 5 | 2 | 0.673469 |
IL1R1 | 2 | 2.857143 | 0 | 0.35 | 1 | 5 | 2 | 0.734694 |
HSPA1A | 2 | 2.357143 | 0.527473 | 0.424242 | 0 | 4 | 2 | 0.806122 |
The PPI network of Hub genes
A total of 10 nodes and 28 edges were obtained from the analysis of the interrelationships of the 10 hub genes by the string database and Cytoscape 3.7.2 software (Fig. 3C).
Differential expression of HUB gene
We determined the differential expression levels by comparing Hub gene expression between the AD group and the control group in the GSE122063 disease gene pool (Fig. 5A). Box plots showed significant differences in the levels of all 10 genes between the AD and control groups (Fig. 5B).
Fig. 5
A) Heat map of DGEs expression; B) Differential expression of 10 central genes in AD group and Control groups (a: HSPA1A; b: NEDD4L; c: CCL2; d: PPBP; e: CCR5; f: MPO; g: GDF15; h: YWHAH; i: IL1R1; j: SNCA).
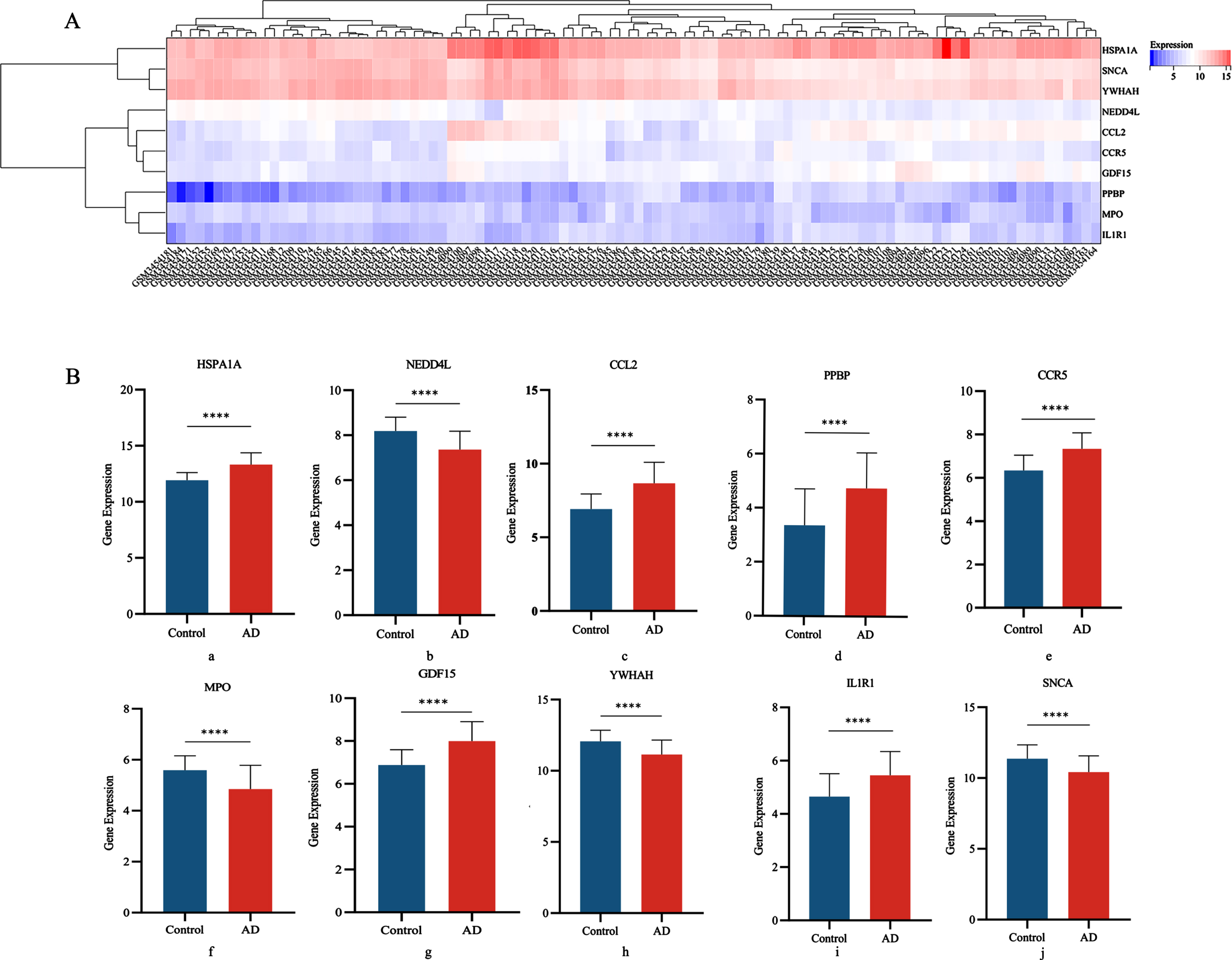
ROC analysis of HUB gene
The diagnostic ability of the 10 DEGs to distinguish AD from control group samples in the GSE122063 dataset showed good diagnostic value: HSPA1A (AUG = 0.8705); NEDD4L (AUG = 0.7918); CCL2 (AUG = 0.8409); PPBP (AUG = 0.7666); CCR5 (AUG = 0.8393); MPO (AUG = 0.7416); GDF15 (AUG = 0.8425); YWHAH (AUG = 0.7526); IL1R1 (AUG = 0.7390); SNCA (AUG = 0.7403) (Fig. 6).
Fig. 6
ROC analysis of HUB gene: A) HSPA1A; B) NEDD4L; C) CCL2; D) PPBP; E) CCR5; F) MPO; G) GDF15; H) YWHAH; I) IL1R1; J) SNCA.
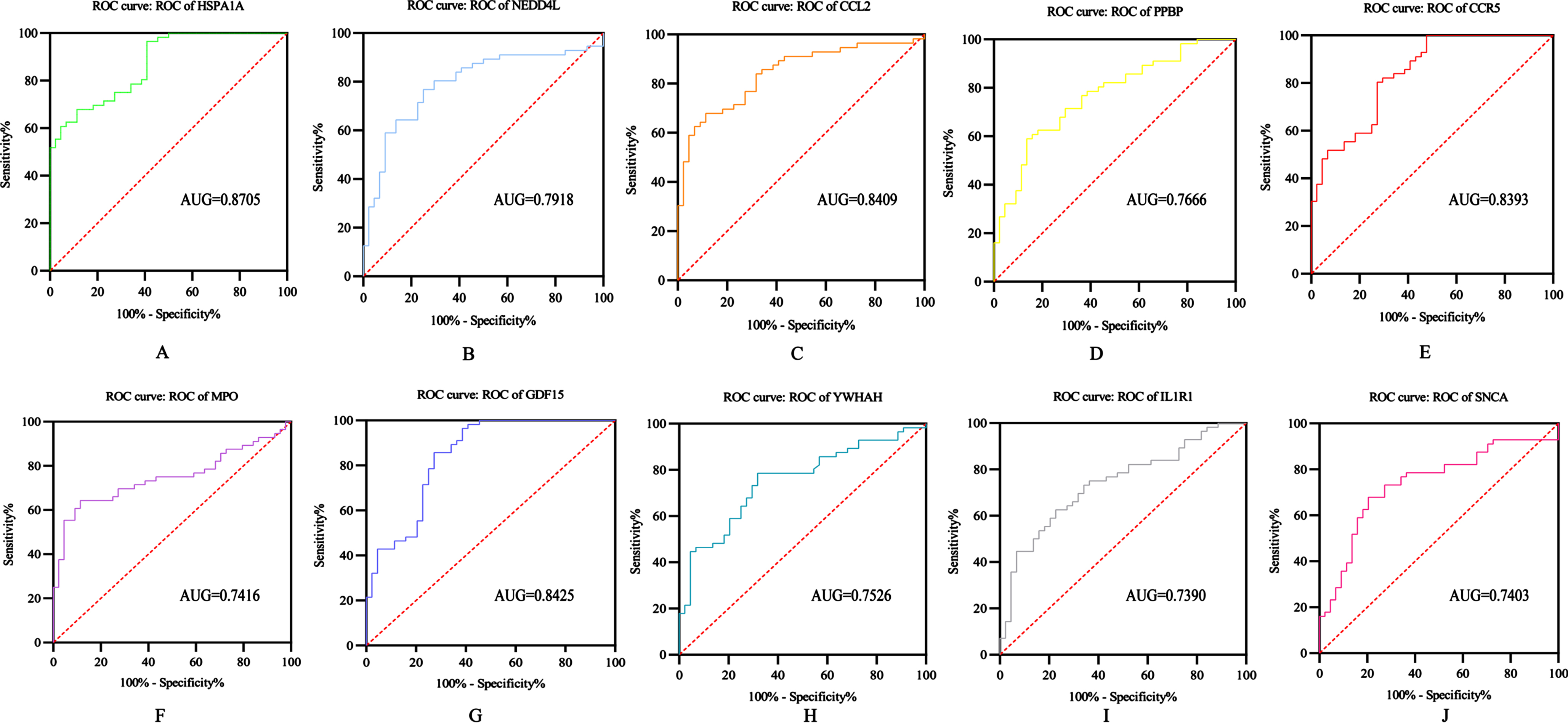
KEGG signaling pathway and Gene Ontology
Analysis of DEGs by Metascape revealed a total of 8 signaling pathways enriched by 10 hub genes p < 0.05, mainly including Cytokine-cytokine receptor interaction, Viral protein interaction with cytokine and cytokine receptor, Chemokine signaling pathway, Human cytomegalovirus infection, Endocytosis, Toxoplasmosis, Fluid shear stress and atherosclerosis, and Viral carcinogenesis (Fig. 7A).
Fig. 7
A) String Chart of KEGG signaling pathway; B) bar graph of Gene Ontology analysis diagram.
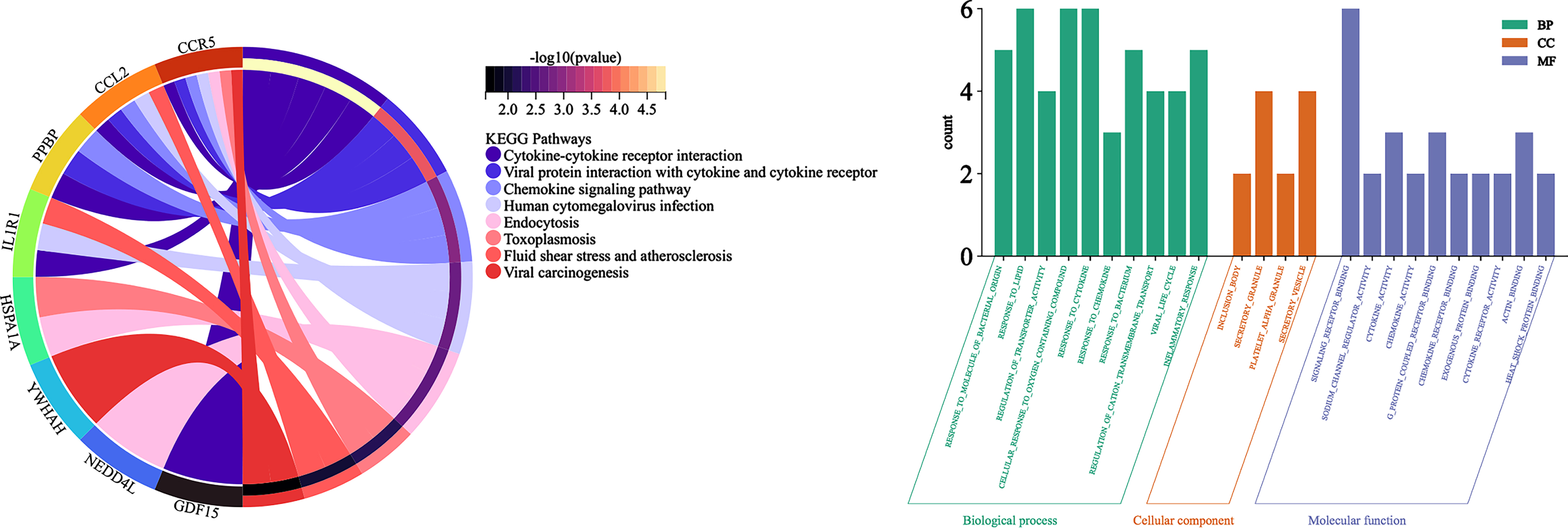
Analysis of DEGs by Metascape revealed a total of 567 items (p < 0.05): there were 60 molecular functions, including signaling receptor binding, sodium channel regulator activity, cytokine activity, chemokine activity, g protein coupled receptor binding; 4 cellular components, including inclusion body, secretory granule, platelet alpha granule, secretory vesicle; and 503 biological processes, including response to molecule of bacterial origin, response to lipid, regulation of transporter activity, cellular response to oxygen containing compound, response to cytokine; the top 10 p-values in each of the three groups were selected for visualization (Fig. 7B).
The regulatory relationship between Hub gene and TFs
A total of 36 TFs of 10 Hub genes were obtained through the TRRUST database, and the regulatory relationship between TFs and target genes are shown in Fig. 8.
Fig. 8
Interaction network diagram of hub gene-TFs (Pink circular nodes are hub genes; purple triangular nodes are transcription factors).
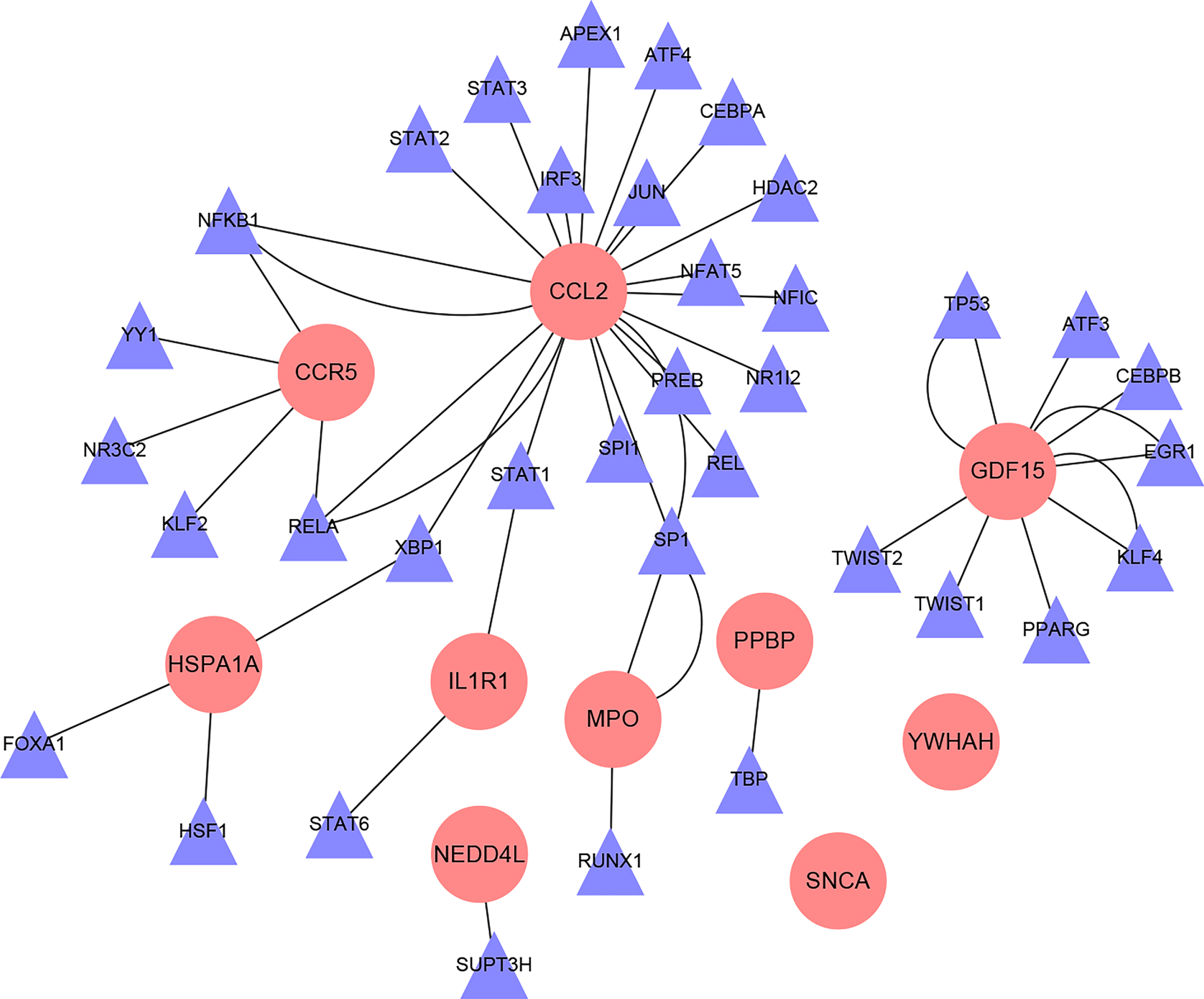
DISCUSSION
With the advent of the aging of the world population and the increase in life expectancy, AD has attracted widespread attention because of its insidious onset, irreversible neurodegenerative changes, and severe reduction in patients’ ability to perform daily living and social functions [29]. AD is a multifactorial disease that is determined by the interaction of genetic susceptibility and environmental factors throughout the life course [30]. Circadian rhythms are the periodic rhythms inherent in many lives and the most common form of rhythm in human life activities, and changes in people’s lifestyles often disrupt this rhythmic cycle. Researchers have thoroughly investigated the complex interrelationships that exist between lifestyle changes and AD-related mechanisms: 24.5% –40% of AD patients are reported to suffer from chronic sleep disorders [31]. In addition, sleep disorders causing circadian rhythm disturbances may be early signs of age-related neurodegenerative diseases and memory loss in the elderly. Melatonin, whose main function is to improve sleep disorders, has achieved significant efficacy in the treatment of AD in clinical studies, further confirming the existence of some linkage between the two [32]. Existing studies confirm that untimely light exposure or sleep deprivation inhibits the expression of Bmal1 (brain and muscle arnt-like) in neurons and glial cells of mice, a central factor in the transcriptional-translational feedback loops of the biological clock, and that Bmal1 deficiency leads to cortical and hippocampal astrocyte proliferation and memory impairment, resulting in an AD-like pathological development [33–35]. In addition, Wagner et al. autopsied brain samples from 32 volunteers and performed single nucleus RNA sequencing (snRNA-seq) on them, and the analysis revealed that APOE ɛ4, the most critical gene leading to the development of AD, causes abnormal accumulation of cholesterol in oligodendrocytes in AD patients, which makes the outer myelin sheath of neuronal axons become less and thinner, affecting neuronal myelin involved in neuronal metabolism and insulation function [36]. At the same time, Hita-Yañez et al. found a significant shortening of rapid eye movement (REM) sleep time in patients with cognitive impairment carrying the APOE ɛ4 gene. AD also has a significant disruptive effect on sleep rhythm [20]. The relationship between circadian rhythm disorders and AD is reciprocal, and a vicious circle is formed between the two, with the occurrence of either one leading to a high risk state for the other [37]. Therefore, an in-depth study of specific biomarkers sensitive to AD and CR is imminent. In this study, we deeply analyzed the differential genes between AD and control in the GEO database, and successfully constructed PPI network of DEGs using the STRING database and network construction tool, and enriched the differential genes; based on this, 10 important HUB genes were also screened and analyzed, including CCL2, CCR5, GDF15, HSPA1A, IL1R1, MPO, NEDD4L, PPBP, SNCA, and YWHAH.
Global gene expression studies (WGCNA) can help us better understand the specific pathobiology between AD and CR. The DGEs of the most important sections were further screened using WGCNA based on obtaining all DGEs of the AD and control groups in GSE122063. To gain more insight into these DEGs, we analyzed selected genes used for GO and KEGG enrichment analysis and found Neuroactive ligand-receptor interaction, Cytokine-cytokine receptor interaction, Long-term potentiation, Gap junction, Endocytosis, Serotonergic synapse, Fluid shear stress and atherosclerosis, Apelin signaling pathway, Lipid and atherosclerosis, Pathways of neurodegeneration - multiple diseases and other signaling pathways play important roles in AD. The pathways play an important role in AD. By studying circadian rhythm disorders in zebrafish larvae, Xiao et al. discovered significant differential expression of clarm1a, bmal1b, per1b, and per2 on neuroactive ligand-receptor interaction [38]. Neuroactive ligand-receptor interaction is also essential in neuroinflammatory diseases. Mesenchymal stem cells (MSCs) were found to activate neuroactive ligand-receptor interactions by secreting trophic factors, anti-apoptotic factors, and intervening in the immune response process, thereby activating the downstream PI3K-Akt signaling pathway, reducing neuroinflammatory response, enhancing neurogenesis, and improving efficacy in the treatment of neurological injury [39]. Thus, the interaction of neuroactive ligands with receptors and the PI3K-Akt signaling pathway may play an important role in neuroinflammation and neurodegenerative diseases caused by CR disorders.
The dopaminergic synapse is an important signal transduction pathway in AD that regulates synaptic function and modulates neurotransmitters, thereby enhancing memory [40]. Dopaminergic synapse is also an important neuromodulatory pathway for animals to maintain circadian rhythm periodicity within their physiological range and has been identified in Drosophila as an important pathway that innervates arousal-promoting neurons. Dopaminergic neurons innervate the large ventral lateral neurons (l-LNvs) of Drosophila and increase cAMP levels in the l-LNvs via signaling to induce the generation of action potentials that inhibit sleep and lead to arousal [41]. Fernandez-Chiappe et al. [42] confirmed this in his study. In his study, he found that Dopamine receptor 1 (Dop1R1) and Dopamine receptor 2 (Dop1R2) regulate daytime and nighttime sleep duration, respectively. In addition to higher receptor levels, correspondingly longer sleep duration, he also found that inhibition of the dopamine pathway following inhibition of the Dop1R2 receptor similarly reduced nighttime sleep duration in Drosophila, and that there is a complex mechanism for maintaining circadian periodicity [42]. Thus, we propose that circadian rhythm disorders may contribute to the development of AD via dopaminergic synapse signaling, neurodegeneration pathways, and multiple diseases.
The human master biological clock is located in the supraoptic nucleus (or core) (SCN). Circadian rhythm-related genes (CRY1, CRY2, DBP, NPAS2, PER1, PER3, PER2, CLOCK, etc.) regulate transcriptional and translational loops in cells. Circadian locomoter output cycles protein kaput (Clock), Basic helix-loop-helix ARNT-like protein 1 (Bmal1), Period circadian protein homolog 1 (PER), and Cryptochrome (Cry), whose expression levels fluctuate in a 24-hour cycle, together form a “transcription-translation-suppression of transcription” feedback loop that regulates the human biological rhythm. Neuronal PAS domain-containing protein 2 (NPAS2), CRY, and PER genes heterodimerize in the nucleus to drive positive feedback regulation of circadian rhythms and encode cyclin (PER and CRY); PER proteins accumulate mainly at night, and a certain amount of accumulation enters the nucleus to repress PER gene retrotranscription to close the feedback loop [43]. The operation of the feedback loop causes a circadian rhythm in the release of neural excitations, transmitters, and hormones, and this affects the cyclical fluctuations in brain function. In physiological states, amyloid β-protein (Aβ) secretion in brain tissue is reduced during sleep, whereas disrupted circadian rhythms cause abnormal Aβ release and accumulation, forming Aβ plaques and leading to the development of AD [44].
Then, we further analyzed the expression of 10 hub genes. The results showed that CCL2, CCR5, GDF15, HSPA1A, IL1R1, and PPBP were highly expressed in the AD group compared with the blank group (p < 0.05), while NEDD4L, MPO, YWHAH, and SNCA showed low expression (p < 0.05). C-C motif chemokine 2 (CCL2), also known as monocyte chemotactic protein 1 (MCP-1), is a small molecular weight cytokine of the CC chemokine family and is partially responsible for the chemotaxis of mononuclear phagocytes (microglia, peripheral monocytes, and macrophages). Increased CCL2 in serum and cerebrospinal fluid has been reported to be associated with neurodegeneration and negatively correlated with cognitive scores in AD patients [45, 46]. Previous studies have demonstrated that transgene overexpression of CCL2 enhances microglia proliferation and induces diffuse amyloid plaque deposition in Tg2576 mice [47]; further studies have shown that CCL2 transgene overexpression in mice as young as 2-3 months is able to accelerate spatial and working memory and deficits in hippocampal synaptic transmission in amyloid precursor protein (APP) mice, mainly because CCL2 is a potent enhancer of Aβ oligomerization, microglia accumulation and cognitive dysfunction in animal models of Aβ degeneration, and can directly promote Aβ uptake, intracellular Aβ oligomerization, and protein secretion [48]. Another study found that the upregulation of circadian gene albumin D site binding protein (DBP) is involved in the activation process of CCL2, while the dynamic changes of CCL2 expression level showed oscillatory changes similar to DBP [49]. C-C chemokine receptor type 5 (CCR5) is a suppressor of neuroplasticity and memory, that is highly enriched in the CA1 region of the hippocampus with the ligand CCL5, regulates learning-related cell signaling and neuronal plasticity, and is involved in many forms of regulation of learning and memory [50]. CCR5 has a role in regulating the expression and secretion of cytokines, promoting the expression of pro-inflammatory mediators such as interleukin (IL)-1β, tumor necrosis factor (TNF)-α, IL-6, and IL-17a, causing neuroinflammation and leading to the development of AD [51]. It was confirmed that elevated levels of CCR5 expression also reduced the overlap of activated neurons present in the memory fragments of mice, thereby inhibiting memory capacity and leading to further aggravation of AD [50]. This suggests that CCR5 could be an effective target for the treatment of AD. There is also a strong link between circadian rhythms and neuroinflammation. A study in depressed mice claimed that the Per2 gene induces neuroinflammatory responses through chemokines (especially CCR5 antagonists) [52]. Myeloperoxidase (MPO) is produced by neutrophils and is involved in the development of AD by participating in the molecular pathway leading to Aβ deposition [53, 54]. Also, MPO binds tightly to the vascular endothelium by interacting with negatively charged glycocalyx in other chronic inflammatory diseases, including heart disease, leading to cerebrovascular endothelial dysfunction and reducing blood-brain barrier function [55]. Other studies have shown a significant daily rhythmic cyclic variation in MPO activity [56]. There is a complex relationship between circadian rhythm and AD in the aspects of inflammation and metabolism, among which the role of CCR and MPO cannot beignored.
In addition, we performed a ROC analysis of the diagnostic value of the HUB gene in AD. The results showed that HSPA1A showed a high diagnostic value (AUC >0.85). Therefore, HSPA1A is considered an important biomarker to study the relationship between AD and circadian rhythm.
Conclusion
We have simultaneously explored the common related genes, pathways and biological processes of AD and CR, and clarified that there are complex molecular and cellular mechanisms between AD and CR. Most importantly, we have identified signaling pathways such as Neuroactive ligand-receptor interaction, Cytokine-cytokine receptor interaction, Viral protein interaction with cytokine and cytokine receptor in our study, further analyzed 10 important potential biomarkers such as CCL2, CCR5, and MPO, and found strong correlations between these associated genes and biological processes such as metabolism and inflammation. We elucidated that CR-related factors play a key role in the development of AD. We hope that our ideas will provide new ideas for further research in the prevention and treatment of AD.
ACKNOWLEDGMENTS
Thanks to Professor Wei Liu for his overall guidance on this paper and to Shang Gao for embellishing the images in this article.
FUNDING
This study was supported by the following funds: Taishan Scholars Project (NO. tsqn201909186); Natural Science Foundation of Shandong Province, No. ZR2019MH056; Special funds for the training of innovative backbone talents of traditional Chinese medicine; Special funds of Shandong TCM high-level Talents Training Project; National Natural Science Foundation of China, No.81273701
CONFLICT OF INTEREST
The authors have no conflict of interest to report.
DATA AVAILABILITY
Data supporting the results of this study are available from the corresponding author upon reasonable request.
REFERENCES
[1] | Robinson M , Lee BY , Hane FT ((2017) ) Recent progress in Alzheimer’s disease research, Part 2: Genetics and epidemiology. J Alzheimers Dis 57: , 317–330. |
[2] | Sengoku R ((2020) ) Aging and Alzheimer’s disease pathology. Neuropathology 40: , 22–29. |
[3] | Armstrong RA ((2019) ) Risk factors for Alzheimer’s disease. Folia Neuropathol 57: , 87–105. |
[4] | Kivipelto M , Mangialasche F , Ngandu T ((2018) ) Lifestyle interventions to prevent cognitive impairment, dementia and Alzheimer disease. Nat Rev Neurol 14: , 653–666. |
[5] | Cline EN , Bicca MA , Viola KL , Klein WL ((2018) ) The amyloid-β oligomer hypothesis: Beginning of the third decade. J Alzheimers Dis 64: , S567–s610. |
[6] | Henderson Z ((1996) ) Responses of basal forebrain cholinergic neurons to damage in the adult brain. Prog Neurobiol 48: , 219–254. |
[7] | Xing F , Meng T , Therriault J , Luo J , Zhang H ((2021) ) Apolipoprotein E ɛ4 and ɛ3 alleles associate with cerebrospinal fluid tau and cognition in the presence of amyloid-β in mild cognitive impairment but not in Alzheimer’s disease. J Integr Neurosci 20: , 277–286. |
[8] | Minter MR , Taylor JM , Crack PJ ((2016) ) The contribution of neuroinflammation to amyloid toxicity in Alzheimer’s disease. J Neurochem 136: , 457–474. |
[9] | Ashleigh T , Swerdlow RH , Beal MF ((2023) ) The role of mitochondrial dysfunction in Alzheimer’s disease pathogenesis. Alzheimers Dement 19: , 333–342. |
[10] | Zhou M , Wang H , Zeng X , Yin P , Zhu J , Chen W , Li X , Wang L , Wang L , Liu Y , Liu J , Zhang M , Qi J , Yu S , Afshin A , Gakidou E , Glenn S , Krish VS , Miller-Petrie MK , Mountjoy-Venning WC , Mullany EC , Redford SB , Liu H , Naghavi M , Hay SI , Wang L , Murray CJL , Liang X ((2019) ) Mortality, morbidity, and risk factors in China and its provinces, 1990-2017: A systematic analysis for the Global Burden of Disease Study 2017. Lancet 394: , 1145–1158. |
[11] | Bedrosian TA , Nelson RJ ((2013) ) Sundowning syndrome in aging and dementia: Research in mouse models. Exp Neurol 243: , 67–73. |
[12] | Ruan W , Yuan X , Eltzschig HK ((2021) ) Circadian rhythm as a therapeutic target. Nat Rev Drug Discov 20: , 287–307. |
[13] | Huang W , Ramsey KM , Marcheva B , Bass J ((2011) ) Circadian rhythms, sleep, and metabolism. J Clin Invest 121: , 2133–2141. |
[14] | Wu H , Dunnett S , Ho YS , Chang RC ((2019) ) The role of sleep deprivation and circadian rhythm disruption as risk factors of Alzheimer’s disease. Front Neuroendocrinol 54: , 100764. |
[15] | Bedrosian TA , Herring KL , Weil ZM , Nelson RJ ((2011) ) Altered temporal patterns of anxiety in aged and amyloid precursor protein (APP) transgenic mice. Proc Natl Acad Sci U S A 108: , 11686–11691. |
[16] | Ooms S , Overeem S , Besse K , Rikkert MO , Verbeek M , Claassen JA ((2014) ) Effect of 1 night of total sleep deprivation on cerebrospinal fluid β-amyloid 42 in healthy middle-aged men: A randomized clinical trial. JAMA Neurol 71: , 971–977. |
[17] | Mander BA , Winer JR , Walker MP ((2017) ) Sleep and human aging. Neuron 94: , 19–36. |
[18] | Holth JK , Fritschi SK , Wang C , Pedersen NP , Cirrito JR , Mahan TE , Finn MB , Manis M , Geerling JC , Fuller PM , Lucey BP , Holtzman DM ((2019) ) The sleep-wake cycle regulates brain interstitial fluid tau in mice and CSF tau in humans. Science 363: , 880–884. |
[19] | de Jonghe A , Korevaar JC , van Munster BC , de Rooij SE ((2010) ) Effectiveness of melatonin treatment on circadian rhythm disturbances in dementia. Are there implications for delirium? A systematic review. Int J Geriatr Psychiatry 25: , 1201–1208. |
[20] | Hita-Yañez E , Atienza M , Gil-Neciga E , Cantero JL ((2012) ) Disturbed sleep patterns in elders with mild cognitive impairment: The role of memory decline and ApoE ɛ4 genotype. Curr Alzheimer Res 9: , 290–297. |
[21] | Cooke JR , Loredo JS , Liu L , Marler M , Corey-Bloom J , Fiorentino L , Harrison T , Ancoli-Israel S ((2006) ) Acetylcholinesterase inhibitors and sleep architecture in patients with Alzheimer’s disease. Drugs Aging 23: , 503–511. |
[22] | Skjerve A , Nygaard HA ((2000) ) Improvement in sundowning in dementia with Lewy bodies after treatment with donepezil. Int J Geriatr Psychiatry 15: , 1147–1151. |
[23] | Barrett T , Wilhite SE , Ledoux P , Evangelista C , Kim IF , Tomashevsky M , Marshall KA , Phillippy KH , Sherman PM , Holko M , Yefanov A , Lee H , Zhang N , Robertson CL , Serova N , Davis S , Soboleva A ((2013) ) NCBI GEO: Archive for functional genomics data sets–update. Nucleic Acids Res 41: , D991–D995. |
[24] | Safran M , Dalah I , Alexander J , Rosen N , Iny Stein T , Shmoish M , Nativ N , Bahir I , Doniger T , Krug H , Sirota-Madi A , Olender T , Golan Y , Stelzer G , Harel A , Lancet D ((2010) ) GeneCards Version 3: The human gene integrator. Database (Oxford) 2010: , baq020. |
[25] | Szklarczyk D , Gable AL , Nastou KC , Lyon D , Kirsch R , Pyysalo S , Doncheva NT , Legeay M , Fang T , Bork P , Jensen LJ , von Mering C ((2021) ) The STRING database in 2021: Customizable protein-protein networks, and functional characterization of user-uploaded gene/measurement sets. Nucleic Acids Res 49: , D605–d612. |
[26] | Zhou Y , Zhou B , Pache L , Chang M , Khodabakhshi AH , Tanaseichuk O , Benner C , Chanda SK ((2019) ) Metascape provides a biologist-oriented resource for the analysis of systems-level datasets. Nat Commun 10: , 1523. |
[27] | Shannon P , Markiel A , Ozier O , Baliga NS , Wang JT , Ramage D , Amin N , Schwikowski B , Ideker T ((2003) ) Cytoscape: A software environment for integrated models of biomolecular interaction networks. Genome Res 13: , 2498–2504. |
[28] | Han H , Cho JW , Lee S , Yun A , Kim H , Bae D , Yang S , Kim CY , Lee M , Kim E , Lee S , Kang B , Jeong D , Kim Y , Jeon HN , Jung H , Nam S , Chung M , Kim JH , Lee I ((2018) ) TRRUST v2: An expanded reference database of human and mouse transcriptional regulatory interactions. Nucleic Acids Res 46: , D380–d386. |
[29] | Pollak CP , Perlick D ((1991) ) Sleep problems and institutionalization of the elderly. J Geriatr Psychiatry Neurol 4: , 204–210. |
[30] | Zhang XX , Tian Y , Wang ZT , Ma YH , Tan L , Yu JT ((2021) ) The epidemiology of Alzheimer’s disease modifiable risk factors and prevention. J Prev Alzheimers Dis 8: , 313–321. |
[31] | Moran M , Lynch CA , Walsh C , Coen R , Coakley D , Lawlor BA ((2005) ) Sleep disturbance in mild to moderate Alzheimer’s disease. Sleep Med 6: , 347–352. |
[32] | Prodhan A , Cavestro C , Kamal MA , Islam MA ((2021) ) Melatonin and sleep disturbances in Alzheimer’s disease. CNS Neurol Disord Drug Targets 20: , 736–754. |
[33] | Mongrain V , La Spada F , Curie T , Franken P ((2011) ) Sleep loss reduces the DNA-binding of BMAL1, CLOCK, and NPAS2 to specific clock genes in the mouse cerebral cortex. PLoS One 6: , e26622. |
[34] | Musiek ES , Lim MM , Yang G , Bauer AQ , Qi L , Lee Y , Roh JH , Ortiz-Gonzalez X , Dearborn JT , Culver JP , Herzog ED , Hogenesch JB , Wozniak DF , Dikranian K , Giasson BI , Weaver DR , Holtzman DM , Fitzgerald GA ((2013) ) Circadian clock proteins regulate neuronal redox homeostasis and neurodegeneration. J Clin Invest 123: , 5389–5400. |
[35] | Wardlaw SM , Phan TX , Saraf A , Chen X , Storm DR ((2014) ) Genetic disruption of the core circadian clock impairs hippocampus-dependent memory. Learn Mem 21: , 417–423. |
[36] | Wagner J , Degenhardt K , Veit M , Louros N , Konstantoulea K , Skodras A , Wild K , Liu P , Obermüller U , Bansal V , Dalmia A , Häsler LM , Lambert M , De Vleeschouwer M , Davies HA , Madine J , Kronenberg-Versteeg D , Feederle R , Del Turco D , Nilsson KPR , Lashley T , Deller T , Gearing M , Walker LC , Heutink P , Rousseau F , Schymkowitz J , Jucker M , Neher JJ ((2022) ) Medin co-aggregates with vascular amyloid-β in Alzheimer’s disease. Nature 612: , 123–131. |
[37] | Sharma A , Sethi G , Tambuwala MM , Aljabali AAA , Chellappan DK , Dua K , Goyal R ((2021) ) Circadian rhythm disruption and Alzheimer’s disease: The dynamics of a vicious cycle. Curr Neuropharmacol 19: , 248–264. |
[38] | Xiao B , Chen TM , Zhong Y ((2016) ) Possible molecular mechanism underlying cadmium-induced circadian rhythms disruption in zebrafish. Biochem Biophys Res Commun 481: , 201–205. |
[39] | He J , Zhang N , Zhu Y , Jin R , Wu F ((2021) ) MSC spheroids-loaded collagen hydrogels simultaneously promote neuronal differentiation and suppress inflammatory reaction through PI3K-Akt signaling pathway. Biomaterials 265: , 120448. |
[40] | Bamford NS , Wightman RM , Sulzer D ((2018) ) Dopamine’s effects on corticostriatal synapses during reward-based behaviors. Neuron 97: , 494–510. |
[41] | Friggi-Grelin F , Coulom H , Meller M , Gomez D , Hirsh J , Birman S ((2003) ) Targeted gene expression in Drosophila dopaminergic cells using regulatory sequences from tyrosine hydroxylase. J Neurobiol 54: , 618–627. |
[42] | Fernandez-Chiappe F , Hermann-Luibl C , Peteranderl A , Reinhard N , Senthilan PR , Hieke M , Selcho M , Yoshii T , Shafer OT , Muraro NI , Helfrich-Förster C ((2020) ) Dopamine signaling in wake-promoting clock neurons is not required for the normal regulation of sleep in Drosophila. J Neurosci 40: , 9617–9633. |
[43] | Linder S , Hoogstraat M , Stelloo S , Eickhoff N , Schuurman K , de Barros H , Alkemade M , Bekers EM , Severson TM , Sanders J , Huang CF , Morova T , Altintas UB , Hoekman L , Kim Y , Baca SC , Sjöström M , Zaalberg A , Hintzen DC , de Jong J , Kluin RJC , de Rink I , Giambartolomei C , Seo JH , Pasaniuc B , Altelaar M , Medema RH , Feng FY , Zoubeidi A , Freedman ML , Wessels LFA , Butler LM , Lack NA , van der Poel H , Bergman AM , Zwart W ((2022) ) Drug-induced epigenomic plasticity reprograms circadian rhythm regulation to drive prostate cancer toward androgen independence. Cancer Discov 12: , 2074–2097. |
[44] | Ju YE , Lucey BP , Holtzman DM ((2014) ) Sleep and Alzheimer disease pathology–a bidirectional relationship. Nat Rev Neurol 10: , 115–119. |
[45] | Galimberti D , Schoonenboom N , Scheltens P , Fenoglio C , Bouwman F , Venturelli E , Guidi I , Blankenstein MA , Bresolin N , Scarpini E ((2006) ) Intrathecal chemokine synthesis in mild cognitive impairment and Alzheimer disease. Arch Neurol 63: , 538–543. |
[46] | Lee WJ , Liao YC , Wang YF , Lin IF , Wang SJ , Fuh JL ((2018) ) Plasma MCP-1 and cognitive decline in patients with Alzheimer’s disease and mild cognitive impairment: A two-year follow-up study. Sci Rep 8: , 1280. |
[47] | Joly-Amado A , Hunter J , Quadri Z , Zamudio F , Rocha-Rangel PV , Chan D , Kesarwani A , Nash K , Lee DC , Morgan D , Gordon MN , Selenica MB ((2020) ) CCL2 overexpression in the brain promotes glial activation and accelerates tau pathology in a mouse model of tauopathy. Front Immunol 11: , 997. |
[48] | Kiyota T , Yamamoto M , Xiong H , Lambert MP , Klein WL , Gendelman HE , Ransohoff RM , Ikezu T ((2009) ) CCL2 accelerates microglia-mediated Abeta oligomer formation and progression of neurocognitive dysfunction. PLoS One 4: , e6197. |
[49] | Lu Y , Mei Y , Chen L , Wu L , Wang X , Zhang Y , Fu B , Chen X , Xie Y , Cai G , Bai X , Li Q , Chen X ((2019) ) The role of transcriptional factor D-site-binding protein in circadian CCL2 gene expression in anti-Thy1 nephritis. Cell Mol Immunol 16: , 735–745. |
[50] | Shen Y , Zhou M , Cai D , Filho DA , Fernandes G , Cai Y , de Sousa AF , Tian M , Kim N , Lee J , Necula D , Zhou C , Li S , Salinas S , Liu A , Kang X , Kamata M , Lavi A , Huang S , Silva T , Do Heo W , Silva AJ ((2022) ) CCR5 closes the temporal window for memory linking. Nature 606: , 146–152. |
[51] | Necula D , Riviere-Cazaux C , Shen Y , Zhou M ((2021) ) Insight into the roles of CCR5 in learning and memory in normal and disordered states. Brain Behav Immun 92: , 1–9. |
[52] | Chen X , Hu Q , Zhang K , Teng H , Li M , Li D , Wang J , Du Q , Zhao M ((2020) ) The clock-controlled chemokine contributes to neuroinflammation-induced depression. FASEB J 34: , 8357–8366. |
[53] | Zappia M , Manna I , Serra P , Cittadella R , Andreoli V , La Russa A , Annesi F , Spadafora P , Romeo N , Nicoletti G , Messina D , Gambardella A , Quattrone A ((2004) ) Increased risk for Alzheimer disease with the interaction of MPO and A2M polymorphisms. Arch Neurol 61: , 341–344. |
[54] | Smyth LCD , Murray HC , Hill M , van Leeuwen E , Highet B , Magon NJ , Osanlouy M , Mathiesen SN , Mockett B , Singh-Bains MK , Morris VK , Clarkson AN , Curtis MA , Abraham WC , Hughes SM , Faull RLM , Kettle AJ , Dragunow M , Hampton MB ((2022) ) Neutrophil-vascular interactions drive myeloperoxidase accumulation in the brain in Alzheimer’s disease. Acta Neuropathol Commun 10: , 38. |
[55] | van Leeuwen E , Hampton MB , Smyth LCD ((2020) ) Redox signalling and regulation of the blood-brain barrier. Int J Biochem Cell Biol 125: , 105794. |
[56] | Lazado CC , Gesto M , Madsen L , Jokumsen A ((2018) ) Interplay between daily rhythmic serum-mediated bacterial killing activity and immune defence factors in rainbow trout (Oncorhynchus mykiss). Fish Shellfish Immunol 72: , 418–425. |