Associations of Pulmonary Function with MRI Brain Volumes: A Coordinated Multi-Study Analysis
Abstract
Background:
Previous studies suggest poor pulmonary function is associated with increased burden of cerebral white matter hyperintensities and brain atrophy among elderly individuals, but the results are inconsistent.
Objective:
To study the cross-sectional associations of pulmonary function with structural brain variables.
Methods:
Data from six large community-based samples (N = 11,091) were analyzed. Spirometric measurements were standardized with respect to age, sex, height, and ethnicity using reference equations of the Global Lung Function Initiative. Associations of forced expiratory volume in 1 second (FEV1), forced vital capacity (FVC), and their ratio FEV1/FVC with brain volume, gray matter volume, hippocampal volume, and volume of white matter hyperintensities were investigated using multivariable linear regressions for each study separately and then combined using random-effect meta-analyses.
Results:
FEV1 and FVC were positively associated with brain volume, gray matter volume, and hippocampal volume, and negatively associated with white matter hyperintensities volume after multiple testing correction, with little heterogeneity present between the studies. For instance, an increase of FVC by one unit was associated with 3.5 ml higher brain volume (95% CI: [2.2, 4.9]). In contrast, results for FEV1/FVC were more heterogeneous across studies, with significant positive associations with brain volume, gray matter volume, and hippocampal volume, but not white matter hyperintensities volume. Associations of brain variables with both FEV1 and FVC were consistently stronger than with FEV1/FVC, specifically with brain volume and white matter hyperintensities volume.
Conclusion:
In cross-sectional analyses, worse pulmonary function is associated with smaller brain volumes and higher white matter hyperintensities burden.
INTRODUCTION
Both clinical and epidemiological studies suggest pulmonary diseases are related to increased risk of vascular brain injury and neurodegeneration in older individuals. For instance, chronic obstructive pulmonary disease (COPD) has been identified as a risk factor for cerebral small vessel disease, stroke, and cognitive impairment [1–3]. COPD is associated with structural brain abnormalities, including impaired white matter integrity [4], white matter hyperintensities [3, 5], and gray matter atrophy [6]. Chronic hypoxemia is a major driver of COPD-related pathology and has been found to be associated with increased risk of cognitive impairment [7]. COPD is characterized by an obstructive spirometry pattern, i.e., difficulties in exhaling quickly, with abnormally low forced expiratory volume in 1 second (FEV1) but preserved or proportionally less reduced forced vital capacity (FVC), resulting in reduced FEV1/FVC. Impaired vital capacity on the other hand is characterized by preserved FEV1/FVC with reduced FVC. It is as prevalent as COPD in the general population (about 10% in western countries) and may be an underappreciated cause of mild cognitive impairment and dementia [2, 8, 9].
The few studies investigating associations of impaired pulmonary function with structural brain variables in community samples have identified cerebellar abnormalities [10], overall brain atrophy [11], and increased burden of white matter hyperintensities [12–14]. However, they relied on relatively small samples and pulmonary function was assessed with only a single spirometry variable in some of these studies. We expanded on this literature by performing a coordinated multi-study analysis of associations of cross-sectional pulmonary function, assessed by FEV1, FVC, and FEV1/FVC, with structural brain variables in 11,091 individuals aged 21 to 97 years using six large community samples within the neurology working group of the Cross-Cohort Collaboration Consortium.
The main objective of our study was to gain insight into the brain structural underpinning of the previously reported association of impaired pulmonary function with increased dementia risk [2, 15]. Structural changes such as brain atrophy are known to precede cognitive decline and dementia by several years [16–18]. We hypothesized that reduced lung function (i.e., lower FEV1 or FVC) is associated with smaller brain volumes and higher burden of white matter hyperintensities.
MATERIALS AND METHODS
Study cohorts
Analyses were performed within the neurology working group of the Cross-Cohort Collaboration Consortium and were based on data from six large-scale community-based studies, namely: Age, Gene/Environment Susceptibility Study (AGES) [19], Atherosclerosis Risk in Communities Study (ARIC) [20], Cardiovascular Health Study (CHS) [21], Framingham Heart Study [22], the Rotterdam Study (RS) [23], and the Study of Health in Pomerania (SHIP) [24]. Participants who underwent both spirometry and brain magnetic resonance imaging (MRI) within 3 years were included. Details on data collection and the analytic samples can be found in the Supplementary Material.
Standardization of spirometric measurements
Forced expiratory volume in 1 second (FEV1) and forced vital capacity (FVC) were measured by spirometry in accordance with guidelines of the American Thoracic Society and the European Respiratory Society. At least two acceptable maneuvers were required. For more details, we refer to the Supplementary Material.
As spirometric measurements are known to decrease with age, airflow obstruction is underestimated in young and overestimated in elderly individuals when relying on unadjusted measurements (e.g., when using the Global Initiative for Chronic Obstructive Lung Disease criterion of FEV1/FVC < 70%) [25]. The measurements were therefore standardized with respect to age, sex, body height, and ethnicity (if applicable) by means of the Global Lung Function Initiative (GLI) 2012 reference equations, which are based on data of more than 70,000 healthy lifelong non-smokers [26]. Using these reference equations, each individual measurement was transformed to a z-score which indicates how many standard deviations it is from the expected value of a healthy non-smoker with the same age, sex, body height, and ethnicity (see [26] Fig. 8 on page 1337 for an illustration). All association analyses were performed with the resulting z-scores. Some studies found small but significant biases of these z-scores in specific populations [27, 28]. Therefore, we additionally included age, sex, and height in the regression models to account for any residual correlations with these important confounders (see below).
Brain imaging
Brain volume, gray matter volume, hippocampal volume (sum of left and right), and white matter hyperintensities volume were determined from T1-weighted and fluid attenuated inversion recovery (FLAIR) images via automated image-processing pipelines in all studies except AGES. In AGES, brain volumes were derived using multispectral MRI based on T1, T2, FLAIR, and proton density images. For details we refer to the Supplementary Material.
Statistical analyses
The main rationale behind the standardization of spirometry measurements based on GLI-2012 reference equations was to remove nuisance variation related to age, sex, and body height. We checked this by comparing the fraction of variance in spirometry measurements explained by these variables in the SHIP sample before and after standardization.
Associations of brain volume, gray matter volume, hippocampal volume, and log-transformed white matter hyperintensities volume (outcomes) with standardized FEV1, FVC, and FEV1/FVC (exposures) were analyzed by multivariable linear regressions in each study sample separately. Analyses were adjusted for age, sex, the interaction of age with sex, intracranial volume, body height, smoking status (never/former/current), and diabetes mellitus. Age was modelled using restricted cubic splines with five knots located study-specifically at the 5%, 27.5%, 50%, 72.5%, and 95% quantiles to account for acceleration of atrophy rates with increasing age [29, 30]. Diagnostic plots were inspected for outliers, which were excluded from the analyses. Results from each study were combined using a random-effects meta-analysis [31]. Heterogeneity was assessed using Cochran’s Q and I2 according to Higgins and Thompson [32]. We used the Benjamini-Hochberg procedure with false discovery rate (FDR)≤5% to account for multiple testing of associations between 4 outcomes and 3 exposures (12 tests) [33]. Statistical analyses were performed with R version 4.0 and the meta package [31]. For standardization of spirometric measurements, the rspiro package was used.
RESULTS
In total, cross-sectional data from 11,091 individuals aged 21 to 97 years were analyzed. Sample characteristics are shown in Table 1. All participants from AGES, ARIC, and CHS were older than 60 years. In total, 8,870 individuals (81%) were older than 60 years of age. Median age was lowest in SHIP (52 years) and highest in CHS (77 years). Prevalence of diabetes was considerably higher in ARIC (28%) compared to the other samples (about 10%).
Table 1
Sample characteristics. Values are given as numbers with percentages, or medians with interquartile ranges. All associations analyses were performed with z-scores of spirometry measurements according to the Global Lung Function Initiative 2012 reference equations. In total, data of 11,091 individuals from six community-based studies were analyzed
AGES | ARIC | CHS | FHS | RS | SHIP | |
Year | 2002 –2006 | 2011 –2013 | 1997 –1999 | 1998 –2001 | 2009 –2013 | 2008 –2011 |
n | 1655 | 1365 | 774 | 1720 | 3857 | 1720 |
Age at spirometry, y | 76 (71, 81) | 76 (72, 80) | 77 (74, 80) | 61 (54, 68) | 67 (62, 74) | 52 (42, 62) |
Time between spirometry | 14 (8, 27) | 284 (128, 460) | 407 (371, 448) | 207 (110, 349) | 23 (13, 43) | 18 (4, 39) |
and MRI (absolute value), d | ||||||
Females (%) | 956 (58) | 823 (60.3) | 455 (59) | 945 (55) | 2131 (55) | 877 (51) |
Smoking (%) | ||||||
never | 711 (43) | 603 (44.2) | 373 (48) | 629 (37) | 1241 (32) | 686 (40) |
former | 736 (45) | 692 (50.7) | 351 (45) | 894 (52) | 2154 (56) | 648 (38) |
current | 208 (12) | 70 (5.1) | 50 (7) | 197 (11) | 450 (12) | 386 (22) |
missing | 0 | 0 | 0 | 0 | 12 | 0 |
Height, m | 1.68 (1.59, 1.74) | 1.64 (1.58, 1.72) | 1.63 (1.56, 1.70) | 1.67 (1.61, 1.75) | 1.69 (1.62, 1.76) | 1.70 (1.63, 1.77) |
missing | 0 | 0 | 0 | 0 | 1 | 0 |
Diabetes (%) | 189 (11) | 381 (28) | 81 (10) | 167 (10) | 548 (14) | 150 (9) |
missing | 0 | 0 | 0 | 5 | 57 | 0 |
Forced expiratory volume in 1 s (FEV1), l | 2.06 (1.65, 2,59) | 2.04 (1.65, 2.47) | 1.88 (1.52, 2.34) | 2.63 (2.17, 3.19) | 2.59 (2.11, 3.17) | 3.22 (2.64, 3.90) |
missing | 0 (0) | 0 | 0 | 0 | 0 | 1 |
Forced vital capacity (FVC), l | 2.86 (2.35, 3.52) | 2.76 (2.27, 3.49) | 2.47 (1.98, 3.06) | 3.56 (3.01, 4.31) | 3.38 (2.77, 4.15) | 4.06 (3.37, 4.88) |
missing | 0 | 0 | 0 | 0 | 0 | 6 |
FEV1/FVC | 0.73 (0.68, 0.77) | 0.74 (0.68, 0.79) | 0.78 (0.73, 0.82) | 0.74 (0.69, 0.78) | 0.77 (0.73, 0.81) | 0.80 (0.76, 0.83) |
missing | 0 | 0 | 0 | 0 | 0 | 4 |
FEV1 GLI-2012 z-score | –0.62 (–1.39, 0.10) | –0.27 (–0.98, 0.41) | –0.49 (–1.32, 0.19) | –0.46 (–1.20, 0.18) | –0.04 (–0.78, 0.65) | 0.15 (–0.57, 0.84) |
missing | 0 | 0 | 0 | 0 | 0 | 1 |
FVC GLI-2012 z-score | –0.34 (–1.00, 0.24) | –0.03 (–0.72, 0.59) | –0.57 (–1.38, –0.00) | –0.06 (–0.69, 0.57) | –0.05 (–0.66, 0.57) | 0.11 (–0.51, 0.72) |
missing | 0 | 0 | 0 | 0 | 0 | 6 |
FEV1/FVC GLI-2012 z-score | –0.44 (–1.14, 0.06) | –0.41 (–1.07, 0.18) | 0.15 (–0.44, 0.60) | –0.74 (–1.32, –0.19) | –0.03 (–0.63, 0.50) | 0.01 (–0.58, 0.53) |
missing | 0 | 0 | 0 | 0 | 0 | 4 |
Intracranial volume, ml | 1490.7 (1398.0,1608.4) | 1366.9 (1268.5, 1484.8) | 1415.1 (1322.4, 1517.4) | 1227.2 (1145.3, 1313.8) | 1132.4 (1053.9, 1216.1) | 1584.1 (1470.6, 1703.8) |
missing | 0 | 0 | 0 | 37 | 0 | 0 |
Brain volume, ml | 1076.3 (1008.2,1151.1) | 1008.9 (940.1, 1088.3) | 956.5 (887.2, 1022.4) | 950.6 (883.3, 1023.4) | 925.0 (860.6, 990.7) | 1139.7 (1057.9, 1223.4) |
missing | 0 | 3 | 0 | 37 | 0 | 0 |
Gray matter volume, ml | 672.9 (631.9, 716.3) | 439.1 (409.8, 472.0) | 523.3 (485.7, 558.9) | 497.3 (461.6, 530.3) | 525.0 (490.0, 562.6) | 630.8 (588.0, 676.8) |
missing | 0 | 0 | 0 | 37 | 0 | 0 |
Total hippocampal volume, ml | 5.60 (5.17, 5.99) | 6.90 (6.30, 7.56) | 6.80 (6.21, 7.45) | 6.66 (6.64, 7.13) | 6.66 (6.21, 7.13) | 7.94 (7.37, 8.49) |
missing | 0 | 7 | 0 | 37 | 299 | 0 |
White matter hyperintensities volume, ml | 13.87 (7.65, 24.59) | 10.99 (6.18, 19.72) | 4.25 (2.56, 7.79) | 0.57 (0.32, 1.05) | 3.45 (1.83, 7.08) | 0.16 (0.06, 0.46) |
missing | 0 | 4 | 0 | 51 | 0 | 4 |
AGES, Age, Gene/Environment Susceptibility Study; ARIC, Atherosclerosis Risk in Communities Study; CHS, Cardiovascular Health Study; FHS, Framingham Heart Study; RS, Rotterdam Study; SHIP, Study of Health in Pomerania.
Overall, medians and quartiles of standardized spirometry measurements in SHIP and RS showed reasonable agreement with theoretical values of the normal distribution. Values were slightly smaller in the other samples comprising older individuals.
Among never-smokers from SHIP, about 75% of the variance of raw values of FEV1 and FVC could be explained by age, sex, and body height. After standardization according to the GLI-2012 reference equations, the explained variance dropped to 2% for FEV1 and 1% for FVC, and the model fit remained significant for FEV1 only (n = 685, F3,681 = 5.2, p = 0.002). However, standardized FEV1 and FVC were still highly correlated (r2 ≈ 0.85). Histograms of the spirometry measurements in SHIP are shown in Supplementary Figure 1, and all subsequent associations analyses were performed with the standardized z-scores.
Results of the association analyses in each study are shown in Fig. 1 and Supplementary Tables 1 and 2. Cohort-specific inspection of regression diagnostic plots led to the exclusion of up to 18 cases per cohort (proportions < 1.1%). Overall effects are shown in Table 2. After correction for multiple testing, FEV1 and FVC were significantly and positively associated with brain volume, gray matter volume, hippocampal volume, and negatively associated with white matter hyperintensities volume with little or no heterogeneity between the studies (I2 < 30%). For instance, an increase of FEV1 by one unit, which corresponds to about one standard deviation of raw FEV1 values, was associated with 3.4 ml higher brain volume (95% CI: [2.1, 4.7]). An increase of FVC by one unit was associated with 3.5 ml higher brain volume (95% CI: [2.2, 4.9]). This is approximately twice the one-year decrease in brain volume (about 1.7 ml) in the SHIP sample. FEV1/FVC was also significantly positively associated with brain volume, gray matter volume, and hippocampal volume, albeit with smaller effect estimates. An increase in FEV1/FVC by one unit was associated with 1.8 ml higher brain volume (95% CI: [0.6, 3.1]). FEV1/FVC was not associated with white matter hyperintensities volume. In contrast to the results for FEV1 and FVC, substantial heterogeneity was present in associations of FEV1/FVC with gray matter volume (I2 = 52%) and white matter hyperintensities volume (I2 = 61%).
Fig. 1
Forest plots of associations of standardized FEV1 (left column), FVC (middle column), and FEV1/FVC (right column) with brain volume (BV, in ml, A-C), gray matter volume (GMV, in ml, D-F), hippocampal volume (HV, in ml, G-I), and white matter hyperintensities volume (WMHV, in mm3, natural log-transformed, J-L). Regression coefficients (lines) and overall effects (diamonds) are shown. All analyses were adjusted for age, sex, intracranial volume, body height, smoking status, and diabetes mellitus. Red diamonds indicate significant overall effects after correction for multiple testing with the Benjamini-Hochberg method (FDR≤5%). FEV1, forced expiratory volume in 1 s; FVC, forced vital capacity. Abbreviations for the studies are defined in the footnote to Table 1.
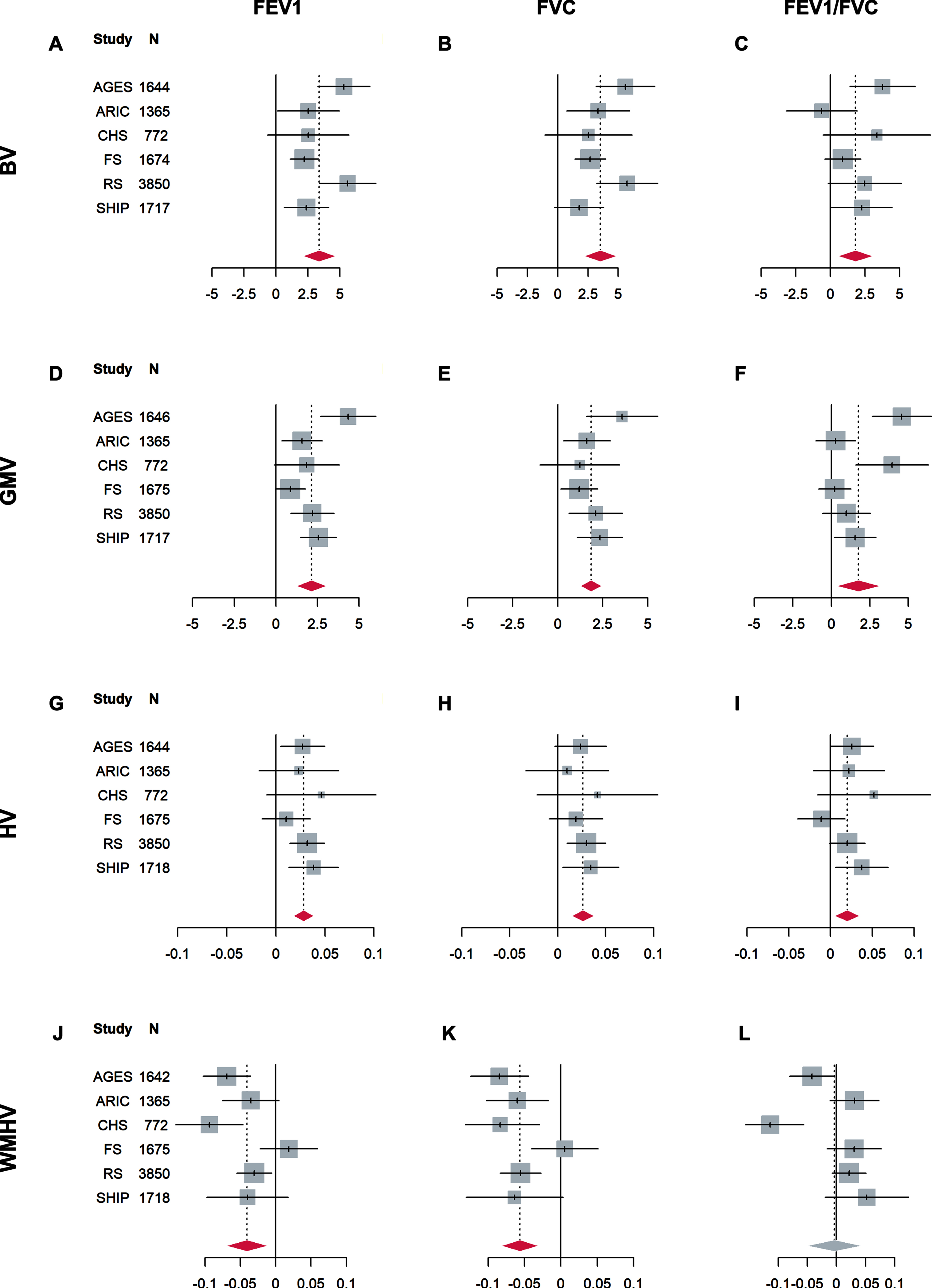
Table 2
Overall effects in linear ordinary regressions of structural brain variables on standardized FEV1, FVC, and FEV1/FVC. Analyses were adjusted for age, sex, intracranial volume, body height, smoking status, and presence of diabetes
Heterogeneity Test | |||||||||
Outcome | Exposure | No. of Studies | Cochran’s Q | p-value | I2 | Overall effect (95% CI) | p-value | pFDR | |
BV | FEV1 | 6 | 12.23 | 0.02 | 5 | 3.388 (2.086, 4.691) | 3.4E-07 | 1.0E-06 | *** |
BV | FVC | 6 | 8.67 | 0.07 | 0 | 3.525 (2.176, 4.873) | 3.0E-07 | 1.0E-06 | *** |
BV | FEV1/FVC | 6 | 8.73 | 0.11 | 0 | 1.811 (0.549, 3.072) | 0.00 | 0.01 | ** |
GMV | FEV1 | 6 | 16.42 | 0.01 | 21 | 2.136 (1.224, 3.049) | 4.5E-06 | 1.1E-05 | *** |
GMV | FVC | 6 | 6.16 | 0.34 | 0 | 1.860 (1.256, 2.463) | 1.6E-09 | 1.9E-08 | *** |
GMV | FEV1/FVC | 6 | 23.80 | 3.6E-4 | 52 | 1.755 (0.322, 3.187) | 0.02 | 0.02 | * |
HV | FEV1 | 6 | 3.56 | 0.66 | 0 | 0.028 (0.018, 0.039) | 9.3E-08 | 5.6E-07 | *** |
HV | FVC | 6 | 1.23 | 0.91 | 0 | 0.026 (0.014, 0.038) | 1.3E-05 | 2.6E-05 | *** |
HV | FEV1/FVC | 6 | 6.87 | 0.24 | 0 | 0.020 (0.005, 0.035) | 0.01 | 0.01 | * |
WMHV | FEV1 | 6 | 17.09 | 0.01 | 29 | –0.041 (–0.071, –0.011) | 0.01 | 0.01 | ** |
WMHV | FVC | 6 | 11.72 | 0.08 | 0 | –0.056 (–0.082, –0.031) | 1.9E-05 | 3.2E-05 | *** |
WMHV | FEV1/FVC | 6 | 29.74 | 5.3E-5 | 61 | –0.003 (–0.051, 0.044) | 0.89 | 0.89 |
FEV1, forced expiratory volume in 1 s; FVC, forced vital capacity; BV, brain volume (in ml); GMV, gray matter volume (in ml); HV, hippocampal volume (in ml); WMHV, volume of white matter hyperintensities (in mm3, natural log-transformed); I2, heterogeneity index; FDR, false discovery rate. ***pFDR < 0.001, **pFDR < 0.01, *pFDR < 0.05.
DISCUSSION
In our coordinated meta-analyses of six large-scale community-based samples comprising 11,091 individuals, we found statistically significant associations of both lower values of FEV1 and FVC with lower values of brain volume, gray matter volume, hippocampal volume, and higher volume of white matter hyperintensities. FEV1/FVC was also statistically significantly, albeit less strongly, associated with brain volume, gray matter volume, and hippocampal volume, but not with white matter hyperintensities volume.
Our findings are line with the general consensus of previous smaller studies. For instance, Sachdev et al. reported associations of FEV1 and FVC, but not FEV1/FVC, with subcortical brain atrophy in a community sample of 469 individuals aged 60-64 years [11]. In contrast to our study, they did not find an association with white matter hyperintensities volume, which may be attributed to the smaller sample size and, on average, younger participants. However, two larger studies did find associations of FEV1 and FVC with markers of cerebral small vessel disease, including lacunes and white matter hyperintensities, which is in line with our results [13, 14].
COPD has long been recognized as an important risk factor for reduced white matter integrity, increased white matter hyperintensities burden, and cognitive decline [4, 5]. It is characterized by an obstructive spirometry pattern, i.e., decreased FEV1 but relatively preserved FVC resulting in reduced FEV1/FVC. Impaired vital capacity on the other hand, characterized by simultaneously decreased FEV1 and FVC but preserved FEV1/FVC, is as prevalent in the general population (about 10% in western countries) and has been found to be associated with diabetes, metabolic syndrome, and systemic inflammation [8, 9]. Besides genetic and developmental factors (e.g., low birth weight, childhood socio-economic status) [34–37], exposure to air pollutants such as particulate matter or nitrogen dioxides is believed to be a major risk factor for the development of restrictive dysfunction, potentially explaining the high prevalence in countries with high usage of biomass fuels for cooking [38–41]. Impaired vital capacity is associated with chronic respiratory symptoms, higher rates of cardiovascular disease, and increased mortality [8]. Two studies conducted within ARIC found impaired pulmonary function, in particular impaired vital capacity, to be associated with worse cognitive function and increased risk of dementia and mild cognitive impairment later in life [2, 42]. While some heterogeneity was present in our study, the overall associations for FVC were consistently stronger than for FEV1/FVC, specifically with brain volume and white matter hyperintensities volume. This might explain the more pronounced association of impaired vital capacity with increased risk of dementia and lower cognitive function found in previous studies. However, reduced FVC can be both due to lung restriction and hyperinflation (sometimes seen in COPD with predominant emphysema) and differentiation requires full pulmonary testing (body plethysmography). This is also reflected in current guidelines which only distinguish between obstruction and non-obstruction [43]. Further studies relying on body plethysmography data are required to assess the differential associations of restrictive and obstructive lung disease with brain aging and dementia.
Pathophysiological mechanisms
Pulmonary function is linked to advanced brain ageing by several pathophysiological mechanisms. For instance, poor pulmonary function is associated with reduced cerebral blood flow, which may lead to hypoxia and ischemic brain injury [3, 44]. Second, a close link also exists with diabetes [45, 46], which itself is associated with cognitive dysfunction and advanced brain ageing [47]. Hypoxemia-induced insulin resistance, and alterations in organogenesis and metabolic pathway programing due to adverse early-life conditions have been suggested as potential mechanisms, among others [45]. Nevertheless, associations in the current study persisted after accounting for comorbid diabetes. Third, systemic inflammation has been found to be related to both advanced brain aging [48] and reduced pulmonary function independent from smoking and obesity [49, 50]. Systemic inflammation may be due to local inflammation within the lungs as part of a defense reaction against environmental toxins or infection agents [50].
Strengths and limitations
In addition to the large sample size, one of the strengths of our study is the standardization of spirometry measurements based on GLI-2012 reference equations [26]. These are based on data from 74,187 life-long non-smokers without lung disease and allow for separating pathological changes from those related to normal ageing, sex, body size, and ethnicity. As shown in the SHIP sample, variance in spirometry measurements related to age, sex, and body height was greatly reduced by this procedure, with the explained variance decreasing from about 75% to 2% for FEV1 and 1% for FVC, respectively. This is of great importance, since these variables are major confounders in association analyses with structural brain variables [26, 30, 51, 52]. The resulting standardized spirometry measurements should be approximately normally distributed, which was seen in our study samples.
While the assessment of airway restriction is based on forced expiratory maneuvers in most studies it can also be measured by maximal inspiration after complete expiration. The so-obtained inspiratory vital capacity (IVC) is generally larger than FVC with the difference being more pronounced in individuals with obstructive impairment due to narrowing of peripheral airways. As a consequence, FVC underestimates the actual vital capacity and FEV1/FVC is spuriously high when being indexed to FVC compared to IVC in those individuals [53, 54]. Future studies should therefore also consider the IVC in addition to FVC. However, reference equations for IVC based different ethnicities and ages ranges are still lacking to date. Another possibility is the measurement of vital capacity during slow (non-forced) exhalation, which also reduces its underestimation compared to forced exhalation. Our study has several additional limitations. First, the cross-sectional nature of the analyses hampers causal inference. Second, selecting participants who underwent both spirometry and MRI may have led to somewhat healthier analytic samples. This might have introduced bias, most likely causing an underestimation of the true effect, for instance when current smokers with worse brain health and lower pulmonary function are less willing to participate. Third, there is the possibility of reverse causality, with subjects performing worse in the spirometric testing due to their cognitive impairment. Fourth, although we considered important potential confounders such as age, body height, smoking, and diabetes, residual confounding cannot be ruled out. For instance, dietary factors (e.g., intake of foods rich in anti-oxidants and vitamins) have been associated with pulmonary function, brain structure, and cognition [55–57]. Finally, as the inspiratory vital capacity exceeds the FVC in particular in individuals with obstructive impairment, overestimations of FEV1/FVC in our study may have attenuated the corresponding effect estimates.
CONCLUSIONS
In summary, impaired pulmonary function is associated with lower brain volumes and increased burden of white matter hyperintensities in six large community-based samples. Our results provide further evidence to the notion that lung function plays an important but underappreciated role in cognitive decline and dementia pathophysiology.
ACKNOWLEDGMENTS
This study was supported by National Institute of Health (NIH) grant AG059421. Additional study-specific acknowledgements can be found in the Supplementary Material.
Authors’ disclosures available online (https://www.j-alz.com/manuscript-disclosures/22-0667r1).
SUPPLEMENTARY MATERIAL
[1] The supplementary material is available in the electronic version of this article: https://dx.doi.org/10.3233/JAD-220667.
REFERENCES
[1] | Lahousse L , Tiemeier H , Ikram MA , Brusselle GG ((2015) ) Chronic obstructive pulmonary disease and cerebrovascular disease: A comprehensive review. Respir Med 109: , 1371–1380. |
[2] | Lutsey PL , Chen N , Mirabelli MC , Lakshminarayan K , Knopman DS , Vossel KA , Gottesman RF , Mosley TH , Alonso A ((2019) ) Impaired lung function, lung disease, and risk of incident dementia. Am J Respir Crit Care Med 199: , 1385–1396. |
[3] | van Dijk EJ , Vermeer SE , de Groot JC , van de Minkelis J , Prins ND , Oudkerk M , Hofman A , Koudstaal PJ , Breteler MMB ((2004) ) Arterial oxygen saturation, COPD, and cerebral small vessel disease. J Neurol Neurosurg Psychiatry 75: , 733–736. |
[4] | Dodd JW , Chung AW , van den Broek MD , Barrick TR , Charlton RA , Jones PW ((2012) ) Brain structure and function in chronic obstructive pulmonary disease: A multimodal cranial magnetic resonance imaging study. Am J Respir Crit Care Med 186: , 240–245. |
[5] | Spilling CA , Jones PW , Dodd JW , Barrick TR ((2017) ) White matter lesions characterise brain involvement in moderate to severe chronic obstructive pulmonary disease, but cerebral atrophy does not. BMC Pulm Med 17: , 92. |
[6] | Spilling CA , Bajaj M-PK , Burrage DR , Ruickbie S , Thai NJ , Baker EH , Jones PW , Barrick TR , Dodd JW ((2019) ) Contributions of cardiovascular risk and smoking to chronic obstructive pulmonary disease (COPD)-related changes in brain structure and function. Int J Chron Obstruct Pulmon Dis 14: , 1855–1866. |
[7] | Thakur N , Blanc PD , Julian LJ , Yelin EH , Katz PP , Sidney S , Iribarren C , Eisner MD ((2010) ) COPD and cognitive impairment: The role of hypoxemia and oxygen therapy. Int J Chron Obstruct Pulmon Dis 5: , 263–269. |
[8] | Godfrey MS , Jankowich MD ((2016) ) The vital capacity is vital: Epidemiology and clinical significance of the restrictive spirometry pattern. Chest 149: , 238–251. |
[9] | Backman H , Eriksson B , Hedman L , Stridsman C , Jansson S-A , Sovijärvi A , Lindberg A , Rönmark E , Lundbäck B ((2016) ) Restrictive spirometric pattern in the general adult population: Methods of defining the condition and consequences on prevalence. Respir Med 120: , 116–123. |
[10] | Taki Y , Kinomura S , Ebihara S , Thyreau B , Sato K , Goto R , Kakizaki M , Tsuji I , Kawashima R , Fukuda H ((2013) ) Correlation between pulmonary function and brain volume in healthy elderly subjects. Neuroradiology 55: , 689–695. |
[11] | Sachdev PS , Anstey KJ , Parslow RA , Wen W , Maller J , Kumar R , Christensen H , Jorm AF ((2006) ) Pulmonary function, cognitive impairment and brain atrophy in a middle-aged community sample. Dement Geriatr Cogn Disord 21: , 300–308. |
[12] | Longstreth Wt , Manolio TA , Arnold A , Burke GL , Bryan N , Jungreis CA , Enright PL , O’Leary D , Fried L ((1996) ) Clinical correlates of white matter findings on cranial magnetic resonance imaging of 3301 elderly people. Stroke 27: , 1274–1282. |
[13] | Liao D , Higgins M , Bryan NR , Eigenbrodt ML , Chambless LE , Lamar V , Burke GL , Heiss G ((1999) ) Lower pulmonary function and cerebral subclinical abnormalities detected by MRI: The Atherosclerosis Risk in Communities Study. Chest 116: , 150–156. |
[14] | Kim Y , Lee H , Son TO , Jang H , Cho SH , Kim SE , Kim SJ , Lee JS , Kim JP , Jung YH , Lockhart SN , Kim HJ , Na DL , Park HY , Seo SW ((2020) ) Reduced forced vital capacity is associated with cerebral smallvessel disease burden in cognitively normal individuals. Neuroimage Clin 25: , 102140. |
[15] | Schaub RT , Münzberg H , Borchelt M , Nieczaj R , Hillen T , Reischies FM , Schlattmann P , Geiselmann B , Steinhagen-Thiessen E ((2000) ) Ventilatory capacity and risk for dementia.M. J Gerontol A Biol Sci Med Sci 55: , 677–683. |
[16] | Jack CR , Shiung MM , Weigand SD , O’Brien PC , Gunter JL , Boeve BF , Knopman DS , Smith GE , Ivnik RJ , Tangalos EG , Petersen RC ((2005) ) Brain atrophy rates predict subsequent clinical conversion in normal elderly and amnestic MCI. Neurology 65: , 1227–1231. |
[17] | Davatzikos C , Bhatt P , Shaw LM , Batmanghelich KN , Trojanowski JQ ((2011) ) Prediction of MCI to AD conversion, via MRI, CSF biomarkers, and pattern classification. Neurobiol Aging 32: , 2322.e19–27. |
[18] | Bateman RJ , Xiong C , Benzinger TLS , Fagan AM , Goate A , Fox NC , Marcus DS , Cairns NJ , Xie X , Blazey TM , Holtzman DM , Santacruz A , Buckles V , Oliver A , Moulder K , Aisen PS , Ghetti B , Klunk WE , McDade E , Martins RN , Masters CL , Mayeux R , Ringman JM , Rossor MN , Schofield PR , Sperling RA , Salloway S , Morris JC ((2012) ) Clinical and biomarker changes in dominantly inherited Alzheimer’s disease. N Engl J Med 367: , 795–804. |
[19] | Harris TB , Launer LJ , Eiriksdottir G , Kjartansson O , Jonsson PV , Sigurdsson G , Thorgeirsson G , Aspelund T , Garcia ME , Cotch MF , Hoffman HJ , Gudnason V ((2007) ) Age, Gene/Environment Susceptibility-Reykjavik Study: Multidisciplinary applied phenomics. Am J Epidemiol 165: , 1076–1087. |
[20] | ((1989) ) The Atherosclerosis Risk in Communities (ARIC) Study: Design and objectives. The ARIC investigators. Am J Epidemiol 129: , 687–702. |
[21] | Fried LP , Borhani NO , Enright P , Furberg CD , Gardin JM , Kronmal RA , Kuller LH , Manolio TA , Mittelmark MB , Newman A ((1991) ) The Cardiovascular Health Study: Design and rationale. Ann Epidemiol 1: , 263–276. |
[22] | Splansky GL , Corey D , Yang Q , Atwood LD , Cupples LA , Benjamin EJ , D’Agostino RB , Fox CS , Larson MG , Murabito JM , O’Donnell CJ , Vasan RS , Wolf PA , Levy D ((2007) ) The Third Generation Cohort of the National Heart, Lung, and Blood Institute’s Framingham Heart Study: Design, recruitment, and initial examination. Am J Epidemiol 165: , 1328–1335. |
[23] | Ikram MA , Brusselle G , Ghanbari M , Goedegebure A , Ikram MK , Kavousi M , Kieboom BCT , Klaver CCW , de Knegt RJ , Luik AI , Nijsten TEC , Peeters RP , van Rooij FJA , Stricker BH , Uitterlinden AG , Vernooij MW , Voortman T ((2020) ) Objectives, design and main findings until 2020 from the Rotterdam Study. Eur J Epidemiol 35: , 483–517. |
[24] | Völzke H , Schössow J , Schmidt CO , Jürgens C , Richter A , Werner A , Werner N , Radke D , Teumer A , Ittermann T , Schauer B , Henck V , Friedrich N , Hannemann A , Winter T , Nauck M , Dörr M , Bahls M , Felix SB , Stubbe B , Ewert R , Frost F , Lerch MM , Grabe HJ , Bülow R , Otto M , Hosten N , Rathmann W , Schminke U , Großjohann R , Tost F , Homuth G , Völker U , Weiss S , Holtfreter S , Bröker BM , Zimmermann K , Kaderali L , Winnefeld M , Kristof B , Berger K , Samietz S , Schwahn C , Holtfreter B , Biffar R , Kindler S , Wittfeld K , Hoffmann W , Kocher T (2022) Cohort Profile Update: The Study of Health in Pomerania (SHIP). Int J Epidemiol, doi: 10.1093/ije/dyac034. |
[25] | Hansen JE , Sun X-G , Wasserman K ((2007) ) Spirometric criteria for airway obstruction: Use percentage of FEV1/FVC ratio below the fifth percentile, not<70% . Chest 131: , 349–355. |
[26] | Quanjer PH , Stanojevic S , Cole TJ , Baur X , Hall GL , Culver BH , Enright PL , Hankinson JL , Ip MSM , Zheng J , Stocks J , ERS Global Lung Function Initiative ((2012) ) Multi-ethnic reference values for spirometry for the 3-95-yr age range: The global lung function 2012 equations. Eur Respir J 40: , 1324–1343. |
[27] | Backman H , Lindberg A , Sovijärvi A , Larsson K , Lundbäck B , Rönmark E ((2015) ) Evaluation of the global lung function initiative 2012 reference values for spirometry in a Swedish population sample. BMC Pulm Med 15: , 26. |
[28] | Zhang J , Hu X , Tian X , Xu K-F ((2018) ) Global lung function initiative 2012 reference values for spirometry in Asian Americans. BMC Pulm Med 18: , 95. |
[29] | Harrell F ((2015) ) Regression Modeling Strategies: With Applications to Linear Models, Logistic and Ordinal Regression, and Survival Analysis, Springer International Publishing. |
[30] | Scahill RI , Frost C , Jenkins R , Whitwell JL , Rossor MN , Fox NC ((2003) ) A longitudinal study of brain volume changes in normal aging using serial registered magnetic resonance imaging. Arch Neurol 60: , 989–994. |
[31] | Schwarzer G , Carpenter JR , Rücker G ((2015) ) Meta-Analysis with R, Springer International Publishing. |
[32] | Higgins JPT , Thompson SG ((2002) ) Quantifying heterogeneity in a meta-analysis. Stat Med 21: , 1539–1558. |
[33] | Benjamini Y , Hochberg Y ((1995) ) Controlling the false discovery rate: A practical and powerful approach to multiple testing. J R Stat Soc Series B Stat Methodol 57: , 289–300. |
[34] | Barker DJ , Godfrey KM , Fall C , Osmond C , Winter PD , Shaheen SO ((1991) ) Relation of birth weight and childhood respiratory infection to adult lung function and death from chronic obstructive airways disease. BMJ 303: , 671–675. |
[35] | Saad NJ , Patel J , Burney P , Minelli C ((2017) ) Birth weight and lung function in adulthood: A systematic review and meta-analysis. Ann Am Thorac Soc 14: , 994–1004. |
[36] | Jackson B , Kubzansky LD , Cohen S , Weiss S , Wright RJ , CARDIA Study ((2004) ) A matter of life and breath: Childhood socioeconomic status is related to young adult pulmonary function in the CARDIA study. Int J Epidemiol 33: , 271–278. |
[37] | Loth DW , Soler Artigas M , Gharib SA , Wain LV , Franceschini N , Koch B , Pottinger TD , Smith AV , Duan Q , Oldmeadow C , Lee MK , Strachan DP , James AL , Huffman JE , Vitart V , Ramasamy A , Wareham NJ , Kaprio J , Wang X-Q , Trochet H , Kähönen M , Flexeder C , Albrecht E , Lopez LM , de Jong K , Thyagarajan B , Alves AC , Enroth S , Omenaas E , Joshi PK , Fall T , Viñuela A , Launer LJ , Loehr LR , Fornage M , Li G , Wilk JB , Tang W , Manichaikul A , Lahousse L , Harris TB , North KE , Rudnicka AR , Hui J , Gu X , Lumley T , Wright AF , Hastie ND , Campbell S , Kumar R , Pin I , Scott RA , Pietiläinen KH , Surakka I , Liu Y , Holliday EG , Schulz H , Heinrich J , Davies G , Vonk JM , Wojczynski M , Pouta A , Johansson A , Wild SH , Ingelsson E , Rivadeneira F , Völzke H , Hysi PG , Eiriksdottir G , Morrison AC , Rotter JI , Gao W , Postma DS , White WB , Rich SS , Hofman A , Aspelund T , Couper D , Smith LJ , Psaty BM , Lohman K , Burchard EG , Uitterlinden AG , Garcia M , Joubert BR , McArdle WL , Musk AB , Hansel N , Heckbert SR , Zgaga L , van Meurs JBJ , Navarro P , Rudan I , Oh Y-M , Redline S , Jarvis DL , Zhao JH , Rantanen T , O’Connor GT , Ripatti S , Scott RJ , Karrasch S , Grallert H , Gaddis NC , Starr JM , Wijmenga C , Minster RL , Lederer DJ , Pekkanen J , Gyllensten U , Campbell H , Morris AP , Gläser S , Hammond CJ , Burkart KM , Beilby J , Kritchevsky SB , Gudnason V , Hancock DB , Williams OD , Polasek O , Zemunik T , Kolcic I , Petrini MF , Wjst M , Kim WJ , Porteous DJ , Scotland G , Smith BH , Viljanen A , Heliövaara M , Attia JR , Sayers I , Hampel R , Gieger C , Deary IJ , Boezen HM , Newman A , Jarvelin M-R , Wilson JF , Lind L , Stricker BH , Teumer A , Spector TD , Melén E , Peters MJ , Lange LA , Barr RG , Bracke KR , Verhamme FM , Sung J , Hiemstra PS , Cassano PA , Sood A , Hayward C , Dupuis J , Hall IP , Brusselle GG , Tobin MD , London SJ ((2014) ) Genome-wide association analysis identifies six new lociassociated with forced vital capacity. Nat Genet 46: , 669–677. |
[38] | Adam M , Schikowski T , Carsin AE , Cai Y , Jacquemin B , Sanchez M , Vierkötter A , Marcon A , Keidel D , Sugiri D , Kanani ZA , Nadif R , Siroux V , Hardy R , Kuh D , Rochat T , Bridevaux P-O , Eeftens M , Tsai M-Y , Villani S , Phuleria HC , Birk M , Cyrys J , Cirach M , Nazelle A de , Nieuwenhuijsen MJ , Forsberg B , Hoogh K de , Declerq C , Bono R , Piccioni P , Quass U , Heinrich J , Jarvis D , Pin I , Beelen R , Hoek G , Brunekreef B , Schindler C , Sunyer J , Krämer U , Kauffmann F , Hansell AL , Künzli N , Probst-Hensch N ((2015) ) Adult lung function and long-term air pollution exposure. ESCAPE: A multicentre cohort study and meta-analysis. Eur Respir J 45: , 38–50. |
[39] | Gauderman WJ , Urman R , Avol E , Berhane K , McConnell R , Rappaport E , Chang R , Lurmann F , Gilliland F ((2015) ) Association of improved air quality with lung development in children. N Engl J Med 372: , 905–913. |
[40] | Mannino DM , McBurnie MA , Tan W , Kocabas A , Anto J , Vollmer WM , Buist AS , BOLD Collaborative Research Group ((2012) ) Restricted spirometry in the Burden of Lung Disease Study. Int J Tuberc Lung Dis 16: , 1405–1411. |
[41] | Jong K de , Vonk JM , Zijlema WL , Stolk RP , van der Plaat DA , Hoek G , Brunekreef B , Postma DS , Boezen HM ((2016) ) Air pollution exposure is associated with restrictive ventilatory patterns. Eur Respir J 48: , 1221–1224. |
[42] | Pathan SS , Gottesman RF , Mosley TH , Knopman DS , Sharrett AR , Alonso A ((2011) ) Association of lung function with cognitive decline and dementia: The Atherosclerosis Risk in Communities (ARIC) Study. Eur J Neurol 18: , 888–898. |
[43] | Graham BL , Steenbruggen I , Miller MR , Barjaktarevic IZ , Cooper BG , Hall GL , Hallstrand TS , Kaminsky DA , McCarthy K , McCormack MC , Oropez CE , Rosenfeld M , Stanojevic S , Swanney MP , Thompson BR ((2019) ) Standardization of Spirometry 2019 Update. An Official American Thoracic Society and European Respiratory Society Technical Statement. Am J Respir Crit Care Med 200: , e70–e88. |
[44] | Peers C , Dallas ML , Boycott HE , Scragg JL , Pearson HA , Boyle JP ((2009) ) Hypoxia and neurodegeneration. Ann N Y Acad Sci 1177: , 169–177. |
[45] | Yeh H-C , Punjabi NM , Wang N-Y , Pankow JS , Duncan BB , Brancati FL ((2005) ) Vital capacity as a predictor of incident type 2 diabetes: The Atherosclerosis Risk in Communities study. Diabetes Care 28: , 1472–1479. |
[46] | Lee HM , Chung SJ , Lopez VA , Wong ND ((2009) ) Association of FVC and total mortality in US adults with metabolic syndrome and diabetes. Chest 136: , 171–176. |
[47] | Cholerton B , Baker LD , Craft S ((2011) ) Insulin resistance and pathological brain ageing. Diabet Med 28: , 1463–1475. |
[48] | Janowitz D , Habes M , Toledo JB , Hannemann A , Frenzel S , Terock J , Davatzikos C , Hoffmann W , Grabe HJ ((2019) ) Inflammatory markers and imaging patterns of advanced brain aging in the general population. Brain Imaging Behav 14: , 1108–1117. |
[49] | Gläser S , Ittermann T , Koch B , Völzke H , Wallaschofski H , Nauck M , Warnke C , Vogelmeier C , Schulz H , Felix SB , Ewert R , Schäper C ((2012) ) Airflow limitation, lung volumes and systemic inflammation in a general population. Eur Respir J 39: , 29–37. |
[50] | Hancox RJ , Poulton R , Greene JM , Filsell S , McLachlan CR , Rasmussen F , Taylor DR , Williams MJA , Williamson A , Sears MR ((2007) ) Systemic inflammation and lung function in young adults. Thorax 62: , 1064–1068. |
[51] | Lotze M , Domin M , Gerlach FH , Gaser C , Lueders E , Schmidt CO , Neumann N ((2019) ) Novel findings from 2,838 adult brains on sex differences in gray matter brain volume. Sci Rep 9: , 1671. |
[52] | Vuoksimaa E , Panizzon MS , Franz CE , Fennema-Notestine C , Hagler DJ , Lyons MJ , Dale AM , Kremen WS ((2018) ) Brain structure mediates the association between height and cognitive ability. Brain Struct Funct 223: , 3487–3494. |
[53] | Quanjer PH , Tammeling GJ , Cotes JE , Pedersen OF , Peslin R , Yernault J-C ((1993) ) Lung volumes and forced ventilatory flows. Eur Respir J 6: , 5–40. |
[54] | Chhabra SK ((1998) ) Forced vital capacity, slow vital capacity, or inspiratory vital capacity: Which is the best measure of vital capacity? J Asthma 35: , 361–365. |
[55] | Schünemann HJ , McCann S , Grant BJB , Trevisan M , Muti P , Freudenheim JL ((2002) ) Lung function in relation to intake of carotenoids and other antioxidant vitamins in a population-based study. Am J Epidemiol 155: , 463–471. |
[56] | Lopez-Garcia E , Schulze MB , Fung TT , Meigs JB , Rifai N , Manson JE , Hu FB ((2004) ) Major dietary patterns are related to plasma concentrations of markers of inflammation and endothelial dysfunction. Am J Clin Nutr 80: , 1029–1035. |
[57] | Melo van Lent D , O’Donnell A , Beiser AS , Vasan RS , DeCarli CS , Scarmeas N , Wagner M , Jacques PF , Seshadri S , Himali JJ , Pase MP ((2021) ) Mind Diet adherence and cognitive performance in the Framingham Heart Study. J Alzheimers Dis 82: , 827–839. |