Associations Between Insulin-Like Growth Factor-1 and Resting-State Functional Connectivity in Cognitively Unimpaired Midlife Adults
Abstract
Background:
Insulin-like growth factor (IGF)-1 plays an important role in Alzheimer’s disease (AD) pathogenesis and increases disease risk. However, prior research examining IGF-1 levels and brain neural network activity is mixed.
Objective:
The present study investigated the relationship between IGF-1 levels and 21 neural networks, as measured by functional magnetic resonance imaging (fMRI) in 13,235 UK Biobank participants.
Methods:
Linear mixed models were used to regress IGF-1 against the intrinsic functional connectivity (i.e., degree of network activity) for each neural network. Interactions between IGF-1 and AD risk factors such as Apolipoprotein E4 (APOE4) genotype, sex, AD family history, and age were also tested.
Results:
Higher IGF-1 was associated with more network activity in the right Executive Function neural network. IGF-1 interactions with APOE4 or sex implicated motor, primary/extrastriate visual, and executive function related neural networks. Neural network activity trends with increasing IGF-1 were different in different age groups. Higher IGF-1 levels relate to much more network activity in the Sensorimotor Network and Cerebellum Network in early-life participants (40–52 years old), compared with mid-life (52–59 years old) and late-life (59–70 years old) participants.
Conclusion:
These findings suggest that sex and APOE4 genotype may modify the relationship between IGF-1 and brain network activities related to visual, motor, and cognitive processing. Additionally, IGF-1 may have an age-dependent effect on neural network connectivity.
INTRODUCTION
Alzheimer’s disease (AD) is an irreversible, progressive neurodegenerative disease [1] characterized by profound brain atrophy and neuronal death [2]. AD hallmarks include amyloid-β (Aβ) oligomerization and hyperphosphorylated tau [3]. Despite over a hundred years of research [4], we still do not have treatments which can stop or slow disease onset and progression [5]. Therefore, it is necessary to continue identifying potential biomarkers or mechanisms that contribute to AD neuropathology. In recent years, metabolic dysfunction has emerged as a potential contributing factor which is related to brain atrophy [6], regional amyloid [7], and regional tau [8]. Most of this work has focused on insulin resistance, as well as insulin-like growth factor-1 (IGF-1) which is an upstream effector that may play an important role in AD etiopathogenesis [9].
IGF-1 can be produced in many peripheral organs [10] and is essential in nervous system development and function [11]. Through the IGF-1 receptor (IGF-1R), IGF-1 can activate Akt and ERK pathways to promote neuronal differentiation, survival, and synapse formation [12]. IGF-1 also mediates neuroprotection [13] and regulates neurogenesis [14]. IGF-1 is transported into the central nervous system (CNS) through the choroid plexus from plasma [15], and it can also be produced locally in the CNS to a limited extent [16]. Even among AD patients without diabetes, insulin signaling disruption has been observed postmortem. Indeed, insulin resistance is an early and common feature in the AD brain which is accompanied by IGF-1 resistance [17]. Evidence suggests that IGF-1 and insulin work separately through different cognate receptor signals that lead to their pleiotropic effects. Specifically, for AD cases without diabetes, responses to insulin and IGF-1 of the hippocampal formation (HF) and to a lesser degree cerebellar cortex, are abrogated through the IR⟶IRS-1⟶PI3K and IGF-1R⟶IRS-2⟶PI3K signaling pathways respectively [17].
IGF-1 is also important in regulating AD pathology biomarkers. Carro et al. found that serum IGF-1 could regulate brain Aβ protein levels with greater IGF-1 reducing Aβ burden [18]. IGF-1 signaling in some AD mice models can delay Aβ accumulation and toxicity [19]. Additionally, studies have shown that IGF-1 inhibits phosphorylation of abnormal tau and Aβ deposition, both in vivo and in vitro [9]. In the aging brain of IGF-1 knockout mice (Igf1–/–), tau phosphorylation was significantly increased on Ser-396 and Ser-202 residues, which are target sites for glycogen synthase kinases [20]. Insulin/IGF-1 activated PI3K/Akt/GSK-3β signaling may also be involved in some tauopathies [21]. In mice, the pro-atherosclerotic diet inhibits insulin/IGF-1 signaling, increases insulin resistance, and leads to tau phosphorylation [22]. Therefore, insulin and IGF-1 are considered promising therapeutic targets for cognitive decline and AD [12].
However, the role of IGF-1 in the brain is still complicated and controversial [23]. Westwood et al. suggested that adults with lower levels of IGF-1 in serum are at a higher risk of developing AD-related dementia [9]. Conversely, another study found that lower serum IGF-1 levels may be protective against cognitive impairment in older adult women [24]. Spatial memory accuracy was improved, and more immature neurons were observed in the dentate gyrus of the hippocampus of 28-month-old Sprague-Dawley female rats, after 18-day intracerebroventricular IGF-I gene therapy [25]. This area is critical for learning and memory.
While brain atrophy typifies AD, it is also useful to look at brain biomarkers that are sensitive to IGF-1 and may reflect earlier disease processes. Resting-state functional magnetic resonance imaging (fMRI) extrapolates brain neural networks [26], which can predict cognitive or emotional behavior [27]. More importantly, fMRI has been used to detect early changes in brain activity for AD-related cognitive impairment [28]. There is generally a stepwise decrease in the intrinsic functional connectivity of neural networks (i.e., degree of network activation) in older adults with AD and mild cognitive impairment (MCI) [29]. However, some independent component analysis studies have reported inconsistencies in this trend for adults with MCI or AD [30]. Specifically, some MCI patients showed less connectivity in the posterior default mode network (DMN), but more connectivity in the ventral and anterior DMN [31]. Another study reported some MCI patients showed less activity in the bilateral precuneus/posterior cingulate cortex, the right inferior parietal lobule, and the left fusiform gyrus, but more activity in the left prefrontal cortex, the inferior parietal lobule, and middle temporal gyrus [32].
Several key AD risk factors, such as apolipoprotein E4 (APOE4) [33], AD family history [34], age [35], and sex [36] are related to AD-like effects on neurovascular coupling, which reflects brain activity. Utilizing fMRI allows one to assess how these risk factors impact the brain from the asymptomatic phase to more observable neuropsychological changes. We hypothesized that these risk factors may interact with IGF-1 to show different association patterns in brain network connectivity. There is evidence suggesting that with aging, primary sensory and cognitive network would decline and network connectivity would reduce [37]. A Mayo Clinic Study of Aging reported that higher IGF-1 levels would be associated with better cognitive and functional performance among mid-age to old female compared with male [38]. IGF-1 signaling alterations were reported to be related with increased dementia incidence in APOE4 carriers [39]. These association effect of IGF-1 on brain network connectivity may be differed by, age, sex, and APOE4 status.
Therefore, using a large, cognitively unimpaired middle-aged to early aged cohort from the UK Biobank [40], we examined if IGF-1 alone or in conjunction with AD risk factors was related to differences in neural network connectivity that reflect AD-like changes. While most studies have focused on the DMN [29] in the context of AD, less is known about other networks that govern relevant processes like executive function or auditory processing. Thus, we systematically explored these association in a latent set of 21 non-noise neural networks.
MATERIALS AND METHODS
Cohort and participants
The UK Biobank cohort includes more than 500,000 middle-aged and older adults at baseline from 22 assessment centers across the United Kingdom [40]. Data collection began in 2006 and is currently ongoing in a subset of participants. UK Biobank encompasses sociodemographic, health, biological, lifestyle, cognitive, neuroimaging, and genetic measures. Informed consent was completed prior to baseline examination. A sub-cohort of total 13,235 participants with MRI, the IGF-1 serum marker, and demographics data were examined in the present study. Analyses with genetic data were limited to 8,989 participants with complete data.
To minimize confounding effects of disease on neural network outcomes, we excluded participants with neurological disorders as classified by ICD-10 codes. Specifically, we excluded adults with one or more central nervous system diseases (G00–G99), cerebrovascular diseases (I60–I69), and mental and behavioral disorders including mood disorders and neurodegenerative diseases such as dementia (F00–F99).
As described in the Supplementary Material, participants who had central nervous system disorders, cerebrovascular diseases, and any of the dementias were excluded.
Resting state fMRI: Acquisition and processing
One of three Siemens Skyra 3T units with a 32-channel RF receiver head coil (Siemens Medical Solutions, Erlangen, Germany) was used to scan participants as described [41]. Briefly, during scanning, participants were asked to keep their eyes open and focus on a crosshair without thinking about anything specifically. Each scan took 6 min and 10 s, resulting in the acquisition of 490 images (TR = 735 ms; TE = 39 ms; 2.4×2.4×2.4 mm voxel resolution; 88×88×64 matrix, multiband factor = 8, in-plane acceleration factor = 1, flip angle 52°). FSL tools were used to grand mean intensity normalize, high-pass temporal filter with sigma = 50.0 s, and motion correct the 4D dataset. Then, the EPI and GDC were unwarped and denoised by using ICA+FIX processing. In total, twenty-one spatially orthogonal, non-noise, distinctive Independent Components (ICs) were derived through FMRIB’s MELODIC that resulted in distinct resting neural networks. These ICs can be visualized online using the Papaya viewer provided: https://www.fmrib.ox.ac.uk/ukbiobank/group_means/rfMRI_ICA_d25_good_nodes.html. To derive intrinsic functional connectivity for each participant, a given IC was spatially backprojected onto their EPI scan. The mean degree of activation was derived from their original T-value map, then converted to a Z-score for easier interpretation. The mean degree of activation for each participant was then used in statistical analyses. More specific details are in UK Biobank white papers (https://biobank.ctsu.ox.ac.uk/crystal/crystal/docs/brain_mri.pdf). The neural networks were described by an expert (AAW) after viewing the activation maps (see Supplementary Table 1).
Insulin-like growth factor 1 (IGF-1)
Approximately 45 mL of blood was collected from each participant, and aliquots were processed and stored as described [42]. IGF-1 was measured in serum using chemiluminescent immunoassay and is expressed as nmol/L. Samples were collected at the baseline visit (∼2008) and a subsequent visit (∼2012). The average IGF-1 levels between the two visits were used for analytic purposes.
Apolipoprotein E (APOE) status
Genotyping was completed via the UK BiLEVE Axiom or UK Biobank Axiom array [43]. The single nucleotide polymorphisms (SNPs) rs7412 and rs429358 were used to determine the APOE ɛ2, ɛ3, and ɛ4 isoforms. Participants were classified as being ɛ4 non-carriers or carriers. Participants with the ɛ2/ɛ4 haplotype were excluded from analyses.
Covariates
Covariates included age at baseline (in years) and sex (male versus female). Additionally, risk factors or markers for vascular damage were used as covariates. This included alcohol status, smoking status, and arterial stiffness. Alcohol status was categorized as never, previous, or current drinker. Smoking status was categorized as never, previous, or current smoker. Arterial stiffness was determined by the time between peaks of the waveform (the peak-to-peak time) and the person’s height.
Statistical analyses
All analyses were completed using SPSS for Windows version 20 (IBM Corp., Armonk, NY). Linear mixed models were used to regress IGF-1 against each resting state IC. This approach was agnostic and exploratory because of the large number of comparisons made. The rationale was to systematically look at associations in not just the DMN, but other networks that underlie executive function and memory [29]. Nonetheless, to limit type 1 error, omnibus MANCOVA testing was first done for main effects and interaction tests. A significant omnibus test ensures that there is an overall effect of interest among dependent variables, with a family-wise error rate of p≤0.05 [44].
Initial analyses tested the main effect of IGF-1 on each neural network with covariates previously listed. Interactions were also explored with age, sex, and APOE4 status, to see if these variables moderated the relationship between IGF-1 and neural network connectivity strength. Analyses with genomics data were limited to 8,989 participants with complete data. Significance was considered at p < 0.05 for main effects, while interactions had an alpha = 0.10 to compensate for the loss of statistical power [45, 46].
RESULTS
Demographics and data summaries
Demographics and baseline characteristics are listed in Table 1. Supplementary Table 1 lists and describes all 21 non-noise derived neural networks.
Table 1
Characteristics of participants
Characteristic | ||
Baseline Age, mean (SD), y | 55.11 (7.51) | Range: 40–70 |
Female, % | 52.62 | |
APOE ɛ4 Status, % | 26.12a | |
Family History of AD, % | 23.97 | |
Smoking Status, % | ||
Never | 60.36 | |
Previous | 33.11 | |
Current | 6.53 | |
Alcohol Status, % | ||
Never | 2.52 | |
Previous | 2.12 | |
Current | 95.36 | |
Arterial Stiffness, mean (SD) | 9.67 (3.65) | Range: 1.54–308.37 |
IGF-1, mean (SD), nmol/L | 21.90 (5.49) | Range: 2.81–85.89 |
AD, Alzheimer’s disease; APOE, apolipoprotein E; IGF-1, insulin-like growth factor 1. All measures were obtained at baseline with the exception of arterial stiffness (collected over three visits and averaged) and IGF-1 (collected over two visits and averaged). aSub-sample of 8,989 participants.
IGF-1 main effects
The omnibus test for the main effect of IGF-1 was non-significant. Subsequently, results are exploratory in nature. Higher IGF-1 levels were only associated with more intrinsic functional connectivity in the right Executive Function neural network (IC 13; β= 0.0013, SE = 0.0006, p = 0.040).
IGF-1 by APOE4 status interactions
The omnibus test for the interaction of IGF-1 and APOE4 genotypes was non-significant, rendering results exploratory. In general, higher IGF-1 levels were associated with greater network activity for both non-APOE4 carriers and especially APOE4 carriers (see Table 2). This was apparent in the Primary Visual (IC 8), Sensorimotor (IC 12), and Motor Execution (IC 11) networks. More diffuse networks included left Executive Function (IC 5) and a network pairing the “what” pathway with prefrontal cortex (IC 21). For example, in Fig. 1 showing IC21, higher IGF-1 corresponded to positive slope in APOE4 carriers for neural network functional connectivity strength.
Table 2
Estimates by group for IGF-1 interactions with AD risk factors
Component | APOE Status | Sex | Age | |||||||||||
APOE4– | APOE4+ | Women | Men | Early-Life | Mid-Life | Late-Life | ||||||||
Beta | SE | Beta | SE | Beta | SE | Beta | SE | Beta | SE | Beta | SE | Beta | SE | |
IC1 | 0.0070 | 0.0003 | 0.0086 | 0.0005 | 0.0064 | 0.0003 | 0.0080 | 0.0003 | 0.0022 | 0.0002 | 0.0014 | 0.0001 | 0.0009 | 0.0002 |
IC2 | 0.0026 | 0.0002 | 0.0067 | 0.0004 | 0.0012 | 0.0002 | 0.0045 | 0.0002 | 0.0007 | 0.0002 | –0.0021 | 0.0001 | –0.0004 | 0.0002 |
IC3 | 0.0054 | 0.0002 | 0.0078 | 0.0004 | 0.0050 | 0.0002 | 0.0060 | 0.0003 | 0.0016 | 0.0001 | 0.0004 | 0.0002 | 0.0027 | 0.0001 |
IC4 | 0.0054 | 0.0003 | 0.0099 | 0.0005 | 0.0038 | 0.0003 ** | 0.0076 | 0.0004 ** | 0.0015 | 0.0002 | –0.0012 | 0.0001 | –0.0006 | 0.0002 |
IC5 | 0.0046 | 0.0002 ** | 0.0097 | 0.0004 ** | 0.0053 | 0.0002 | 0.0057 | 0.0003 | 0.0035 | 0.0002 | 0.0005 | 0.0001 | 0.0015 | 0.0001 |
IC6 | 0.0050 | 0.0002* | 0.0090 | 0.0004* | 0.0052 | 0.0002 | 0.0050 | 0.0002 | 0.0014 | 0.0002 | 0.0022 | 0.0001 | 0.0026 | 0.0002 |
IC7 | 0.0030 | 0.0002 | 0.0055 | 0.0003 | 0.0031 | 0.0002 | 0.0048 | 0.0002 | 0.0011 | 0.0001 | –0.0016 | 0.0001 | 0.0005 | 0.0001 |
IC8 | 0.0080 | 0.0004 ** | 0.0178 | 0.0007 ** | 0.0073 | 0.0004 | 0.0112 | 0.0005 | 0.0056 | 0.0003 | 0.0013 | 0.0002 | –0.0008 | 0.0003 |
IC9 | 0.0045 | 0.0003 | 0.0069 | 0.0004 | 0.0029 | 0.0002 | 0.0039 | 0.0003 | 0.0001 | 0.0002 | 0.0005 | 0.0002 | 0.0010 | 0.0002 |
IC10 | 0.0060 | 0.0003* | 0.0119 | 0.0005* | 0.0042 | 0.0002 | 0.0051 | 0.0002 | 0.0042 | 0.0003 | 0.0006 | 0.0004 | 0.0042 | 0.0004 |
IC11 | 0.0043 | 0.0002 ** | 0.0110 | 0.0004 ** | 0.0041 | 0.0001 | 0.0046 | 0.0002 | 0.0030 | 0.0003 | 0.0018 | 0.0003 | 0.0052 | 0.0003 |
IC12 | 0.0056 | 0.0002 ** | 0.0120 | 0.0004 ** | 0.0055 | 0.0002 | 0.0065 | 0.0002 | 0.0062 | 0.0002 | 0.0009 | 0.0002 ** | 0.0024 | 0.0002 |
IC13 | 0.0043 | 0.0001* | 0.0074 | 0.0002* | 0.0048 | 0.0001 | 0.0037 | 0.0002 | 0.0024 | 0.0001 | 0.0014 | 0.0001 | 0.0032 | 0.0001 |
IC14 | 0.0039 | 0.0002 | 0.0053 | 0.0003 | 0.0030 | 0.0002 ** | 0.0047 | 0.0002 ** | 0.0021 | 0.0001 | –0.0003 | 0.0001 | 0.0008 | 0.0001 |
IC15 | 0.0042 | 0.0002 | 0.0059 | 0.0003 | 0.0037 | 0.0001 | 0.0044 | 0.0002 | 0.0034 | 0.0001 | 0.0002 | 0.0001 ** | 0.0006 | 0.0001 |
IC16 | 0.0065 | 0.0003 | 0.0102 | 0.0005 | 0.0065 | 0.0003 | 0.0058 | 0.0003 | 0.0042 | 0.0002 | 0.0008 | 0.0002 | 0.0020 | 0.0002 |
IC17 | 0.0063 | 0.0003* | 0.0113 | 0.0005* | 0.0059 | 0.0003 | 0.0063 | 0.0003 | 0.0037 | 0.0003 | 0.0007 | 0.0003 | 0.0028 | 0.0003 |
IC18 | 0.0046 | 0.0002 | 0.0064 | 0.0003 | 0.0054 | 0.0002 | 0.0044 | 0.0002 | 0.0025 | 0.0001 | –0.0006 | 0.0001 | 0.0017 | 0.0001 |
IC19 | 0.0042 | 0.0003* | 0.0104 | 0.0005* | 0.0022 | 0.0003 ** | 0.0076 | 0.0003 ** | 0.0032 | 0.0002 | –0.0007 | 0.0002 | –0.0011 | 0.0002 |
IC20 | 0.0035 | 0.0002* | 0.0067 | 0.0004* | 0.0037 | 0.0002 | 0.0046 | 0.0002 | 0.0016 | 0.0001 | –0.0021 | 0.0001 | –0.0006 | 0.0001 |
IC21 | 0.0039 | 0.0002 ** | 0.0106 | 0.0003 ** | 0.0041 | 0.0002 | 0.0052 | 0.0002 | 0.0016 | 0.0001 | 0.0009 | 0.0001 | 0.0025 | 0.0001 |
*p < 0.10; **p < 0.05.
Fig. 1
The association between IGF-1 levels and intrinsic functional connectivity (i.e., neural network activity) in adults without or with an APOE4 genotype (“positive”, “negative”). Blue circles and red stars respectively represent APOE4 negative and APOE4 positive participants. *p < 0.05.
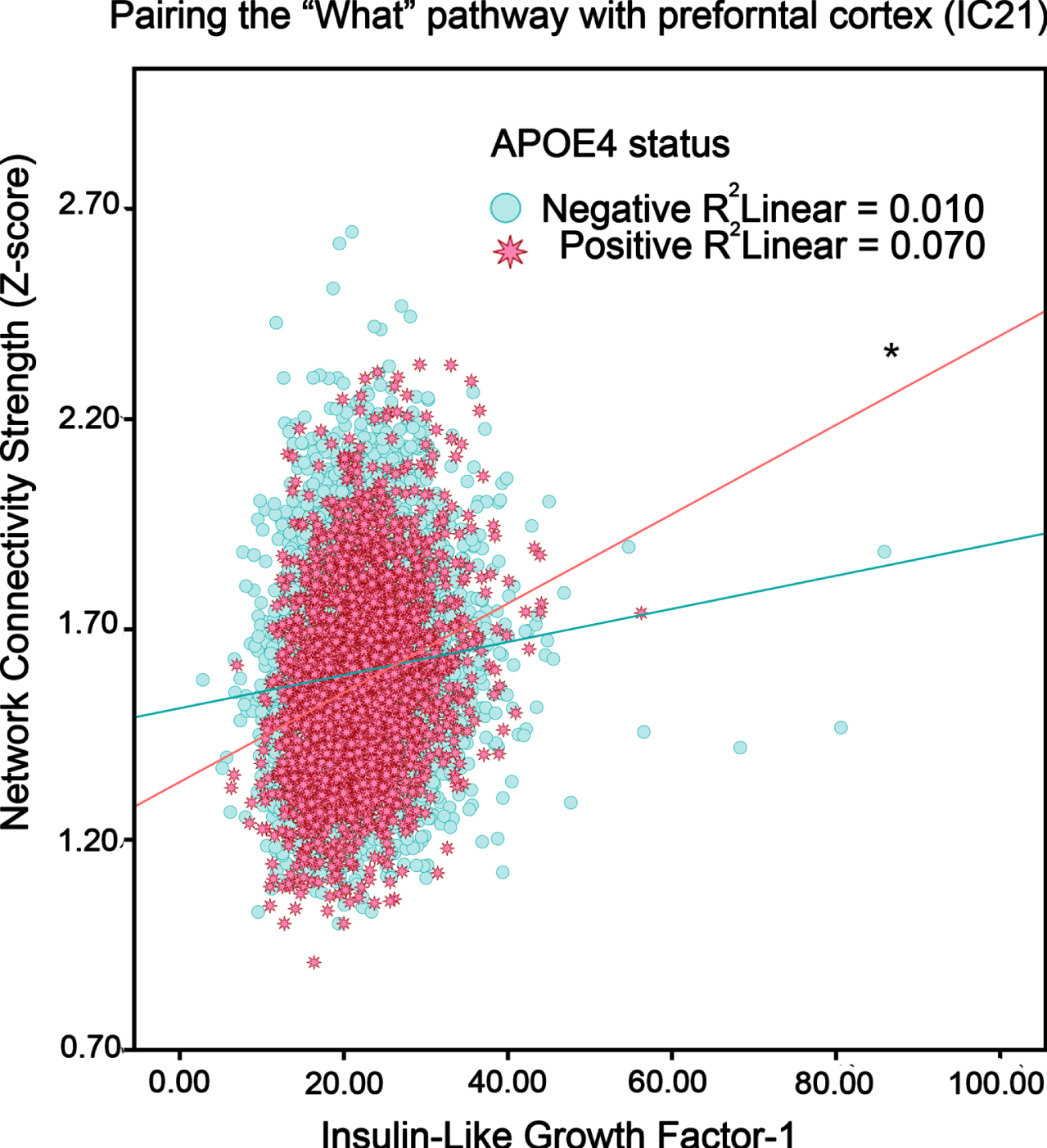
IGF-1 by sex interactions
The omnibus testing for the interaction of IGF-1 and sex was non-significant. Thus, results should be interpreted with caution. Significant sex by IGF-1 interactions showed that relative to women, higher IGF-1 levels in men corresponded to more activity in neural networks such as Extrastriate Visual (IC 4), Fronto-Cingular (IC 14), and posterior Primary Visual (IC 19). Table 2 lists Beta estimates by sex, while Fig. 2 shows that men with higher IGF-1 had more Fronto-Cingular network activity, whereas no association existed for women. Network connectivity of IC 4 and IC 19 were presented in Supplementary Figures 1 and 2.
Fig. 2
The association between IGF-1 levels and Fronto-Cingular network (i.e., neural network activity) in adults in different sex (“Female”, “Male”). Pink squares and blue triangles respectively represent Female and Male participants. *p < 0.05.

IGF-1 by age interactions
The omnibus test for the interaction of IGF-1 and age was non-significant. Thus, results are exploratory. An IGF-1×Age interaction was found for Sensorimotor Network and Cerebellum Network (IC 12 and IC 15; Table 2). To better assess the effects per year increase in age, participants were split to tertile groups: early midlife (40–52 years old), midlife (52–59 years old), and late midlife (≥59 years old). Figure 3 shows that higher IGF-1 was linked to more activity only in early midlife.
Fig. 3
The association between IGF-1 levels and localized networks like Sensorimotor (i.e., neural network activity) in adults in different ages (“Early Mid- life”, “Mid-life”, and “Late Mid-life”). Blue circles, red circle and green circles respectively represent early mid-life (40–52 years), mid-life (52–59 years) and later-life (≥59 years) participants. *p < 0.05.
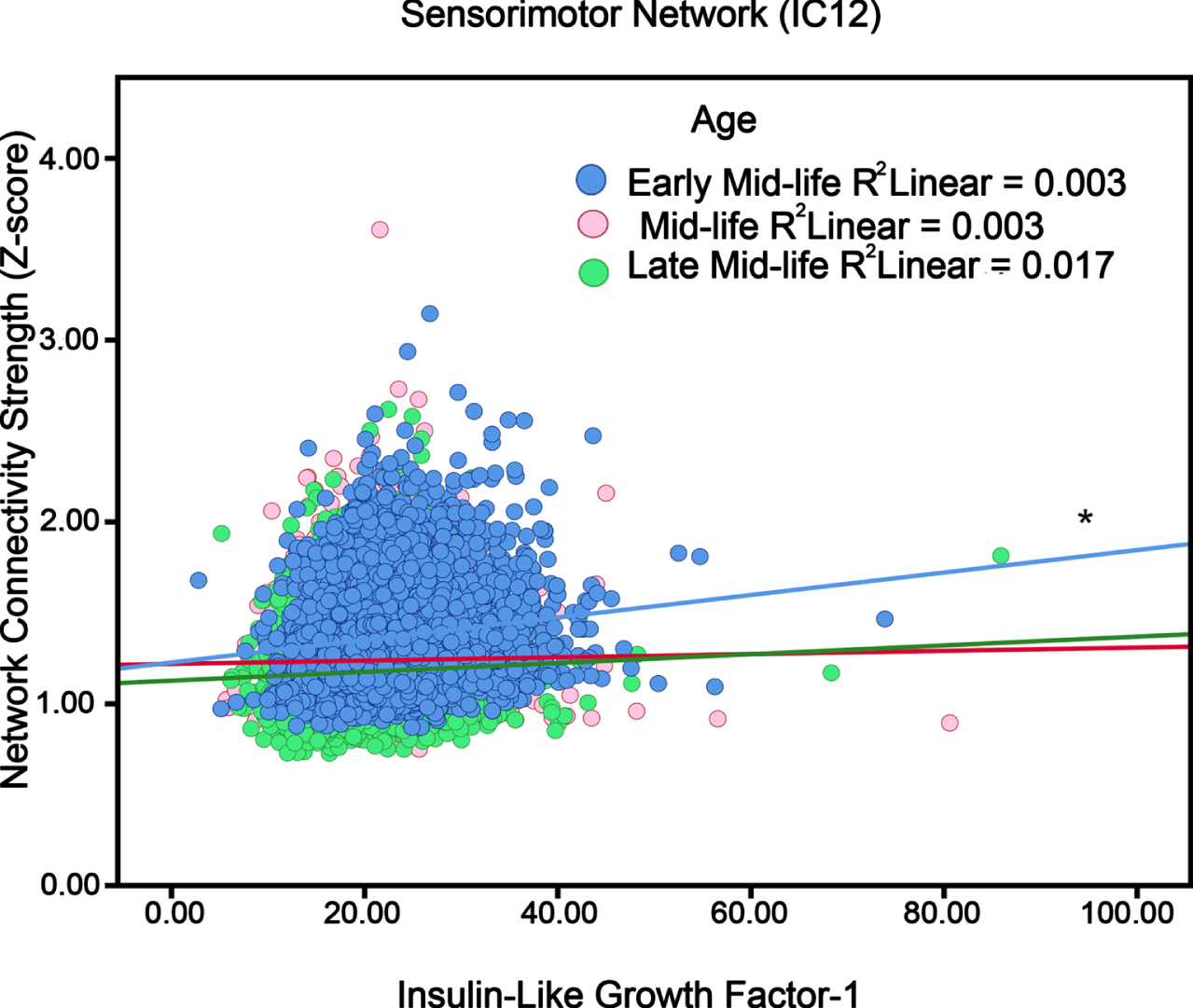
DISCUSSION
In the present study, we explored relationships between IGF-1 levels and neural network activity (i.e., intrinsic neural network functional connectivity). Further, different AD risk factors, APOE4 genotype, sex, and age, were tested as moderators. The main effects we observed included IGF-1 levels associated with more network connectivity in the Right Executive Function neural network located in the prefrontal region. This positive association may be beneficial for cognitive tasks involved with planning and adequately processing stimuli [47]. Insulin infusion has been associated with increased regional cerebral glucose metabolic rate (CMRglu) in prefrontal cortices, an area that plays an important role in memory [48]. Insulin resistance is also associated with prefrontal hypometabolism at baseline (i.e., less CMRglu) [49]. Taken together, IGF-1 and downstream effectors like insulin may play a role in maintenance of the executive function network.
APOE4 carriers with higher IGF-1 levels showed more connectivity in many neural networks, including Left Executive Function (IC 5) and a network described as the ‘what’ pathway with coverage in prefrontal cortex (IC 21). These implicated networks correspond to AD-like hypometabolism or atrophy in APOE4 carriers, including parietotemporal, frontal areas [50], and medial temporal lobe [51]. Some studies suggest that the APOE4 genotype could contribute to CMRglu abnormalities independent of insulin resistance [49]. Rodent models have demonstrated that APOE4 inhibits glucose metabolism through glucose transporters and glycolysis [52]. In a recent study, APOE4 carriers with higher levels of IGF-1 stimulation had a greater risk of developing dementia [53].
Our results suggest that men had greater activity with increasing IGF-1 levels for Extrastriate Visual, Fronto-Cingular, and Posterior Primary Visual neural networks. The lack of association with women was curious, as a recent study suggested that higher IGF-1 levels among women are related to better attention, visuospatial, and global cognitive performance [38]. Other reports suggest worse cognitive outcomes [54]. However, these discrepancies could be due to differences in age [38].
Aging itself is regarded as a complex process in which multiple conditions could potentially emerge, including metabolic dysregulation that has been tied to AD risk [55]. Both sensory and cognitive networks show evidence of age-related decline [37], therefore IGF-1 may be linked with network activity strength due to age effects. For instance, our results suggest differential effects of IGF-1 by age. For the Sensorimotor and Cerebellum networks, only early midlife participants showed IGF-1 level associations with greater network activity. Recent research has suggested that lower serum IGF-1 has shown different effects on cognitive function. In participants over 90 years old, it was shown to be beneficial [24]; however with adults around the age of 70, the effect was unfavorable [56].
This is the first study to analyze brain network activities, the marker of metabolic function IGF-1, and several different AD risk factors (as moderators) together. This study is based on a large population sample, and the pattern of results offer more insights than focusing on only the DMN. There are some limitations in this study. Associations that we discovered were not longitudinal. IGF-1 associations were modest in effect size, where longitudinal data may help to confirm robustness and utility of different relationships. According to other research, neural network activity may substantially differ in mid-life, late-life, and “oldest old” people (over 90 s) [24]. Therefore, the ages range (40–70 years old) of our study may not be wide enough. Finally, due to the exploratory nature of this study into all latent neural networks, it was not feasible to correct for type 1 error using Bonferroni or other test-level corrections. While we tried to use MANCOVA omnibus analyses to control type 1 error, the number of outcomes made it unlikely these omnibuses would be significant. Therefore, results should be interpreted with caution.
In conclusion, higher IGF-1 showed modest positive relationships with several neural networks as modified by APOE4 status, age, and sex.
ACKNOWLEDGMENTS
This research has been conducted using UK Biobank Resource under Application Number 25057.
FUNDING
This work is supported by Iowa State University, National Institutes of Health (NIH) R00 AG047282, and Alzheimer’s Association Research Grant to Promote Diversity AARGD-17-529552. Funding sources have no influence on the development or conduct of this study.
CONFLICT OF INTEREST
The authors have no conflict of interest to report.
DATA AVAILABILITY
The data in this study is owned by the UK Biobank (https://www.ukbiobank.ac.uk), a data repository that can be accessed through the UK Biobank Access Management System (https://www.ukbiobank.ac.uk/register-apply). Due to the legal agreement, as researchers, we do not have permission to share the data. We are not entitled to republish or make any UK Biobank data available at the individual participant level. All analyses and intellectual content separate from UK Biobank are available on a request basis.
SUPPLEMENTARY MATERIAL
[1] The supplementary material is available in the electronic version of this article: https://dx.doi.org/10.3233/JAD-220608.
REFERENCES
[1] | Lawrence E , Vegvari C , Ower A , Hadjichrysanthou C , De Wolf F , Anderson RM ((2017) ) A systematic review of longitudinal studies which measure Alzheimer’s disease biomarkers, J Alzheimers Dis 59: , 1359–1379. |
[2] | Small DH , Mok SS , Bornstein JC ((2001) ) Alzheimer’s disease and Abeta toxicity: From top to bottom, Nat Rev Neurosci 2: , 595–598. |
[3] | Lashley T , Rohrer JD , Mead S , Revesz T ((2015) ) Review: An update on clinical, genetic and pathological aspects of frontotemporal lobar degenerations, Neuropathol Appl Neurobiol 41: , 858–881. |
[4] | Alzheimer A , Stelzmann RA , Schnitzlein HN , Murtagh FR ((1995) ) An English translation of Alzheimer’s 1907 paper, “Uber eine eigenartige Erkankung der Hirnrinde", Clin Anat 8: , 429–431. |
[5] | Lane CA , Hardy J , Schott JM ((2018) ) Alzheimer’s disease, Eur J Neurol 25: , 59–70. |
[6] | Willette AA , Xu G , Johnson SC , Birdsill AC , Jonaitis EM , Sager MA , Hermann BP , La Rue A , Asthana S , Bendlin BB ((2013) ) Insulin resistance, brain atrophy, and cognitive performance in late middle-aged adults, Diabetes Care 36: , 443–449. |
[7] | Willette AA , Johnson SC , Birdsill AC , Sager MA , Christian B , Baker LD , Craft S , Oh J , Statz E , Hermann BP , Jonaitis EM , Koscik RL , La Rue A , Asthana S , Bendlin BB ((2015) ) Insulin resistance predicts brain amyloid deposition in late middle-aged adults, Alzheimers Dement 11: , 504–510e501. |
[8] | Pappas C , Klinedinst BS , Le S , Wang Q , Larsen B , McLimans K , Lockhart SN , Allenspach-Jorn K , Mochel JP , Willette AA , Alzheimer’s Disease Neuroimaging Initiative ((2020) ) CSF glucose tracks regional tau progression based on Alzheimer’s disease risk factors, Alzheimers Dement (N Y) 6: , e12080. |
[9] | Westwood AJ , Beiser A , Decarli C , Harris TB , Chen TC , He XM , Roubenoff R , Pikula A , Au R , Braverman LE , Wolf PA , Vasan RS , Seshadri S ((2014) ) Insulin-like growth factor-1 and risk of Alzheimer dementia and brain atrophy, Neurology 82: , 1613–1619. |
[10] | Labandeira-Garcia JL , Costa-Besada MA , Labandeira CM , Villar-Cheda B , Rodriguez-Perez AI ((2017) ) Insulin-like growth factor-1 and neuroinflammation, Front Aging Neurosci 9: , 365. |
[11] | Lewitt MS , Boyd GW ((2019) ) The role of insulin-like growth factors and insulin-like growth factor-binding proteins in the nervous system, Biochem Insights 12: , 1178626419842176. |
[12] | Kim B , Elzinga SE , Henn RE , McGinley LM , Feldman EL ((2019) ) The effects of insulin and insulin-like growth factor I on amyloid precursor protein phosphorylation in and models of Alzheimer’s disease, Neurobiol Dis 132: , 104541. |
[13] | Fernandez AM , Torres-Aleman I ((2012) ) The many faces of insulin-like peptide signalling in the brain, Nat Rev Neurosci 13: , 225–239. |
[14] | Mir S , Cai W , Carlson SW , Saatman KE , Andres DA ((2017) ) IGF-1 mediated neurogenesis involves a novel RIT1/Akt/Sox2 cascade, Sci Rep 7: , 3283. |
[15] | Santi A , Genis L , Torres Aleman I ((2018) ) A coordinated action of blood-borne and brain insulin-like growth factor I in the response to traumatic brain injury, Cereb Cortex 28: , 2007–2014. |
[16] | Rodriguez-Perez AI , Borrajo A , Diaz-Ruiz C , Garrido-Gil P , Labandeira-Garcia JL ((2016) ) Crosstalk between insulin-like growth factor-1 and angiotensin-II in dopaminergic neurons and glial cells: Role in neuroinflammation and aging, Oncotarget 7: , 30049–30067. |
[17] | Talbot K , Wang HY , Kazi H , Han LY , Bakshi KP , Stucky A , Fuino RL , Kawaguchi KR , Samoyedny AJ , Wilson RS , Arvanitakis Z , Schneider JA , Wolf BA , Bennett DA , Trojanowski JQ , Arnold SE ((2012) ) Demonstrated brain insulin resistance in Alzheimer’s disease patients is associated with IGF-1 resistance, IRS-1 dysregulation, and cognitive decline, J Clin Invest 122: , 1316–1338. |
[18] | Carro E , Trejo JL , Gomez-Isla T , LeRoith D , Torres-Aleman I ((2002) ) Serum insulin-like growth factor I regulates brain amyloid-beta levels, Nat Med 8: , 1390–1397. |
[19] | Cohen E , Paulsson JF , Blinder P , Burstyn-Cohen T , Du D , Estepa G , Adame A , Pham HM , Holzenberger M , Kelly JW , Masliah E , Dillin A ((2009) ) Reduced IGF-1 signaling delays age-associated proteotoxicity in mice, Cell 139: , 1157–1169. |
[20] | Cheng CM , Tseng V , Wang J , Wang D , Matyakhina L , Bondy CA ((2005) ) Tau is hyperphosphorylated in the insulin-like growth factor-I null brain, Endocrinology 146: , 5086–5091. |
[21] | Yang L , Wang H , Liu L , Xie A ((2018) ) The role of insulin/IGF-1/PI3K/Akt/GSK3beta signaling in Parkinson’s disease dementia, Front Neurosci 12: , 73. |
[22] | Bhat NR , Thirumangalakudi L ((2013) ) Increased tau phosphorylation and impaired brain insulin/IGF signaling in mice fed a high fat/high cholesterol diet, J Alzheimers Dis 36: , 781–789. |
[23] | Gubbi S , Quipildor GF , Barzilai N , Huffman DM , Milman S ((2018) ) 40 years of IGF1: IGF1: The Jekyll and Hyde of the aging brain, J Mol Endocrinol 61: , T171–T185. |
[24] | Perice L , Barzilai N , Verghese J , Weiss EF , Holtzer R , Cohen P , Milman S ((2016) ) Lower circulating insulin-like growth factor-I is associated with better cognition in females with exceptional longevity without compromise to muscle mass and function, Aging (Albany NY) 8: , 2414–2424. |
[25] | Pardo J , Uriarte M , Console GM , Reggiani PC , Outeiro TF , Morel GR , Goya RG ((2016) ) Insulin-like growth factor-I gene therapy increases hippocampal neurogenesis, astrocyte branching and improves spatial memory in female aging rats, Eur J Neurosci 44: , 2120–2128. |
[26] | Damoiseaux JS , Rombouts SA , Barkhof F , Scheltens P , Stam CJ , Smith SM , Beckmann CF ((2006) ) Consistent resting-state networks across healthy subjects, Proc Natl Acad Sci U S A 103: , 13848–13853. |
[27] | Seeley WW , Menon V , Schatzberg AF , Keller J , Glover GH , Kenna H , Reiss AL , Greicius MD ((2007) ) Dissociable intrinsic connectivity networks for salience processing and executive control, J Neurosci 27: , 2349–2356. |
[28] | Liu Z , Zhang Y , Yan H , Bai L , Dai R , Wei W , Zhong C , Xue T , Wang H , Feng Y , You Y , Zhang X , Tian J ((2012) ) Altered topological patterns of brain networks in mild cognitive impairment and Alzheimer’s disease: A resting-state fMRI study, Psychiatry Res 202: , 118–125. |
[29] | Jones DT , Machulda MM , Vemuri P , McDade EM , Zeng G , Senjem ML , Gunter JL , Przybelski SA , Avula RT , Knopman DS , Boeve BF , Petersen RC , Jack CR Jr ((2011) ) Age-related changes in the default mode network are more advanced in Alzheimer disease, Neurology 77: , 1524–1531. |
[30] | Joo SH , Lim HK , Lee CU ((2016) ) Three large-scale functional brain networks from resting-state functional MRI in subjects with different levels of cognitive impairment, Psychiatry Investig 13: , 1–7. |
[31] | Damoiseaux JS , Prater KE , Miller BL , Greicius MD ((2012) ) Functional connectivity tracks clinical deterioration in Alzheimer’s disease, Neurobiol Aging 33: , 828.e819–830. |
[32] | Qi Z , Wu X , Wang Z , Zhang N , Dong H , Yao L , Li K ((2010) ) Impairment and compensation coexist in amnestic MCI default mode network, Neuroimage 50: , 48–55. |
[33] | Safieh M , Korczyn AD , Michaelson DM ((2019) ) ApoE4: An emerging therapeutic target for Alzheimer’s disease, BMC Med 17: , 64. |
[34] | Lyketsos CG , Tune LE , Pearlson G , Steele C ((1996) ) Major depression in Alzheimer’s disease. An interaction between gender and family history, Psychosomatics 37: , 380–384. |
[35] | Riedel BC , Thompson PM , Brinton RD ((2016) ) Age, APOE and sex: Triad of risk of Alzheimer’s disease, J Steroid Biochem Mol Biol 160: , 134–147. |
[36] | Nebel RA , Aggarwal NT , Barnes LL , Gallagher A , Goldstein JM , Kantarci K , Mallampalli MP , Mormino EC , Scott L , Yu WH , Maki PM , Mielke MM ((2018) ) Understanding the impact of sex and gender in Alzheimer’s disease: A call to action, Alzheimers Dement 14: , 1171–1183. |
[37] | Varangis E , Habeck CG , Razlighi QR , Stern Y ((2019) ) The effect of aging on resting state connectivity of predefined networks in the brain, Front Aging Neurosci 11: , 234. |
[38] | Wennberg AMV , Hagen CE , Machulda MM , Hollman JH , Roberts RO , Knopman DS , Petersen RC , Mielke MM ((2018) ) The association between peripheral total IGF-1, IGFBP-3, and IGF-1/IGFBP-3 and functional and cognitive outcomes in the Mayo Clinic Study of Aging, Neurobiol Aging 66: , 68–74. |
[39] | Galle SA , Geraedts IK , Deijen JB , Milders MV , Drent ML ((2020) ) The interrelationship between insulin-like growth factor 1, Apolipoprotein E epsilon4, lifestyle factors, and the aging body and brain, J Prev Alzheimers Dis 7: , 265–273. |
[40] | Sudlow C , Gallacher J , Allen N , Beral V , Burton P , Danesh J , Downey P , Elliott P , Green J , Landray M , Liu B , Matthews P , Ong G , Pell J , Silman A , Young A , Sprosen T , Peakman T , Collins R ((2015) ) UK biobank: An open access resource for identifying the causes of a wide range of complex diseases of middle and old age, PLoS Med 12: , e1001779. |
[41] | Miller KL , Alfaro-Almagro F , Bangerter NK , Thomas DL , Yacoub E , Xu J , Bartsch AJ , Jbabdi S , Sotiropoulos SN , Andersson JL , Griffanti L , Douaud G , Okell TW , Weale P , Dragonu I , Garratt S , Hudson S , Collins R , Jenkinson M , Matthews PM , Smith SM ((2016) ) Multimodal population brain imaging in the UK Biobank prospective epidemiological study, Nat Neurosci 19: , 1523–1536. |
[42] | Elliott P , Peakman TC , Biobank UK ((2008) ) The UK Biobank sample handling and storage protocol for the collection, processing and archiving of human blood and urine, Int J Epidemiol 37: , 234–244. |
[43] | Bycroft C , Freeman C , Petkova D , Band G , Elliott LT , Sharp K , Motyer A , Vukcevic D , Delaneau O , O’Connell J , Cortes A , Welsh S , Young A , Effingham M , McVean G , Leslie S , Allen N , Donnelly P , Marchini J ((2018) ) The UK Biobank resource with deep phenotyping and genomic data, Nature 562: , 203–209. |
[44] | Coombs WT , Algina J , Oltman DO ((1996) ) Univariate and multivariate omnibus hypothesis tests selected to control type I error rates when population variances are not necessarily equal, Rev Educ Res 66: , 137–179. |
[45] | Geijselaers SLC , Aalten P , Ramakers I , De Deyn PP , Heijboer AC , Koek HL , OldeRikkert MGM , Papma JM , Reesink FE , Smits LL , Stehouwer CDA , Teunissen CE , Verhey FRJ , van der Flier WM , Biessels GJ , Parelsnoer Institute Neurodegenerative Diseases study group ((2018) ) Association of cerebrospinal fluid (CSF) insulin with cognitive performance and CSF biomarkers of Alzheimer’s disease, J Alzheimers Dis 61: , 309–320. |
[46] | Selvin S ((2004) ) Statistical analysis of epidemiologic data, Oxford University Press, Oxford, New York. |
[47] | Fuster JM ((2019) ) The prefrontal cortex in the neurology clinic, Handb Clin Neurol 163: , 3–15. |
[48] | Anthony K , Reed LJ , Dunn JT , Bingham E , Hopkins D , Marsden PK , Amiel SA ((2006) ) Attenuation of insulin-evoked responses in brain networks controlling appetite and reward in insulin resistance: The cerebral basis for impaired control of food intake in metabolic syndrome, Diabetes 55: , 2986–2992. |
[49] | Baker LD , Cross DJ , Minoshima S , Belongia D , Watson GS , Craft S ((2011) ) Insulin resistance and Alzheimer-like reductions in regional cerebral glucose metabolism for cognitively normal adults with prediabetes or early type 2 diabetes, Arch Neurol 68: , 51–57. |
[50] | Reiman EM , Chen K , Langbaum JB , Lee W , Reschke C , Bandy D , Alexander GE , Caselli RJ ((2010) ) Higher serum total cholesterol levels in late middle age are associated with glucose hypometabolism in brain regions affected by Alzheimer’s disease and normal aging, Neuroimage 49: , 169–176. |
[51] | Soldan A , Pettigrew C , Lu Y , Wang MC , Selnes O , Albert M , Brown T , Ratnanather JT , Younes L , Miller MI , Team BR ((2015) ) Relationship of medial temporal lobe atrophy, APOE genotype, and cognitive reserve in preclinical Alzheimer’s disease, Hum Brain Mapp 36: , 2826–2841. |
[52] | Wu L , Zhang X , Zhao L ((2018) ) Human ApoE isoforms differentially modulate brain glucose and ketone body metabolism: Implications for Alzheimer’s disease risk reduction and early intervention, J Neurosci 38: , 6665–6681. |
[53] | Galle SA , van der Spek A , Drent ML , Brugts MP , Scherder EJA , Janssen J , Ikram MA , van Duijn CM ((2019) ) Revisiting the role of insulin-like growth factor-I receptor stimulating activity and the apolipoprotein E in Alzheimer’s disease, Front Aging Neurosci 11: , 20. |
[54] | Doi T , Shimada H , Makizako H , Tsutsumimoto K , Hotta R , Nakakubo S , Suzuki T ((2016) ) Insulin-like growth factor-1 related to disability among older adults. J Gerontol A Biol Sci Med Sci 71: , 797–802. |
[55] | Rettberg JR , Dang H , Hodis HN , Henderson VW , St John JA , Mack WJ , Brinton RD ((2016) ) Identifying postmenopausal women at risk for cognitive decline within a healthy cohort using a panel of clinical metabolic indicators: Potential for detecting an at-Alzheimer’s risk metabolic phenotype, Neurobiol Aging 40: , 155–163. |
[56] | Doi T , Shimada H , Makizako H , Tsutsumimoto K , Hotta R , Nakakubo S , Suzuki T ((2015) ) Association of insulin-like growth factor-1 with mild cognitive impairment and slow gait speed.. Neurobiol Aging 36: , 942–947. |