Brain Deep Medullary Veins on 7T MRI in Dutch-Type Hereditary Cerebral Amyloid Angiopathy
Abstract
Background:
Deep medullary vein (DMV) changes occur in cerebral small vessel diseases (SVD) and in Alzheimer’s disease. Cerebral amyloid angiopathy (CAA) is a common SVD that has a high co-morbidity with Alzheimer’s disease. So far, DMVs have not been evaluated in CAA.
Objective:
To evaluate DMVs in Dutch-type hereditary CAA (D-CAA) mutation carriers and controls, in relation to MRI markers associated with D-CAA.
Methods:
Quantitative DMV parameters length, tortuosity, inhomogeneity, and density were quantified on 7 Tesla 3D susceptibility weighted MRI in pre-symptomatic D-CAA mutation carriers (n = 8), symptomatic D-CAA mutation carriers (n = 8), and controls (n = 25). Hemorrhagic MRI markers (cerebral microbleeds, intracerebral hemorrhages, cortical superficial siderosis, convexity subarachnoid hemorrhage), non-hemorrhagic MRI markers (white matter hyperintensities, enlarged perivascular spaces, lacunar infarcts, cortical microinfarcts), cortical grey matter perfusion, and diffusion tensor imaging parameters were assessed in D-CAA mutation carriers. Univariate general linear analysis was used to determine associations between DMV parameters and MRI markers.
Results:
Quantitative DMV parameters length, tortuosity, inhomogeneity, and density did not differ between pre-symptomatic D-CAA mutation carriers, symptomatic D-CAA mutation carriers, and controls. No associations were found between DMV parameters and MRI markers associated with D-CAA.
Conclusion:
This study indicates that vascular amyloid-β deposition does not affect DMV parameters. In patients with CAA, DMVs do not seem to play a role in the pathogenesis of MRI markers associated with CAA.
INTRODUCTION
Deep medullary veins (DMV) originate 1-2 cm below the cortical gray matter, pass through deep medullary white matter, and drain into subependymal veins of the lateral ventricles. DMVs drain venous blood from the deep and periventricular white matter. At autopsy, periventricular venous collagenosis is associated with the presence of periventricular leukoaraiosis in patients of 60 years and older [1] and in patients with Alzheimer’s disease(AD) [2].
Radiological studies reported changes of DMVs on susceptibility weighted MRI in patients with cerebral small vessel diseases (SVD), including cerebral autosomal dominant arteriopathy with subcortical infarcts and leukoencephalopathy [3, 4], with an inverse relationship between number and/or integrity of DMVs and SVD markers on MRI, in particular with white matter hyperintensities (WMHs) [4–6]. Age also effects DMVs, with decreased number of DMVs associating with the increase of age [7]. Further, in aging an association was shown between venous pathological changes and leukoaraiosis [8], and increased DMV tortuosity in patients with early AD and mild cognitive impairment [9]. Collagenosis of DMVs is assumed to be the underlying pathophysiology of leukoaraiosis in cerebral SVDs [8]. In patients with AD, it is hypothesized that DMV abnormalities are associated with increased pulsatility of venous blood flow and possibly a remote effect of arterial amyloid-β (Aβ) deposition in the cortex on the veins of the white matter in patients with concomitant cerebral amyloid angiopathy (CAA) [9].
CAA is one of the most common forms of cerebral SVD and is highly prevalent in the elderly population [10], but also commonly (>80%) found as co-morbidity in patients with AD [11]. CAA is characterized by deposition of Aβ proteins in the walls of small leptomeningeal and cortical arterioles. Further, Aβ proteins also aggregate to some extent in leptomeningeal and cortical venules of the brain [12]. As such, the pathophysiology of CAA clearly differs from that of other non-amyloid SVDs. Currently, the effect of vascular Aβ deposition on deep venous structures has not been evaluated in patients with CAA and the relationship between DMV changes and SVD markers on MRI— especially WMH— in this population is unknown. Moreover, knowledge on occurrence of DMV changes in patients with CAA might provide insight into the underlying pathophysiology of the observed DMV changes in patients with AD, considering the high co-morbidity of CAA and AD.
Dutch-type CAA (D-CAA, also known as Hereditary Cerebral Hemorrhages with Amyloidosis-Dutch type (HCHWA-D)) is an autosomal dominant hereditary form of CAA with similar chemical composition and underlying pathology of Aβ deposits to that of sporadic CAA [13]. Symptomatology is also similar to sporadic CAA, both are characterized by intracerebral hemorrhages and cognitive impairment, but the onset of symptoms in D-CAA occurs at a relatively young age (50–60 years). Because vascular risk factors are in general not often present at this age, D-CAA can be considered a ‘pure’ model of CAA. Further, concomitant AD is also unlikely to occur in this age category. D-CAA, therefore, provides a unique opportunity to evaluate if vascular Aβ accumulation results in changes in DMVs.
The objective of this study was to evaluate if DMV changes occur in D-CAA mutation carriers, as compared to controls. The second aim was to evaluate whether these DMV changes correlate with other MRI markers associated with D-CAA.
METHODS
Study population
D-CAA mutation carriers and age-matched controls who participated in the Early Diagnosis of Amyloid angiopathy Network (EDAN) study [14] were included. The aim of the EDAN study was, among others, to identify early markers of D-CAA on 3T and 7T MRI and included adult patients who had been diagnosed with D-CAA via genetic testing, divided into either pre-symptomatic or symptomatic D-CAA mutation carriers. Subjects were considered symptomatic when they had experienced signs of the disease reported to a general practitioner [14]. Data on age, sex, and mutation status were available for all. The study was carried out in accordance with the principals of the Declaration of Helsinki and approved by the local Institutional Review Board. Written informed consent was obtained from all participants.
MRI
All subjects underwent both 7T and 3T MRI (Philips Healthcare, Best, The Netherlands). The protocol for the EDAN study consisted of a variety of sequences at both field strengths to enable the analysis of hemorrhagic and non-hemorrhagic MRI markers of CAA; for a detailed description of the sequence parameters we refer to the EDAN protocol [14]. Hemorrhagic MRI markers (cerebral microbleeds, intracerebral hemorrhages, cortical superficial siderosis, and convexity subarachnoid hemorrhages) were assessed on 7T T2*-weighted sequences. Cortical microinfarcts were scored as described previously [15]. Non-hemorrhagic MRI markers were assessed on 3T (WMHs, dilatation of perivascular spaces in basal ganglia and centrum semiovale, lacunar infarcts on fluid-attenuated inversion recovery (FLAIR), T1-weighted and T2-weighted images). Cortical grey matter regional cerebral blood flow (rCBF) was measured on 3T MRI using pseudo-continuous arterial spin labelling [16] and diffusion tensor images were acquired on 3T MRI along 32 noncollinear directions with a b-value of 1000 s/mm2 [17]. We assessed DMVs on 7T MRI dual echo 3D flow-compensated T2*-weighted gradient echo scan (TR 19.6/first TE 3 ms/second TE 15 ms, flip angle 20, FOV of 200×180×100 mm, 512 reconstructed matrix size, resulting in a voxel size of 0.39×0.45×0.30 mm reconstructed in-plane to 0.39 mm).
DMV analysis
A semi-automated method based on a previous protocol described by Kuijf et al. [18] was used for the detection and quantification of DMVs. In short, grey matter, white matter, and cerebrospinal fluid (CSF) were segmented using the 7T T1-weighted MRI of each subject. Subsequently, the ventricle segmentation was transformed to the 7T 3D flow-compensated T2*-weighted images and corrected for inhomogeneities by applying a bias field correction prior to automatic detection of DMVs. Expansion of the ventricular surface was set at a distance of 5 to 5.5 mm from the ventricles to detect DMVs that intersect with this curved 3D surface (Fig. 2). To assess DMVs that drain the white matter of the centrum semiovale, we selected slices cranial from the level of the basal ganglia and splenium of the corpus callosum. Detection of DMVs was performed by applying an automated detection algorithm, after which detected veins were evaluated individually to exclude false positives. Number of DMVs were determined per patient and the overall mean value for the number of veins per subject group (pre-symptomatic and symptomatic D-CAA mutation carriers, and controls) was obtained. Finally, a bidirectional tubular tracking was applied and values derived from the tracking procedure were used to produce the following quantitative DMV parameters: 1) the total length of each vein, to calculate the mean venous length per subject; 2) venous tortuosity, determined by taking the total length of the vein divided by the distance between start and finish of the vein in a straight line within 3D space (i.e., the Euclidean distance), to calculate a mean value for venous tortuosity per subject; 3) venous inhomogeneity, by calculating the derivative of intensity changes of veins. In more detail, occurrence and frequency of signal intensity transitions within tracked veins was counted and expressed as absolute venous inhomogeneity; a higher number of intensity transitions within a tracked vein will translate into a higher absolute venous inhomogeneity and vice versa; and lastly 4) venous density, by dividing the number of veins by the volume in which they are detected. When presence of an intracerebral hemorrhage in a single hemisphere precluded evaluation of DMVs, this hemisphere was excluded from further analysis and DMVs were only evaluated in the contralateral hemisphere. If this was the case for both hemispheres, or in case of severe motion artefacts, the individual was excluded from further analysis.
Hemorrhagic and non-hemorrhagic MRI markers and other MRI measures
DMV parameters were related to presence and number of lobar cerebral microbleeds, intracerebral hemorrhages, convexity subarachnoid hemorrhages, and presence of cortical superficial siderosis on 7T T2*-weighted sequences. DMV parameters were related to volume of WMHs and dilated perivascular spaces, according to previously described methods [19], and number of lacunar infarcts on 3T MRI. In addition, DMV parameters were related to perfusion of supratentorial cortical grey matter volume outside areas of intracerebral hemorrhages to make sure we measured grey matter [16] and to diffusion tensor imaging (DTI) parameters fractional anisotropy (FA), mean diffusivity (MD), axial diffusivity (AD), and radial diffusivity (RD) [17]. 3D T1-weighted images were co-registered with a standard brain from which a ventricle segmentation was selected. The transformed ventricle segmentation was subsequently used to determine ventricular volume for each patient. Total intracranial volume (ICV) and total brain volume (TBV) were also obtained from 3T T1 weighted images.
Statistical analysis
Differences in group means between D-CAA mutation carriers and control subjects were tested with the independent t-test. A univariate general linear model was used to adjust for sex, age, and TBV as percentage of intracranial volume (TBV% ICV). The following between group comparisons were tested: 1) all D-CAA mutation carriers versus controls; 2) pre-symptomatic D-CAA mutation carriers versus controls; and 3) symptomatic D-CAA mutation carriers versus controls. Mean values of venous length, venous tortuosity, absolute venous homogeneity, and venous density were entered as dependent variable.
To assess the correlation between DMV parameters and SVD markers, univariate general linear modeling was used. Dependent variables were the same as mentioned previously, SVD MRI-markers were entered as either a fixed variable or covariate depending on the data. The correlation assessments were done within D-CAA mutation carriers and adjusted for age, sex, and TBV% ICV. To adjust for multiple testing, we divided an α-value of 0.05 by the number of tests performed and considered a p-value <0.001 to be statistically significant. All statistical analysis were performed within SPSS (version 26.0).
RESULTS
Of the 60 persons initially included in the EDAN study, 41 were analyzed— see flowchart in Fig. 1; 16 D-CAA mutation carriers (8 pre-symptomatic [88% female]; mean age: 31.5 years, range 20–51, and 8 symptomatic [63% female]; mean age: 54.9 years, range 45–63), and 25 controls [60% female]; mean age: 45.6 years, range 30–67). In 4 symptomatic D-CAA patients, one hemisphere was excluded from analysis, because the presence of intracerebral hemorrhages precluded DMV detection; in these 4 patients only the contralateral hemisphere was used for DMV analysis.
Fig. 1
Flowchart of inclusion of patients.
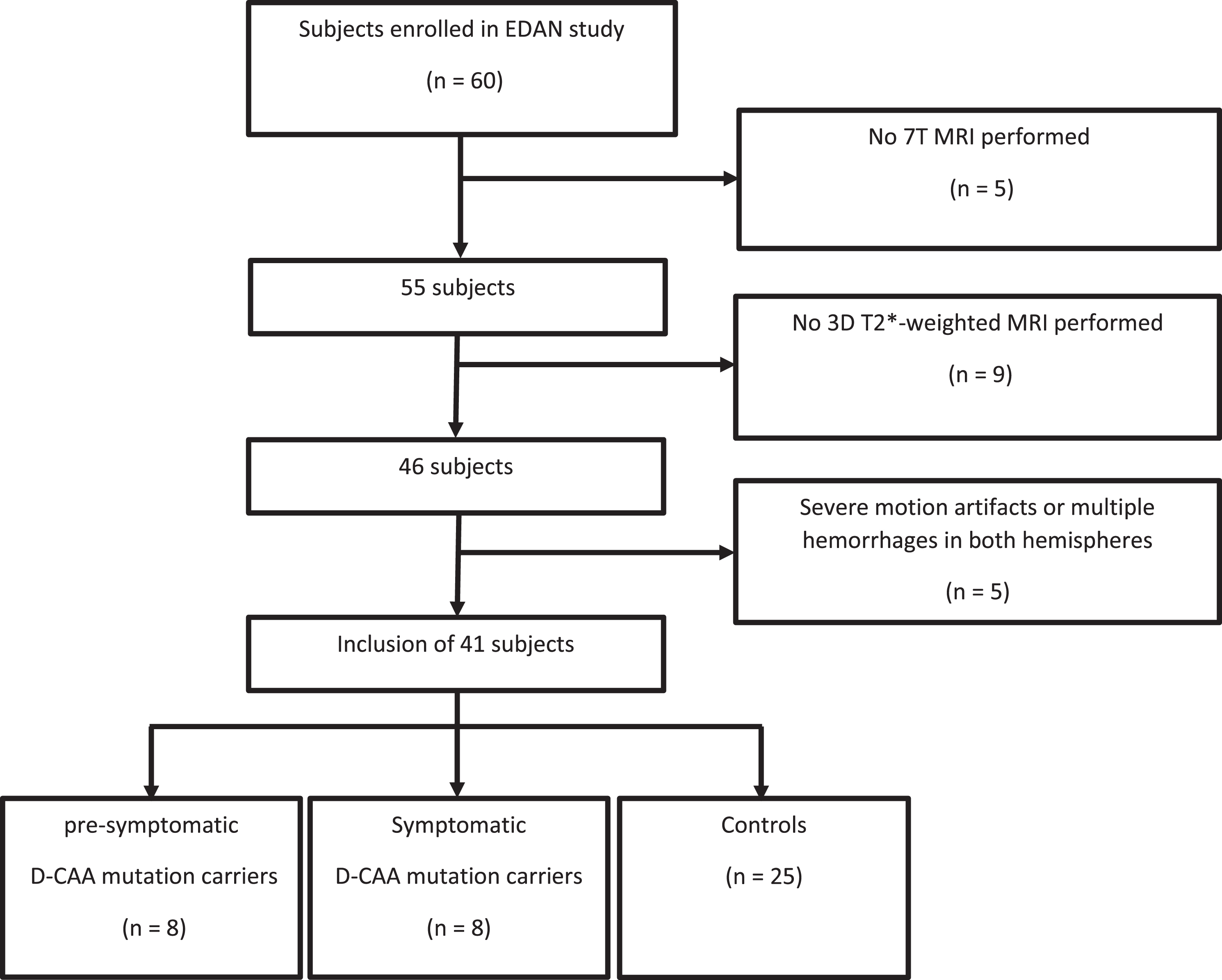
DMV parameters between D-CAA mutation carriers and controls
The mean values of DMV parameters for D-CAA mutation carriers and controls are shown in Table 1. Mean venous length did not differ between controls (14.7 mm, 95% confidence interval (CI) 14.3–16.1), pre-symptomatic D-CAA mutation carriers (14.3 mm, 95% confidence interval (CI) 12.6–16.1) and symptomatic D-CAA mutation carriers (13.4 mm, 95% CI 11.6–15.2). Also, mean tortuosity (controls 1.09, 95% CI 1.08–1.10; pre-symptomatic D-CAA mutation carriers 1.09, 95% CI 1.07–1.12; symptomatic D-CAA mutation carriers 1.09, 95% CI 1.07–1.10), mean absolute inhomogeneity (controls 30.5, 95% CI 28.4–32.5; pre-symptomatic D-CAA mutation carriers 26.6, 95% CI 23.7–29.5; symptomatic D-CAA mutation carriers 29.4, 95% CI 25.6–33.3) and mean venous density (controls 0.23 veins/cm3, 95% CI 0.20–0.26; pre-symptomatic D-CAA mutation carriers 0.21 veins/cm3, 95% CI 0.18–0.24; symptomatic D-CAA mutation carriers 0.23 veins/cm2, 95% CI 0.18–0.28) was not different between groups. No association between age and any DMV parameter was found in any of the 3 groups.
Table 1
Demographics and DMV parameters for D-CAA mutation carriers and controls
D-CAA mutation carriers (n = 16) | |||||||
Controls (n = 25) | All | p versus controls | Pre-symptomatic (n = 8) | p versus controls | Symptomatic (n = 8) | p versus controls | |
Age (y) | 45.6±11.8 | 43.2±15.7 | 0.13 | 31.5±13.2 | 0.15 | 54.9±6.3 | 0.02 |
Sex | |||||||
Male, n (%) | 10 (40) | 4 (25) | 0.33 | 1 (1.5) | 0.16 | 3 (37.5) | 0.90 |
Female, n (%) | 15 (60) | 12 (75) | 7 (87.5) | 5 (62.5) | |||
DMV parameters | |||||||
Length (mm) | 14.7±1.9 | 13.9±2.1 | 0.52 | 14.3±2.1 | 0.43 | 13.4±2.1 | 0.52 |
Tortuosity | 1.09±0.02 | 1.09±0.03 | 0.86 | 1.09±0.03 | 0.69 | 1.09±0.02 | 0.99 |
Absolute inhomogeneity | 30.5±4.9 | 28.0±4.2 | 0.75 | 26.6±3.5 | 0.95 | 29.4±4.6 | 0.80 |
Density (veins/cm3) | 0.23±0.07 | 0.22±0.05 | 0.61 | 0.21±0.04 | 0.82 | 0.23±0.62 | 0.33 |
Mean values±standard deviations are given, group means were compared. For sex the number and % within each group are given. A Chi-Square test was used to report p values for sex. Reported p values for DMV parameters were obtained by using a univariate general linear model. DMV, deep medullary vein; D-CAA, Dutch-type cerebral amyloid angiopathy.
Relation between quantitative DMV parameters and MRI markers associated with D-CAA
The mean values of MRI markers associated with D-CAA in D-CAA mutation carriers and controls are shown in Table 2. In D-CAA mutation carriers, venous parameters did not correlate with any non-hemorrhagic or hemorrhagic SVD markers on MRI; further, no association was present with DTI parameters (FA, MD, AD, RD) or grey matter perfusion values.
Table 2
Small vessel disease markers on MRI in D-CAA mutation carriers and controls
D-CAA mutation carriers (n = 16) | ||||
Controls (n = 25) | All | Pre-symptomatic (n = 8) | Symptomatic (n = 8) | |
Microbleeds >2, n | – | 9 | 1 | 8 |
ICH >1, n | – | 8 | – | 8 |
cSAH >1, n | – | 5 | 1 | 4 |
Presence cSS, n | – | 7 | – | 7 |
Cortical microinfarct >1, n | 1 | 8 | 0 | 8 |
WMH volume, cm3 | 2.90±2.85 | 17.12±20.94 | 2.53±1.13 | 33.80±20.32 |
Dilated PVS volume, cm3 | 0.02±0.02 | 0.05±0.44 | 0.02±0.03 | 0.08±0.04 |
Lacunar infarcts >1, n | 7 | 2 | 2 | – |
GM perfusion, mL/min/100 g brain tissue | 60.6±13.2 | 59.7±12.4 | 65.1±5.6 | 54.4±15.2 |
FA | 0.43±0.02 | 0.40±0.04 | 0.43±0,01 | 0.37±0.04 |
MD, ×10–4, 1000 s/mm2 | 7.37±0.22 | 8.03±1.01 | 7.34±0.16 | 8.71±1.04 |
AD, ×10–3, 1000 s/mm2 | 1.11±0.02 | 1.16±0.09 | 1.10±0.02 | 1.22±0.02 |
RD, ×10–4, 1000 s/mm2 | 8.80±0.21 | 9.44±0.97 | 8.78±0.15 | 10.10±1.0 |
Normalized GM, cm3 | 658±58 | 661±76 | 715±55 | 600±42 |
Total ICV, cm3 | 1543±183 | 1554±220 | 1477±236 | 1631±185 |
Ventricular volume, cm3 | 32±9 | 38±16 | 28±9 | 48±16 |
Ventricular volume % ICV | 2.0±0.5 | 2.4±0.9 | 1.9±0.4 | 2.9±0.9 |
TBV % ICV | 77±6 | 77±10 | 83±12 | 72±5 |
Mean values±standard deviations are given; n are the participants scoring >1 or >2 for the given parameters. WMH, white matter hyperintensities, natural logarithm taken from original values for normal distribution; ICH, intracerebral hemorrhage; cSAH, convexity subarachnoid hemorrhage; cSS, cortical superficial siderosis; PVS, perivascular spaces; GM, grey matter; FA, fractional anisotropy; MD, mean diffusivity; AD, axial diffusivity; RD, radial diffusivity; ICV, intracranial volume; TBV, total brain volume. Total ICV values were scaled to a standardized brain, the scaling factor was used to calculate the values.
Fig. 2
Examples of transversal 6 mm thick maximum intensity projections of 7T T2*-weighted sequence with tracked veins displayed in green (A–C) and corresponding 3D-rendering of the deep medullary veins (DMVs) with the expanded surface shown in white and the reconstructed veins in yellow (D–F). Although mean venous length of the 56-year-old control subject (A, D) was higher as compared to the 51-year-old pre-symptomatic (B, E) and 55-year-old symptomatic (C, F) D-CAA mutation carrier (18.4 mm, 16.1 mm and 16.3 mm, resp), this difference was not statistically significant between groups. Also, mean venous density was higher in this control subject (0.32) as compared to the pre-symptomatic (0.24) and symptomatic (0.25) D-CAA mutation carriers, although this difference was also not significant between groups.
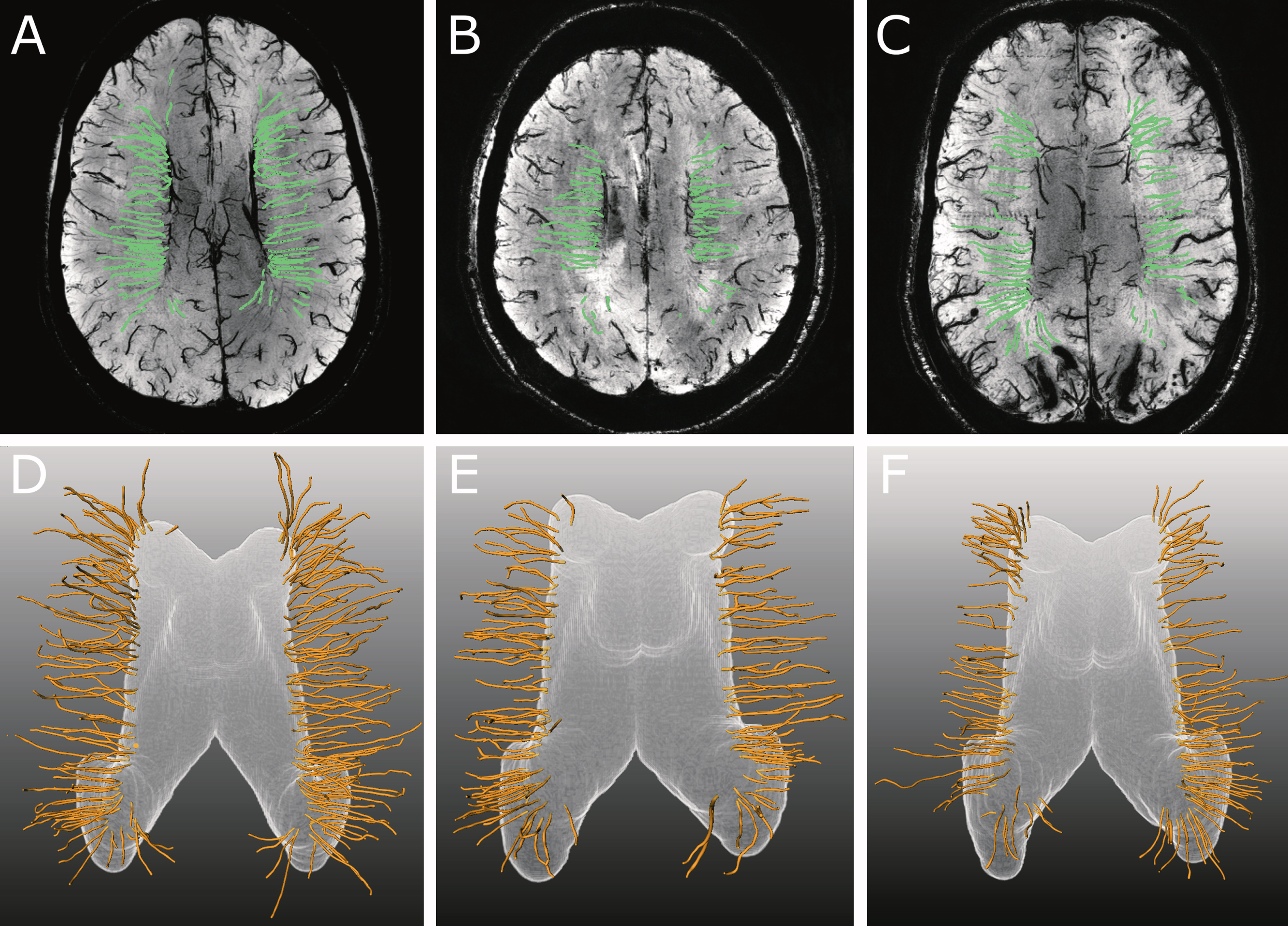
DISCUSSION
We did not observe a difference in DMV length, tortuosity, homogeneity, and density between D-CAA mutation carriers and controls. Also, no differences in DMV parameters were found between pre-symptomatic and symptomatic D-CAA mutation carriers. Secondly, in D-CAA mutation carriers no association was found between DMV parameters and MRI markers associated with D-CAA.
Several studies have suggested that arterial and/or venular Aβ depositions affect the cerebral vasculature in AD patients, including DMVs [9, 20]. Arterial Aβ deposition has been shown to stiffen vessels and thereby increases pulsatility, causing physical stress on venules. DMVs are suggested to be increasingly susceptible to stress because of their lower baseline resistance. Venular Aβ deposition has been suggested to impair Aβ clearance, similar to arterial Aβ deposition, and is suggested to be an integral part of AD pathogenesis [20]. Although we acknowledge that only a small number of individuals were included in our study, our results did not indicate a clear association between MRI markers of CAA pathology in the cortex and venous pathology in the white matter and this study therefore does not support the hypothesis that concomitant CAA pathology might be a contributing factor to DMV changes in patients with AD.
In contrast to findings in patients with non-amyloid cerebral SVD [3–6, 21], we did not find an association between DMV parameters and non-hemorrhagic and hemorrhagic markers on MRI in D-CAA mutation carriers; more specifically, a relation with WMH volume was not present. Our results suggest that in patients with CAA, a remote CAA-induced change of DMVs do not play a role in the pathogenesis of WMH or other non-hemorrhagic or hemorrhagic markers on MRI. However, considering the typical posterior predominance of WMH in patients with CAA together with the possible relationship between WMH and DMV parameters [4–6], the relationship between posterior WMH and DMV parameters could be addressed further in patients with CAA in future studies. No association was present with DTI parameters or cortical grey matter perfusion values. DMV inhomogeneity has been associated with FA of DMVs drainage area in cerebral SVD patients [22] and number of DMVs with FA and MD of the white matter in a population-based cohort [23]. Our results, however, do not indicate that DMVs play a role in white matter microstructural integrity loss in patients with CAA.
A major limitation of this study is the small sample size. This is inevitably coupled to the relatively small number of D-CAA mutation carriers living in the Netherlands and subsequently only a small number of individuals was included in the original EDAN study. In addition, we had to exclude 5 participants from analysis because of severe motion artifacts and/or presence of multiple large intracerebral hemorrhages, resulting in a further decrease of the sample size.
Bouvy and coworkers [9] found in only a slightly larger group size a significant difference in venous tortuosity in the early AD population as compared to controls, whereas in our study venous tortuosity was similar in all our 3 groups. In the study of Bouvy and coworkers, difference in SVD pathology was more than a factor 3 smaller as concluded from the smaller difference in WMH volumes between groups. We therefore assume that, although the number of participants was small, we would have found a significant difference when venous tortuosity would be an important factor in D-CAApathology.
A strength of our study is that we used a validated technique for the automated detection and quantification of DMVs on T2*-weighted 7T MRI [18]. Although our sample size is small, the study is performed in a unique population that is considered a ‘pure’ model for sporadic CAA.
In conclusion, this study showed that quantitative DMV parameters did not differ between D-CAA mutation carriers and controls. In patients with CAA, DMVs likely do not play a role in the pathogenesis of SVD MRI markers associated with D-CAA.
ACKNOWLEDGMENTS
This study was supported by the National Institutes of Health grant R01 NS070834, by the Dutch Heart Foundation and the Netherlands Organisation for Scientific Research (NWO), as part of their joint strategic research programme: “Earlier recognition of cardiovascular diseases”. This project is partially financed by the PPP Allowance made available by Top Sector Life Sciences & Health to the Dutch Heart foundation to stimulate public-private partnerships, and by a Clinical Established Investigator grant of the Netherlands Heart Foundation 2016T86 Dr Wermer
Authors’ disclosures available online (https://www.j-alz.com/manuscript-disclosures/22-0354r1).
REFERENCES
[1] | Moody DM , Brown WR , Challa VR , Anderson RL ((1995) ) Periventricular venous collagenosis - association with leukoaraiosis . Radiology 194: , 469–476. |
[2] | Keith J , Gao FQ , Noor R , Kiss A , Balasubramaniam G , Au K , Rogaeva E , Masellis M , Black SE ((2017) ) Collagenosis of the deep medullary veins: An underrecognized pathologic correlate of white matter hyperintensities and periventricular infarction? J Neuropathol Expl Neurol 76: , 299–312. |
[3] | De Guio F , Vignaud A , Ropele S , Duering M , Duchesnay E , Chabriat H , Jouvent E ((2014) ) Loss of venous integrity in cerebral small vessel disease: A 7-T MRI Study in cerebral autosomal-dominant arteriopathy with subcortical infarcts and leukoencephalopathy (CADASIL). Stroke 45: , 2124–2126. |
[4] | Chen XD , Wei L , Wang JH , Shan YL , Cai W , Men XJ , Liu SX , Kang Z , Lu ZQ , Mok VCT , Wu AM ((2020) ) Decreased visible deep medullary veins is a novel imaging marker for cerebral small vessel disease. Neurol Sci 41: , 1497–1506. |
[5] | Xu ZH , Li FF , Wang B , Xing DX , Pei YS , Yang BQ , Duan Y ((2020) ) New insights in addressing cerebral small vessel disease: Association with the deep medullary veins. Front Aging Neurosci 12: , 597799. |
[6] | Zhang RT , Zhou Y , Yan SQ , Zhong GL , Liu C , Jiaerken YF , Song RR , Yu XF , Zhang MM , Lou M ((2017) ) A brain region-based deep medullary veins visual score on susceptibility weighted imaging. Front Aging Neurosci 9: , 269. |
[7] | Ao DH , Zhang DD , Zhai FF , Zhang JT , Han F , Li ML , Ni J , Yao M , Zhang SY , Cui LY , Jin ZY , Zhou LX , Zhu YC ((2021) ) Brain deep medullary veins on 3-T MRI in a population-based cohort. J Cereb Blood Flow Metab 41: , 561–568. |
[8] | Nan D , Cheng YY , Feng LS , Zhao MM , Ma D , Feng JC ((2019) ) Potential mechanism of venous system for leukoaraiosis: From post-mortem to in vivo research. Neurodegener Dis 19: , 101–108. |
[9] | Bouvy WH , Kuijf HJ , Zwanenburg JJM , Koek HL , Kappelle LJ , Luijten PR , Ikram MK , Biessels GJ , Cognitive UV ((2017) ) Abnormalities of cerebral deep medullary veins on 7 Tesla MRI in amnestic mild cognitive impairment and early Alzheimer’s disease: A pilot study.. J Alzheimers Dis 57: , 705–710. |
[10] | Jakel L , De Kort AM , Klijn CJM , Schreuder FHBM , Verbeek MM ((2022) ) Prevalence of cerebral amyloid angiopathy: A systematic review and meta-analysis. Alzheimers Dement 18: , 10–28. |
[11] | Attems J , Lauda F , Jellinger KA ((2008) ) Unexpectedly low prevalence of intracerebral hemorrhages in sporadic cerebral amyloid angiopathy - An autopsy study. J Neurol 255: , 70–76. |
[12] | Thal DR , Ghebremedhin E , Rub U , Yamaguchi H , Del Tredici K , Braak H ((2002) ) Two types of sporadic cerebral amyloid angiopathy. J Neuropathol Exp Neurol 61: , 282–293. |
[13] | Zhang-Nunes SX , Maat-Schieman MLC , van Duinen SG , Roos RAC , Frosch MP , Greenberg SM ((2006) ) The cerebral beta-amyloid angiopathies: Hereditary and sporadic. Brain Pathol 16: , 30–39. |
[14] | van Rooden S , van Opstal AM , Labadie G , Terwindt GM , Wermer MJH , Webb AG , Middelkoop HAM , Greenberg SM , van der Grond J , van Buchem MA ((2016) ) Early magnetic resonance imaging and cognitive markers of hereditary cerebral amyloid angiopathy. Stroke 47: , 3041–3044. |
[15] | van Veluw SJ , Biessels GJ , Luijten PR , Zwanenburg JJM ((2015) ) Assessing cortical cerebral microinfarcts on high resolution MR images. J Vis Exp 105: , 1–8. |
[16] | van Opstal AM , van Rooden S , van Harten T , Ghariq E , Labadie G , Fotiadis P , Gurol ME , Terwindt GM , Wermer MJH , van Buchem MA , Greenberg SM , van der Grond J ((2017) ) Cerebrovascular function in presymptomatic and symptomatic individuals with hereditary cerebral amyloid angiopathy: A case-control study. Lancet Neurol 16: , 115–122. |
[17] | Schouten TM , de Vos F , van Rooden S , Bouts MJRJ , van Opstal AM , Feis RA , Terwindt GM , Wermer MJH , van Buchem MA , Greenberg SM , de Rooij M , Rombouts SARB , van der Grond J ((2019) ) Multiple approaches todiffusion magnetic resonance imaging in hereditary cerebral amyloidangiopathy mutation carriers. J Am Heart Assoc 8: , e011288. |
[18] | Kuijf HJ , Bouvy WH , Zwanenburg JJM , Schultz TBR , Viergever MA , Vincken KL , Biessels GJ ((2016) ) Quantification of deep medullary veins at 7 T brain MRI. Eur Radiol 26: , 3412–3418. |
[19] | Martinez-Ramirez S , van Rooden S , Charidimou A , van Opstal AM , Wermer M , Gurol ME , Terwindt G , van der Grond J , Greenberg SM , van Buchem M , Viswanathan A ((2018) ) Perivascular spaces volume in sporadic and hereditary (Dutch-type) cerebral amyloid angiopathy. Stroke 49: , 1913–1919. |
[20] | Morrone CD , Bishay J , McLaurin J ((2020) ) Potential role of venular amyloid in Alzheimer’s disease pathogenesis. Int J Mol Sci 21: , 1985. |
[21] | Zhou Y , Li QQ , Zhang RT , Zhang WH , Yan SQ , Xu JJ , Wang SY , Zhang MM , Lou M ((2020) ) Role of deep medullary veins in pathogenesis of lacunes: Longitudinal observations from the CIRCLE study. J Cereb Blood Flow Metab 40: , 1797–1805. |
[22] | Zhang RT , Huang PY , Jiaerken Y , Wang SY , Hong H , Luo X , Xu XP , Yu XF , Li KC , Zeng QZ , Wu X , Lou M , Zhang MM ((2021) ) Venous disruption affects white matter integrity through increased interstitial fluid in cerebral small vessel disease. J Cereb Blood Flow Metab 41: , 157–165. |
[23] | Liu ZY , Zhai FF , Ao DH , Han F , Li ML , Zhou LX , Ni J , Yao M , Zhang SY , Cui LY , Jin ZY , Zhu YC ((2021) ) Deep medullary veins are associated with widespread brain structural abnormalities. J Cereb Blood Flow Metab 41: , 1–10. |